Synthetic Datasets For Statistical Disclosure Control
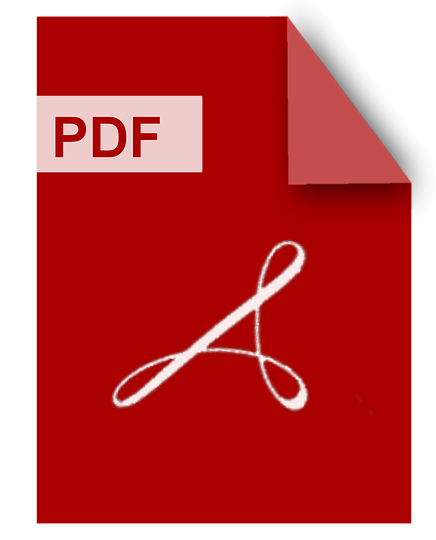
DOWNLOAD
Download Synthetic Datasets For Statistical Disclosure Control PDF/ePub or read online books in Mobi eBooks. Click Download or Read Online button to get Synthetic Datasets For Statistical Disclosure Control book now. This website allows unlimited access to, at the time of writing, more than 1.5 million titles, including hundreds of thousands of titles in various foreign languages. If the content not found or just blank you must refresh this page
Synthetic Datasets For Statistical Disclosure Control
DOWNLOAD
Author : Jörg Drechsler
language : en
Publisher: Springer Science & Business Media
Release Date : 2011-06-24
Synthetic Datasets For Statistical Disclosure Control written by Jörg Drechsler and has been published by Springer Science & Business Media this book supported file pdf, txt, epub, kindle and other format this book has been release on 2011-06-24 with Social Science categories.
The aim of this book is to give the reader a detailed introduction to the different approaches to generating multiply imputed synthetic datasets. It describes all approaches that have been developed so far, provides a brief history of synthetic datasets, and gives useful hints on how to deal with real data problems like nonresponse, skip patterns, or logical constraints. Each chapter is dedicated to one approach, first describing the general concept followed by a detailed application to a real dataset providing useful guidelines on how to implement the theory in practice. The discussed multiple imputation approaches include imputation for nonresponse, generating fully synthetic datasets, generating partially synthetic datasets, generating synthetic datasets when the original data is subject to nonresponse, and a two-stage imputation approach that helps to better address the omnipresent trade-off between analytical validity and the risk of disclosure. The book concludes with a glimpse into the future of synthetic datasets, discussing the potential benefits and possible obstacles of the approach and ways to address the concerns of data users and their understandable discomfort with using data that doesn’t consist only of the originally collected values. The book is intended for researchers and practitioners alike. It helps the researcher to find the state of the art in synthetic data summarized in one book with full reference to all relevant papers on the topic. But it is also useful for the practitioner at the statistical agency who is considering the synthetic data approach for data dissemination in the future and wants to get familiar with the topic.
Synthetic Datasets For Statistical Disclosure Control
DOWNLOAD
Author : J. Rg Drechsler
language : en
Publisher:
Release Date : 2011-06-26
Synthetic Datasets For Statistical Disclosure Control written by J. Rg Drechsler and has been published by this book supported file pdf, txt, epub, kindle and other format this book has been release on 2011-06-26 with categories.
Privacy In Statistical Databases
DOWNLOAD
Author : Josep Domingo-Ferrer
language : en
Publisher: Springer
Release Date : 2018-09-07
Privacy In Statistical Databases written by Josep Domingo-Ferrer and has been published by Springer this book supported file pdf, txt, epub, kindle and other format this book has been release on 2018-09-07 with Computers categories.
This book constitutes the refereed proceedings of the International Conference on Privacy in Statistical Databases, PSD 2018, held in Valencia, Spain, in September 2018 under the sponsorship of the UNESCO Chair in Data Privacy. The 23 revised full papers presented were carefully reviewed and selected from 42 submissions. The papers are organized into the following topics: tabular data protection; synthetic data; microdata and big data masking; record linkage; and spatial and mobility data. Chapter "SwapMob: Swapping Trajectories for Mobility Anonymization" is available open access under a Creative Commons Attribution 4.0 International License via link.springer.com.
Toward A Universal Privacy And Information Preserving Framework For Individual Data Exchange
DOWNLOAD
Author : Nicolas Ruiz
language : en
Publisher:
Release Date : 2019
Toward A Universal Privacy And Information Preserving Framework For Individual Data Exchange written by Nicolas Ruiz and has been published by this book supported file pdf, txt, epub, kindle and other format this book has been release on 2019 with categories.
Data on individual subjects, which are increasingly gathered and exchanged, provide a rich amount of information that can inform statistical and policy analysis in a meaningful way. However, due to the legal obligations surrounding such data, this wealth of information is often not fully exploited in order to protect the confidentiality of respondents. The issue is thus the following: how to ensure a sufficient level of data protection to meet releasers' concerns in terms of legal and ethical requirements, while still offering users a reasonable level of information. This question has raised a range concerns about the privacy/information trade-off and has driven a quest for best practices that can be both useful to users but also respectful of individuals' privacy. Statistical disclosure control research has historically provided the analytical apparatus through which the privacy/information trade-off can be assessed and implemented. In recent years, the literature has burgeoned in many directions. In particular, techniques applicable to micro data offer a wide variety of tools to protect the confidentiality of respondents while maximizing the information content of the data released, for the benefit of society at large. Such diversity is undoubtedly useful but has several major drawbacks. In fact, there is currently a clear lack of agreement and clarity as to the appropriate choice of tools in a given context, and as a consequence, there is no comprehensive view (or at best an incomplete one) of the relative performances of the techniques available. The practical scope of current micro data protection methods is not fully exploited precisely because there is no overarching framework: all methods generally carry their own analytical environment, underlying approaches and definitions of privacy and information. Moreover, the evaluation of utility and privacy for each method is metric and data-dependent, meaning that comparisons across different methods and datasets is a daunting task. Against this backdrop, this thesis focuses on establishing some common grounds for individual data anonymization by developing a new, universal approach. Recent contributions to the literature point to the fact that permutations happen to be the essential principle upon which individual data anonymization can be based. In this thesis, we demonstrate that this principle allows for the proposal of a universal analytical environment for data anonymization. The first contribution of this thesis takes an ex-post approach by proposing some universal measures of disclosure risk and information loss that can be computed in a simple fashion and used for the evaluation of any anonymization method, independently of the context under which they operate. In particular, they exhibit distributional independence. These measures establish a common language for comparing different mechanisms, all with potentially varying parametrizations applied to the same data set or to different data sets. The second contribution of this thesis takes an ex-ante approach by developing a new approach to data anonymization. Bringing data anonymization closer to cryptography, it formulates a general cipher based on permutation keys which appears to be equivalent to a general form of rank swapping. Beyond all the existing methods that this cipher can universally reproduce, it also offers a new way to practice data anonymization based on the ex-ante exploration of different permutation structures. The subsequent study of the cipher's properties additionally reveals new insights as to the nature of the task of anonymization taken at a general level of functioning. The final two contributions of this thesis aim at exploring two specific areas using the above results. The first area is longitudinal data anonymization. Despite the fact that the SDC literature offers a wide variety of tools suited to different contexts and data types, there have been very few attempts to deal with the challenges posed by longitudinal data. This thesis thus develops a general framework and some associated metrics of disclosure risk and information loss, tailored to the specific challenges posed by longitudinal data anonymization. Notably, it builds on a permutation approach where the effect of time on time-variant attributes can be seen as an anonymization method that can be captured by temporal permutations. The second area considered is synthetic data. By challenging the information and privacy guarantees of synthetic data, it is shown that any synthetic data set can always be expressed as a permutation of the original data, in a way similar to non-synthetic SDC techniques. In fact, releasing synthetic data sets with the same privacy properties but with an improved level of information appears to be invariably possible as the marginal distributions can always be preserved without increasing risk. On the privacy front, this leads to the consequence that the distinction drawn in the literature between non-synthetic and synthetic data is not so clear-cut. Indeed, it is shown that the practice of releasing several synthetic data sets for a single original data set entails privacy issues that do not arise in non-synthetic anonymization.
Privacy In Statistical Databases
DOWNLOAD
Author : Josep Domingo-Ferrer
language : en
Publisher: Springer
Release Date : 2012-09-12
Privacy In Statistical Databases written by Josep Domingo-Ferrer and has been published by Springer this book supported file pdf, txt, epub, kindle and other format this book has been release on 2012-09-12 with Computers categories.
This book constitutes the refereed proceedings of the International Conference on Privacy in Statistical Databases, PSD 2012, held in Palermo, Italy, in September 2012 under the sponsorship of the UNESCO chair in Data Privacy. The 27 revised full papers presented were carefully reviewed and selected from 38 submissions. The papers are organized in topical sections on tabular data protection; microdata protection: methods and disclosure risk; microdata protection: case studies; spatial data protection; differential privacy; on-line databases and remote access; privacy-preserving protocols.
Statistical Disclosure Control For Microdata
DOWNLOAD
Author : Matthias Templ
language : en
Publisher: Springer
Release Date : 2017-05-05
Statistical Disclosure Control For Microdata written by Matthias Templ and has been published by Springer this book supported file pdf, txt, epub, kindle and other format this book has been release on 2017-05-05 with Social Science categories.
This book on statistical disclosure control presents the theory, applications and software implementation of the traditional approach to (micro)data anonymization, including data perturbation methods, disclosure risk, data utility, information loss and methods for simulating synthetic data. Introducing readers to the R packages sdcMicro and simPop, the book also features numerous examples and exercises with solutions, as well as case studies with real-world data, accompanied by the underlying R code to allow readers to reproduce all results. The demand for and volume of data from surveys, registers or other sources containing sensible information on persons or enterprises have increased significantly over the last several years. At the same time, privacy protection principles and regulations have imposed restrictions on the access and use of individual data. Proper and secure microdata dissemination calls for the application of statistical disclosure control methods to the da ta before release. This book is intended for practitioners at statistical agencies and other national and international organizations that deal with confidential data. It will also be interesting for researchers working in statistical disclosure control and the health sciences.
Statistical Disclosure Control
DOWNLOAD
Author : Anco Hundepool
language : en
Publisher: John Wiley & Sons
Release Date : 2012-07-05
Statistical Disclosure Control written by Anco Hundepool and has been published by John Wiley & Sons this book supported file pdf, txt, epub, kindle and other format this book has been release on 2012-07-05 with Mathematics categories.
A reference to answer all your statistical confidentiality questions. This handbook provides technical guidance on statistical disclosure control and on how to approach the problem of balancing the need to provide users with statistical outputs and the need to protect the confidentiality of respondents. Statistical disclosure control is combined with other tools such as administrative, legal and IT in order to define a proper data dissemination strategy based on a risk management approach. The key concepts of statistical disclosure control are presented, along with the methodology and software that can be used to apply various methods of statistical disclosure control. Numerous examples and guidelines are also featured to illustrate the topics covered. Statistical Disclosure Control: Presents a combination of both theoretical and practical solutions Introduces all the key concepts and definitions involved with statistical disclosure control. Provides a high level overview of how to approach problems associated with confidentiality. Provides a broad-ranging review of the methods available to control disclosure. Explains the subtleties of group disclosure control. Features examples throughout the book along with case studies demonstrating how particular methods are used. Discusses microdata, magnitude and frequency tabular data, and remote access issues. Written by experts within leading National Statistical Institutes. Official statisticians, academics and market researchers who need to be informed and make decisions on disclosure limitation will benefit from this book.
Privacy In Statistical Databases
DOWNLOAD
Author : Josep Domingo-Ferrer
language : en
Publisher: Springer
Release Date : 2008-09-22
Privacy In Statistical Databases written by Josep Domingo-Ferrer and has been published by Springer this book supported file pdf, txt, epub, kindle and other format this book has been release on 2008-09-22 with Computers categories.
Privacy in statistical databases is a discipline whose purpose is to provide solutions to the tension between the increasing social, political and economical demand of accurate information, and the legal and ethical obligation to protect the privacy of the various parties involved. Those parties are the respondents (the individuals and enterprises to which the database records refer), the data owners (those organizations spending money in data collection) and the users (the ones querying the database, who would like their queries to stay con?d- tial). Beyond law and ethics, there are also practical reasons for data collecting agencies to invest in respondent privacy: if individual respondents feel their p- vacyguaranteed,they arelikelyto providemoreaccurateresponses. Data owner privacy is primarily motivated by practical considerations: if an enterprise c- lects data at its own expense, it may wish to minimize leakage of those data to other enterprises (even to those with whom joint data exploitation is planned). Finally, user privacy results in increased user satisfaction, even if it may curtail the ability of the database owner to pro?le users. Thereareatleasttwotraditionsinstatisticaldatabaseprivacy,bothofwhich started in the 1970s: one stems from o?cial statistics, where the discipline is also known as statistical disclosure control (SDC), and the other originatesfrom computer science and database technology. In o?cial statistics, the basic c- cern is respondent privacy.
Statistical Data Privacy Methods For Increasing Research Opportunities
DOWNLOAD
Author : Joshua Snoke
language : en
Publisher:
Release Date : 2018
Statistical Data Privacy Methods For Increasing Research Opportunities written by Joshua Snoke and has been published by this book supported file pdf, txt, epub, kindle and other format this book has been release on 2018 with categories.
In this dissertation, we develop statistical methods for providing access to sensitive data, with the goal of simultaneously protecting individuals privacy and enabling high quality research. In addition to the theoretical contributions we provide to the area of statistical data privacy, our work is motivated by collaborations with practitioners and real policy problems, and as such is meant to be highly practical and easy to implement. We present two alternative paradigms for providing researchers access to sensitive data that build on ideas from statistical disclosure control (SDC) methodology, and techniques of secure multiparty computation (SMPC) and differential privacy (DP) from computer science.First, under the SMPC framework we develop an algorithm for computing secure maximum likelihood estimates (MLE) over partitioned databases without sharing any data or intermediate statistics. This is motivated by the scenario where different entities (or individuals) hold separate partitions of data, and researchers wish to obtain model estimates or statistics utilizing all the data which cannot be combined. We show that under a certain set of assumptions our method for estimation across these partitions achieves identical results as estimation with the full data but without violating privacy. We demonstrate the utility of the algorithm through the simulations and estimation of structural equation models with real data, and point out that is more widely applicable to factor models, linear regression, and PCA.Second, we provide new theoretical results for the utility evaluation of synthetic data based on its distributional similarity to the original data. The release of synthetic data is motivated by the desire for researchers to have downloadable microdata which they can use for exploration and model testing but that do not violate privacy. We derive new theoretical results for the propensity score mean-squared-error (pMSE) utility measure, and demonstrate how its use can improve on the choice of synthetic data models. We further combine the pMSE with differentially private methodology to produce synthetic data that maximize distributional similarity under the constraints of epsilon-DP. This ensures that we not only release synthetic data that others high utility, but it also guarantees quantifiable and provable privacy protections for the individuals in the data.
Privacy In Statistical Databases
DOWNLOAD
Author : Josep Domingo-Ferrer
language : en
Publisher: Springer
Release Date : 2020-08-21
Privacy In Statistical Databases written by Josep Domingo-Ferrer and has been published by Springer this book supported file pdf, txt, epub, kindle and other format this book has been release on 2020-08-21 with Computers categories.
This book constitutes the refereed proceedings of the International Conference on Privacy in Statistical Databases, PSD 2020, held in Tarragona, Spain, in September 2020 under the sponsorship of the UNESCO Chair in Data Privacy. The 25 revised full papers presented were carefully reviewed and selected from 49 submissions. The papers are organized into the following topics: privacy models; microdata protection; protection of statistical tables; protection of interactive and mobility databases; record linkage and alternative methods; synthetic data; data quality; and case studies. The Chapter “Explaining recurrent machine learning models: integral privacy revisited” is available open access under a Creative Commons Attribution 4.0 International License via link.springer.com.