Concave Selection In Generalized Linear Models
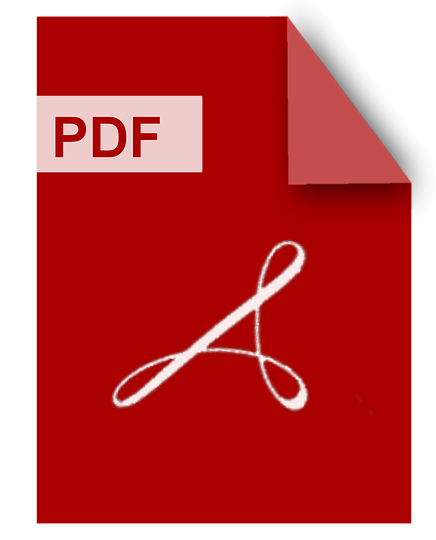
DOWNLOAD
Download Concave Selection In Generalized Linear Models PDF/ePub or read online books in Mobi eBooks. Click Download or Read Online button to get Concave Selection In Generalized Linear Models book now. This website allows unlimited access to, at the time of writing, more than 1.5 million titles, including hundreds of thousands of titles in various foreign languages. If the content not found or just blank you must refresh this page
Concave Selection In Generalized Linear Models
DOWNLOAD
Author : Dingfeng Jiang
language : en
Publisher:
Release Date : 2012
Concave Selection In Generalized Linear Models written by Dingfeng Jiang and has been published by this book supported file pdf, txt, epub, kindle and other format this book has been release on 2012 with Regression analysis categories.
We first propose an l2 grouped concave penalty to incorporate such group information in a regression model. The l2 grouped concave penalty performs group selection and includes group Lasso as a special case. An efficient algorithm is developed and its theoretical convergence property is established under certain regularity conditions. The group selection property of the l2 grouped concave penalty is desirable in some applications; while in other applications selection at both group and individual levels is needed. Hence, we propose an l1 grouped concave penalty for variable selection at both individual and group levels. An efficient algorithm is also developed for the l1 grouped concave penalty. Simulation studies are performed to evaluate the finite-sample performance of the two grouped concave selection methods. The new grouped penalties are also used in analyzing two motivation datasets. The results from both the simulation and real data analyses demonstrate certain benefits of using grouped penalties. Therefore, the proposed concave group penalties are valuable alternatives to the standard concave penalties.
Variable Selection In Generalized Linear Models By Empirical Likelihood
DOWNLOAD
Author : Asokan Mulayath Variyath
language : en
Publisher:
Release Date : 2006
Variable Selection In Generalized Linear Models By Empirical Likelihood written by Asokan Mulayath Variyath and has been published by this book supported file pdf, txt, epub, kindle and other format this book has been release on 2006 with categories.
Foundations Of Linear And Generalized Linear Models
DOWNLOAD
Author : Alan Agresti
language : en
Publisher: John Wiley & Sons
Release Date : 2015-01-15
Foundations Of Linear And Generalized Linear Models written by Alan Agresti and has been published by John Wiley & Sons this book supported file pdf, txt, epub, kindle and other format this book has been release on 2015-01-15 with Mathematics categories.
A valuable overview of the most important ideas and results in statistical modeling Written by a highly-experienced author, Foundations of Linear and Generalized Linear Models is a clear and comprehensive guide to the key concepts and results of linearstatistical models. The book presents a broad, in-depth overview of the most commonly usedstatistical models by discussing the theory underlying the models, R software applications,and examples with crafted models to elucidate key ideas and promote practical modelbuilding. The book begins by illustrating the fundamentals of linear models, such as how the model-fitting projects the data onto a model vector subspace and how orthogonal decompositions of the data yield information about the effects of explanatory variables. Subsequently, the book covers the most popular generalized linear models, which include binomial and multinomial logistic regression for categorical data, and Poisson and negative binomial loglinear models for count data. Focusing on the theoretical underpinnings of these models, Foundations ofLinear and Generalized Linear Models also features: An introduction to quasi-likelihood methods that require weaker distributional assumptions, such as generalized estimating equation methods An overview of linear mixed models and generalized linear mixed models with random effects for clustered correlated data, Bayesian modeling, and extensions to handle problematic cases such as high dimensional problems Numerous examples that use R software for all text data analyses More than 400 exercises for readers to practice and extend the theory, methods, and data analysis A supplementary website with datasets for the examples and exercises An invaluable textbook for upper-undergraduate and graduate-level students in statistics and biostatistics courses, Foundations of Linear and Generalized Linear Models is also an excellent reference for practicing statisticians and biostatisticians, as well as anyone who is interested in learning about the most important statistical models for analyzing data.
Alternative Methods For Variable Selection In Generalized Linear Models With Binary Outcomes And Incomplete Covariates
DOWNLOAD
Author : Gang Liu
language : en
Publisher:
Release Date : 2007
Alternative Methods For Variable Selection In Generalized Linear Models With Binary Outcomes And Incomplete Covariates written by Gang Liu and has been published by this book supported file pdf, txt, epub, kindle and other format this book has been release on 2007 with categories.
Generalized Linear Models
DOWNLOAD
Author : Dipak K. Dey
language : en
Publisher: CRC Press
Release Date : 2000-05-25
Generalized Linear Models written by Dipak K. Dey and has been published by CRC Press this book supported file pdf, txt, epub, kindle and other format this book has been release on 2000-05-25 with Mathematics categories.
This volume describes how to conceptualize, perform, and critique traditional generalized linear models (GLMs) from a Bayesian perspective and how to use modern computational methods to summarize inferences using simulation. Introducing dynamic modeling for GLMs and containing over 1000 references and equations, Generalized Linear Models considers
Prediction Emphasized Covariate Selection For Generalized Linear Models
DOWNLOAD
Author :
language : en
Publisher:
Release Date : 2009
Prediction Emphasized Covariate Selection For Generalized Linear Models written by and has been published by this book supported file pdf, txt, epub, kindle and other format this book has been release on 2009 with categories.
Shrinkage Parameter Selection In Generalized Linear And Mixed Models
DOWNLOAD
Author : Erin K. Melcon
language : en
Publisher:
Release Date : 2014
Shrinkage Parameter Selection In Generalized Linear And Mixed Models written by Erin K. Melcon and has been published by this book supported file pdf, txt, epub, kindle and other format this book has been release on 2014 with categories.
Penalized likelihood methods such as lasso, adaptive lasso, and SCAD have been highly utilized in linear models. Selection of the penalty parameter is an important step in modeling with penalized techniques. Traditionally, information criteria or cross validation are used to select the penalty parameter. Although methods of selecting this have been evaluated in linear models, general linear models and linear mixed models have not been so thoroughly explored.This dissertation will introduce a data-driven bootstrap (Empirical Optimal Selection, or EOS) approach for selecting the penalty parameter with a focus on model selection. We implement EOS on selecting the penalty parameter in the case of lasso and adaptive lasso. In generalized linear models we will introduce the method, show simulations comparing EOS to information criteria and cross validation, and give theoretical justification for this approach. We also consider a practical upper bound for the penalty parameter, with theoretical justification. In linear mixed models, we use EOS with two different objective functions; the traditional log-likelihood approach (which requires an EM algorithm), and a predictive approach. In both of these cases, we compare selecting the penalty parameter with EOS to selection with information criteria. Theoretical justification for both objective functions and a practical upper bound for the penalty parameter in the log-likelihood case are given. We also applied our technique to two datasets; the South African heart data (logistic regression) and the Yale infant data (a linear mixed model). For the South African data, we compare the final models using EOS and information criteria via the mean squared prediction error (MSPE). For the Yale infant data, we compare our results to those obtained by Ibrahim et al. (2011).
A Procedure For Selection Of Explanatory Variables In Generalized Linear Models
DOWNLOAD
Author : Lennart Nordberg
language : en
Publisher:
Release Date : 1981
A Procedure For Selection Of Explanatory Variables In Generalized Linear Models written by Lennart Nordberg and has been published by this book supported file pdf, txt, epub, kindle and other format this book has been release on 1981 with categories.
Building Regression Models With Sas
DOWNLOAD
Author : Robert N. Rodriguez
language : en
Publisher: SAS Institute
Release Date : 2023-04-18
Building Regression Models With Sas written by Robert N. Rodriguez and has been published by SAS Institute this book supported file pdf, txt, epub, kindle and other format this book has been release on 2023-04-18 with Computers categories.
Advance your skills in building predictive models with SAS! Building Regression Models with SAS: A Guide for Data Scientists teaches data scientists, statisticians, and other analysts who use SAS to train regression models for prediction with large, complex data. Each chapter focuses on a particular model and includes a high-level overview, followed by basic concepts, essential syntax, and examples using new procedures in both SAS/STAT and SAS Viya. By emphasizing introductory examples and interpretation of output, this book provides readers with a clear understanding of how to build the following types of models: general linear models quantile regression models logistic regression models generalized linear models generalized additive models proportional hazards regression models tree models models based on multivariate adaptive regression splines Building Regression Models with SAS is an essential guide to learning about a variety of models that provide interpretability as well as predictive performance.
Robust And Multivariate Statistical Methods
DOWNLOAD
Author : Mengxi Yi
language : en
Publisher: Springer Nature
Release Date : 2023-04-19
Robust And Multivariate Statistical Methods written by Mengxi Yi and has been published by Springer Nature this book supported file pdf, txt, epub, kindle and other format this book has been release on 2023-04-19 with Mathematics categories.
This book presents recent developments in multivariate and robust statistical methods. Featuring contributions by leading experts in the field it covers various topics, including multivariate and high-dimensional methods, time series, graphical models, robust estimation, supervised learning and normal extremes. It will appeal to statistics and data science researchers, PhD students and practitioners who are interested in modern multivariate and robust statistics. The book is dedicated to David E. Tyler on the occasion of his pending retirement and also includes a review contribution on the popular Tyler’s shape matrix.