Analysis Of Missing Data With Random Forests
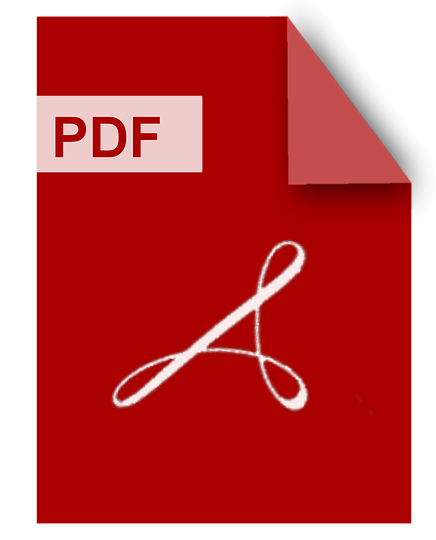
DOWNLOAD
Download Analysis Of Missing Data With Random Forests PDF/ePub or read online books in Mobi eBooks. Click Download or Read Online button to get Analysis Of Missing Data With Random Forests book now. This website allows unlimited access to, at the time of writing, more than 1.5 million titles, including hundreds of thousands of titles in various foreign languages. If the content not found or just blank you must refresh this page
Analysis Of Missing Data With Random Forests
DOWNLOAD
Author : Alexander Hapfelmeier
language : en
Publisher:
Release Date : 2012
Analysis Of Missing Data With Random Forests written by Alexander Hapfelmeier and has been published by this book supported file pdf, txt, epub, kindle and other format this book has been release on 2012 with categories.
Random Forest Missing Data Approaches
DOWNLOAD
Author : Fei Tang
language : en
Publisher:
Release Date : 2017
Random Forest Missing Data Approaches written by Fei Tang and has been published by this book supported file pdf, txt, epub, kindle and other format this book has been release on 2017 with categories.
Random forest (RF) missing data algorithms are an attractive approach for imputing missing data. They have the desirable properties of being able to handle mixed types of missing data, they are adaptive to interactions and nonlinearity, and they have the potential to scale to big data settings. Currently there are many different RF imputation algorithms, but relatively little guidance about their efficacy. Using a large, diverse collection of data sets, imputation performance of various RF algorithms was assessed under different missing data mechanisms. Algorithms included proximity imputation, on the fly imputation, and imputation utilizing multivariate unsupervised and supervised splittingthe latter class representing a generalization of a new promising imputation algorithm called missForest. Our findings reveal RF imputation to be generally robust with performance improving with increasing correlation. Performance was good under moderate to high missingness, and even (in certain cases) when data was missing not at random. Real data analysis using the RF imputation methods was conducted on the MESA data.
Optimization Of Random Forest Based Methods Applying The Genetic Algorithms
DOWNLOAD
Author : Zahra Aback
language : en
Publisher:
Release Date : 2018
Optimization Of Random Forest Based Methods Applying The Genetic Algorithms written by Zahra Aback and has been published by this book supported file pdf, txt, epub, kindle and other format this book has been release on 2018 with categories.
In this century access to large and complex datasets is much easier. These datasets are large in dimension and volume, and researchers are interested in methods that are able to handle this type of data and at the same time produce accurate results. Machine learning methods are particularly efficient for this type of data, where the emphasis is on data analysis, and not on fitting a statistical model. A very popular method from this group is Random Forests which have been applied in different areas of study on two types of problems: classification and regression. The former is more popular, while the latter can be applied for data analysis. Moreover, many efficient techniques for missing value imputation were added to Random Forest over time. One of these methods which can handle all types of variables is MissForest. There are several studies that applied different approaches to improve the performance of classification type of Random Forests, but there are not many studies available for regression type. In the present study, it is evaluated if the performance of regression type of Random Forests and MissForests could be improved by applying Genetic Algorithms as an optimization method. The experiments were conducted on five datasets to minimize the mean square error (MSE) of the Random Forest and imputation errors of the MissForest. The results showed the superiority of the proposed method in comparison to the classical Random Forest methods.
Flexible Imputation Of Missing Data Second Edition
DOWNLOAD
Author : Stef van Buuren
language : en
Publisher: CRC Press
Release Date : 2018-07-17
Flexible Imputation Of Missing Data Second Edition written by Stef van Buuren and has been published by CRC Press this book supported file pdf, txt, epub, kindle and other format this book has been release on 2018-07-17 with Mathematics categories.
Missing data pose challenges to real-life data analysis. Simple ad-hoc fixes, like deletion or mean imputation, only work under highly restrictive conditions, which are often not met in practice. Multiple imputation replaces each missing value by multiple plausible values. The variability between these replacements reflects our ignorance of the true (but missing) value. Each of the completed data set is then analyzed by standard methods, and the results are pooled to obtain unbiased estimates with correct confidence intervals. Multiple imputation is a general approach that also inspires novel solutions to old problems by reformulating the task at hand as a missing-data problem. This is the second edition of a popular book on multiple imputation, focused on explaining the application of methods through detailed worked examples using the MICE package as developed by the author. This new edition incorporates the recent developments in this fast-moving field. This class-tested book avoids mathematical and technical details as much as possible: formulas are accompanied by verbal statements that explain the formula in accessible terms. The book sharpens the reader’s intuition on how to think about missing data, and provides all the tools needed to execute a well-grounded quantitative analysis in the presence of missing data.
Soft Computing For Biomedical Applications And Related Topics
DOWNLOAD
Author : Vladik Kreinovich
language : en
Publisher: Springer Nature
Release Date : 2020-06-29
Soft Computing For Biomedical Applications And Related Topics written by Vladik Kreinovich and has been published by Springer Nature this book supported file pdf, txt, epub, kindle and other format this book has been release on 2020-06-29 with Technology & Engineering categories.
This book presents innovative intelligent techniques, with an emphasis on their biomedical applications. Although many medical doctors are willing to share their knowledge – e.g. by incorporating it in computer-based advisory systems that can benefit other doctors – this knowledge is often expressed using imprecise (fuzzy) words from natural language such as “small,” which are difficult for computers to process. Accordingly, we need fuzzy techniques to handle such words. It is also desirable to extract general recommendations from the records of medical doctors’ decisions – by using machine learning techniques such as neural networks. The book describes state-of-the-art fuzzy, neural, and other techniques, especially those that are now being used, or potentially could be used, in biomedical applications. Accordingly, it will benefit all researchers and students interested in the latest developments, as well as practitioners who want to learn about new techniques.
Random Forests With R
DOWNLOAD
Author : Robin Genuer
language : en
Publisher: Springer Nature
Release Date : 2020-09-10
Random Forests With R written by Robin Genuer and has been published by Springer Nature this book supported file pdf, txt, epub, kindle and other format this book has been release on 2020-09-10 with Mathematics categories.
This book offers an application-oriented guide to random forests: a statistical learning method extensively used in many fields of application, thanks to its excellent predictive performance, but also to its flexibility, which places few restrictions on the nature of the data used. Indeed, random forests can be adapted to both supervised classification problems and regression problems. In addition, they allow us to consider qualitative and quantitative explanatory variables together, without pre-processing. Moreover, they can be used to process standard data for which the number of observations is higher than the number of variables, while also performing very well in the high dimensional case, where the number of variables is quite large in comparison to the number of observations. Consequently, they are now among the preferred methods in the toolbox of statisticians and data scientists. The book is primarily intended for students in academic fields such as statistical education, but also for practitioners in statistics and machine learning. A scientific undergraduate degree is quite sufficient to take full advantage of the concepts, methods, and tools discussed. In terms of computer science skills, little background knowledge is required, though an introduction to the R language is recommended. Random forests are part of the family of tree-based methods; accordingly, after an introductory chapter, Chapter 2 presents CART trees. The next three chapters are devoted to random forests. They focus on their presentation (Chapter 3), on the variable importance tool (Chapter 4), and on the variable selection problem (Chapter 5), respectively. After discussing the concepts and methods, we illustrate their implementation on a running example. Then, various complements are provided before examining additional examples. Throughout the book, each result is given together with the code (in R) that can be used to reproduce it. Thus, the book offers readers essential information and concepts, together with examples and the software tools needed to analyse data using random forests.
Feature Engineering And Selection
DOWNLOAD
Author : Max Kuhn
language : en
Publisher: CRC Press
Release Date : 2019-07-25
Feature Engineering And Selection written by Max Kuhn and has been published by CRC Press this book supported file pdf, txt, epub, kindle and other format this book has been release on 2019-07-25 with Business & Economics categories.
The process of developing predictive models includes many stages. Most resources focus on the modeling algorithms but neglect other critical aspects of the modeling process. This book describes techniques for finding the best representations of predictors for modeling and for nding the best subset of predictors for improving model performance. A variety of example data sets are used to illustrate the techniques along with R programs for reproducing the results.
Hands On Machine Learning With R
DOWNLOAD
Author : Brad Boehmke
language : en
Publisher: CRC Press
Release Date : 2019-11-07
Hands On Machine Learning With R written by Brad Boehmke and has been published by CRC Press this book supported file pdf, txt, epub, kindle and other format this book has been release on 2019-11-07 with Business & Economics categories.
Hands-on Machine Learning with R provides a practical and applied approach to learning and developing intuition into today’s most popular machine learning methods. This book serves as a practitioner’s guide to the machine learning process and is meant to help the reader learn to apply the machine learning stack within R, which includes using various R packages such as glmnet, h2o, ranger, xgboost, keras, and others to effectively model and gain insight from their data. The book favors a hands-on approach, providing an intuitive understanding of machine learning concepts through concrete examples and just a little bit of theory. Throughout this book, the reader will be exposed to the entire machine learning process including feature engineering, resampling, hyperparameter tuning, model evaluation, and interpretation. The reader will be exposed to powerful algorithms such as regularized regression, random forests, gradient boosting machines, deep learning, generalized low rank models, and more! By favoring a hands-on approach and using real word data, the reader will gain an intuitive understanding of the architectures and engines that drive these algorithms and packages, understand when and how to tune the various hyperparameters, and be able to interpret model results. By the end of this book, the reader should have a firm grasp of R’s machine learning stack and be able to implement a systematic approach for producing high quality modeling results. Features: · Offers a practical and applied introduction to the most popular machine learning methods. · Topics covered include feature engineering, resampling, deep learning and more. · Uses a hands-on approach and real world data.
Data Analysis Classification And The Forward Search
DOWNLOAD
Author : Sergio Zani
language : en
Publisher: Springer Science & Business Media
Release Date : 2007-08-06
Data Analysis Classification And The Forward Search written by Sergio Zani and has been published by Springer Science & Business Media this book supported file pdf, txt, epub, kindle and other format this book has been release on 2007-08-06 with Language Arts & Disciplines categories.
This book presents new developments in data analysis, classification and multivariate statistics, and in their algorithmic implementation. The volume offers contributions to the theory of clustering and discrimination, multidimensional data analysis, data mining, and robust statistics with a special emphasis on the novel Forward Search approach. Many papers provide significant insight in a wide range of fields of application. Customer satisfaction and service evaluation are two examples of such emerging fields.
Multivariate Statistical Machine Learning Methods For Genomic Prediction
DOWNLOAD
Author : Osval Antonio Montesinos López
language : en
Publisher: Springer Nature
Release Date : 2022-02-14
Multivariate Statistical Machine Learning Methods For Genomic Prediction written by Osval Antonio Montesinos López and has been published by Springer Nature this book supported file pdf, txt, epub, kindle and other format this book has been release on 2022-02-14 with Technology & Engineering categories.
This book is open access under a CC BY 4.0 license This open access book brings together the latest genome base prediction models currently being used by statisticians, breeders and data scientists. It provides an accessible way to understand the theory behind each statistical learning tool, the required pre-processing, the basics of model building, how to train statistical learning methods, the basic R scripts needed to implement each statistical learning tool, and the output of each tool. To do so, for each tool the book provides background theory, some elements of the R statistical software for its implementation, the conceptual underpinnings, and at least two illustrative examples with data from real-world genomic selection experiments. Lastly, worked-out examples help readers check their own comprehension.The book will greatly appeal to readers in plant (and animal) breeding, geneticists and statisticians, as it provides in a very accessible way the necessary theory, the appropriate R code, and illustrative examples for a complete understanding of each statistical learning tool. In addition, it weighs the advantages and disadvantages of each tool.