The Empirical Similarity Approach For Volatility Prediction
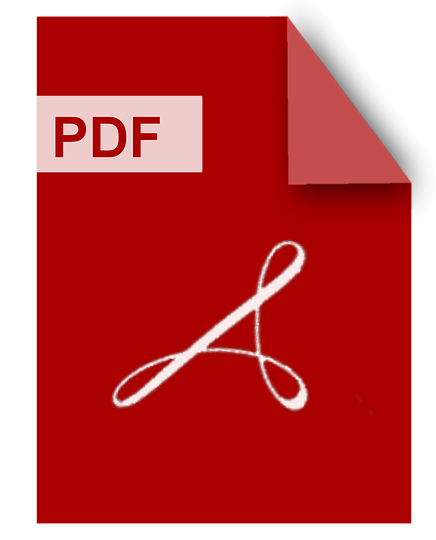
DOWNLOAD
Download The Empirical Similarity Approach For Volatility Prediction PDF/ePub or read online books in Mobi eBooks. Click Download or Read Online button to get The Empirical Similarity Approach For Volatility Prediction book now. This website allows unlimited access to, at the time of writing, more than 1.5 million titles, including hundreds of thousands of titles in various foreign languages. If the content not found or just blank you must refresh this page
The Empirical Similarity Approach For Volatility Prediction
DOWNLOAD
Author : Vasyl Golosnoy
language : en
Publisher:
Release Date : 2014
The Empirical Similarity Approach For Volatility Prediction written by Vasyl Golosnoy and has been published by this book supported file pdf, txt, epub, kindle and other format this book has been release on 2014 with categories.
In this paper we adapt the empirical similarity (ES) concept for the purpose of combining forecasts originating from different models. Our ES approach is suitable for situations where a decision maker refrains from evaluating success probabilities of forecasting models but prefers to think by analogy. It allows to determine weights of the forecasting combination by quantifying distances between model predictions and corresponding realizations of the process of interest as they are perceived by decision makers. The proposed ES approach is applied for combining models in order to forecast daily volatility of the major stock market indices.
Forecasting Volatility With Empirical Similarity And Google Trends
DOWNLOAD
Author : Moritz Heiden
language : en
Publisher:
Release Date : 2015
Forecasting Volatility With Empirical Similarity And Google Trends written by Moritz Heiden and has been published by this book supported file pdf, txt, epub, kindle and other format this book has been release on 2015 with categories.
This paper proposes an empirical similarity approach to forecast weekly volatility by using search engine data as a measure of investors attention to the stock market index. Our model is assumption free with respect to the underlying process of investors attention and significantly outperforms conventional time-series models in an out-of-sample forecasting framework. We find that especially in high-volatility market phases prediction accuracy increases together with investor attention. The practical implications for risk management are highlighted in a Value-at-Risk forecasting exercise, where our model produces significantly more accurate forecasts while requiring less capital due to fewer overpredictions.
Handbook Of Volatility Models And Their Applications
DOWNLOAD
Author : Luc Bauwens
language : en
Publisher: John Wiley & Sons
Release Date : 2012-03-22
Handbook Of Volatility Models And Their Applications written by Luc Bauwens and has been published by John Wiley & Sons this book supported file pdf, txt, epub, kindle and other format this book has been release on 2012-03-22 with Business & Economics categories.
A complete guide to the theory and practice of volatility models in financial engineering Volatility has become a hot topic in this era of instant communications, spawning a great deal of research in empirical finance and time series econometrics. Providing an overview of the most recent advances, Handbook of Volatility Models and Their Applications explores key concepts and topics essential for modeling the volatility of financial time series, both univariate and multivariate, parametric and non-parametric, high-frequency and low-frequency. Featuring contributions from international experts in the field, the book features numerous examples and applications from real-world projects and cutting-edge research, showing step by step how to use various methods accurately and efficiently when assessing volatility rates. Following a comprehensive introduction to the topic, readers are provided with three distinct sections that unify the statistical and practical aspects of volatility: Autoregressive Conditional Heteroskedasticity and Stochastic Volatility presents ARCH and stochastic volatility models, with a focus on recent research topics including mean, volatility, and skewness spillovers in equity markets Other Models and Methods presents alternative approaches, such as multiplicative error models, nonparametric and semi-parametric models, and copula-based models of (co)volatilities Realized Volatility explores issues of the measurement of volatility by realized variances and covariances, guiding readers on how to successfully model and forecast these measures Handbook of Volatility Models and Their Applications is an essential reference for academics and practitioners in finance, business, and econometrics who work with volatility models in their everyday work. The book also serves as a supplement for courses on risk management and volatility at the upper-undergraduate and graduate levels.
Interdisciplinary Approaches To Understanding And Forecasting Volatility
DOWNLOAD
Author : Irena Vodenska-Chitkushev
language : en
Publisher:
Release Date : 2009
Interdisciplinary Approaches To Understanding And Forecasting Volatility written by Irena Vodenska-Chitkushev and has been published by this book supported file pdf, txt, epub, kindle and other format this book has been release on 2009 with categories.
Abstract: Volatility is a measure of financial market risk and understanding the statistical characteristics of volatility is essential for effective risk management. In this thesis we use statistical physics approaches to analyze the stochastic nature of financial markets and explore the existence of a universal law that governs the financial market system. Such universal law will allow us to study the statistical characteristics of extreme events for which the data is limited, by analyzing more common events where the data is abundant. We use the analysis of return intervals to study the volatility of the S&P 500 Index for different periods between 1984 and 2009, and explore the existence of memory and scaling in the return intervals datasets. Our results show that the long memory in volatility leads to a clustering of above-median as well as below-median return intervals. In addition, we find that the short return intervals form larger clusters compared to the long return intervals. We also study specific market crashes and the behavior of the market after such crashes. We find that the crashes are characterized by the Omori law, which describes the decay in the rate of aftershocks of a given size. We find that within the aftercrash period there are smaller shocks that themselves constitute Omori processes on smaller scales, similar to the Omori process after the large crash. To further analyze the statistical characteristics of the S&P 500 index data, we compare the empirical results with two models, autoregressive moving average - fractionally integrated generalized autoregressive conditional heteroskedastic (ARMA-FIGARCH) model and fractional Brownian motion (fBm) model. We observe that in general, the ARMA-FIGARCH model is statistically different from the market behavior for intermediate thresholds, and the fBm model is statistically different from the market data for small and large thresholds. Also, both ARMA-FIGARCH and fBm capture the long-term dependence in return intervals to a certain extent, but only fBm accounts for the scaling. Finally, we propose a novel method for forecasting high and low volatility periods based on the long memory in the S&P 500 return intervals. We then analyze different derivative-based strategies and compare them with the "long only" strategy where only long equity positions are held and no derivatives are used. Our findings suggest that a protective put option strategy significantly outperforms the "long only" strategy during high volatility periods, while it underperforms the "long only" strategy during periods of low volatility. On the other hand, the covered call strategy does not offer proper protection of the portfolio for high volatility periods, and has limited upside potential when volatility is low.
A Symbolic Dynamics Approach To Volatility Prediction
DOWNLOAD
Author : Peter Tino
language : en
Publisher:
Release Date : 1998
A Symbolic Dynamics Approach To Volatility Prediction written by Peter Tino and has been published by this book supported file pdf, txt, epub, kindle and other format this book has been release on 1998 with categories.
Empirical Evidence On The Importance Of Aggregation Asymmetry And Jumps For Volatility Prediction
DOWNLOAD
Author : Diep Duong
language : en
Publisher:
Release Date : 2013
Empirical Evidence On The Importance Of Aggregation Asymmetry And Jumps For Volatility Prediction written by Diep Duong and has been published by this book supported file pdf, txt, epub, kindle and other format this book has been release on 2013 with categories.
Many recent modelling advances in finance topics ranging from the pricing of volatility-based derivative products to asset management are predicated on the importance of jumps, or discontinuous movements in asset returns. In light of this, a number of recent papers have addressed volatility predictability, some from the perspective of the usefulness of jumps in forecasting volatility. Key papers in this area include Andersen, Bollerslev, Diebold and Labys (2003), Corsi (2004), Andersen, Bollerslev and Diebold (2007), Corsi, Pirino and Reno (2008), Barndorff, Kinnebrock, and Shephard (2010), Patton and Shephard (2011), and the references cited therein. In this paper, we review the extant literature and then present new empirical evidence on the predictive content of realized measures of jump power variations (including upside and downside risk, jump asymmetry, and truncated jump variables), constructed using instantaneous returns, i.e., |r_{t}|^{q}, 0≤q≤6, in the spirit of Ding, Granger and Engle (1993) and Ding and Granger (1996). Our prediction experiments use high frequency price returns constructed using S&P500 futures data as well as stocks in the Dow 30; and our empirical implementation involves estimating linear and nonlinear heterogeneous autoregressive realized volatility (HAR-RV) type models. We find that past "large" jump power variations help less in the prediction of future realized volatility, than past "small" jump power variations. Additionally, we find evidence that past realized signed jump power variations, which have not previously been examined in this literature, are strongly correlated with future volatility, and that past downside jump variations matter in prediction. Finally, incorporation of downside and upside jump power variations does improve predictability, albeit to a limited extent.
Volatility Forecasting Models
DOWNLOAD
Author : Marcial Messmer
language : en
Publisher:
Release Date : 2012
Volatility Forecasting Models written by Marcial Messmer and has been published by this book supported file pdf, txt, epub, kindle and other format this book has been release on 2012 with categories.
We compare two more sophisticated GARCH-like models in terms of their out-of-sample forecasting power. Namely, we consider the tree-structured GARCH introduced by Audrino and Bühlmann (2001) and the GARCHMIDAS by Engle, Ghysels, and Sohn (2008). Additionally, we include the classical GARCH(1,1). We estimate the models using daily S&P 500 futures data. The models are evaluated out-of-sample based on the Diebold and Mariano Test, where the volatility proxy is constructed from high frequency data. We find evidence that the tree-structured GARCH is superior to the GARCH-MIDAS model and to the GARCH(1,1).
Volatility Estimation And Price Prediction Using A Hidden Markov Model With Empirical Study
DOWNLOAD
Author : Pei Yin
language : en
Publisher:
Release Date : 2007
Volatility Estimation And Price Prediction Using A Hidden Markov Model With Empirical Study written by Pei Yin and has been published by this book supported file pdf, txt, epub, kindle and other format this book has been release on 2007 with Capital investments categories.
This work provides a solid development of a hidden Markov model (HMM) from the economic insight to the mathematic formulation. In this model, we assume both drift and volatility of the security return process are driven by certain underlying economic forces which evolve together as a finite-state, time-invariant Markov chain. Unfortunately, this chain is unobservable. Through stochastic filtering techniques and EM algorithm with modified iteration steps, we estimate the state space and transition matrix of the Markov chain, as well as the state spaces of the drift and volatility. With these estimates we can smooth and predict the drift and volatility processes and apply them to the security price prediction. On an empirical level, we first use Monte Carlo simulation to show the robustness of our estimates, and then implement HMM on various data sets of historical prices including: major indices, bonds, mutual funds, common stocks, and ETFs to back test the predicability of the model. Moreover, we compare the applicability of HMM with the well established GARCH(1,1) model, as far as the prediction performance is concerned, our results indicate HMM outperforms GARCH(1,1).
Topics In Modeling Volatility Based On High Frequency Data
DOWNLOAD
Author : Constantin Roth
language : en
Publisher:
Release Date : 2018
Topics In Modeling Volatility Based On High Frequency Data written by Constantin Roth and has been published by this book supported file pdf, txt, epub, kindle and other format this book has been release on 2018 with categories.
In the first chapter, I compare the forecasting accuracy of different high-frequency based volatility models. The empirical analysis shows that the HEAVY and the Realized GARCH generally outperform the rest of the models. The inclusion of overnight returns considerably improves volatility forecasts for stocks across all models. Furthermore, the analysis shows that models based on realized volatility benefit much less from allowing leverage effects than do models based on daily returns. In the second chapter, the cause for this observation is investigated more deeply. I explain it by documenting that realized volatility tends to be higher on down-days than on up-days and that a similar asymmetry cannot be found in squared daily returns. I show that leverage effects are present already at high return-frequencies and that these are capable of generating asymmetries in realized variance but not in squared returns. In the third chapter, a conservative test based on the adaptive lasso is applied to investigate the optimal lag structure for modeling realized volatility dynamics. The empirical analysis shows that the optimal significant lag structure is time-varying and subject to drastic regime shifts. The accuracy of the HAR model can be explained by the observation that in many cases the relevant information for prediction is included in the first 22 lags. In the fourth chapter, a wild multiplicative bootstrap is introduced for M- and GMM estimators of time series. In Monte Carlo simulations, the wild bootstrap always outperforms inference which is based on standard asymptotic theory. Moreover, in most cases the accuracy of the wild bootstrap is also higher and more stable than that of the block bootstrap whose accuracy depends heavily on the choice of the block size.
A Machine Learning Approach To Volatility Forecasting
DOWNLOAD
Author : Kim Christensen
language : en
Publisher:
Release Date : 2021
A Machine Learning Approach To Volatility Forecasting written by Kim Christensen and has been published by this book supported file pdf, txt, epub, kindle and other format this book has been release on 2021 with categories.