Targeted Minimum Loss Based Estimation
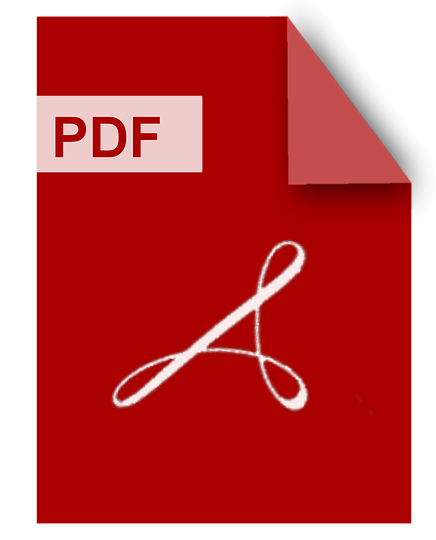
DOWNLOAD
Download Targeted Minimum Loss Based Estimation PDF/ePub or read online books in Mobi eBooks. Click Download or Read Online button to get Targeted Minimum Loss Based Estimation book now. This website allows unlimited access to, at the time of writing, more than 1.5 million titles, including hundreds of thousands of titles in various foreign languages. If the content not found or just blank you must refresh this page
Targeted Minimum Loss Based Estimation
DOWNLOAD
Author : Samuel David Lendle
language : en
Publisher:
Release Date : 2015
Targeted Minimum Loss Based Estimation written by Samuel David Lendle and has been published by this book supported file pdf, txt, epub, kindle and other format this book has been release on 2015 with categories.
Causal inference generally requires making some assumptions on a causal mechanism followed by statistical estimation. The statistical estimation problem in causal inference is often that of estimating a pathwise differentiable parameter in a semiparametric or nonparametric model. Targeted minimum loss-based estimating (TMLE) is a framework for constructing an asymptotically linear plug-in estimator for such parameters. The natural direct effect (NDE) is a parameter that quantifies how some treatment affects some outcome directly, as opposed to indirectly through some mediator value between the treatment and outcome on the causal pathway. In Chapter 2, we introduce the NDE among the untreated and show that under some assumptions the NDE among the untreated is identifiable and equivalent to a statistical parameter as the so called average treatment effect among the untreated. We then present a locally efficient, doubly robust TMLE for the statistical target parameter and apply it to the estimation of the NDE among the untreated in simulations and of the NDE in a data set from an RCT. Some estimators that adjust for the propensity score (PS) nonparametrically, such as PS matching or stratification by the PS, are robust to slight misspecification of the PS estimator. In particular, if the PS estimator fails to estimate the true propensity score, but still approximates some other balancing score, such methods are still consistent for average treatment effect (ATE). In Chapter 3, we extend a traditional TMLE for the ATE to have this property while still being locally efficient and doubly robust and investigate the performance of the proposed estimator in a simulation study. Online estimators are estimators that process a relatively small piece of a data set at a time, and can be updated as more data becomes available. Typically, online estimators are used in the large scale machine learning literature, but to our knowledge, have not been used to estimate statistical parameters associated with causal parameters. In Chapter 4, we propose two online estimators for the ATE that are asymptotically efficient and doubly robust in a single pass through a data set. The first is similar to the augmented inverse probability of treatment weighting estimator in the batch setting, and the second involves an additional targeting step inspired by TMLE, which improves performance in some cases. We investigate the performance of both in a simulation study.
Targeted Minimum Loss Based Estimation For Longitudinal Data
DOWNLOAD
Author : Paul H. Chaffee
language : en
Publisher:
Release Date : 2012
Targeted Minimum Loss Based Estimation For Longitudinal Data written by Paul H. Chaffee and has been published by this book supported file pdf, txt, epub, kindle and other format this book has been release on 2012 with categories.
Sequential Randomized Controlled Trials (SRCTs) are rapidly becoming essential tools in the search for optimized treatment regimes in ongoing treatment settings. Analyzing data for multiple time-point treatments with a view toward optimal treatment regimes is of interest in many types of afflictions: HIV infection, Attention Deficit Hyperactivity Disorder in children, leukemia, prostate cancer, renal failure, and many others. Methods for analyzing data from SRCTs exist but they are either inefficient or suffer from the drawbacks of estimating equation methodology. This dissertation describes the development of a general methodology for estimating parameters that would typically be of interest both in SRCTs and in observational studies which are longitudinal in nature, and have multiple time-point exposures or treatments. It is expected in such contexts that time-dependant confounding is either present (observational studies) or actually designed in as part of a study (SRCTs). The method, targeted minimum loss based estimation (TMLE), has been fully developed and implemented in point treatment settings and for various outcome types, including time to event outcomes, and binary and continuous outcomes. Here we develop and implement TMLE in the longitudinal setting, and pay special attention to dynamic treatments or exposures, as might be seen in SRCTs. Dynamic exposures are not limited to SRCTs however. The idea of a rule-based intervention turns out be a very fruitful one when one faces complex treatment or exposure patterns, or when one encounters challenges in defining an intervention that must depend on time-varying factors. As in the former settings, the TMLE procedure is targeted toward a pre-specified parameter of the distribution of the observed data, and thereby achieves important bias reduction over non-targeted procedures in estimation of that parameter. As with the so-called Augmented Inverse Probability of Censoring Weight (A-IPCW) estimator, TMLE is double-robust and locally efficient. We develop some of the background involving the causal and statistical models and report the results of several simulation studies under various data-generating distributions and for two outcome types (binary, and continuous on [0,1]). In our results we include comparisons from a number of other estimators in current use. Chapter 1 develops the background and context in which this estimator appears, gives a brief history of other estimators used in SRCTs and describes some of the theory behind TMLE in the longitudinal setting. Two different TMLE algorithms are described in detail, and results of a simulation study for three separate causal parameters are presented. Chapter 2 concerns the development of a new TMLE that solves the efficient influence curve estimating equation directly by numerical methods, rather than indirectly, which is the usual procedure. A new set of simulations is performed here that compare this TMLE with the preceding two (presented in chapter 1). Its performance is comparable to those described in chapter 1, but it is somewhat easier to implement. Chapter 3 is a comparison of still another new TMLE (described in van der Laan and Gruber, 2012) with one of the three described above. This TMLE arguably shows the most promise generally, since it's implementation does not require discretization of the intermediate factors of the likelihood as does the three preceding TMLEs. Further, under the right conditions it exhibits superior performance in terms of MSE. We also explore a new, targeted criterion for selecting the initial estimators involved. Chapter 4 describes a detailed analysis of the estimation of the effect of gestational weight gain on women's long term BMI using the preferred TMLE described in chapter 3. Many issues were encountered during this analysis concerning censoring of the exposure variable that led to the redefinition of the parameter of interest, and the implementation of a different type of TMLE for the first time (described originally in van der Laan, 2008). We also encountered issues arising from sparsity in the data and propose and implement corresponding solutions. The analysis was performed using data from the national longitudinal survey of youth, begun in 1979 and ending in 2008.
Targeted Minimum Loss Estimation
DOWNLOAD
Author : Wenjing Zheng
language : en
Publisher:
Release Date : 2011
Targeted Minimum Loss Estimation written by Wenjing Zheng and has been published by this book supported file pdf, txt, epub, kindle and other format this book has been release on 2011 with categories.
Estimating Optimal Surrogate Endpoints By Machine Learning And Targeted Minimum Loss Based Estimation In Two Phase Sampling Studies
DOWNLOAD
Author : Brenda Price
language : en
Publisher:
Release Date : 2020
Estimating Optimal Surrogate Endpoints By Machine Learning And Targeted Minimum Loss Based Estimation In Two Phase Sampling Studies written by Brenda Price and has been published by this book supported file pdf, txt, epub, kindle and other format this book has been release on 2020 with categories.
This dissertation provides contributions in two areas: the application of TMLE in estimation of an optimal surrogate and implementation of inverse probability of censoring weighted targeted minimum loss-based estimation (IPCW-TMLE). In Chapter 1 we develop methodology for the estimation of optimal surrogates in randomized trials using targeted minimum loss-based estimation (TMLE), first in the setting of complete data, and then in Chapter 2, extended to the setting of two-phase data, seeking to make the methodology more applicable to real randomized trials. In Chapter 3 we present a comparison of IPCW-TMLE to a commonly used method of Breslow and Holubkov for parameter estimation in two-phase studies. The simulation study presented assesses the comparative differences in bias and efficiency of estimates obtained by both methods. In Chapter 4, IPCW-TMLE is elaborated for estimation of causal parameters of interest in right-censored two-phase studies. The methods developed in this dissertation have broad application to randomized clinical trials with two-phase designs for measuring biomarkers. Many of the methods described in this dissertation are illustrated with application to two dengue phase 3 vaccine efficacy trials.
Targeted Learning
DOWNLOAD
Author : Mark J. van der Laan
language : en
Publisher: Springer Science & Business Media
Release Date : 2011-06-17
Targeted Learning written by Mark J. van der Laan and has been published by Springer Science & Business Media this book supported file pdf, txt, epub, kindle and other format this book has been release on 2011-06-17 with Mathematics categories.
The statistics profession is at a unique point in history. The need for valid statistical tools is greater than ever; data sets are massive, often measuring hundreds of thousands of measurements for a single subject. The field is ready to move towards clear objective benchmarks under which tools can be evaluated. Targeted learning allows (1) the full generalization and utilization of cross-validation as an estimator selection tool so that the subjective choices made by humans are now made by the machine, and (2) targeting the fitting of the probability distribution of the data toward the target parameter representing the scientific question of interest. This book is aimed at both statisticians and applied researchers interested in causal inference and general effect estimation for observational and experimental data. Part I is an accessible introduction to super learning and the targeted maximum likelihood estimator, including related concepts necessary to understand and apply these methods. Parts II-IX handle complex data structures and topics applied researchers will immediately recognize from their own research, including time-to-event outcomes, direct and indirect effects, positivity violations, case-control studies, censored data, longitudinal data, and genomic studies.
Targeted Learning In Data Science
DOWNLOAD
Author : Mark J. van der Laan
language : en
Publisher: Springer
Release Date : 2018-03-28
Targeted Learning In Data Science written by Mark J. van der Laan and has been published by Springer this book supported file pdf, txt, epub, kindle and other format this book has been release on 2018-03-28 with Mathematics categories.
This textbook for graduate students in statistics, data science, and public health deals with the practical challenges that come with big, complex, and dynamic data. It presents a scientific roadmap to translate real-world data science applications into formal statistical estimation problems by using the general template of targeted maximum likelihood estimators. These targeted machine learning algorithms estimate quantities of interest while still providing valid inference. Targeted learning methods within data science area critical component for solving scientific problems in the modern age. The techniques can answer complex questions including optimal rules for assigning treatment based on longitudinal data with time-dependent confounding, as well as other estimands in dependent data structures, such as networks. Included in Targeted Learning in Data Science are demonstrations with soft ware packages and real data sets that present a case that targeted learning is crucial for the next generation of statisticians and data scientists. Th is book is a sequel to the first textbook on machine learning for causal inference, Targeted Learning, published in 2011. Mark van der Laan, PhD, is Jiann-Ping Hsu/Karl E. Peace Professor of Biostatistics and Statistics at UC Berkeley. His research interests include statistical methods in genomics, survival analysis, censored data, machine learning, semiparametric models, causal inference, and targeted learning. Dr. van der Laan received the 2004 Mortimer Spiegelman Award, the 2005 Van Dantzig Award, the 2005 COPSS Snedecor Award, the 2005 COPSS Presidential Award, and has graduated over 40 PhD students in biostatistics and statistics. Sherri Rose, PhD, is Associate Professor of Health Care Policy (Biostatistics) at Harvard Medical School. Her work is centered on developing and integrating innovative statistical approaches to advance human health. Dr. Rose’s methodological research focuses on nonparametric machine learning for causal inference and prediction. She co-leads the Health Policy Data Science Lab and currently serves as an associate editor for the Journal of the American Statistical Association and Biostatistics.
Variable And Model Selection For Propensity Score Estimators In Causal Inference
DOWNLOAD
Author : Cheng Ju
language : en
Publisher:
Release Date : 2018
Variable And Model Selection For Propensity Score Estimators In Causal Inference written by Cheng Ju and has been published by this book supported file pdf, txt, epub, kindle and other format this book has been release on 2018 with categories.
Robust inference of a low-dimensional parameter in a large semi-parametric model relies on external estimators of infinite-dimensional features of the distribution of the data. Typically, only one of the latter is optimized for the sake of constructing a well behaved estimator of the low-dimensional parameter of interest. Optimizing more than one of them for the sake of achieving a better bias-variance trade-off in the estimation of the parameter of interest is the core idea driving the general template of the collaborative targeted minimum loss-based estimation (C-TMLE) procedure. In this dissertation, we first resolves the computational issue in the widely-used greedy variable selection C-TMLE. Then we further investigate how to extend the discrete, variable selection C-TMLE for a more general model selection purpose. Chapter 1 begins by introducing the framework of causal inference in observational studies. We introduce the non-parametric structural equation model for modeling the data generating distribution. We briefly review the targeted minimum loss-based estimation (TMLE). We also introduce the general template of C-TMLE and its greedy-search variable selection version. In chapter 2, we propose the template for scalable variable selection C-TMLEs to overcome the computational burden in the greedy variable selection C-TMLE. The original instantiation of the C-TMLE template can be presented as a greedy forward stepwise C-TMLE algorithm. It does not scale well when the number $p$ of covariates increases drastically. This motivates the introduction of a novel instantiation of the C-TMLE template where the covariates are pre-ordered. Its time complexity is $\mathcal{O}(p)$ as opposed to the original $\mathcal{O}(p^2)$, a remarkable gain. We propose two pre-ordering strategies and suggest a rule of thumb to develop other meaningful strategies. Because it is usually unclear a priori which pre-ordering strategy to choose, we also introduce another instantiation called SL-C-TMLE algorithm that enables the data-driven choice of the better pre-ordering strategy given the problem at hand. Its time complexity is $\mathcal{O}(p)$ as well. The computational burden and relative performance of these algorithms were compared in simulation studies involving fully synthetic data or partially synthetic data based on a real world large electronic health database; and in analyses of three real, large electronic health databases. In all analyses involving electronic health databases, the greedy C-TMLE algorithm is unacceptably slow. Simulation studies seem to indicate that our scalable C-TMLE and SL-C-TMLE algorithms work well. In chapter 3, we extend C-TMLE to a more general model selection problem: we apply C-TMLE to select from a set of continuously-indexed nuisance parameter (the propensity score, PS) estimators. The propensity score models have traditionally been selected based on the goodness-of-fit for the treatment mechanism itself, without consideration of the causal parameter of interest. In contrast, the C-TMLE takes into account information on the causal parameter of interest when selecting a PS model. This ``collaborative learning'' considers variable associations with both treatment and outcome when selecting a PS model in order to minimize a bias-variance trade off in the estimated treatment effect. In this study, we introduce a novel approach for collaborative model selection when using the LASSO estimator for PS estimation in high-dimensional covariate settings. To demonstrate the importance of selecting the PS model collaboratively, we designed quasi-experiments based on a real electronic healthcare database, where only the potential outcomes were manually generated, and the treatment and baseline covariates remained unchanged. Results showed that the C-TMLE algorithm outperformed other competing estimators for both point estimation and confidence interval coverage. In addition, the PS model selected by C-TMLE could be applied to other PS-based estimators, which also resulted in substantive improvement for both point estimation and confidence interval coverage. We illustrate the discussed concepts through an empirical example comparing the effects of non-selective Nonsteroidal anti-inflammatory drugs with selective COX-2 inhibitors on gastrointestinal complications in a population of Medicare beneficiaries. In chapter 4, we propose using C-TMLE to adaptively truncated the propensity score when there exist practical positivity violations. The positivity assumption, or the experimental treatment assignment (ETA) assumption, is important for identifiability in causal inference. Even if the positivity assumption holds, practical violations of this assumption may jeopardize the finite sample performance of the causal estimator. One of the consequences of practical violations of the positivity assumption is extreme values in the estimated propensity score. A common practice to address this issue is truncating the PS estimate when constructing PS-based estimators. In this study, we propose a novel adaptive truncation method, Positivity-C-TMLE, based on the C-TMLE methodology. We further show how to construct a robust confidence interval by a targeted variance estimator. We demonstrate the outstanding performance of our novel approach in a variety of simulations by comparing it with other commonly studied estimators, for both point estimation and confidence interval coverage. Results show that by adaptively truncating the estimated PS with a more targeted objective function, the Positivity-C-TMLE estimator achieves the best performance for both point estimation and confidence interval coverage among all estimators considered. The code for all the variations of C-TMLE in this dissertation are publicly available in the \emph{ctmle} R package.
Interval Censored Time To Event Data
DOWNLOAD
Author : Ding-Geng (Din) Chen
language : en
Publisher: CRC Press
Release Date : 2012-07-19
Interval Censored Time To Event Data written by Ding-Geng (Din) Chen and has been published by CRC Press this book supported file pdf, txt, epub, kindle and other format this book has been release on 2012-07-19 with Mathematics categories.
Interval-Censored Time-to-Event Data: Methods and Applications collects the most recent techniques, models, and computational tools for interval-censored time-to-event data. Top biostatisticians from academia, biopharmaceutical industries, and government agencies discuss how these advances are impacting clinical trials and biomedical research. Divided into three parts, the book begins with an overview of interval-censored data modeling, including nonparametric estimation, survival functions, regression analysis, multivariate data analysis, competing risks analysis, and other models for interval-censored data. The next part presents interval-censored methods for current status data, Bayesian semiparametric regression analysis of interval-censored data with monotone splines, Bayesian inferential models for interval-censored data, an estimator for identifying causal effect of treatment, and consistent variance estimation for interval-censored data. In the final part, the contributors use Monte Carlo simulation to assess biases in progression-free survival analysis as well as correct bias in interval-censored time-to-event applications. They also present adaptive decision making methods to optimize the rapid treatment of stroke, explore practical issues in using weighted logrank tests, and describe how to use two R packages. A practical guide for biomedical researchers, clinicians, biostatisticians, and graduate students in biostatistics, this volume covers the latest developments in the analysis and modeling of interval-censored time-to-event data. It shows how up-to-date statistical methods are used in biopharmaceutical and public health applications.
Handbook Of Matching And Weighting Adjustments For Causal Inference
DOWNLOAD
Author : José R. Zubizarreta
language : en
Publisher: CRC Press
Release Date : 2023-04-11
Handbook Of Matching And Weighting Adjustments For Causal Inference written by José R. Zubizarreta and has been published by CRC Press this book supported file pdf, txt, epub, kindle and other format this book has been release on 2023-04-11 with Mathematics categories.
An observational study infers the effects caused by a treatment, policy, program, intervention, or exposure in a context in which randomized experimentation is unethical or impractical. One task in an observational study is to adjust for visible pretreatment differences between the treated and control groups. Multivariate matching and weighting are two modern forms of adjustment. This handbook provides a comprehensive survey of the most recent methods of adjustment by matching, weighting, machine learning and their combinations. Three additional chapters introduce the steps from association to causation that follow after adjustments are complete. When used alone, matching and weighting do not use outcome information, so they are part of the design of an observational study. When used in conjunction with models for the outcome, matching and weighting may enhance the robustness of model-based adjustments. The book is for researchers in medicine, economics, public health, psychology, epidemiology, public program evaluation, and statistics who examine evidence of the effects on human beings of treatments, policies or exposures.
Comparative Causal Effect Estimation And Robust Variance For Longitudinal Data Structures With Applications To Observational Hiv Treatment
DOWNLOAD
Author : Linh Mai Tran
language : en
Publisher:
Release Date : 2016
Comparative Causal Effect Estimation And Robust Variance For Longitudinal Data Structures With Applications To Observational Hiv Treatment written by Linh Mai Tran and has been published by this book supported file pdf, txt, epub, kindle and other format this book has been release on 2016 with categories.
This dissertation discusses the application and comparative performance of double robust estimators for estimating the intervention specific mean outcome in longitudinal settings with time-dependent confounding as well as the corresponding estimator variances. Specifically, we focus on carefully defining target causal parameters to avoid known positivity issues, estimating these parameters using the asymptotically efficient and double robust targeted minimum loss-based estimation, comparing this to other double robust estimators of the same causal parameter, and estimating the corresponding variances in a way which demonstrates valid Type I errors while retaining statistical power. Chapter 1 begins by introducing the open problem in statistics. We present the International epidemiologic Databases to Evaluate AIDS, East Africa region (IeDEA-EA) cohort and the implementation of a low risk express care program implemented between 2007-2009. We continue in Chapter 2 by presenting the targeted learning road map for causal inference. This road map is applied, as a case study, to the IeDEA-EA cohort in evaluating the impact of the low risk express care program. Targeted minimum loss-based estimation is used to estimate the intervention specific mean outcome using data adaptive machine learning candidate estimators for the nuisance parameters. Practical issues are addressed, including carefully defining the causal parameters (and the corresponding causal contrasts) and remaining within the boundaries implied by the statistical model while using the machine learning algorithms. In Chapter 3, we compare additional estimators for the intervention specific mean outcome. The iterated conditional expectation estimator, inverse probability weighted estimator, augmented inverse probability weighted estimator, double robust iterated conditional expectation estimator, and targeted minimum loss-based estimator are presented. Additionally, variations on the double robust iterated conditional expectation estimator and targeted minimum loss-based estimator are reviewed and implemented. Simulations are conducted to analyze the finite sample performance of each estimator, in both correct and mis-specified models. The estimators are also applied to estimating the impact of enrollment into the low risk express care program in the IeDEA-EA cohort. Chapter 4 studies the estimation of estimator variance for estimators solving the efficient influence function. A robust approach of estimating the efficient influence function variance is presented, followed by approaches for estimating the derived expectation of the variance. This robust approach of estimating the EIF variance can be used to raise a red flag for unreliable statistical inference due to sparsity, thereby declaring that the target parameter is practically not identifiable from the data, and that the reported variance estimates (though large) will themselves be imprecise. We additionally present a bootstrap approach based on fitting the initial density of the data once, followed by a non-parametric bootstrap of the targeting step. This bootstrap approach can be used to estimate the variance of substitution based estimators solving the efficient influence function. Simulations are conducted, demonstrating the bias, variance, coverage, and statistical power resulting from each of the variance estimators. Standard errors and confidence intervals are calculated using each of the variance estimators in estimating the impact of enrollment into the low risk express care program in the IeDEA-EA cohort. The primary appendices present relevant proofs for the analyses conducted in this dissertation, while R code for implementing the various estimators are provided in secondary appendices.