Genetic Algorithms For Machine Learning
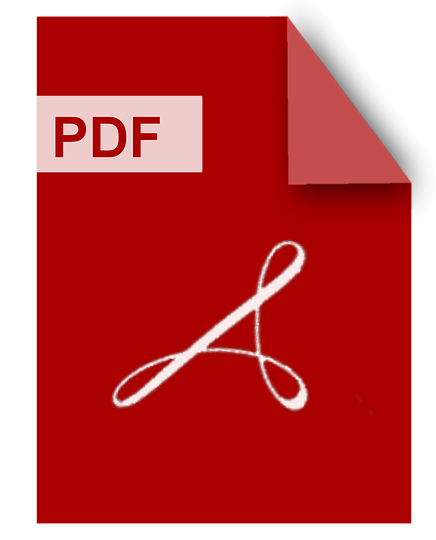
DOWNLOAD
Download Genetic Algorithms For Machine Learning PDF/ePub or read online books in Mobi eBooks. Click Download or Read Online button to get Genetic Algorithms For Machine Learning book now. This website allows unlimited access to, at the time of writing, more than 1.5 million titles, including hundreds of thousands of titles in various foreign languages. If the content not found or just blank you must refresh this page
Hands On Genetic Algorithms With Python
DOWNLOAD
Author : Eyal Wirsansky
language : en
Publisher: Packt Publishing Ltd
Release Date : 2020-01-31
Hands On Genetic Algorithms With Python written by Eyal Wirsansky and has been published by Packt Publishing Ltd this book supported file pdf, txt, epub, kindle and other format this book has been release on 2020-01-31 with Computers categories.
Explore the ever-growing world of genetic algorithms to solve search, optimization, and AI-related tasks, and improve machine learning models using Python libraries such as DEAP, scikit-learn, and NumPy Key Features Explore the ins and outs of genetic algorithms with this fast-paced guide Implement tasks such as feature selection, search optimization, and cluster analysis using Python Solve combinatorial problems, optimize functions, and enhance the performance of artificial intelligence applications Book DescriptionGenetic algorithms are a family of search, optimization, and learning algorithms inspired by the principles of natural evolution. By imitating the evolutionary process, genetic algorithms can overcome hurdles encountered in traditional search algorithms and provide high-quality solutions for a variety of problems. This book will help you get to grips with a powerful yet simple approach to applying genetic algorithms to a wide range of tasks using Python, covering the latest developments in artificial intelligence. After introducing you to genetic algorithms and their principles of operation, you'll understand how they differ from traditional algorithms and what types of problems they can solve. You'll then discover how they can be applied to search and optimization problems, such as planning, scheduling, gaming, and analytics. As you advance, you'll also learn how to use genetic algorithms to improve your machine learning and deep learning models, solve reinforcement learning tasks, and perform image reconstruction. Finally, you'll cover several related technologies that can open up new possibilities for future applications. By the end of this book, you'll have hands-on experience of applying genetic algorithms in artificial intelligence as well as in numerous other domains.What you will learn Understand how to use state-of-the-art Python tools to create genetic algorithm-based applications Use genetic algorithms to optimize functions and solve planning and scheduling problems Enhance the performance of machine learning models and optimize deep learning network architecture Apply genetic algorithms to reinforcement learning tasks using OpenAI Gym Explore how images can be reconstructed using a set of semi-transparent shapes Discover other bio-inspired techniques, such as genetic programming and particle swarm optimization Who this book is for This book is for software developers, data scientists, and AI enthusiasts who want to use genetic algorithms to carry out intelligent tasks in their applications. Working knowledge of Python and basic knowledge of mathematics and computer science will help you get the most out of this book.
An Introduction To Genetic Algorithms
DOWNLOAD
Author : Melanie Mitchell
language : en
Publisher: MIT Press
Release Date : 1998-03-02
An Introduction To Genetic Algorithms written by Melanie Mitchell and has been published by MIT Press this book supported file pdf, txt, epub, kindle and other format this book has been release on 1998-03-02 with Computers categories.
Genetic algorithms have been used in science and engineering as adaptive algorithms for solving practical problems and as computational models of natural evolutionary systems. This brief, accessible introduction describes some of the most interesting research in the field and also enables readers to implement and experiment with genetic algorithms on their own. It focuses in depth on a small set of important and interesting topics—particularly in machine learning, scientific modeling, and artificial life—and reviews a broad span of research, including the work of Mitchell and her colleagues. The descriptions of applications and modeling projects stretch beyond the strict boundaries of computer science to include dynamical systems theory, game theory, molecular biology, ecology, evolutionary biology, and population genetics, underscoring the exciting "general purpose" nature of genetic algorithms as search methods that can be employed across disciplines. An Introduction to Genetic Algorithms is accessible to students and researchers in any scientific discipline. It includes many thought and computer exercises that build on and reinforce the reader's understanding of the text. The first chapter introduces genetic algorithms and their terminology and describes two provocative applications in detail. The second and third chapters look at the use of genetic algorithms in machine learning (computer programs, data analysis and prediction, neural networks) and in scientific models (interactions among learning, evolution, and culture; sexual selection; ecosystems; evolutionary activity). Several approaches to the theory of genetic algorithms are discussed in depth in the fourth chapter. The fifth chapter takes up implementation, and the last chapter poses some currently unanswered questions and surveys prospects for the future of evolutionary computation.
Learning Genetic Algorithms With Python
DOWNLOAD
Author : Ivan Gridin
language : en
Publisher: BPB Publications
Release Date : 2021-02-13
Learning Genetic Algorithms With Python written by Ivan Gridin and has been published by BPB Publications this book supported file pdf, txt, epub, kindle and other format this book has been release on 2021-02-13 with Computers categories.
Refuel your AI Models and ML applications with High-Quality Optimization and Search Solutions DESCRIPTION Genetic algorithms are one of the most straightforward and powerful techniques used in machine learning. This book ÔLearning Genetic Algorithms with PythonÕ guides the reader right from the basics of genetic algorithms to its real practical implementation in production environments.Ê Each of the chapters gives the reader an intuitive understanding of each concept. You will learn how to build a genetic algorithm from scratch and implement it in real-life problems. Covered with practical illustrated examples, you will learn to design and choose the best model architecture for the particular tasks. Cutting edge examples like radar and football manager problem statements, you will learn to solve high-dimensional big data challenges with ways of optimizing genetic algorithms. KEY FEATURESÊÊ _ Complete coverage on practical implementation of genetic algorithms. _ Intuitive explanations and visualizations supply theoretical concepts. _ Added examples and use-cases on the performance of genetic algorithms. _ Use of Python libraries and a niche coverage on the performance optimization of genetic algorithms. WHAT YOU WILL LEARNÊ _ Understand the mechanism of genetic algorithms using popular python libraries. _ Learn the principles and architecture of genetic algorithms. _ Apply and Solve planning, scheduling and analytics problems in Enterprise applications. _Ê Expert learning on prime concepts like Selection, Mutation and Crossover. WHO THIS BOOK IS FORÊÊ The book is for Data Science team, Analytics team, AI Engineers, ML Professionals who want to integrate genetic algorithms to refuel their ML and AI applications. No special expertise about machine learning is required although a basic knowledge of Python is expected. TABLE OF CONTENTS 1. Introduction 2. Genetic Algorithm Flow 3. Selection 4. Crossover 5. Mutation 6. Effectiveness 7. Parameter Tuning 8. Black-box Function 9. Combinatorial Optimization: Binary Gene Encoding 10. Combinatorial Optimization: Ordered Gene Encoding 11. Other Common Problems 12. Adaptive Genetic Algorithm 13. Improving Performance
Classification And Learning Using Genetic Algorithms
DOWNLOAD
Author : Sanghamitra Bandyopadhyay
language : en
Publisher: Springer Science & Business Media
Release Date : 2007-05-17
Classification And Learning Using Genetic Algorithms written by Sanghamitra Bandyopadhyay and has been published by Springer Science & Business Media this book supported file pdf, txt, epub, kindle and other format this book has been release on 2007-05-17 with Computers categories.
This book provides a unified framework that describes how genetic learning can be used to design pattern recognition and learning systems. It examines how a search technique, the genetic algorithm, can be used for pattern classification mainly through approximating decision boundaries. Coverage also demonstrates the effectiveness of the genetic classifiers vis-à-vis several widely used classifiers, including neural networks.
Genetic Algorithms For Machine Learning
DOWNLOAD
Author : John J. Grefenstette
language : en
Publisher: Springer Science & Business Media
Release Date : 1993-11-30
Genetic Algorithms For Machine Learning written by John J. Grefenstette and has been published by Springer Science & Business Media this book supported file pdf, txt, epub, kindle and other format this book has been release on 1993-11-30 with Computers categories.
The articles presented here were selected from preliminary versions presented at the International Conference on Genetic Algorithms in June 1991, as well as at a special Workshop on Genetic Algorithms for Machine Learning at the same Conference. Genetic algorithms are general-purpose search algorithms that use principles inspired by natural population genetics to evolve solutions to problems. The basic idea is to maintain a population of knowledge structure that represent candidate solutions to the problem of interest. The population evolves over time through a process of competition (i.e. survival of the fittest) and controlled variation (i.e. recombination and mutation). Genetic Algorithms for Machine Learning contains articles on three topics that have not been the focus of many previous articles on GAs, namely concept learning from examples, reinforcement learning for control, and theoretical analysis of GAs. It is hoped that this sample will serve to broaden the acquaintance of the general machine learning community with the major areas of work on GAs. The articles in this book address a number of central issues in applying GAs to machine learning problems. For example, the choice of appropriate representation and the corresponding set of genetic learning operators is an important set of decisions facing a user of a genetic algorithm. The study of genetic algorithms is proceeding at a robust pace. If experimental progress and theoretical understanding continue to evolve as expected, genetic algorithms will continue to provide a distinctive approach to machine learning. Genetic Algorithms for Machine Learning is an edited volume of original research made up of invited contributions by leading researchers.
Evolutionary Machine Learning Techniques
DOWNLOAD
Author : Seyedali Mirjalili
language : en
Publisher: Springer Nature
Release Date : 2019-11-11
Evolutionary Machine Learning Techniques written by Seyedali Mirjalili and has been published by Springer Nature this book supported file pdf, txt, epub, kindle and other format this book has been release on 2019-11-11 with Technology & Engineering categories.
This book provides an in-depth analysis of the current evolutionary machine learning techniques. Discussing the most highly regarded methods for classification, clustering, regression, and prediction, it includes techniques such as support vector machines, extreme learning machines, evolutionary feature selection, artificial neural networks including feed-forward neural networks, multi-layer perceptron, probabilistic neural networks, self-optimizing neural networks, radial basis function networks, recurrent neural networks, spiking neural networks, neuro-fuzzy networks, modular neural networks, physical neural networks, and deep neural networks. The book provides essential definitions, literature reviews, and the training algorithms for machine learning using classical and modern nature-inspired techniques. It also investigates the pros and cons of classical training algorithms. It features a range of proven and recent nature-inspired algorithms used to train different types of artificial neural networks, including genetic algorithm, ant colony optimization, particle swarm optimization, grey wolf optimizer, whale optimization algorithm, ant lion optimizer, moth flame algorithm, dragonfly algorithm, salp swarm algorithm, multi-verse optimizer, and sine cosine algorithm. The book also covers applications of the improved artificial neural networks to solve classification, clustering, prediction and regression problems in diverse fields.
Genetic Algorithms Data Structures Evolution Programs
DOWNLOAD
Author : Zbigniew Michalewicz
language : en
Publisher: Springer Science & Business Media
Release Date : 2013-03-09
Genetic Algorithms Data Structures Evolution Programs written by Zbigniew Michalewicz and has been published by Springer Science & Business Media this book supported file pdf, txt, epub, kindle and other format this book has been release on 2013-03-09 with Computers categories.
Genetic algorithms are founded upon the principle of evolution, i.e., survival of the fittest. Hence evolution programming techniques, based on genetic algorithms, are applicable to many hard optimization problems, such as optimization of functions with linear and nonlinear constraints, the traveling salesman problem, and problems of scheduling, partitioning, and control. The importance of these techniques has been growing in the last decade, since evolution programs are parallel in nature, and parallelism is one of the most promising directions in computer science. The book is self-contained and the only prerequisite is basic undergraduate mathematics. It is aimed at researchers, practitioners, and graduate students in computer science and artificial intelligence, operations research, and engineering. This second edition includes several new sections and many references to recent developments. A simple example of genetic code and an index are also added. Writing an evolution program for a given problem should be an enjoyable experience - this book may serve as a guide to this task.
Introduction To Genetic Algorithms
DOWNLOAD
Author : S.N. Sivanandam
language : en
Publisher: Springer Science & Business Media
Release Date : 2007-10-24
Introduction To Genetic Algorithms written by S.N. Sivanandam and has been published by Springer Science & Business Media this book supported file pdf, txt, epub, kindle and other format this book has been release on 2007-10-24 with Technology & Engineering categories.
Theoriginofevolutionaryalgorithmswasanattempttomimicsomeoftheprocesses taking place in natural evolution. Although the details of biological evolution are not completely understood (even nowadays), there exist some points supported by strong experimental evidence: • Evolution is a process operating over chromosomes rather than over organisms. The former are organic tools encoding the structure of a living being, i.e., a cr- ture is “built” decoding a set of chromosomes. • Natural selection is the mechanism that relates chromosomes with the ef ciency of the entity they represent, thus allowing that ef cient organism which is we- adapted to the environment to reproduce more often than those which are not. • The evolutionary process takes place during the reproduction stage. There exists a large number of reproductive mechanisms in Nature. Most common ones are mutation (that causes the chromosomes of offspring to be different to those of the parents) and recombination (that combines the chromosomes of the parents to produce the offspring). Based upon the features above, the three mentioned models of evolutionary c- puting were independently (and almost simultaneously) developed.
Genetic Algorithms
DOWNLOAD
Author : David E. Goldberg
language : en
Publisher: Pearson Education India
Release Date : 2013-02
Genetic Algorithms written by David E. Goldberg and has been published by Pearson Education India this book supported file pdf, txt, epub, kindle and other format this book has been release on 2013-02 with Computers categories.
This book, suitable for both course work and self-study, brings together for the first time, in an informal, tutorial fashion, the computer techniques, mathematical tools, and research results that will enable both students and practitioners to apply genetic algorithms to problems in many fields: programmers, scientists, engineers, mathematicians, statisticians and management scientists will all find interesting possibilities here. Major concepts are illustrated with running examples, and major algorithms are illustrated by Pascal computer programs. Chapter concludes with exercises and computer assignments. No prior knowledge of Gas or genetics is assumed.
Artificial Intelligence Concepts Methodologies Tools And Applications
DOWNLOAD
Author : Management Association, Information Resources
language : en
Publisher: IGI Global
Release Date : 2016-12-12
Artificial Intelligence Concepts Methodologies Tools And Applications written by Management Association, Information Resources and has been published by IGI Global this book supported file pdf, txt, epub, kindle and other format this book has been release on 2016-12-12 with Computers categories.
Ongoing advancements in modern technology have led to significant developments in artificial intelligence. With the numerous applications available, it becomes imperative to conduct research and make further progress in this field. Artificial Intelligence: Concepts, Methodologies, Tools, and Applications provides a comprehensive overview of the latest breakthroughs and recent progress in artificial intelligence. Highlighting relevant technologies, uses, and techniques across various industries and settings, this publication is a pivotal reference source for researchers, professionals, academics, upper-level students, and practitioners interested in emerging perspectives in the field of artificial intelligence.