Bayesian Core A Practical Approach To Computational Bayesian Statistics
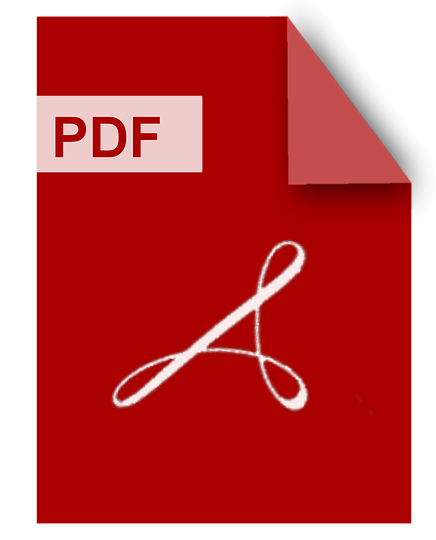
DOWNLOAD
Download Bayesian Core A Practical Approach To Computational Bayesian Statistics PDF/ePub or read online books in Mobi eBooks. Click Download or Read Online button to get Bayesian Core A Practical Approach To Computational Bayesian Statistics book now. This website allows unlimited access to, at the time of writing, more than 1.5 million titles, including hundreds of thousands of titles in various foreign languages. If the content not found or just blank you must refresh this page
Bayesian Core A Practical Approach To Computational Bayesian Statistics
DOWNLOAD
Author : Jean-Michel Marin
language : en
Publisher: Springer Science & Business Media
Release Date : 2007-02-06
Bayesian Core A Practical Approach To Computational Bayesian Statistics written by Jean-Michel Marin and has been published by Springer Science & Business Media this book supported file pdf, txt, epub, kindle and other format this book has been release on 2007-02-06 with Computers categories.
This Bayesian modeling book provides the perfect entry for gaining a practical understanding of Bayesian methodology. It focuses on standard statistical models and is backed up by discussed real datasets available from the book website.
Bayesian Core A Practical Approach To Computational Bayesian Statistics
DOWNLOAD
Author : Jean-Michel Marin
language : en
Publisher: Springer Science & Business Media
Release Date : 2007-05-26
Bayesian Core A Practical Approach To Computational Bayesian Statistics written by Jean-Michel Marin and has been published by Springer Science & Business Media this book supported file pdf, txt, epub, kindle and other format this book has been release on 2007-05-26 with Mathematics categories.
This Bayesian modeling book is intended for practitioners and applied statisticians looking for a self-contained entry to computational Bayesian statistics. Focusing on standard statistical models and backed up by discussed real datasets available from the book website, it provides an operational methodology for conducting Bayesian inference, rather than focusing on its theoretical justifications. Special attention is paid to the derivation of prior distributions in each case and specific reference solutions are given for each of the models. Similarly, computational details are worked out to lead the reader towards an effective programming of the methods given in the book.
Bayesian Essentials With R
DOWNLOAD
Author : Jean-Michel Marin
language : en
Publisher: Springer Science & Business Media
Release Date : 2013-10-28
Bayesian Essentials With R written by Jean-Michel Marin and has been published by Springer Science & Business Media this book supported file pdf, txt, epub, kindle and other format this book has been release on 2013-10-28 with Computers categories.
This Bayesian modeling book provides a self-contained entry to computational Bayesian statistics. Focusing on the most standard statistical models and backed up by real datasets and an all-inclusive R (CRAN) package called bayess, the book provides an operational methodology for conducting Bayesian inference, rather than focusing on its theoretical and philosophical justifications. Readers are empowered to participate in the real-life data analysis situations depicted here from the beginning. Special attention is paid to the derivation of prior distributions in each case and specific reference solutions are given for each of the models. Similarly, computational details are worked out to lead the reader towards an effective programming of the methods given in the book. In particular, all R codes are discussed with enough detail to make them readily understandable and expandable. Bayesian Essentials with R can be used as a textbook at both undergraduate and graduate levels. It is particularly useful with students in professional degree programs and scientists to analyze data the Bayesian way. The text will also enhance introductory courses on Bayesian statistics. Prerequisites for the book are an undergraduate background in probability and statistics, if not in Bayesian statistics.
Bayesian Data Analysis Third Edition
DOWNLOAD
Author : Andrew Gelman
language : en
Publisher: CRC Press
Release Date : 2013-11-01
Bayesian Data Analysis Third Edition written by Andrew Gelman and has been published by CRC Press this book supported file pdf, txt, epub, kindle and other format this book has been release on 2013-11-01 with Mathematics categories.
Now in its third edition, this classic book is widely considered the leading text on Bayesian methods, lauded for its accessible, practical approach to analyzing data and solving research problems. Bayesian Data Analysis, Third Edition continues to take an applied approach to analysis using up-to-date Bayesian methods. The authors—all leaders in the statistics community—introduce basic concepts from a data-analytic perspective before presenting advanced methods. Throughout the text, numerous worked examples drawn from real applications and research emphasize the use of Bayesian inference in practice. New to the Third Edition Four new chapters on nonparametric modeling Coverage of weakly informative priors and boundary-avoiding priors Updated discussion of cross-validation and predictive information criteria Improved convergence monitoring and effective sample size calculations for iterative simulation Presentations of Hamiltonian Monte Carlo, variational Bayes, and expectation propagation New and revised software code The book can be used in three different ways. For undergraduate students, it introduces Bayesian inference starting from first principles. For graduate students, the text presents effective current approaches to Bayesian modeling and computation in statistics and related fields. For researchers, it provides an assortment of Bayesian methods in applied statistics. Additional materials, including data sets used in the examples, solutions to selected exercises, and software instructions, are available on the book’s web page.
Bayesian Modeling And Computation In Python
DOWNLOAD
Author : Osvaldo A. Martin
language : en
Publisher: CRC Press
Release Date : 2021-12-28
Bayesian Modeling And Computation In Python written by Osvaldo A. Martin and has been published by CRC Press this book supported file pdf, txt, epub, kindle and other format this book has been release on 2021-12-28 with Computers categories.
Bayesian Modeling and Computation in Python aims to help beginner Bayesian practitioners to become intermediate modelers. It uses a hands on approach with PyMC3, Tensorflow Probability, ArviZ and other libraries focusing on the practice of applied statistics with references to the underlying mathematical theory. The book starts with a refresher of the Bayesian Inference concepts. The second chapter introduces modern methods for Exploratory Analysis of Bayesian Models. With an understanding of these two fundamentals the subsequent chapters talk through various models including linear regressions, splines, time series, Bayesian additive regression trees. The final chapters include Approximate Bayesian Computation, end to end case studies showing how to apply Bayesian modelling in different settings, and a chapter about the internals of probabilistic programming languages. Finally the last chapter serves as a reference for the rest of the book by getting closer into mathematical aspects or by extending the discussion of certain topics. This book is written by contributors of PyMC3, ArviZ, Bambi, and Tensorflow Probability among other libraries.
Statistical Rethinking
DOWNLOAD
Author : Richard McElreath
language : en
Publisher: CRC Press
Release Date : 2018-01-03
Statistical Rethinking written by Richard McElreath and has been published by CRC Press this book supported file pdf, txt, epub, kindle and other format this book has been release on 2018-01-03 with Mathematics categories.
Statistical Rethinking: A Bayesian Course with Examples in R and Stan builds readers’ knowledge of and confidence in statistical modeling. Reflecting the need for even minor programming in today’s model-based statistics, the book pushes readers to perform step-by-step calculations that are usually automated. This unique computational approach ensures that readers understand enough of the details to make reasonable choices and interpretations in their own modeling work. The text presents generalized linear multilevel models from a Bayesian perspective, relying on a simple logical interpretation of Bayesian probability and maximum entropy. It covers from the basics of regression to multilevel models. The author also discusses measurement error, missing data, and Gaussian process models for spatial and network autocorrelation. By using complete R code examples throughout, this book provides a practical foundation for performing statistical inference. Designed for both PhD students and seasoned professionals in the natural and social sciences, it prepares them for more advanced or specialized statistical modeling. Web Resource The book is accompanied by an R package (rethinking) that is available on the author’s website and GitHub. The two core functions (map and map2stan) of this package allow a variety of statistical models to be constructed from standard model formulas.
Multiscale Modeling
DOWNLOAD
Author : Marco A.R. Ferreira
language : en
Publisher: Springer Science & Business Media
Release Date : 2007-07-27
Multiscale Modeling written by Marco A.R. Ferreira and has been published by Springer Science & Business Media this book supported file pdf, txt, epub, kindle and other format this book has been release on 2007-07-27 with Business & Economics categories.
This highly useful book contains methodology for the analysis of data that arise from multiscale processes. It brings together a number of recent developments and makes them accessible to a wider audience. Taking a Bayesian approach allows for full accounting of uncertainty, and also addresses the delicate issue of uncertainty at multiple scales. These methods can handle different amounts of prior knowledge at different scales, as often occurs in practice.
Practical Bayesian Inference
DOWNLOAD
Author : Coryn A. L. Bailer-Jones
language : en
Publisher: Cambridge University Press
Release Date : 2017-04-27
Practical Bayesian Inference written by Coryn A. L. Bailer-Jones and has been published by Cambridge University Press this book supported file pdf, txt, epub, kindle and other format this book has been release on 2017-04-27 with Mathematics categories.
This book introduces the major concepts of probability and statistics, along with the necessary computational tools, for undergraduates and graduate students.
Bayesian Methods For Hackers
DOWNLOAD
Author : Cameron Davidson-Pilon
language : en
Publisher: Addison-Wesley Professional
Release Date : 2015-09-30
Bayesian Methods For Hackers written by Cameron Davidson-Pilon and has been published by Addison-Wesley Professional this book supported file pdf, txt, epub, kindle and other format this book has been release on 2015-09-30 with Computers categories.
Master Bayesian Inference through Practical Examples and Computation–Without Advanced Mathematical Analysis Bayesian methods of inference are deeply natural and extremely powerful. However, most discussions of Bayesian inference rely on intensely complex mathematical analyses and artificial examples, making it inaccessible to anyone without a strong mathematical background. Now, though, Cameron Davidson-Pilon introduces Bayesian inference from a computational perspective, bridging theory to practice–freeing you to get results using computing power. Bayesian Methods for Hackers illuminates Bayesian inference through probabilistic programming with the powerful PyMC language and the closely related Python tools NumPy, SciPy, and Matplotlib. Using this approach, you can reach effective solutions in small increments, without extensive mathematical intervention. Davidson-Pilon begins by introducing the concepts underlying Bayesian inference, comparing it with other techniques and guiding you through building and training your first Bayesian model. Next, he introduces PyMC through a series of detailed examples and intuitive explanations that have been refined after extensive user feedback. You’ll learn how to use the Markov Chain Monte Carlo algorithm, choose appropriate sample sizes and priors, work with loss functions, and apply Bayesian inference in domains ranging from finance to marketing. Once you’ve mastered these techniques, you’ll constantly turn to this guide for the working PyMC code you need to jumpstart future projects. Coverage includes • Learning the Bayesian “state of mind” and its practical implications • Understanding how computers perform Bayesian inference • Using the PyMC Python library to program Bayesian analyses • Building and debugging models with PyMC • Testing your model’s “goodness of fit” • Opening the “black box” of the Markov Chain Monte Carlo algorithm to see how and why it works • Leveraging the power of the “Law of Large Numbers” • Mastering key concepts, such as clustering, convergence, autocorrelation, and thinning • Using loss functions to measure an estimate’s weaknesses based on your goals and desired outcomes • Selecting appropriate priors and understanding how their influence changes with dataset size • Overcoming the “exploration versus exploitation” dilemma: deciding when “pretty good” is good enough • Using Bayesian inference to improve A/B testing • Solving data science problems when only small amounts of data are available Cameron Davidson-Pilon has worked in many areas of applied mathematics, from the evolutionary dynamics of genes and diseases to stochastic modeling of financial prices. His contributions to the open source community include lifelines, an implementation of survival analysis in Python. Educated at the University of Waterloo and at the Independent University of Moscow, he currently works with the online commerce leader Shopify.