High Dimensional Covariance Matrix Estimation Shrinkage Toward A Diagonal Target
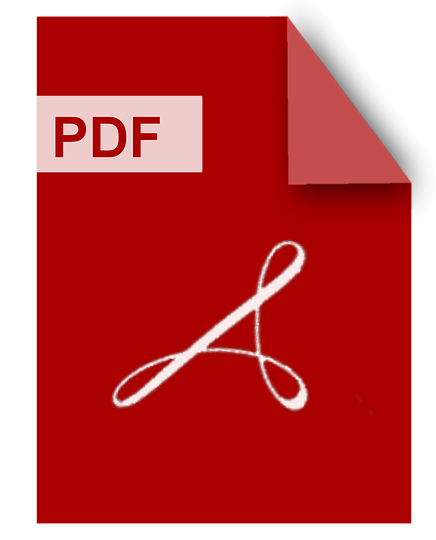
DOWNLOAD
Download High Dimensional Covariance Matrix Estimation Shrinkage Toward A Diagonal Target PDF/ePub or read online books in Mobi eBooks. Click Download or Read Online button to get High Dimensional Covariance Matrix Estimation Shrinkage Toward A Diagonal Target book now. This website allows unlimited access to, at the time of writing, more than 1.5 million titles, including hundreds of thousands of titles in various foreign languages. If the content not found or just blank you must refresh this page
High Dimensional Covariance Matrix Estimation Shrinkage Toward A Diagonal Target
DOWNLOAD
Author : Mr. Sakai Ando
language : en
Publisher: International Monetary Fund
Release Date : 2023-12-08
High Dimensional Covariance Matrix Estimation Shrinkage Toward A Diagonal Target written by Mr. Sakai Ando and has been published by International Monetary Fund this book supported file pdf, txt, epub, kindle and other format this book has been release on 2023-12-08 with Business & Economics categories.
This paper proposes a novel shrinkage estimator for high-dimensional covariance matrices by extending the Oracle Approximating Shrinkage (OAS) of Chen et al. (2009) to target the diagonal elements of the sample covariance matrix. We derive the closed-form solution of the shrinkage parameter and show by simulation that, when the diagonal elements of the true covariance matrix exhibit substantial variation, our method reduces the Mean Squared Error, compared with the OAS that targets an average variance. The improvement is larger when the true covariance matrix is sparser. Our method also reduces the Mean Squared Error for the inverse of the covariance matrix.
High Dimensional Covariance Estimation
DOWNLOAD
Author : Mohsen Pourahmadi
language : en
Publisher: John Wiley & Sons
Release Date : 2013-05-28
High Dimensional Covariance Estimation written by Mohsen Pourahmadi and has been published by John Wiley & Sons this book supported file pdf, txt, epub, kindle and other format this book has been release on 2013-05-28 with Mathematics categories.
Methods for estimating sparse and large covariance matrices Covariance and correlation matrices play fundamental roles in every aspect of the analysis of multivariate data collected from a variety of fields including business and economics, health care, engineering, and environmental and physical sciences. High-Dimensional Covariance Estimation provides accessible and comprehensive coverage of the classical and modern approaches for estimating covariance matrices as well as their applications to the rapidly developing areas lying at the intersection of statistics and machine learning. Recently, the classical sample covariance methodologies have been modified and improved upon to meet the needs of statisticians and researchers dealing with large correlated datasets. High-Dimensional Covariance Estimation focuses on the methodologies based on shrinkage, thresholding, and penalized likelihood with applications to Gaussian graphical models, prediction, and mean-variance portfolio management. The book relies heavily on regression-based ideas and interpretations to connect and unify many existing methods and algorithms for the task. High-Dimensional Covariance Estimation features chapters on: Data, Sparsity, and Regularization Regularizing the Eigenstructure Banding, Tapering, and Thresholding Covariance Matrices Sparse Gaussian Graphical Models Multivariate Regression The book is an ideal resource for researchers in statistics, mathematics, business and economics, computer sciences, and engineering, as well as a useful text or supplement for graduate-level courses in multivariate analysis, covariance estimation, statistical learning, and high-dimensional data analysis.
Smooth Forecast Reconciliation
DOWNLOAD
Author : Mr. Sakai Ando
language : en
Publisher: International Monetary Fund
Release Date : 2024-03-22
Smooth Forecast Reconciliation written by Mr. Sakai Ando and has been published by International Monetary Fund this book supported file pdf, txt, epub, kindle and other format this book has been release on 2024-03-22 with Business & Economics categories.
How to make forecasts that (1) satisfy constraints, like accounting identities, and (2) are smooth over time? Solving this common forecasting problem manually is resource-intensive, but the existing literature provides little guidance on how to achieve both objectives. This paper proposes a new method to smooth mixed-frequency multivariate time series subject to constraints by integrating the minimum-trace reconciliation and Hodrick-Prescott filter. With linear constraints, the method has a closed-form solution, convenient for a high-dimensional environment. Three examples show that the proposed method can reproduce the smoothness of professional forecasts subject to various constraints and slightly improve forecast performance.
Introduction To High Dimensional Statistics
DOWNLOAD
Author : Christophe Giraud
language : en
Publisher: CRC Press
Release Date : 2021-08-25
Introduction To High Dimensional Statistics written by Christophe Giraud and has been published by CRC Press this book supported file pdf, txt, epub, kindle and other format this book has been release on 2021-08-25 with Business & Economics categories.
Praise for the first edition: "[This book] succeeds singularly at providing a structured introduction to this active field of research. ... it is arguably the most accessible overview yet published of the mathematical ideas and principles that one needs to master to enter the field of high-dimensional statistics. ... recommended to anyone interested in the main results of current research in high-dimensional statistics as well as anyone interested in acquiring the core mathematical skills to enter this area of research." —Journal of the American Statistical Association Introduction to High-Dimensional Statistics, Second Edition preserves the philosophy of the first edition: to be a concise guide for students and researchers discovering the area and interested in the mathematics involved. The main concepts and ideas are presented in simple settings, avoiding thereby unessential technicalities. High-dimensional statistics is a fast-evolving field, and much progress has been made on a large variety of topics, providing new insights and methods. Offering a succinct presentation of the mathematical foundations of high-dimensional statistics, this new edition: Offers revised chapters from the previous edition, with the inclusion of many additional materials on some important topics, including compress sensing, estimation with convex constraints, the slope estimator, simultaneously low-rank and row-sparse linear regression, or aggregation of a continuous set of estimators. Introduces three new chapters on iterative algorithms, clustering, and minimax lower bounds. Provides enhanced appendices, minimax lower-bounds mainly with the addition of the Davis-Kahan perturbation bound and of two simple versions of the Hanson-Wright concentration inequality. Covers cutting-edge statistical methods including model selection, sparsity and the Lasso, iterative hard thresholding, aggregation, support vector machines, and learning theory. Provides detailed exercises at the end of every chapter with collaborative solutions on a wiki site. Illustrates concepts with simple but clear practical examples.
Volatility And Correlation
DOWNLOAD
Author : Riccardo Rebonato
language : en
Publisher: John Wiley & Sons
Release Date : 2005-07-08
Volatility And Correlation written by Riccardo Rebonato and has been published by John Wiley & Sons this book supported file pdf, txt, epub, kindle and other format this book has been release on 2005-07-08 with Business & Economics categories.
In Volatility and Correlation 2nd edition: The Perfect Hedger and the Fox, Rebonato looks at derivatives pricing from the angle of volatility and correlation. With both practical and theoretical applications, this is a thorough update of the highly successful Volatility & Correlation – with over 80% new or fully reworked material and is a must have both for practitioners and for students. The new and updated material includes a critical examination of the ‘perfect-replication’ approach to derivatives pricing, with special attention given to exotic options; a thorough analysis of the role of quadratic variation in derivatives pricing and hedging; a discussion of the informational efficiency of markets in commonly-used calibration and hedging practices. Treatment of new models including Variance Gamma, displaced diffusion, stochastic volatility for interest-rate smiles and equity/FX options. The book is split into four parts. Part I deals with a Black world without smiles, sets out the author’s ‘philosophical’ approach and covers deterministic volatility. Part II looks at smiles in equity and FX worlds. It begins with a review of relevant empirical information about smiles, and provides coverage of local-stochastic-volatility, general-stochastic-volatility, jump-diffusion and Variance-Gamma processes. Part II concludes with an important chapter that discusses if and to what extent one can dispense with an explicit specification of a model, and can directly prescribe the dynamics of the smile surface. Part III focusses on interest rates when the volatility is deterministic. Part IV extends this setting in order to account for smiles in a financially motivated and computationally tractable manner. In this final part the author deals with CEV processes, with diffusive stochastic volatility and with Markov-chain processes. Praise for the First Edition: “In this book, Dr Rebonato brings his penetrating eye to bear on option pricing and hedging.... The book is a must-read for those who already know the basics of options and are looking for an edge in applying the more sophisticated approaches that have recently been developed.” —Professor Ian Cooper, London Business School “Volatility and correlation are at the very core of all option pricing and hedging. In this book, Riccardo Rebonato presents the subject in his characteristically elegant and simple fashion...A rare combination of intellectual insight and practical common sense.” —Anthony Neuberger, London Business School
Statistical Foundations Of Data Science
DOWNLOAD
Author : Jianqing Fan
language : en
Publisher: CRC Press
Release Date : 2020-09-21
Statistical Foundations Of Data Science written by Jianqing Fan and has been published by CRC Press this book supported file pdf, txt, epub, kindle and other format this book has been release on 2020-09-21 with Mathematics categories.
Statistical Foundations of Data Science gives a thorough introduction to commonly used statistical models, contemporary statistical machine learning techniques and algorithms, along with their mathematical insights and statistical theories. It aims to serve as a graduate-level textbook and a research monograph on high-dimensional statistics, sparsity and covariance learning, machine learning, and statistical inference. It includes ample exercises that involve both theoretical studies as well as empirical applications. The book begins with an introduction to the stylized features of big data and their impacts on statistical analysis. It then introduces multiple linear regression and expands the techniques of model building via nonparametric regression and kernel tricks. It provides a comprehensive account on sparsity explorations and model selections for multiple regression, generalized linear models, quantile regression, robust regression, hazards regression, among others. High-dimensional inference is also thoroughly addressed and so is feature screening. The book also provides a comprehensive account on high-dimensional covariance estimation, learning latent factors and hidden structures, as well as their applications to statistical estimation, inference, prediction and machine learning problems. It also introduces thoroughly statistical machine learning theory and methods for classification, clustering, and prediction. These include CART, random forests, boosting, support vector machines, clustering algorithms, sparse PCA, and deep learning.
An Introduction To Matrix Concentration Inequalities
DOWNLOAD
Author : Joel Tropp
language : en
Publisher:
Release Date : 2015-05-27
An Introduction To Matrix Concentration Inequalities written by Joel Tropp and has been published by this book supported file pdf, txt, epub, kindle and other format this book has been release on 2015-05-27 with Computers categories.
Random matrices now play a role in many areas of theoretical, applied, and computational mathematics. It is therefore desirable to have tools for studying random matrices that are flexible, easy to use, and powerful. Over the last fifteen years, researchers have developed a remarkable family of results, called matrix concentration inequalities, that achieve all of these goals. This monograph offers an invitation to the field of matrix concentration inequalities. It begins with some history of random matrix theory; it describes a flexible model for random matrices that is suitable for many problems; and it discusses the most important matrix concentration results. To demonstrate the value of these techniques, the presentation includes examples drawn from statistics, machine learning, optimization, combinatorics, algorithms, scientific computing, and beyond.
Mathematical And Statistical Methods For Actuarial Sciences And Finance
DOWNLOAD
Author : Marco Corazza
language : en
Publisher: Springer Nature
Release Date : 2021-12-13
Mathematical And Statistical Methods For Actuarial Sciences And Finance written by Marco Corazza and has been published by Springer Nature this book supported file pdf, txt, epub, kindle and other format this book has been release on 2021-12-13 with Business & Economics categories.
The cooperation and contamination between mathematicians, statisticians and econometricians working in actuarial sciences and finance is improving the research on these topics and producing numerous meaningful scientific results. This volume presents new ideas, in the form of four- to six-page papers, presented at the International Conference eMAF2020 – Mathematical and Statistical Methods for Actuarial Sciences and Finance. Due to the now sadly famous COVID-19 pandemic, the conference was held remotely through the Zoom platform offered by the Department of Economics of the Ca’ Foscari University of Venice on September 18, 22 and 25, 2020. eMAF2020 is the ninth edition of an international biennial series of scientific meetings, started in 2004 at the initiative of the Department of Economics and Statistics of the University of Salerno. The effectiveness of this idea has been proven by wide participation in all editions, which have been held in Salerno (2004, 2006, 2010 and 2014), Venice (2008, 2012 and 2020), Paris (2016) and Madrid (2018). This book covers a wide variety of subjects: artificial intelligence and machine learning in finance and insurance, behavioral finance, credit risk methods and models, dynamic optimization in finance, financial data analytics, forecasting dynamics of actuarial and financial phenomena, foreign exchange markets, insurance models, interest rate models, longevity risk, models and methods for financial time series analysis, multivariate techniques for financial markets analysis, pension systems, portfolio selection and management, real-world finance, risk analysis and management, trading systems, and others. This volume is a valuable resource for academics, PhD students, practitioners, professionals and researchers. Moreover, it is also of interest to other readers with quantitative background knowledge.
Comprehensive Chemometrics
DOWNLOAD
Author : Steven Brown
language : en
Publisher: Elsevier
Release Date : 2020-05-26
Comprehensive Chemometrics written by Steven Brown and has been published by Elsevier this book supported file pdf, txt, epub, kindle and other format this book has been release on 2020-05-26 with Science categories.
Comprehensive Chemometrics, Second Edition, Four Volume Set features expanded and updated coverage, along with new content that covers advances in the field since the previous edition published in 2009. Subject of note include updates in the fields of multidimensional and megavariate data analysis, omics data analysis, big chemical and biochemical data analysis, data fusion and sparse methods. The book follows a similar structure to the previous edition, using the same section titles to frame articles. Many chapters from the previous edition are updated, but there are also many new chapters on the latest developments. Presents integrated reviews of each chemical and biological method, examining their merits and limitations through practical examples and extensive visuals Bridges a gap in knowledge, covering developments in the field since the first edition published in 2009 Meticulously organized, with articles split into 4 sections and 12 sub-sections on key topics to allow students, researchers and professionals to find relevant information quickly and easily Written by academics and practitioners from various fields and regions to ensure that the knowledge within is easily understood and applicable to a large audience Presents integrated reviews of each chemical and biological method, examining their merits and limitations through practical examples and extensive visuals Bridges a gap in knowledge, covering developments in the field since the first edition published in 2009 Meticulously organized, with articles split into 4 sections and 12 sub-sections on key topics to allow students, researchers and professionals to find relevant information quickly and easily Written by academics and practitioners from various fields and regions to ensure that the knowledge within is easily understood and applicable to a large audience
Spectral Analysis Of Large Dimensional Random Matrices
DOWNLOAD
Author : Zhidong Bai
language : en
Publisher: Springer Science & Business Media
Release Date : 2009-12-10
Spectral Analysis Of Large Dimensional Random Matrices written by Zhidong Bai and has been published by Springer Science & Business Media this book supported file pdf, txt, epub, kindle and other format this book has been release on 2009-12-10 with Mathematics categories.
The aim of the book is to introduce basic concepts, main results, and widely applied mathematical tools in the spectral analysis of large dimensional random matrices. The core of the book focuses on results established under moment conditions on random variables using probabilistic methods, and is thus easily applicable to statistics and other areas of science. The book introduces fundamental results, most of them investigated by the authors, such as the semicircular law of Wigner matrices, the Marcenko-Pastur law, the limiting spectral distribution of the multivariate F matrix, limits of extreme eigenvalues, spectrum separation theorems, convergence rates of empirical distributions, central limit theorems of linear spectral statistics, and the partial solution of the famous circular law. While deriving the main results, the book simultaneously emphasizes the ideas and methodologies of the fundamental mathematical tools, among them being: truncation techniques, matrix identities, moment convergence theorems, and the Stieltjes transform. Its treatment is especially fitting to the needs of mathematics and statistics graduate students and beginning researchers, having a basic knowledge of matrix theory and an understanding of probability theory at the graduate level, who desire to learn the concepts and tools in solving problems in this area. It can also serve as a detailed handbook on results of large dimensional random matrices for practical users. This second edition includes two additional chapters, one on the authors' results on the limiting behavior of eigenvectors of sample covariance matrices, another on applications to wireless communications and finance. While attempting to bring this edition up-to-date on recent work, it also provides summaries of other areas which are typically considered part of the general field of random matrix theory.