Problems In Generalized Linear Model Selection And Predictive Evaluation For Binary Outcomes
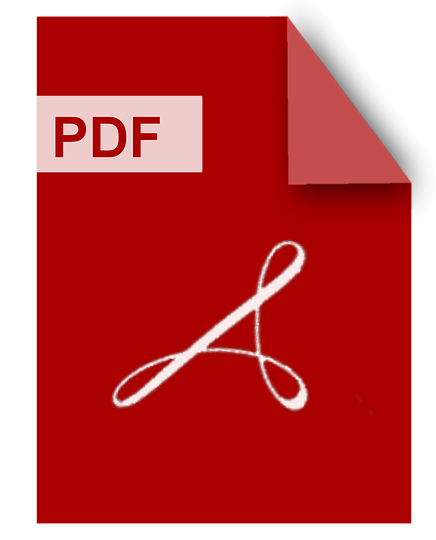
DOWNLOAD
Download Problems In Generalized Linear Model Selection And Predictive Evaluation For Binary Outcomes PDF/ePub or read online books in Mobi eBooks. Click Download or Read Online button to get Problems In Generalized Linear Model Selection And Predictive Evaluation For Binary Outcomes book now. This website allows unlimited access to, at the time of writing, more than 1.5 million titles, including hundreds of thousands of titles in various foreign languages. If the content not found or just blank you must refresh this page
Problems In Generalized Linear Model Selection And Predictive Evaluation For Binary Outcomes
DOWNLOAD
Author : Patrick Ten Eyck
language : en
Publisher:
Release Date : 2015
Problems In Generalized Linear Model Selection And Predictive Evaluation For Binary Outcomes written by Patrick Ten Eyck and has been published by this book supported file pdf, txt, epub, kindle and other format this book has been release on 2015 with Forecasting categories.
This manuscript consists of three papers which formulate novel generalized linear model methodologies. In Chapter 1, we introduce a variant of the traditional concordance statistic that is associated with logistic regression. This adjusted c − statistic as we call it utilizes the differences in predicted probabilities as weights for each event/non- event observation pair. We highlight an extensive comparison of the adjusted and traditional c-statistics using simulations and apply these measures in a modeling application. In Chapter 2, we feature the development and investigation of three model selection criteria based on cross-validatory c-statistics: Model Misspecification Pre- diction Error, Fitting Sample Prediction Error, and Sum of Prediction Errors. We examine the properties of the corresponding selection criteria based on the cross- validatory analogues of the traditional and adjusted c-statistics via simulation and illustrate these criteria in a modeling application. In Chapter 3, we propose and investigate an alternate approach to pseudo- likelihood model selection in the generalized linear mixed model framework. After outlining the problem with the pseudo-likelihood model selection criteria found using the natural approach to generalized linear mixed modeling, we feature an alternate approach, implemented using a SAS macro, that obtains and applies the pseudo-data from the full model for fitting all candidate models. We justify the propriety of the resulting pseudo-likelihood selection criteria using simulations and implement this new method in a modeling application.
Generalized Linear Models For Insurance Rating
DOWNLOAD
Author : Mark Goldburd
language : en
Publisher:
Release Date : 2016-06-08
Generalized Linear Models For Insurance Rating written by Mark Goldburd and has been published by this book supported file pdf, txt, epub, kindle and other format this book has been release on 2016-06-08 with categories.
Alternative Methods For Variable Selection In Generalized Linear Models With Binary Outcomes And Incomplete Covariates
DOWNLOAD
Author : Gang Liu
language : en
Publisher:
Release Date : 2007
Alternative Methods For Variable Selection In Generalized Linear Models With Binary Outcomes And Incomplete Covariates written by Gang Liu and has been published by this book supported file pdf, txt, epub, kindle and other format this book has been release on 2007 with categories.
Generalized Linear Models
DOWNLOAD
Author : P. McCullagh
language : en
Publisher: Routledge
Release Date : 2019-01-22
Generalized Linear Models written by P. McCullagh and has been published by Routledge this book supported file pdf, txt, epub, kindle and other format this book has been release on 2019-01-22 with Mathematics categories.
The success of the first edition of Generalized Linear Models led to the updated Second Edition, which continues to provide a definitive unified, treatment of methods for the analysis of diverse types of data. Today, it remains popular for its clarity, richness of content and direct relevance to agricultural, biological, health, engineering, and ot
Applying Generalized Linear Models
DOWNLOAD
Author : James K. Lindsey
language : en
Publisher: Springer Science & Business Media
Release Date : 2008-01-15
Applying Generalized Linear Models written by James K. Lindsey and has been published by Springer Science & Business Media this book supported file pdf, txt, epub, kindle and other format this book has been release on 2008-01-15 with Mathematics categories.
This book describes how generalised linear modelling procedures can be used in many different fields, without becoming entangled in problems of statistical inference. The author shows the unity of many of the commonly used models and provides readers with a taste of many different areas, such as survival models, time series, and spatial analysis, and of their unity. As such, this book will appeal to applied statisticians and to scientists having a basic grounding in modern statistics. With many exercises at the end of each chapter, it will equally constitute an excellent text for teaching applied statistics students and non- statistics majors. The reader is assumed to have knowledge of basic statistical principles, whether from a Bayesian, frequentist, or direct likelihood point of view, being familiar at least with the analysis of the simpler normal linear models, regression and ANOVA.
Fundamentals Of Clinical Data Science
DOWNLOAD
Author : Pieter Kubben
language : en
Publisher: Springer
Release Date : 2018-12-21
Fundamentals Of Clinical Data Science written by Pieter Kubben and has been published by Springer this book supported file pdf, txt, epub, kindle and other format this book has been release on 2018-12-21 with Medical categories.
This open access book comprehensively covers the fundamentals of clinical data science, focusing on data collection, modelling and clinical applications. Topics covered in the first section on data collection include: data sources, data at scale (big data), data stewardship (FAIR data) and related privacy concerns. Aspects of predictive modelling using techniques such as classification, regression or clustering, and prediction model validation will be covered in the second section. The third section covers aspects of (mobile) clinical decision support systems, operational excellence and value-based healthcare. Fundamentals of Clinical Data Science is an essential resource for healthcare professionals and IT consultants intending to develop and refine their skills in personalized medicine, using solutions based on large datasets from electronic health records or telemonitoring programmes. The book’s promise is “no math, no code”and will explain the topics in a style that is optimized for a healthcare audience.
Flexible Semi And Non Parametric Modelling And Prognosis For Discrete Outcomes
DOWNLOAD
Author : Harald Binder
language : en
Publisher:
Release Date : 2006
Flexible Semi And Non Parametric Modelling And Prognosis For Discrete Outcomes written by Harald Binder and has been published by this book supported file pdf, txt, epub, kindle and other format this book has been release on 2006 with categories.
This thesis presents new models and estimation techniques based on the generalized linear model framework. In Chapter 1 the GAMBoost procedure for estimation of generalized additive models is developed. Based on boosting and gradient descent in function space it generalizes the notion of repeated fitting of residuals to exponential family responses. By using a flexible number of updates for each covariate the selection of smoothing parameters is reduced to the selection of the number of boosting steps. The latter is chosen based on approximate effective degrees of freedom. The resulting procedure shows good performance for a wide variety of examples with binary and Poisson response data. A considerable advantage compared to other procedures is found for a large number of covariates and a low level of information. An application to real data is presented. In Chapter 2 a flexible model for discrete time survival data is developed that allows for non-linear covariate effects that vary over time. For estimation an iterative two-step procedure based on Fisher scoring is given. A simulation study with various levels of complexity underlying the data compares the performance of adequate models to the performance of models that are too restrictive, too flexible or that provide the wrong kind of flexibility. It is shown that effective degrees of freedom work well as a basis for selection of smoothing parameters as well as for model selection. An example with real data is given. In Chapter 3 a technique for classification with binary response data is developed based on logistic regression. It uses local models with local selection of predictors for reduction of complexity and penalized estimation for numerical stability. Standard simulated data examples are used to evaluate components of the algorithm such as the kernel for local weight calculation, and to compare the performance to other procedures. It is found that the procedure is competitive for a wide range of examples and that selection of predictors is crucial for local quadratic models while being regulated rather well for local linear models. Good performance can also be seen for real data examples.
Practical Statistics For Data Scientists
DOWNLOAD
Author : Peter Bruce
language : en
Publisher: "O'Reilly Media, Inc."
Release Date : 2017-05-10
Practical Statistics For Data Scientists written by Peter Bruce and has been published by "O'Reilly Media, Inc." this book supported file pdf, txt, epub, kindle and other format this book has been release on 2017-05-10 with Computers categories.
Statistical methods are a key part of of data science, yet very few data scientists have any formal statistics training. Courses and books on basic statistics rarely cover the topic from a data science perspective. This practical guide explains how to apply various statistical methods to data science, tells you how to avoid their misuse, and gives you advice on what's important and what's not. Many data science resources incorporate statistical methods but lack a deeper statistical perspective. If you’re familiar with the R programming language, and have some exposure to statistics, this quick reference bridges the gap in an accessible, readable format. With this book, you’ll learn: Why exploratory data analysis is a key preliminary step in data science How random sampling can reduce bias and yield a higher quality dataset, even with big data How the principles of experimental design yield definitive answers to questions How to use regression to estimate outcomes and detect anomalies Key classification techniques for predicting which categories a record belongs to Statistical machine learning methods that “learn” from data Unsupervised learning methods for extracting meaning from unlabeled data
Discrepancy Based Algorithms For Best Subset Model Selection
DOWNLOAD
Author : Tao Zhang
language : en
Publisher:
Release Date : 2013
Discrepancy Based Algorithms For Best Subset Model Selection written by Tao Zhang and has been published by this book supported file pdf, txt, epub, kindle and other format this book has been release on 2013 with Akaike Information Criterion categories.
The selection of a best-subset regression model from a candidate family is a common problem that arises in many analyses. In best-subset model selection, we consider all possible subsets of regressor variables; thus, numerous candidate models may need to be fit and compared. One of the main challenges of best-subset selection arises from the size of the candidate model family: specifically, the probability of selecting an inappropriate model generally increases as the size of the family increases. For this reason, it is usually difficult to select an optimal model when best-subset selection is attempted based on a moderate to large number of regressor variables. Model selection criteria are often constructed to estimate discrepancy measures used to assess the disparity between each fitted candidate model and the generating model. The Akaike information criterion (AIC) and the corrected AIC (AICc) are designed to estimate the expected Kullback-Leibler (K-L) discrepancy. For best-subset selection, both AIC and AICc are negatively biased, and the use of either criterion will lead to overfitted models. To correct for this bias, we introduce a criterion AICi, which has a penalty term evaluated from Monte Carlo simulation. A multistage model selection procedure AICaps, which utilizes AICi, is proposed for best-subset selection. In the framework of linear regression models, the Gauss discrepancy is another frequently applied measure of proximity between a fitted candidate model and the generating model. Mallows' conceptual predictive statistic (Cp) and the modified Cp (MCp) are designed to estimate the expected Gauss discrepancy. For best-subset selection, Cp and MCp exhibit negative estimation bias. To correct for this bias, we propose a criterion CPSi that again employs a penalty term evaluated from Monte Carlo simulation. We further devise a multistage procedure, CPSaps, which selectively utilizes CPSi. In this thesis, we consider best-subset selection in two different modeling frameworks: linear models and generalized linear models. Extensive simulation studies are compiled to compare the selection behavior of our methods and other traditional model selection criteria. We also apply our methods to a model selection problem in a study of bipolar disorder.
Generalized Linear Mixed Models
DOWNLOAD
Author : Charles E. McCulloch
language : en
Publisher: IMS
Release Date : 2003
Generalized Linear Mixed Models written by Charles E. McCulloch and has been published by IMS this book supported file pdf, txt, epub, kindle and other format this book has been release on 2003 with Mathematics categories.
Wiley Series in Probability and Statistics A modern perspective on mixed models The availability of powerful computing methods in recent decades has thrust linear and nonlinear mixed models into the mainstream of statistical application. This volume offers a modern perspective on generalized, linear, and mixed models, presenting a unified and accessible treatment of the newest statistical methods for analyzing correlated, nonnormally distributed data. As a follow-up to Searle's classic, Linear Models, and Variance Components by Searle, Casella, and McCulloch, this new work progresses from the basic one-way classification to generalized linear mixed models. A variety of statistical methods are explained and illustrated, with an emphasis on maximum likelihood and restricted maximum likelihood. An invaluable resource for applied statisticians and industrial practitioners, as well as students interested in the latest results, Generalized, Linear, and Mixed Models features: * A review of the basics of linear models and linear mixed models * Descriptions of models for nonnormal data, including generalized linear and nonlinear models * Analysis and illustration of techniques for a variety of real data sets * Information on the accommodation of longitudinal data using these models * Coverage of the prediction of realized values of random effects * A discussion of the impact of computing issues on mixed models