Some Problems In Model Selection
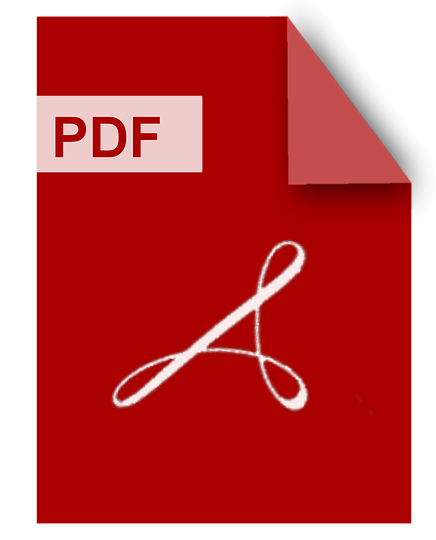
DOWNLOAD
Download Some Problems In Model Selection PDF/ePub or read online books in Mobi eBooks. Click Download or Read Online button to get Some Problems In Model Selection book now. This website allows unlimited access to, at the time of writing, more than 1.5 million titles, including hundreds of thousands of titles in various foreign languages. If the content not found or just blank you must refresh this page
Some Problems In Model Selection
DOWNLOAD
Author : Chenlei Leng
language : en
Publisher:
Release Date : 2004
Some Problems In Model Selection written by Chenlei Leng and has been published by this book supported file pdf, txt, epub, kindle and other format this book has been release on 2004 with categories.
Model Selection And Error Estimation In A Nutshell
DOWNLOAD
Author : Luca Oneto
language : en
Publisher: Springer
Release Date : 2019-07-17
Model Selection And Error Estimation In A Nutshell written by Luca Oneto and has been published by Springer this book supported file pdf, txt, epub, kindle and other format this book has been release on 2019-07-17 with Technology & Engineering categories.
How can we select the best performing data-driven model? How can we rigorously estimate its generalization error? Statistical learning theory answers these questions by deriving non-asymptotic bounds on the generalization error of a model or, in other words, by upper bounding the true error of the learned model based just on quantities computed on the available data. However, for a long time, Statistical learning theory has been considered only an abstract theoretical framework, useful for inspiring new learning approaches, but with limited applicability to practical problems. The purpose of this book is to give an intelligible overview of the problems of model selection and error estimation, by focusing on the ideas behind the different statistical learning theory approaches and simplifying most of the technical aspects with the purpose of making them more accessible and usable in practice. The book starts by presenting the seminal works of the 80’s and includes the most recent results. It discusses open problems and outlines future directions for research.
Econometric Analysis Of Model Selection And Model Testing
DOWNLOAD
Author : M. Ishaq Bhatti
language : en
Publisher: Routledge
Release Date : 2017-03-02
Econometric Analysis Of Model Selection And Model Testing written by M. Ishaq Bhatti and has been published by Routledge this book supported file pdf, txt, epub, kindle and other format this book has been release on 2017-03-02 with Business & Economics categories.
In recent years econometricians have examined the problems of diagnostic testing, specification testing, semiparametric estimation and model selection. In addition researchers have considered whether to use model testing and model selection procedures to decide the models that best fit a particular dataset. This book explores both issues with application to various regression models, including the arbitrage pricing theory models. It is ideal as a reference for statistical sciences postgraduate students, academic researchers and policy makers in understanding the current status of model building and testing techniques.
The Robustness Of Model Selection Rules
DOWNLOAD
Author : Jochen A. Jungeilges
language : en
Publisher: LIT Verlag Münster
Release Date : 1992
The Robustness Of Model Selection Rules written by Jochen A. Jungeilges and has been published by LIT Verlag Münster this book supported file pdf, txt, epub, kindle and other format this book has been release on 1992 with Business & Economics categories.
The Problem Of Model Selection And Scientific Realism
DOWNLOAD
Author : Stanislav Larski
language : en
Publisher:
Release Date : 2012
The Problem Of Model Selection And Scientific Realism written by Stanislav Larski and has been published by this book supported file pdf, txt, epub, kindle and other format this book has been release on 2012 with categories.
This thesis has two goals. Firstly, we consider the problem of model selection for the purposes of prediction. In modern science predictive mathematical models are ubiquitous and can be found in such diverse fields as weather forecasting, economics, ecology, mathematical psychology, sociology, etc. It is often the case that for a given domain of inquiry there are several plausible models, and the issue then is how to discriminate between them - this is the problem of model selection. We consider approaches to model selection that are used in classical [also known as frequentist] statistics, and fashionable in recent years methods of Akaike Information Criterion [AIC] and Bayes Information Criterion [BIC], the latter being a part of a broader Bayesian approach. We show the connection between AIC and BIC, and provide comparison of performance of these methods. Secondly, we consider some philosophical arguments that arise within the setting of the model selection approaches investigated in the first part. These arguments aim to provide counterexamples to the epistemic thesis of scientific realism, viz., that predictively successful scientific theories are approximately true, and to the idea that truth and predictive accuracy go together. We argue for the following claims: 1) that none of the criticisms brought forward in the philosophical literature against the AIC methodology are devastating, and AIC remains a viable method of model selection; 2) that the BIC methodology likewise survives the numerous criticisms; 3) that the counterexamples to scientific realism that ostensibly arise within the framework of model selection are flawed; 4) that in general the model selection methods discussed in this thesis are neutral with regards to the issue of scientific realism; 5) that a plurality of methodologies should be applied to the problem of model selection with full awareness of the foundational issues that each of these methodologies has.
Model Selection And Inference
DOWNLOAD
Author : Kenneth P. Burnham
language : en
Publisher: Springer Science & Business Media
Release Date : 2013-11-11
Model Selection And Inference written by Kenneth P. Burnham and has been published by Springer Science & Business Media this book supported file pdf, txt, epub, kindle and other format this book has been release on 2013-11-11 with Mathematics categories.
Statisticians and applied scientists must often select a model to fit empirical data. This book discusses the philosophy and strategy of selecting such a model using the information theory approach pioneered by Hirotugu Akaike. This approach focuses critical attention on a priori modeling and the selection of a good approximating model that best represents the inference supported by the data. The book includes practical applications in biology and environmental science.
Model Selection And Multimodel Inference
DOWNLOAD
Author : Kenneth P. Burnham
language : en
Publisher: Springer Science & Business Media
Release Date : 2003-12-04
Model Selection And Multimodel Inference written by Kenneth P. Burnham and has been published by Springer Science & Business Media this book supported file pdf, txt, epub, kindle and other format this book has been release on 2003-12-04 with Mathematics categories.
A unique and comprehensive text on the philosophy of model-based data analysis and strategy for the analysis of empirical data. The book introduces information theoretic approaches and focuses critical attention on a priori modeling and the selection of a good approximating model that best represents the inference supported by the data. It contains several new approaches to estimating model selection uncertainty and incorporating selection uncertainty into estimates of precision. An array of examples is given to illustrate various technical issues. The text has been written for biologists and statisticians using models for making inferences from empirical data.
Problems In Generalized Linear Model Selection And Predictive Evaluation For Binary Outcomes
DOWNLOAD
Author : Patrick Ten Eyck
language : en
Publisher:
Release Date : 2015
Problems In Generalized Linear Model Selection And Predictive Evaluation For Binary Outcomes written by Patrick Ten Eyck and has been published by this book supported file pdf, txt, epub, kindle and other format this book has been release on 2015 with Forecasting categories.
This manuscript consists of three papers which formulate novel generalized linear model methodologies. In Chapter 1, we introduce a variant of the traditional concordance statistic that is associated with logistic regression. This adjusted c − statistic as we call it utilizes the differences in predicted probabilities as weights for each event/non- event observation pair. We highlight an extensive comparison of the adjusted and traditional c-statistics using simulations and apply these measures in a modeling application. In Chapter 2, we feature the development and investigation of three model selection criteria based on cross-validatory c-statistics: Model Misspecification Pre- diction Error, Fitting Sample Prediction Error, and Sum of Prediction Errors. We examine the properties of the corresponding selection criteria based on the cross- validatory analogues of the traditional and adjusted c-statistics via simulation and illustrate these criteria in a modeling application. In Chapter 3, we propose and investigate an alternate approach to pseudo- likelihood model selection in the generalized linear mixed model framework. After outlining the problem with the pseudo-likelihood model selection criteria found using the natural approach to generalized linear mixed modeling, we feature an alternate approach, implemented using a SAS macro, that obtains and applies the pseudo-data from the full model for fitting all candidate models. We justify the propriety of the resulting pseudo-likelihood selection criteria using simulations and implement this new method in a modeling application.
Using The Penalized Likelihood Method For Model Selection With Nuisance Parameters Present Only Under The Alternative
DOWNLOAD
Author : Arie Preminger
language : en
Publisher:
Release Date : 2005
Using The Penalized Likelihood Method For Model Selection With Nuisance Parameters Present Only Under The Alternative written by Arie Preminger and has been published by this book supported file pdf, txt, epub, kindle and other format this book has been release on 2005 with categories.
We study the problem of model selection with nuisance parameters present only under the alternative. The common approach for testing in this case is to determine the true model through the use of some functionals over the nuisance parameters space. Since in such cases the distribution of these statistics is not known, critical values had to be approximated usually through computationally intensive simulations. Furthermore, the computed critical values are data and model dependent and hence cannot be tabulated. We address this problem by using the penalized likelihood method to choose the correct model. We start by viewing the likelihood ratio as a function of the unidentified parameters. By using the empirical process theory and the uniform law of the iterated logarithm (LIL) together with sufficient conditions on the penalty term, we derive the consistency properties of this method. Our approach generates a simple and consistent procedure for model selection. This methodology is presented in the context of switching regression models. We also provide some Monte Carlo simulations to analyze the finite sample performance of our procedure.
Model Selection
DOWNLOAD
Author : H. Linhart
language : en
Publisher:
Release Date : 1986-11-19
Model Selection written by H. Linhart and has been published by this book supported file pdf, txt, epub, kindle and other format this book has been release on 1986-11-19 with Mathematics categories.
The first work to deal exclusively with objective criteria for comparing statistical models. Using a simple framework, it outlines a general strategy for selecting a model and applies this strategy to develop methods useful for solving specific selection problems. Topics covered include histograms, univariate distributions, simple and multiple regression, the analysis of variance and covariance, the analysis of proportions and contingency tables, time series analysis, and spatial analysis.