Alternative Methods For Variable Selection In Generalized Linear Models With Binary Outcomes And Incomplete Covariates
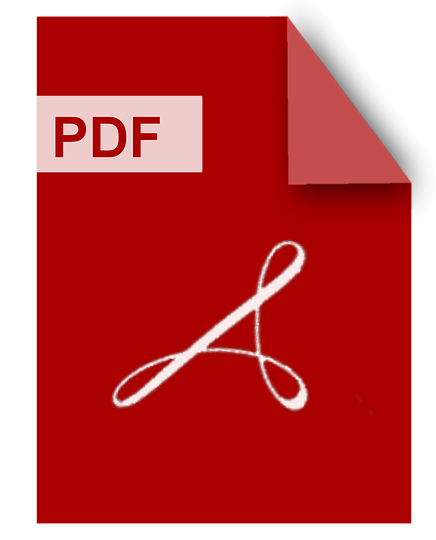
DOWNLOAD
Download Alternative Methods For Variable Selection In Generalized Linear Models With Binary Outcomes And Incomplete Covariates PDF/ePub or read online books in Mobi eBooks. Click Download or Read Online button to get Alternative Methods For Variable Selection In Generalized Linear Models With Binary Outcomes And Incomplete Covariates book now. This website allows unlimited access to, at the time of writing, more than 1.5 million titles, including hundreds of thousands of titles in various foreign languages. If the content not found or just blank you must refresh this page
Alternative Methods For Variable Selection In Generalized Linear Models With Binary Outcomes And Incomplete Covariates
DOWNLOAD
Author : Gang Liu
language : en
Publisher:
Release Date : 2007
Alternative Methods For Variable Selection In Generalized Linear Models With Binary Outcomes And Incomplete Covariates written by Gang Liu and has been published by this book supported file pdf, txt, epub, kindle and other format this book has been release on 2007 with categories.
Alternative Methods Of Variable Selection In Linear Regression Models For Incomplete Multivariate Normal Data
DOWNLOAD
Author : Xiaowei Yang
language : en
Publisher:
Release Date : 2002
Alternative Methods Of Variable Selection In Linear Regression Models For Incomplete Multivariate Normal Data written by Xiaowei Yang and has been published by this book supported file pdf, txt, epub, kindle and other format this book has been release on 2002 with categories.
Variable Selection By Regularization Methods For Generalized Mixed Models
DOWNLOAD
Author : Andreas Groll
language : en
Publisher: Cuvillier Verlag
Release Date : 2011-12-13
Variable Selection By Regularization Methods For Generalized Mixed Models written by Andreas Groll and has been published by Cuvillier Verlag this book supported file pdf, txt, epub, kindle and other format this book has been release on 2011-12-13 with Business & Economics categories.
A regression analysis describes the dependency of random variables in the form of a functional relationship. One distinguishes between the dependent response variable and one or more independent influence variables. There is a variety of model classes and inference methods available, ranging from the conventional linear regression model up to recent non- and semiparametric regression models. The so-called generalized regression models form a methodically consistent framework incorporating many regression approaches with response variables that are not necessarily normally distributed, including the conventional linear regression model based on the normal distribution assumption as a special case. When repeated measurements are modeled in addition to fixed effects also random effects or coefficients can be included. Such models are known as Random Effects Models or Mixed Models. As a consequence, regression procedures are applicable extremely versatile and consider very different problems. In this dissertation regularization techniques for generalized mixed models are developed that are able to perform variable selection. These techniques are especially appropriate when many potential influence variables are present and existing approaches tend to fail. First of all a componentwise boosting technique for generalized linear mixed models is presented which is based on the likelihood function and works by iteratively fitting the residuals using weak learners. The complexity of the resulting estimator is determined by information criteria. For the estimation of variance components two approaches are considered, an estimator resulting from maximizing the profile likelihood, and an estimator which can be calculated using an approximative EM-algorithm. Then the boosting concept is extended to mixed models with ordinal response variables. Two different types of ordered models are considered, the threshold model, also known as cumulative model, and the sequential model. Both are based on the assumption that the observed response variable results from a categorized version of a latent metric variable. In the further course of the thesis the boosting approach is extended to additive predictors. The unknown functions to be estimated are expanded in B-spline basis functions, whose smoothness is controlled by penalty terms. Finally, a suitable L1-regularization technique for generalized linear models is presented, which is based on a combination of Fisher scoring and gradient optimization. Extensive simulation studies and numerous applications illustrate the competitiveness of the methods constructed in this thesis compared to conventional approaches. For the calculation of standard errors bootstrap methods are used.
Generalized Linear Models
DOWNLOAD
Author : P. McCullagh
language : en
Publisher: Routledge
Release Date : 2019-01-22
Generalized Linear Models written by P. McCullagh and has been published by Routledge this book supported file pdf, txt, epub, kindle and other format this book has been release on 2019-01-22 with Mathematics categories.
The success of the first edition of Generalized Linear Models led to the updated Second Edition, which continues to provide a definitive unified, treatment of methods for the analysis of diverse types of data. Today, it remains popular for its clarity, richness of content and direct relevance to agricultural, biological, health, engineering, and ot
Topics On Bayesian Analysis Of Missing Data
DOWNLOAD
Author : Yun Kai Jiang
language : en
Publisher:
Release Date : 2011
Topics On Bayesian Analysis Of Missing Data written by Yun Kai Jiang and has been published by this book supported file pdf, txt, epub, kindle and other format this book has been release on 2011 with categories.
This dissertation focuses on model selection in logistic regression with incompletely observed data. In particular, methods are presented for using Markov Chain Monte Carlo imputation and Bayesian variable selection to model a binary outcome. We consider multivariate missing covariates, with different types of predictors, such as continuous, counts, and categorical variables. Such type of data is considered in the analysis of Project Talent recorded from a longitudinal study. Roughly 400,000 were selected for the study from United States high school students in grades 9 through 12 during the year 1960; follow-up surveys were conducted 1, 5, and 11 years after graduation. We extend a methodology developed by Yang, Belin, and Boscardin (2005), to this Project Talent for a logistic regression model with incomplete covariates. The idea is to use data information as much as possible to fill in the missing values and study associations between a binary response variable and covariates. According to Yang, Belin, and Boscardin, one approach under a multivariate normal assumption for data, is to conduct Bayesian variable selection and missing data imputation simultaneously within one Gibbs Sampling process, called "Simultaneously Impute And Select" (SIAS). A modified strategy of SIAS is extended to a mixed data structure that allows for categorical, counts, and continuous variables. The first chapter consists of an introduction to some approaches to variable selection for missing data. The fact that missing data arise commonly in statistical analyses, leads to a variety of methods to handle missing data. The missing data mechanism needs to be considered in imputations. The multiple imputation methods and Markov Chain Mote Carlo (MCMC) algorithms are presented as general statistical approaches to missing data analysis. In the MCMC computational toolbox, various implementation methods for imputation are discussed: Metropolis-Hasting, Gibbs Sampler, and Data Augmentation. Compared to model selection methods in frequentist and likelihood inference, Bayesian inference takes an entirely different approach. The frequentist approach only looks at the current data to make inference. The Bayesian approach requires the specification of the prior distribution, which can come from historical data or expert opinion. Stochastic Search Variable Selection (SSVS) and Gibbs Variable Selection (GVS) are reviewed for model selection. Two alternative strategies, Impute Then Select (ITS) and Simultaneously Impute And Select (SIAS), are studied. In the second chapter, imputation and Bayesian variable selection methods for linear regression are extended to a binary response variable that is completely observed, but some covariates have missing values. We focus on extending SIAS strategy to logistic regression models via two alternative imputations, decomposition and Fully Conditional Specification (FCS). The decomposition method breaks a multivariate distribution into a series of univariate ones by decomposing the joint density function p(Y, X1, ..., X[p]) into the product of conditional distributions, using the factorization p(A, B) = p(A[vertical line]B)p(B). The FCS aims to involve iteratively sampling from the conditional distributions for one random variable, given all the others. These two methods are implemented in the imputation step of the SIAS procedure then applied to the Project Talent data. Simulations are also performed to validate these results and demonstrate the superiority of FCS over the decomposition method under certain circumstances. The third chapter presents a new approach for incorporating the sampling weight into imputation and Bayesian variable selection in logistic regression models. We develop the approach that extends SIAS by a Bayesian version of iterative weighted least squares algorithm to include a sampling step based on Gibbs sampler. This approach is illustrated using both simulation studies and Project Talent data.
Applying Generalized Linear Models
DOWNLOAD
Author : James K. Lindsey
language : en
Publisher: Springer Science & Business Media
Release Date : 2008-01-15
Applying Generalized Linear Models written by James K. Lindsey and has been published by Springer Science & Business Media this book supported file pdf, txt, epub, kindle and other format this book has been release on 2008-01-15 with Mathematics categories.
This book describes how generalised linear modelling procedures can be used in many different fields, without becoming entangled in problems of statistical inference. The author shows the unity of many of the commonly used models and provides readers with a taste of many different areas, such as survival models, time series, and spatial analysis, and of their unity. As such, this book will appeal to applied statisticians and to scientists having a basic grounding in modern statistics. With many exercises at the end of each chapter, it will equally constitute an excellent text for teaching applied statistics students and non- statistics majors. The reader is assumed to have knowledge of basic statistical principles, whether from a Bayesian, frequentist, or direct likelihood point of view, being familiar at least with the analysis of the simpler normal linear models, regression and ANOVA.
Generalized Linear Models For Insurance Rating
DOWNLOAD
Author : Mark Goldburd
language : en
Publisher:
Release Date : 2016-06-08
Generalized Linear Models For Insurance Rating written by Mark Goldburd and has been published by this book supported file pdf, txt, epub, kindle and other format this book has been release on 2016-06-08 with categories.
Concave Selection In Generalized Linear Models
DOWNLOAD
Author : Dingfeng Jiang
language : en
Publisher:
Release Date : 2012
Concave Selection In Generalized Linear Models written by Dingfeng Jiang and has been published by this book supported file pdf, txt, epub, kindle and other format this book has been release on 2012 with Regression analysis categories.
We first propose an l2 grouped concave penalty to incorporate such group information in a regression model. The l2 grouped concave penalty performs group selection and includes group Lasso as a special case. An efficient algorithm is developed and its theoretical convergence property is established under certain regularity conditions. The group selection property of the l2 grouped concave penalty is desirable in some applications; while in other applications selection at both group and individual levels is needed. Hence, we propose an l1 grouped concave penalty for variable selection at both individual and group levels. An efficient algorithm is also developed for the l1 grouped concave penalty. Simulation studies are performed to evaluate the finite-sample performance of the two grouped concave selection methods. The new grouped penalties are also used in analyzing two motivation datasets. The results from both the simulation and real data analyses demonstrate certain benefits of using grouped penalties. Therefore, the proposed concave group penalties are valuable alternatives to the standard concave penalties.
Dissertation Abstracts International
DOWNLOAD
Author :
language : en
Publisher:
Release Date : 2008
Dissertation Abstracts International written by and has been published by this book supported file pdf, txt, epub, kindle and other format this book has been release on 2008 with Dissertations, Academic categories.
Flexible Imputation Of Missing Data Second Edition
DOWNLOAD
Author : Stef van Buuren
language : en
Publisher: CRC Press
Release Date : 2018-07-17
Flexible Imputation Of Missing Data Second Edition written by Stef van Buuren and has been published by CRC Press this book supported file pdf, txt, epub, kindle and other format this book has been release on 2018-07-17 with Mathematics categories.
Missing data pose challenges to real-life data analysis. Simple ad-hoc fixes, like deletion or mean imputation, only work under highly restrictive conditions, which are often not met in practice. Multiple imputation replaces each missing value by multiple plausible values. The variability between these replacements reflects our ignorance of the true (but missing) value. Each of the completed data set is then analyzed by standard methods, and the results are pooled to obtain unbiased estimates with correct confidence intervals. Multiple imputation is a general approach that also inspires novel solutions to old problems by reformulating the task at hand as a missing-data problem. This is the second edition of a popular book on multiple imputation, focused on explaining the application of methods through detailed worked examples using the MICE package as developed by the author. This new edition incorporates the recent developments in this fast-moving field. This class-tested book avoids mathematical and technical details as much as possible: formulas are accompanied by verbal statements that explain the formula in accessible terms. The book sharpens the reader’s intuition on how to think about missing data, and provides all the tools needed to execute a well-grounded quantitative analysis in the presence of missing data.