Causal Artificial Intelligence
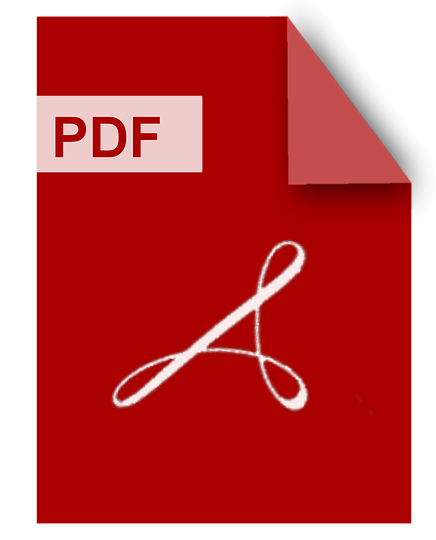
DOWNLOAD eBooks
Download Causal Artificial Intelligence PDF/ePub or read online books in Mobi eBooks. Click Download or Read Online button to get Causal Artificial Intelligence book now. This website allows unlimited access to, at the time of writing, more than 1.5 million titles, including hundreds of thousands of titles in various foreign languages. If the content not found or just blank you must refresh this page
Causal Artificial Intelligence
DOWNLOAD eBooks
Author : Judith S. Hurwitz
language : en
Publisher: John Wiley & Sons
Release Date : 2023-08-23
Causal Artificial Intelligence written by Judith S. Hurwitz and has been published by John Wiley & Sons this book supported file pdf, txt, epub, kindle and other format this book has been release on 2023-08-23 with Computers categories.
Discover the next major revolution in data science and AI and how it applies to your organization In Causal Artificial Intelligence: The Next Step in Effective, Efficient, and Practical AI, a team of dedicated tech executives delivers a business-focused approach based on a deep and engaging exploration of the models and data used in causal AI. The book’s discussions include both accessible and understandable technical detail and business context and concepts that frame causal AI in familiar business settings. Useful for both data scientists and business-side professionals, the book offers: Clear and compelling descriptions of the concept of causality and how it can benefit your organization Detailed use cases and examples that vividly demonstrate the value of causality for solving business problems Useful strategies for deciding when to use correlation-based approaches and when to use causal inference An enlightening and easy-to-understand treatment of an essential business topic, Causal Artificial Intelligence is a must-read for data scientists, subject matter experts, and business leaders seeking to familiarize themselves with a rapidly growing area of AI application and research.
Artificial Intelligence And Causal Inference
DOWNLOAD eBooks
Author : Momiao Xiong
language : en
Publisher:
Release Date : 2022
Artificial Intelligence And Causal Inference written by Momiao Xiong and has been published by this book supported file pdf, txt, epub, kindle and other format this book has been release on 2022 with Artificial intelligence categories.
"Artificial Intelligence and Causal Inference address the recent development of relationships between artificial intelligence (AI) and causal inference. Despite significant progress in AI, a great challenge in AI development we are still facing is to understand mechanism underlying intelligence, including reasoning, planning and imagination. Understanding, transfer and generalization are major principles that give rise intelligence. One of a key component for understanding is causal inference. Causal inference includes intervention, domain shift learning, temporal structure and counterfactual thinking as major concepts to understand causation and reasoning. Unfortunately, these essential components of the causality are often overlooked by machine learning, which leads to some failure of the deep learning. AI and causal inference involve (1) using AI techniques as major tools for causal analysis and (2) applying the causal concepts and causal analysis methods to solving AI problems. The purpose of this book is to fill the gap between the AI and modern causal analysis for further facilitating the AI revolution. This book is ideal for graduate students and researchers in AI, data science, causal inference, statistics, genomics, bioinformatics and precision medicine"--
Machine Learning For Causal Inference
DOWNLOAD eBooks
Author : Sheng Li
language : en
Publisher: Springer Nature
Release Date : 2023-11-25
Machine Learning For Causal Inference written by Sheng Li and has been published by Springer Nature this book supported file pdf, txt, epub, kindle and other format this book has been release on 2023-11-25 with Technology & Engineering categories.
This book provides a deep understanding of the relationship between machine learning and causal inference. It covers a broad range of topics, starting with the preliminary foundations of causal inference, which include basic definitions, illustrative examples, and assumptions. It then delves into the different types of classical causal inference methods, such as matching, weighting, tree-based models, and more. Additionally, the book explores how machine learning can be used for causal effect estimation based on representation learning and graph learning. The contribution of causal inference in creating trustworthy machine learning systems to accomplish diversity, non-discrimination and fairness, transparency and explainability, generalization and robustness, and more is also discussed. The book also provides practical applications of causal inference in various domains such as natural language processing, recommender systems, computer vision, time series forecasting, and continual learning. Each chapter of the book is written by leading researchers in their respective fields. Machine Learning for Causal Inference explores the challenges associated with the relationship between machine learning and causal inference, such as biased estimates of causal effects, untrustworthy models, and complicated applications in other artificial intelligence domains. However, it also presents potential solutions to these issues. The book is a valuable resource for researchers, teachers, practitioners, and students interested in these fields. It provides insights into how combining machine learning and causal inference can improve the system's capability to accomplish causal artificial intelligence based on data. The book showcases promising research directions and emphasizes the importance of understanding the causal relationship to construct different machine-learning models from data.
Causality Correlation And Artificial Intelligence For Rational Decision Making
DOWNLOAD eBooks
Author : Tshilidzi Marwala
language : en
Publisher: World Scientific
Release Date : 2015-01-02
Causality Correlation And Artificial Intelligence For Rational Decision Making written by Tshilidzi Marwala and has been published by World Scientific this book supported file pdf, txt, epub, kindle and other format this book has been release on 2015-01-02 with Computers categories.
Causality has been a subject of study for a long time. Often causality is confused with correlation. Human intuition has evolved such that it has learned to identify causality through correlation. In this book, four main themes are considered and these are causality, correlation, artificial intelligence and decision making. A correlation machine is defined and built using multi-layer perceptron network, principal component analysis, Gaussian Mixture models, genetic algorithms, expectation maximization technique, simulated annealing and particle swarm optimization. Furthermore, a causal machine is defined and built using multi-layer perceptron, radial basis function, Bayesian statistics and Hybrid Monte Carlo methods. Both these machines are used to build a Granger non-linear causality model. In addition, the Neyman–Rubin, Pearl and Granger causal models are studied and are unified. The automatic relevance determination is also applied to extend Granger causality framework to the non-linear domain. The concept of rational decision making is studied, and the theory of flexibly-bounded rationality is used to extend the theory of bounded rationality within the principle of the indivisibility of rationality. The theory of the marginalization of irrationality for decision making is also introduced to deal with satisficing within irrational conditions. The methods proposed are applied in biomedical engineering, condition monitoring and for modelling interstate conflict. Contents:Introduction to Artificial Intelligence based Decision MakingWhat is a Correlation Machine?What is a Causal Machine?Correlation Machines Using Optimization MethodsNeural Networks for Modeling Granger CausalityRubin, Pearl and Granger Causality Models: A Unified ViewCausal, Correlation and Automatic Relevance Determination Machines for Granger CausalityFlexibly-bounded RationalityMarginalization of Irrationality in Decision MakingConclusions and Further Work Readership: Graduate students, researchers and professionals in the field of artificial intelligence. Key Features:It proposes fresh definition of causality and proposes two new theories i.e. flexibly bounded rationality and marginalization of irrationality theory for decision makingIt also applies these techniques to a diverse areas in engineering, political science and biomedical engineeringKeywords:Causality;Correlation;Artificial Intelligence;Rational Decision Making
Causal Models And Intelligent Data Management
DOWNLOAD eBooks
Author : Alex Gammerman
language : en
Publisher: Springer Science & Business Media
Release Date : 2012-12-06
Causal Models And Intelligent Data Management written by Alex Gammerman and has been published by Springer Science & Business Media this book supported file pdf, txt, epub, kindle and other format this book has been release on 2012-12-06 with Computers categories.
The need to electronically store, manipulate and analyze large-scale, high-dimensional data sets requires new computational methods. This book presents new intelligent data management methods and tools, including new results from the field of inference. Leading experts also map out future directions of intelligent data analysis. This book will be a valuable reference for researchers exploring the interdisciplinary area between statistics and computer science as well as for professionals applying advanced data analysis methods in industry.
Probabilistic And Causal Inference
DOWNLOAD eBooks
Author : Hector Geffner
language : en
Publisher: Morgan & Claypool
Release Date : 2022-03-10
Probabilistic And Causal Inference written by Hector Geffner and has been published by Morgan & Claypool this book supported file pdf, txt, epub, kindle and other format this book has been release on 2022-03-10 with Computers categories.
Professor Judea Pearl won the 2011 Turing Award “for fundamental contributions to artificial intelligence through the development of a calculus for probabilistic and causal reasoning.” This book contains the original articles that led to the award, as well as other seminal works, divided into four parts: heuristic search, probabilistic reasoning, causality, first period (1988–2001), and causality, recent period (2002–2020). Each of these parts starts with an introduction written by Judea Pearl. The volume also contains original, contributed articles by leading researchers that analyze, extend, or assess the influence of Pearl’s work in different fields: from AI, Machine Learning, and Statistics to Cognitive Science, Philosophy, and the Social Sciences. The first part of the volume includes a biography, a transcript of his Turing Award Lecture, two interviews, and a selected bibliography annotated by him.
Elements Of Causal Inference
DOWNLOAD eBooks
Author : Jonas Peters
language : en
Publisher: MIT Press
Release Date : 2017-12-29
Elements Of Causal Inference written by Jonas Peters and has been published by MIT Press this book supported file pdf, txt, epub, kindle and other format this book has been release on 2017-12-29 with Computers categories.
A concise and self-contained introduction to causal inference, increasingly important in data science and machine learning. The mathematization of causality is a relatively recent development, and has become increasingly important in data science and machine learning. This book offers a self-contained and concise introduction to causal models and how to learn them from data. After explaining the need for causal models and discussing some of the principles underlying causal inference, the book teaches readers how to use causal models: how to compute intervention distributions, how to infer causal models from observational and interventional data, and how causal ideas could be exploited for classical machine learning problems. All of these topics are discussed first in terms of two variables and then in the more general multivariate case. The bivariate case turns out to be a particularly hard problem for causal learning because there are no conditional independences as used by classical methods for solving multivariate cases. The authors consider analyzing statistical asymmetries between cause and effect to be highly instructive, and they report on their decade of intensive research into this problem. The book is accessible to readers with a background in machine learning or statistics, and can be used in graduate courses or as a reference for researchers. The text includes code snippets that can be copied and pasted, exercises, and an appendix with a summary of the most important technical concepts.
Discovering Causal Structure
DOWNLOAD eBooks
Author : Clark Glymour
language : en
Publisher: Academic Press
Release Date : 2014-05-10
Discovering Causal Structure written by Clark Glymour and has been published by Academic Press this book supported file pdf, txt, epub, kindle and other format this book has been release on 2014-05-10 with Social Science categories.
Discovering Causal Structure: Artificial Intelligence, Philosophy of Science, and Statistical Modeling provides information pertinent to the fundamental aspects of a computer program called TETRAD. This book discusses the version of the TETRAD program, which is designed to assist in the search for causal explanations of statistical data. or alternative models. This text then examines the notion of applying artificial intelligence methods to problems of statistical model specification. Other chapters consider how the TETRAD program can help to find god alternative models where they exist, and how it can help detect the existence of important neglected variables. This book discusses as well the procedures for specifying a model or models to account for non-experimental or quasi-experimental data. The final chapter presents a description of the format of input files and a description of each command. This book is a valuable resource for social scientists and researchers.
Causality
DOWNLOAD eBooks
Author : Judea Pearl
language : en
Publisher: Cambridge University Press
Release Date : 2009-09-14
Causality written by Judea Pearl and has been published by Cambridge University Press this book supported file pdf, txt, epub, kindle and other format this book has been release on 2009-09-14 with Computers categories.
Causality offers the first comprehensive coverage of causal analysis in many sciences, including recent advances using graphical methods. Pearl presents a unified account of the probabilistic, manipulative, counterfactual and structural approaches to causation, and devises simple mathematical tools for analyzing the relationships between causal connections, statistical associations, actions and observations. The book will open the way for including causal analysis in the standard curriculum of statistics, artificial intelligence ...
Cause Effect Pairs In Machine Learning
DOWNLOAD eBooks
Author : Isabelle Guyon
language : en
Publisher: Springer Nature
Release Date : 2019-10-22
Cause Effect Pairs In Machine Learning written by Isabelle Guyon and has been published by Springer Nature this book supported file pdf, txt, epub, kindle and other format this book has been release on 2019-10-22 with Computers categories.
This book presents ground-breaking advances in the domain of causal structure learning. The problem of distinguishing cause from effect (“Does altitude cause a change in atmospheric pressure, or vice versa?”) is here cast as a binary classification problem, to be tackled by machine learning algorithms. Based on the results of the ChaLearn Cause-Effect Pairs Challenge, this book reveals that the joint distribution of two variables can be scrutinized by machine learning algorithms to reveal the possible existence of a “causal mechanism”, in the sense that the values of one variable may have been generated from the values of the other. This book provides both tutorial material on the state-of-the-art on cause-effect pairs and exposes the reader to more advanced material, with a collection of selected papers. Supplemental material includes videos, slides, and code which can be found on the workshop website. Discovering causal relationships from observational data will become increasingly important in data science with the increasing amount of available data, as a means of detecting potential triggers in epidemiology, social sciences, economy, biology, medicine, and other sciences.