Random Forests With R
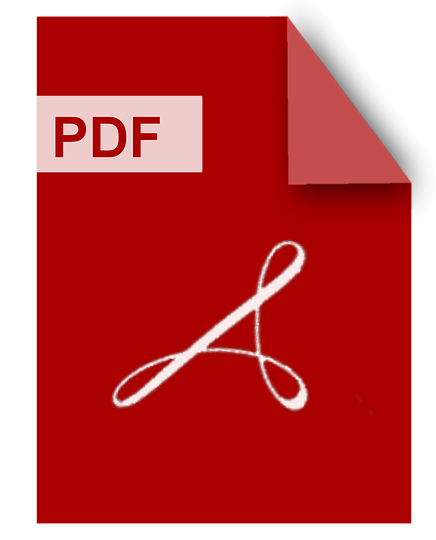
DOWNLOAD
Download Random Forests With R PDF/ePub or read online books in Mobi eBooks. Click Download or Read Online button to get Random Forests With R book now. This website allows unlimited access to, at the time of writing, more than 1.5 million titles, including hundreds of thousands of titles in various foreign languages. If the content not found or just blank you must refresh this page
Random Forests With R
DOWNLOAD
Author : Robin Genuer
language : en
Publisher: Springer Nature
Release Date : 2020-09-10
Random Forests With R written by Robin Genuer and has been published by Springer Nature this book supported file pdf, txt, epub, kindle and other format this book has been release on 2020-09-10 with Mathematics categories.
This book offers an application-oriented guide to random forests: a statistical learning method extensively used in many fields of application, thanks to its excellent predictive performance, but also to its flexibility, which places few restrictions on the nature of the data used. Indeed, random forests can be adapted to both supervised classification problems and regression problems. In addition, they allow us to consider qualitative and quantitative explanatory variables together, without pre-processing. Moreover, they can be used to process standard data for which the number of observations is higher than the number of variables, while also performing very well in the high dimensional case, where the number of variables is quite large in comparison to the number of observations. Consequently, they are now among the preferred methods in the toolbox of statisticians and data scientists. The book is primarily intended for students in academic fields such as statistical education, but also for practitioners in statistics and machine learning. A scientific undergraduate degree is quite sufficient to take full advantage of the concepts, methods, and tools discussed. In terms of computer science skills, little background knowledge is required, though an introduction to the R language is recommended. Random forests are part of the family of tree-based methods; accordingly, after an introductory chapter, Chapter 2 presents CART trees. The next three chapters are devoted to random forests. They focus on their presentation (Chapter 3), on the variable importance tool (Chapter 4), and on the variable selection problem (Chapter 5), respectively. After discussing the concepts and methods, we illustrate their implementation on a running example. Then, various complements are provided before examining additional examples. Throughout the book, each result is given together with the code (in R) that can be used to reproduce it. Thus, the book offers readers essential information and concepts, together with examples and the software tools needed to analyse data using random forests.
Hands On Machine Learning With R
DOWNLOAD
Author : Brad Boehmke
language : en
Publisher: CRC Press
Release Date : 2019-11-07
Hands On Machine Learning With R written by Brad Boehmke and has been published by CRC Press this book supported file pdf, txt, epub, kindle and other format this book has been release on 2019-11-07 with Business & Economics categories.
Hands-on Machine Learning with R provides a practical and applied approach to learning and developing intuition into today’s most popular machine learning methods. This book serves as a practitioner’s guide to the machine learning process and is meant to help the reader learn to apply the machine learning stack within R, which includes using various R packages such as glmnet, h2o, ranger, xgboost, keras, and others to effectively model and gain insight from their data. The book favors a hands-on approach, providing an intuitive understanding of machine learning concepts through concrete examples and just a little bit of theory. Throughout this book, the reader will be exposed to the entire machine learning process including feature engineering, resampling, hyperparameter tuning, model evaluation, and interpretation. The reader will be exposed to powerful algorithms such as regularized regression, random forests, gradient boosting machines, deep learning, generalized low rank models, and more! By favoring a hands-on approach and using real word data, the reader will gain an intuitive understanding of the architectures and engines that drive these algorithms and packages, understand when and how to tune the various hyperparameters, and be able to interpret model results. By the end of this book, the reader should have a firm grasp of R’s machine learning stack and be able to implement a systematic approach for producing high quality modeling results. Features: · Offers a practical and applied introduction to the most popular machine learning methods. · Topics covered include feature engineering, resampling, deep learning and more. · Uses a hands-on approach and real world data.
Computational Genomics With R
DOWNLOAD
Author : Altuna Akalin
language : en
Publisher: CRC Press
Release Date : 2020-12-16
Computational Genomics With R written by Altuna Akalin and has been published by CRC Press this book supported file pdf, txt, epub, kindle and other format this book has been release on 2020-12-16 with Mathematics categories.
Computational Genomics with R provides a starting point for beginners in genomic data analysis and also guides more advanced practitioners to sophisticated data analysis techniques in genomics. The book covers topics from R programming, to machine learning and statistics, to the latest genomic data analysis techniques. The text provides accessible information and explanations, always with the genomics context in the background. This also contains practical and well-documented examples in R so readers can analyze their data by simply reusing the code presented. As the field of computational genomics is interdisciplinary, it requires different starting points for people with different backgrounds. For example, a biologist might skip sections on basic genome biology and start with R programming, whereas a computer scientist might want to start with genome biology. After reading: You will have the basics of R and be able to dive right into specialized uses of R for computational genomics such as using Bioconductor packages. You will be familiar with statistics, supervised and unsupervised learning techniques that are important in data modeling, and exploratory analysis of high-dimensional data. You will understand genomic intervals and operations on them that are used for tasks such as aligned read counting and genomic feature annotation. You will know the basics of processing and quality checking high-throughput sequencing data. You will be able to do sequence analysis, such as calculating GC content for parts of a genome or finding transcription factor binding sites. You will know about visualization techniques used in genomics, such as heatmaps, meta-gene plots, and genomic track visualization. You will be familiar with analysis of different high-throughput sequencing data sets, such as RNA-seq, ChIP-seq, and BS-seq. You will know basic techniques for integrating and interpreting multi-omics datasets. Altuna Akalin is a group leader and head of the Bioinformatics and Omics Data Science Platform at the Berlin Institute of Medical Systems Biology, Max Delbrück Center, Berlin. He has been developing computational methods for analyzing and integrating large-scale genomics data sets since 2002. He has published an extensive body of work in this area. The framework for this book grew out of the yearly computational genomics courses he has been organizing and teaching since 2015.
Ensemble Machine Learning
DOWNLOAD
Author : Cha Zhang
language : en
Publisher: Springer Science & Business Media
Release Date : 2012-02-17
Ensemble Machine Learning written by Cha Zhang and has been published by Springer Science & Business Media this book supported file pdf, txt, epub, kindle and other format this book has been release on 2012-02-17 with Computers categories.
It is common wisdom that gathering a variety of views and inputs improves the process of decision making, and, indeed, underpins a democratic society. Dubbed “ensemble learning” by researchers in computational intelligence and machine learning, it is known to improve a decision system’s robustness and accuracy. Now, fresh developments are allowing researchers to unleash the power of ensemble learning in an increasing range of real-world applications. Ensemble learning algorithms such as “boosting” and “random forest” facilitate solutions to key computational issues such as face recognition and are now being applied in areas as diverse as object tracking and bioinformatics. Responding to a shortage of literature dedicated to the topic, this volume offers comprehensive coverage of state-of-the-art ensemble learning techniques, including the random forest skeleton tracking algorithm in the Xbox Kinect sensor, which bypasses the need for game controllers. At once a solid theoretical study and a practical guide, the volume is a windfall for researchers and practitioners alike.
Small Sample Size Solutions
DOWNLOAD
Author : Rens van de Schoot
language : en
Publisher: Routledge
Release Date : 2020-02-13
Small Sample Size Solutions written by Rens van de Schoot and has been published by Routledge this book supported file pdf, txt, epub, kindle and other format this book has been release on 2020-02-13 with Psychology categories.
Researchers often have difficulties collecting enough data to test their hypotheses, either because target groups are small or hard to access, or because data collection entails prohibitive costs. Such obstacles may result in data sets that are too small for the complexity of the statistical model needed to answer the research question. This unique book provides guidelines and tools for implementing solutions to issues that arise in small sample research. Each chapter illustrates statistical methods that allow researchers to apply the optimal statistical model for their research question when the sample is too small. This essential book will enable social and behavioral science researchers to test their hypotheses even when the statistical model required for answering their research question is too complex for the sample sizes they can collect. The statistical models in the book range from the estimation of a population mean to models with latent variables and nested observations, and solutions include both classical and Bayesian methods. All proposed solutions are described in steps researchers can implement with their own data and are accompanied with annotated syntax in R. The methods described in this book will be useful for researchers across the social and behavioral sciences, ranging from medical sciences and epidemiology to psychology, marketing, and economics.
Decision Forests
DOWNLOAD
Author : Antonio Criminisi
language : en
Publisher: Now Pub
Release Date : 2012-04-01
Decision Forests written by Antonio Criminisi and has been published by Now Pub this book supported file pdf, txt, epub, kindle and other format this book has been release on 2012-04-01 with Computers categories.
Presents a unified, efficient model of random decision forests which can be used in a number of applications such as scene recognition from photographs, object recognition in images, automatic diagnosis from radiological scans and document analysis.
Geocomputation With R
DOWNLOAD
Author : Robin Lovelace
language : en
Publisher: CRC Press
Release Date : 2019-03-22
Geocomputation With R written by Robin Lovelace and has been published by CRC Press this book supported file pdf, txt, epub, kindle and other format this book has been release on 2019-03-22 with Mathematics categories.
Geocomputation with R is for people who want to analyze, visualize and model geographic data with open source software. It is based on R, a statistical programming language that has powerful data processing, visualization, and geospatial capabilities. The book equips you with the knowledge and skills to tackle a wide range of issues manifested in geographic data, including those with scientific, societal, and environmental implications. This book will interest people from many backgrounds, especially Geographic Information Systems (GIS) users interested in applying their domain-specific knowledge in a powerful open source language for data science, and R users interested in extending their skills to handle spatial data. The book is divided into three parts: (I) Foundations, aimed at getting you up-to-speed with geographic data in R, (II) extensions, which covers advanced techniques, and (III) applications to real-world problems. The chapters cover progressively more advanced topics, with early chapters providing strong foundations on which the later chapters build. Part I describes the nature of spatial datasets in R and methods for manipulating them. It also covers geographic data import/export and transforming coordinate reference systems. Part II represents methods that build on these foundations. It covers advanced map making (including web mapping), "bridges" to GIS, sharing reproducible code, and how to do cross-validation in the presence of spatial autocorrelation. Part III applies the knowledge gained to tackle real-world problems, including representing and modeling transport systems, finding optimal locations for stores or services, and ecological modeling. Exercises at the end of each chapter give you the skills needed to tackle a range of geospatial problems. Solutions for each chapter and supplementary materials providing extended examples are available at https://geocompr.github.io/geocompkg/articles/.
Multivariate Statistical Machine Learning Methods For Genomic Prediction
DOWNLOAD
Author : Osval Antonio Montesinos López
language : en
Publisher: Springer Nature
Release Date : 2022-02-14
Multivariate Statistical Machine Learning Methods For Genomic Prediction written by Osval Antonio Montesinos López and has been published by Springer Nature this book supported file pdf, txt, epub, kindle and other format this book has been release on 2022-02-14 with Technology & Engineering categories.
This book is open access under a CC BY 4.0 license This open access book brings together the latest genome base prediction models currently being used by statisticians, breeders and data scientists. It provides an accessible way to understand the theory behind each statistical learning tool, the required pre-processing, the basics of model building, how to train statistical learning methods, the basic R scripts needed to implement each statistical learning tool, and the output of each tool. To do so, for each tool the book provides background theory, some elements of the R statistical software for its implementation, the conceptual underpinnings, and at least two illustrative examples with data from real-world genomic selection experiments. Lastly, worked-out examples help readers check their own comprehension.The book will greatly appeal to readers in plant (and animal) breeding, geneticists and statisticians, as it provides in a very accessible way the necessary theory, the appropriate R code, and illustrative examples for a complete understanding of each statistical learning tool. In addition, it weighs the advantages and disadvantages of each tool.
Python Data Science Handbook
DOWNLOAD
Author : Jake VanderPlas
language : en
Publisher: "O'Reilly Media, Inc."
Release Date : 2016-11-21
Python Data Science Handbook written by Jake VanderPlas and has been published by "O'Reilly Media, Inc." this book supported file pdf, txt, epub, kindle and other format this book has been release on 2016-11-21 with Computers categories.
For many researchers, Python is a first-class tool mainly because of its libraries for storing, manipulating, and gaining insight from data. Several resources exist for individual pieces of this data science stack, but only with the Python Data Science Handbook do you get them all—IPython, NumPy, Pandas, Matplotlib, Scikit-Learn, and other related tools. Working scientists and data crunchers familiar with reading and writing Python code will find this comprehensive desk reference ideal for tackling day-to-day issues: manipulating, transforming, and cleaning data; visualizing different types of data; and using data to build statistical or machine learning models. Quite simply, this is the must-have reference for scientific computing in Python. With this handbook, you’ll learn how to use: IPython and Jupyter: provide computational environments for data scientists using Python NumPy: includes the ndarray for efficient storage and manipulation of dense data arrays in Python Pandas: features the DataFrame for efficient storage and manipulation of labeled/columnar data in Python Matplotlib: includes capabilities for a flexible range of data visualizations in Python Scikit-Learn: for efficient and clean Python implementations of the most important and established machine learning algorithms
Machine Learning Essentials
DOWNLOAD
Author : Alboukadel Kassambara
language : en
Publisher: STHDA
Release Date : 2018-03-10
Machine Learning Essentials written by Alboukadel Kassambara and has been published by STHDA this book supported file pdf, txt, epub, kindle and other format this book has been release on 2018-03-10 with Computers categories.
Discovering knowledge from big multivariate data, recorded every days, requires specialized machine learning techniques. This book presents an easy to use practical guide in R to compute the most popular machine learning methods for exploring real word data sets, as well as, for building predictive models. The main parts of the book include: A) Unsupervised learning methods, to explore and discover knowledge from a large multivariate data set using clustering and principal component methods. You will learn hierarchical clustering, k-means, principal component analysis and correspondence analysis methods. B) Regression analysis, to predict a quantitative outcome value using linear regression and non-linear regression strategies. C) Classification techniques, to predict a qualitative outcome value using logistic regression, discriminant analysis, naive bayes classifier and support vector machines. D) Advanced machine learning methods, to build robust regression and classification models using k-nearest neighbors methods, decision tree models, ensemble methods (bagging, random forest and boosting). E) Model selection methods, to select automatically the best combination of predictor variables for building an optimal predictive model. These include, best subsets selection methods, stepwise regression and penalized regression (ridge, lasso and elastic net regression models). We also present principal component-based regression methods, which are useful when the data contain multiple correlated predictor variables. F) Model validation and evaluation techniques for measuring the performance of a predictive model. G) Model diagnostics for detecting and fixing a potential problems in a predictive model. The book presents the basic principles of these tasks and provide many examples in R. This book offers solid guidance in data mining for students and researchers. Key features: - Covers machine learning algorithm and implementation - Key mathematical concepts are presented - Short, self-contained chapters with practical examples.