Machine Learning Essentials
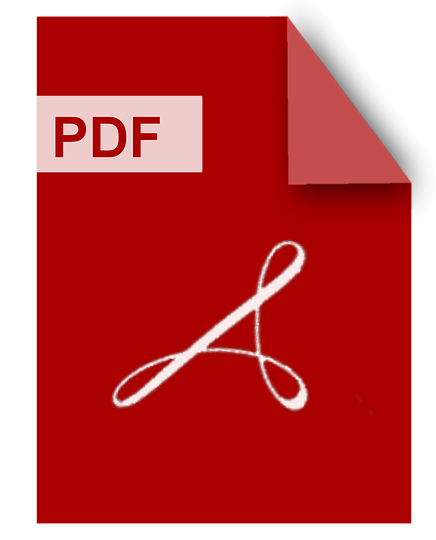
DOWNLOAD
Download Machine Learning Essentials PDF/ePub or read online books in Mobi eBooks. Click Download or Read Online button to get Machine Learning Essentials book now. This website allows unlimited access to, at the time of writing, more than 1.5 million titles, including hundreds of thousands of titles in various foreign languages. If the content not found or just blank you must refresh this page
Machine Learning Essentials
DOWNLOAD
Author : Alboukadel Kassambara
language : en
Publisher: STHDA
Release Date : 2018-03-10
Machine Learning Essentials written by Alboukadel Kassambara and has been published by STHDA this book supported file pdf, txt, epub, kindle and other format this book has been release on 2018-03-10 with Computers categories.
Discovering knowledge from big multivariate data, recorded every days, requires specialized machine learning techniques. This book presents an easy to use practical guide in R to compute the most popular machine learning methods for exploring real word data sets, as well as, for building predictive models. The main parts of the book include: A) Unsupervised learning methods, to explore and discover knowledge from a large multivariate data set using clustering and principal component methods. You will learn hierarchical clustering, k-means, principal component analysis and correspondence analysis methods. B) Regression analysis, to predict a quantitative outcome value using linear regression and non-linear regression strategies. C) Classification techniques, to predict a qualitative outcome value using logistic regression, discriminant analysis, naive bayes classifier and support vector machines. D) Advanced machine learning methods, to build robust regression and classification models using k-nearest neighbors methods, decision tree models, ensemble methods (bagging, random forest and boosting). E) Model selection methods, to select automatically the best combination of predictor variables for building an optimal predictive model. These include, best subsets selection methods, stepwise regression and penalized regression (ridge, lasso and elastic net regression models). We also present principal component-based regression methods, which are useful when the data contain multiple correlated predictor variables. F) Model validation and evaluation techniques for measuring the performance of a predictive model. G) Model diagnostics for detecting and fixing a potential problems in a predictive model. The book presents the basic principles of these tasks and provide many examples in R. This book offers solid guidance in data mining for students and researchers. Key features: - Covers machine learning algorithm and implementation - Key mathematical concepts are presented - Short, self-contained chapters with practical examples.
Microsoft Azure Essentials Azure Machine Learning
DOWNLOAD
Author : Jeff Barnes
language : en
Publisher: Microsoft Press
Release Date : 2015-04-25
Microsoft Azure Essentials Azure Machine Learning written by Jeff Barnes and has been published by Microsoft Press this book supported file pdf, txt, epub, kindle and other format this book has been release on 2015-04-25 with Computers categories.
Microsoft Azure Essentials from Microsoft Press is a series of free ebooks designed to help you advance your technical skills with Microsoft Azure. This third ebook in the series introduces Microsoft Azure Machine Learning, a service that a developer can use to build predictive analytics models (using training datasets from a variety of data sources) and then easily deploy those models for consumption as cloud web services. The ebook presents an overview of modern data science theory and principles, the associated workflow, and then covers some of the more common machine learning algorithms in use today. It builds a variety of predictive analytics models using real world data, evaluates several different machine learning algorithms and modeling strategies, and then deploys the finished models as machine learning web services on Azure within a matter of minutes. The ebook also expands on a working Azure Machine Learning predictive model example to explore the types of client and server applications you can create to consume Azure Machine Learning web services. Watch Microsoft Press’s blog and Twitter (@MicrosoftPress) to learn about other free ebooks in the Microsoft Azure Essentials series.
The Essentials Of Machine Learning In Finance And Accounting
DOWNLOAD
Author : Mohammad Zoynul Abedin
language : en
Publisher: Routledge
Release Date : 2021-06-20
The Essentials Of Machine Learning In Finance And Accounting written by Mohammad Zoynul Abedin and has been published by Routledge this book supported file pdf, txt, epub, kindle and other format this book has been release on 2021-06-20 with Business & Economics categories.
This book introduces machine learning in finance and illustrates how we can use computational tools in numerical finance in real-world context. These computational techniques are particularly useful in financial risk management, corporate bankruptcy prediction, stock price prediction, and portfolio management. The book also offers practical and managerial implications of financial and managerial decision support systems and how these systems capture vast amount of financial data. Business risk and uncertainty are two of the toughest challenges in the financial industry. This book will be a useful guide to the use of machine learning in forecasting, modeling, trading, risk management, economics, credit risk, and portfolio management.
Machine Learning Essentials
DOWNLOAD
Author : Barrett Williams
language : en
Publisher: Barrett Williams
Release Date : 2024-12-01
Machine Learning Essentials written by Barrett Williams and has been published by Barrett Williams this book supported file pdf, txt, epub, kindle and other format this book has been release on 2024-12-01 with Computers categories.
Unlock the potential of data and step into the future with "Machine Learning Essentials," the ultimate guide for mastering predictive analytics. Whether you're a newcomer or looking to deepen your understanding, this comprehensive eBook is designed to equip you with the tools and knowledge you need to excel in the dynamic field of machine learning. Begin your journey by exploring the foundational principles of machine learning and its transformative impact on predictive analytics. Learn how to expertly prepare and engineer your data, selecting and extracting the features that matter most. Dive into handling imbalanced data with precision, ensuring your models are accurate and robust. Discover the power of classification algorithms with insights into decision trees, random forests, support vector machines, and logistic regression. Transition smoothly into regression techniques, harnessing the potential of linear, polynomial, and regularization methods. Explore the realm of unsupervised learning to unveil predictive insights using clustering methods, dimensionality reduction techniques, and anomaly detection. Evaluate model performance like a pro with cross-validation strategies, confusion matrices, and ROC/AUC metrics. Venture into neural networks, unlocking the basics of their architecture, activation functions, and training methodologies. Delve into advanced deep learning topics with convolutional, recurrent, and generative adversarial networks. Optimize your models through hyperparameter tuning and feature importance analysis, selecting the most effective techniques for your goals. Gain practical business insights by implementing machine learning in marketing analytics, risk assessment, and fraud detection. Familiarize yourself with essential tools and libraries like Python, Scikit-Learn, TensorFlow, and PyTorch. Learn from real-world case studies in retail, healthcare, and finance, and tackle ethical considerations in algorithmic bias and data security. Prepare for the future with insights into automated machine learning, IoT, and evolving AI technologies. Take practical steps to launch your machine learning journey, setting up your environment and connecting with a vibrant community of practitioners. "Machine Learning Essentials" is your all-in-one resource for gaining actionable expertise and driving innovation in today's data-driven world. Start your learning adventure today and transform your career with this indispensable guide.
Machine Learning Essentials And Applications
DOWNLOAD
Author : Mrs. N. Jayasri
language : en
Publisher: RK Publication
Release Date : 2024-07-27
Machine Learning Essentials And Applications written by Mrs. N. Jayasri and has been published by RK Publication this book supported file pdf, txt, epub, kindle and other format this book has been release on 2024-07-27 with Computers categories.
Machine Learning Essentials and Applications a comprehensive of machine learning's core principles, methodologies, and real-world applications. This book is designed for both beginners and professionals, covering essential topics like supervised and unsupervised learning, neural networks, and deep learning. With clear explanations and practical examples, it connects theory to practice, showcasing machine learning’s impact across industries such as healthcare, finance, and technology. Ideal for readers seeking foundational knowledge and insights into the transformative potential of machine learning in various fields.
Hands On Machine Learning With R
DOWNLOAD
Author : Brad Boehmke
language : en
Publisher: CRC Press
Release Date : 2019-11-07
Hands On Machine Learning With R written by Brad Boehmke and has been published by CRC Press this book supported file pdf, txt, epub, kindle and other format this book has been release on 2019-11-07 with Business & Economics categories.
Hands-on Machine Learning with R provides a practical and applied approach to learning and developing intuition into today’s most popular machine learning methods. This book serves as a practitioner’s guide to the machine learning process and is meant to help the reader learn to apply the machine learning stack within R, which includes using various R packages such as glmnet, h2o, ranger, xgboost, keras, and others to effectively model and gain insight from their data. The book favors a hands-on approach, providing an intuitive understanding of machine learning concepts through concrete examples and just a little bit of theory. Throughout this book, the reader will be exposed to the entire machine learning process including feature engineering, resampling, hyperparameter tuning, model evaluation, and interpretation. The reader will be exposed to powerful algorithms such as regularized regression, random forests, gradient boosting machines, deep learning, generalized low rank models, and more! By favoring a hands-on approach and using real word data, the reader will gain an intuitive understanding of the architectures and engines that drive these algorithms and packages, understand when and how to tune the various hyperparameters, and be able to interpret model results. By the end of this book, the reader should have a firm grasp of R’s machine learning stack and be able to implement a systematic approach for producing high quality modeling results. Features: · Offers a practical and applied introduction to the most popular machine learning methods. · Topics covered include feature engineering, resampling, deep learning and more. · Uses a hands-on approach and real world data.
Machine Learning Essentials And It S Application
DOWNLOAD
Author : Prof. Yogendra Kumar
language : en
Publisher: Academic Guru Publishing House
Release Date : 2024-08-05
Machine Learning Essentials And It S Application written by Prof. Yogendra Kumar and has been published by Academic Guru Publishing House this book supported file pdf, txt, epub, kindle and other format this book has been release on 2024-08-05 with Study Aids categories.
The book "Machine Learning Essentials and Its Applications" is an informative investigation of the basic concepts of machine learning as well as the many applications of this fascinating field. The fundamental ideas, methods, and algorithms that provide the foundation of machine learning are presented in this book in a format that is designed to lead readers through the process. In order to ensure that the reader has a complete grasp of the discipline, it covers a broad variety of topics, such as supervised and unsupervised learning, neural networks, natural language processing, and computer vision. In addition to providing theoretical information, the book has an emphasis on practical applications, demonstrating how machine learning can be used in a variety of fields, including healthcare, finance, transportation, and entertainment, among others. Every chapter contains case studies and hands-on activities to help readers get a more in-depth grasp of the subject matter and to motivate them to apply what they have learnt in the classroom to situations that they will encounter in the real world. The purpose of this book is to serve as a vital resource for everyone who is interested in understanding the transformational potential of machine learning. It was designed for students, instructors, and industry experts. The book "Machine Learning Essentials and Its Applications" is a necessary travel companion on iv the path to becoming an expert in this rapidly evolving topic since it provides lucid explanations, examples that illustrate the concepts, and important insights.
Deep Learning
DOWNLOAD
Author : John D. Kelleher
language : en
Publisher: MIT Press
Release Date : 2019-09-10
Deep Learning written by John D. Kelleher and has been published by MIT Press this book supported file pdf, txt, epub, kindle and other format this book has been release on 2019-09-10 with Computers categories.
An accessible introduction to the artificial intelligence technology that enables computer vision, speech recognition, machine translation, and driverless cars. Deep learning is an artificial intelligence technology that enables computer vision, speech recognition in mobile phones, machine translation, AI games, driverless cars, and other applications. When we use consumer products from Google, Microsoft, Facebook, Apple, or Baidu, we are often interacting with a deep learning system. In this volume in the MIT Press Essential Knowledge series, computer scientist John Kelleher offers an accessible and concise but comprehensive introduction to the fundamental technology at the heart of the artificial intelligence revolution. Kelleher explains that deep learning enables data-driven decisions by identifying and extracting patterns from large datasets; its ability to learn from complex data makes deep learning ideally suited to take advantage of the rapid growth in big data and computational power. Kelleher also explains some of the basic concepts in deep learning, presents a history of advances in the field, and discusses the current state of the art. He describes the most important deep learning architectures, including autoencoders, recurrent neural networks, and long short-term networks, as well as such recent developments as Generative Adversarial Networks and capsule networks. He also provides a comprehensive (and comprehensible) introduction to the two fundamental algorithms in deep learning: gradient descent and backpropagation. Finally, Kelleher considers the future of deep learning—major trends, possible developments, and significant challenges.
R Deep Learning Essentials
DOWNLOAD
Author : Mark Hodnett
language : en
Publisher: Packt Publishing Ltd
Release Date : 2018-08-24
R Deep Learning Essentials written by Mark Hodnett and has been published by Packt Publishing Ltd this book supported file pdf, txt, epub, kindle and other format this book has been release on 2018-08-24 with Computers categories.
Implement neural network models in R 3.5 using TensorFlow, Keras, and MXNet Key Features Use R 3.5 for building deep learning models for computer vision and text Apply deep learning techniques in cloud for large-scale processing Build, train, and optimize neural network models on a range of datasets Book Description Deep learning is a powerful subset of machine learning that is very successful in domains such as computer vision and natural language processing (NLP). This second edition of R Deep Learning Essentials will open the gates for you to enter the world of neural networks by building powerful deep learning models using the R ecosystem. This book will introduce you to the basic principles of deep learning and teach you to build a neural network model from scratch. As you make your way through the book, you will explore deep learning libraries, such as Keras, MXNet, and TensorFlow, and create interesting deep learning models for a variety of tasks and problems, including structured data, computer vision, text data, anomaly detection, and recommendation systems. You’ll cover advanced topics, such as generative adversarial networks (GANs), transfer learning, and large-scale deep learning in the cloud. In the concluding chapters, you will learn about the theoretical concepts of deep learning projects, such as model optimization, overfitting, and data augmentation, together with other advanced topics. By the end of this book, you will be fully prepared and able to implement deep learning concepts in your research work or projects. What you will learn Build shallow neural network prediction models Prevent models from overfitting the data to improve generalizability Explore techniques for finding the best hyperparameters for deep learning models Create NLP models using Keras and TensorFlow in R Use deep learning for computer vision tasks Implement deep learning tasks, such as NLP, recommendation systems, and autoencoders Who this book is for This second edition of R Deep Learning Essentials is for aspiring data scientists, data analysts, machine learning developers, and deep learning enthusiasts who are well versed in machine learning concepts and are looking to explore the deep learning paradigm using R. Fundamental understanding of the R language is necessary to get the most out of this book.
Machine Learning Revised And Updated Edition
DOWNLOAD
Author : Ethem Alpaydin
language : en
Publisher: MIT Press
Release Date : 2021-08-17
Machine Learning Revised And Updated Edition written by Ethem Alpaydin and has been published by MIT Press this book supported file pdf, txt, epub, kindle and other format this book has been release on 2021-08-17 with Computers categories.
MIT presents a concise primer on machine learning—computer programs that learn from data and the basis of applications like voice recognition and driverless cars. No in-depth knowledge of math or programming required! Today, machine learning underlies a range of applications we use every day, from product recommendations to voice recognition—as well as some we don’t yet use every day, including driverless cars. It is the basis for a new approach to artificial intelligence that aims to program computers to use example data or past experience to solve a given problem. In this volume in the MIT Press Essential Knowledge series, Ethem Alpaydin offers a concise and accessible overview of “the new AI.” This expanded edition offers new material on such challenges facing machine learning as privacy, security, accountability, and bias. Alpaydin explains that as Big Data has grown, the theory of machine learning—the foundation of efforts to process that data into knowledge—has also advanced. He covers: • The evolution of machine learning • Important learning algorithms and example applications • Using machine learning algorithms for pattern recognition • Artificial neural networks inspired by the human brain • Algorithms that learn associations between instances • Reinforcement learning • Transparency, explainability, and fairness in machine learning • The ethical and legal implicates of data-based decision making A comprehensive introduction to machine learning, this book does not require any previous knowledge of mathematics or programming—making it accessible for everyday readers and easily adoptable for classroom syllabi.