Visual Saliency Computation
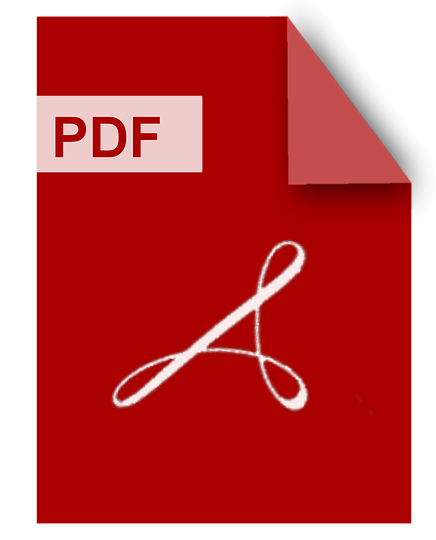
DOWNLOAD
Download Visual Saliency Computation PDF/ePub or read online books in Mobi eBooks. Click Download or Read Online button to get Visual Saliency Computation book now. This website allows unlimited access to, at the time of writing, more than 1.5 million titles, including hundreds of thousands of titles in various foreign languages. If the content not found or just blank you must refresh this page
Visual Saliency Computation
DOWNLOAD
Author : Jia Li
language : en
Publisher: Springer
Release Date : 2014-04-12
Visual Saliency Computation written by Jia Li and has been published by Springer this book supported file pdf, txt, epub, kindle and other format this book has been release on 2014-04-12 with Computers categories.
This book covers fundamental principles and computational approaches relevant to visual saliency computation. As an interdisciplinary problem, visual saliency computation is introduced in this book from an innovative perspective that combines both neurobiology and machine learning. The book is also well-structured to address a wide range of readers, from specialists in the field to general readers interested in computer science and cognitive psychology. With this book, a reader can start from the very basic question of "what is visual saliency?" and progressively explore the problems in detecting salient locations, extracting salient objects, learning prior knowledge, evaluating performance, and using saliency in real-world applications. It is highly expected that this book will spark a great interest of research in the related communities in years to come.
Visual Saliency Computation
DOWNLOAD
Author : Jia Li
language : en
Publisher:
Release Date : 2014-05-19
Visual Saliency Computation written by Jia Li and has been published by this book supported file pdf, txt, epub, kindle and other format this book has been release on 2014-05-19 with categories.
Visual Saliency From Pixel Level To Object Level Analysis
DOWNLOAD
Author : Jianming Zhang
language : en
Publisher: Springer
Release Date : 2019-01-21
Visual Saliency From Pixel Level To Object Level Analysis written by Jianming Zhang and has been published by Springer this book supported file pdf, txt, epub, kindle and other format this book has been release on 2019-01-21 with Computers categories.
This book provides an introduction to recent advances in theory, algorithms and application of Boolean map distance for image processing. Applications include modeling what humans find salient or prominent in an image, and then using this for guiding smart image cropping, selective image filtering, image segmentation, image matting, etc. In this book, the authors present methods for both traditional and emerging saliency computation tasks, ranging from classical low-level tasks like pixel-level saliency detection to object-level tasks such as subitizing and salient object detection. For low-level tasks, the authors focus on pixel-level image processing approaches based on efficient distance transform. For object-level tasks, the authors propose data-driven methods using deep convolutional neural networks. The book includes both empirical and theoretical studies, together with implementation details of the proposed methods. Below are the key features for different types of readers. For computer vision and image processing practitioners: Efficient algorithms based on image distance transforms for two pixel-level saliency tasks; Promising deep learning techniques for two novel object-level saliency tasks; Deep neural network model pre-training with synthetic data; Thorough deep model analysis including useful visualization techniques and generalization tests; Fully reproducible with code, models and datasets available. For researchers interested in the intersection between digital topological theories and computer vision problems: Summary of theoretic findings and analysis of Boolean map distance; Theoretic algorithmic analysis; Applications in salient object detection and eye fixation prediction. Students majoring in image processing, machine learning and computer vision: This book provides up-to-date supplementary reading material for course topics like connectivity based image processing, deep learning for image processing; Some easy-to-implement algorithms for course projects with data provided (as links in the book); Hands-on programming exercises in digital topology and deep learning.
A Discriminant Hypothesis For Visual Saliency
DOWNLOAD
Author : Dashan Gao
language : en
Publisher:
Release Date : 2008
A Discriminant Hypothesis For Visual Saliency written by Dashan Gao and has been published by this book supported file pdf, txt, epub, kindle and other format this book has been release on 2008 with categories.
It has long been known that visual attention and saliency mechanisms play an important role in human visual perception. However, there have been no computational principles that could explain the fundamental properties of biological visual saliency. In this thesis, we propose, and study the plausibility of a novel principle for human visual saliency, which we denote as discriminant saliency hypothesis. The hypothesis states that all saliency decisions are optimal in a decision-theoretic sense. Under this formulation, optimality is defined in the minimum probability of error sense, under a constraint of computational parsimony. The discriminant saliency hypothesis naturally adapts to both stimulus-driven (bottom-up) and goal-driven (top-down) saliency problems, for which we derive the optimal discriminant saliency detectors, in an information-theoretic sense. Statistical properties of natural stimuli are also exploited in the derivation for the constraint of computational parsimony. To study the biological plausibility of discriminant saliency, we show that, under the assumption that saliency is driven by linear filtering, the computations of discriminant saliency are completely consistent with the standard neural architecture in the primary visual cortex (V1). The discriminant saliency detectors are also applied to the set of classical displays, used in the study of human saliency behaviors, and shown to explain both qualitative and quantitative properties of human saliency. These results not only justify the biological plausibility of the discriminant hypothesis for saliency, but also offer explanations to the neural organization of perceptual systems. For example, we show that the basic neural structures in V1 are capable of computing the fundamental operations of statistical inference, e.g., assessment of probabilities, implementation of decision rules, and feature selection. Finally, we evaluate the performance of the derived discriminant saliency detectors for computer vision problems. In particular, we apply the top-down saliency detector to the problem of weakly supervised learning for object recognition, and show that the detector outperforms the state-of-the-art saliency detectors in 1) capturing important information for object recognition tasks, 2) accurately localizing objects of interest from image clutter, 3) providing stable salient locations with respect to various geometric and photometric transformations, and 4) adapting to diverse visual attributes for saliency. We then evaluate the performance of the bottom-up discriminant saliency detector in the applications where no recognition is defined. In particular, we show that the bottom-up discriminant saliency implementation accurately predicts human eye fixation locations on natural scenes. In another application of discriminant saliency, we discuss a Bayesian framework to integrate top-down and bottom-up saliency outputs, where the top-down saliency is interpreted as a focus-of-attention mechanism. Experimental results show that this framework combines the selectivity of the top-down saliency with the localization ability of the bottom-up interest point detectors, and improves the object recognition performance. Overall, the excellent performance of discriminant saliency in both biological and computer vision evaluations justifies the plausibility of discriminant hypothesis as an explanation for human visual saliency.
Linear Vs Nonlinear Feature Combination For Saliency Computation A Comparison With Human Vision
DOWNLOAD
Author :
language : en
Publisher:
Release Date :
Linear Vs Nonlinear Feature Combination For Saliency Computation A Comparison With Human Vision written by and has been published by this book supported file pdf, txt, epub, kindle and other format this book has been release on with categories.
In the heart of the computer model of visual attention, an interest or saliency map is derived from an input image in a process that encompasses several data combination steps. While several combination strategies are possible and the choice of a method influences the final saliency substantially, there is a real need for a performance comparison for the purpose of model improvement. This paper presents contributing work in which model performances are measured by comparing saliency maps with human eye fixations. Four combination methods are compared in experiments involving the viewing of 40 images by 20 observers. Similarity is evaluated qualitatively by visual tests and quantitatively by use of a similarity score. With similarity scores lying 100% higher, non-linear combinations outperform linear methods. The comparison with human vision thus shows the superiority of non-linear over linear combination schemes and speaks for their preferred use in computer models.
Optimal Cue Combination For Saliency Computation A Comparison With Human Vision
DOWNLOAD
Author :
language : en
Publisher:
Release Date :
Optimal Cue Combination For Saliency Computation A Comparison With Human Vision written by and has been published by this book supported file pdf, txt, epub, kindle and other format this book has been release on with categories.
The computer model of visual attention derives an interest or saliency map from an input image in a process that encompasses several data combination steps. While several combination strategies are possible, not all perform equally well. This paper compares main cue combination strategies by measuring the performance of the considered models with respect to human eye movements. Six main combination methods are compared in experiments involving the viewing of 40 images by 20 observers. Similarity is evaluated qualitatively by visual tests and quantitatively by use of a similarity score. The study provides insight into the map combination mechanisms and proposes in this respect an overall optimal strategy for a computer saliency model.
Advanced Computational Models For Human Fixation Prediction And Visual Saliency Detection
DOWNLOAD
Author : 林汝喆
language : en
Publisher:
Release Date : 2015
Advanced Computational Models For Human Fixation Prediction And Visual Saliency Detection written by 林汝喆 and has been published by this book supported file pdf, txt, epub, kindle and other format this book has been release on 2015 with categories.
From 2d To Stereoscopic 3d Visual Saliency
DOWNLOAD
Author : Junle Wang
language : fr
Publisher:
Release Date : 2012
From 2d To Stereoscopic 3d Visual Saliency written by Junle Wang and has been published by this book supported file pdf, txt, epub, kindle and other format this book has been release on 2012 with categories.
L'attention visuelle est l'un des mécanismes les plus importants mis en oeuvre par le système visuel humain (SVH) afin de réduire la quantité d'information que le cerveau a besoin de traiter pour appréhender le contenu d'une scène. Un nombre croissant de travaux est consacré à l'étude de l'attention visuelle, et en particulier à sa modélisation computationnelle. Dans cette thèse, nous présentons des études portant sur plusieurs aspects de cette recherche. Nos travaux peuvent être classés globalement en deux parties. La première concerne les questions liées à la vérité de terrain utilisée, la seconde est relative à la modélisation de l'attention visuelle dans des conditions de visualisation 3D. Dans la première partie, nous analysons la fiabilité de cartes de densité de fixation issues de différentes bases de données occulométriques. Ensuite, nous identifions quantitativement les similitudes et les différences entre carte de densité de fixation et carte d'importance visuelle, ces deux types de carte étant les vérités de terrain communément utilisées par les applications relatives à l'attention. Puis, pour faire face au manque de vérité de terrain exploitable pour la modélisation de l'attention visuelle 3D, nous procédons à une expérimentation oculométrique binoculaire qui aboutit à la création d'une nouvelle base de données avec des images stéréoscopiques 3D. Dans la seconde partie, nous commençons par examiner l'impact de la profondeur sur l'attention visuelle dans des conditions de visualisation 3D. Nous quantifions d'abord le " biais de profondeur " lié à la visualisation de contenus synthétiques 3D sur écran plat stéréoscopique. Ensuite, nous étendons notre étude avec l'usage d'images 3D au contenu naturel. Nous proposons un modèle de l'attention visuelle 3D basé saillance de profondeur, modèle qui repose sur le contraste de profondeur de la scène. Deux façons différentes d'exploiter l'information de profondeur par notre modèle sont comparées. Ensuite, nous étudions le biais central et les différences qui existent selon que les conditions de visualisation soient 2D ou 3D. Nous intégrons aussi le biais central à notre modèle de l'attention visuelle 3D. Enfin, considérant que l'attention visuelle combinée à une technique de floutage peut améliorer la qualité d'expérience de la TV-3D, nous étudions l'influence de flou sur la perception de la profondeur, et la relation du flou avec la disparité binoculaire.
Visual Saliency Application In Object Detection For Search Space Reduction
DOWNLOAD
Author : Sai Rama Srivatsava Gorthy
language : en
Publisher:
Release Date : 2017
Visual Saliency Application In Object Detection For Search Space Reduction written by Sai Rama Srivatsava Gorthy and has been published by this book supported file pdf, txt, epub, kindle and other format this book has been release on 2017 with Computer vision categories.
Vision is the ability to see and interpret any visual stimulus. It is one of the most fundamental and complex tasks the brain performs. Its complexity can be understood from the fact that close to 50% of the human brain is dedicated to vision. The brain receives an overwhelming amount of sensory information from the retina - estimated at up to 100 Mbps per optic nerve. Parallel processing of the entire visual field in real time is likely impossible for even the most sophisticated brains due to the high computational complexity of the task [1]. Yet, organisms can efficiently process this information to parse complex scenes in real time. This amazing feat of nature relies on selective attention which allows the brain to filter sensory information to select only a small subset of it for further processing. Today, Computer Vision has become ubiquitous in our society with several in image understanding, medicine, drones, self-driving cars and many more. With the advent of GPUs and the availability of huge datasets like ImageNet, Convolutional Neural Networks (CNNs) have come to play a very important role in solving computer vision tasks, e.g object detection. However, the size of the networks become prohibitive when higher accuracies are needed, which in turn demands more hardware. This hinders the application of CNNs to mobile platforms and stops them from hitting the real-time mark. The computational efficiency of a computer vision task, like object detection, can be enhanced by adopting a selective attention mechanism into the algorithm. In this work, this idea is explored by using Visual Proto Object Saliency algorithm [1] to crop out the areas of an image without relevant objects before a computationally intensive network like the Faster R-CNN [2] processes it.
Saliency And Tracking In Compressed Video
DOWNLOAD
Author : SayedHossein Khatoonabadi
language : en
Publisher:
Release Date : 2015
Saliency And Tracking In Compressed Video written by SayedHossein Khatoonabadi and has been published by this book supported file pdf, txt, epub, kindle and other format this book has been release on 2015 with categories.
Visual saliency is the propensity of a part of the scene to attract attention. Computational modeling of visual saliency has become an important research problem in recent years, with applications in quality assessment, compression, object tracking, and so on. While most saliency estimation models for dynamic scenes operate on raw video, their high computational complexity is a serious drawback when it comes to practical applications. Our approach for decreasing the complexity and memory requirements is to avoid decoding the compressed bitstream as much as possible. Since most modern cameras incorporate video encoders, this paves the way for in-camera saliency estimation, which could be useful in a variety of computer vision applications. In this dissertation we present compressed-domain features that are highly indicative of saliency in natural video. Using these features, we construct two simple and effective saliency estimation models for compressed video. The proposed models have been extensively tested on two ground truth datasets using several accuracy metrics, and shown to yield considerable improvement over several state-of-the-art compressed-domain and pixel-domain saliency models. Another contribution is a tracking algorithm that also uses only compressed-domain information to isolate moving regions and estimate their trajectories. The algorithm has been tested on a number of standard sequences, and the results demonstrate its advantages over state-of-the-art for compressed-domain tracking and segmentation, with over 30% improvement in F-measure.