Interpretability In Deep Learning
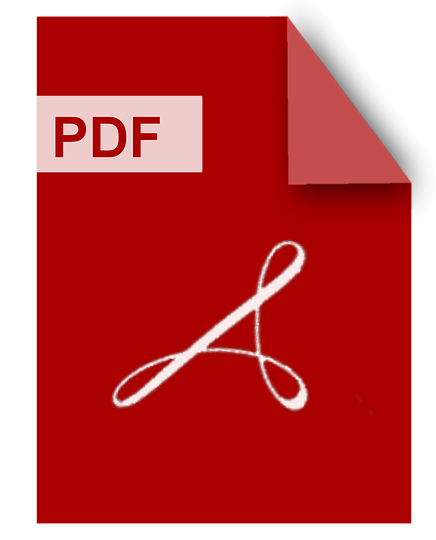
DOWNLOAD
Download Interpretability In Deep Learning PDF/ePub or read online books in Mobi eBooks. Click Download or Read Online button to get Interpretability In Deep Learning book now. This website allows unlimited access to, at the time of writing, more than 1.5 million titles, including hundreds of thousands of titles in various foreign languages. If the content not found or just blank you must refresh this page
Interpretability In Deep Learning
DOWNLOAD
Author : Ayush Somani
language : en
Publisher: Springer Nature
Release Date : 2023-06-01
Interpretability In Deep Learning written by Ayush Somani and has been published by Springer Nature this book supported file pdf, txt, epub, kindle and other format this book has been release on 2023-06-01 with Computers categories.
This book is a comprehensive curation, exposition and illustrative discussion of recent research tools for interpretability of deep learning models, with a focus on neural network architectures. In addition, it includes several case studies from application-oriented articles in the fields of computer vision, optics and machine learning related topic. The book can be used as a monograph on interpretability in deep learning covering the most recent topics as well as a textbook for graduate students. Scientists with research, development and application responsibilities benefit from its systematic exposition.
Interpretable Machine Learning
DOWNLOAD
Author : Christoph Molnar
language : en
Publisher: Lulu.com
Release Date : 2020
Interpretable Machine Learning written by Christoph Molnar and has been published by Lulu.com this book supported file pdf, txt, epub, kindle and other format this book has been release on 2020 with Artificial intelligence categories.
This book is about making machine learning models and their decisions interpretable. After exploring the concepts of interpretability, you will learn about simple, interpretable models such as decision trees, decision rules and linear regression. Later chapters focus on general model-agnostic methods for interpreting black box models like feature importance and accumulated local effects and explaining individual predictions with Shapley values and LIME. All interpretation methods are explained in depth and discussed critically. How do they work under the hood? What are their strengths and weaknesses? How can their outputs be interpreted? This book will enable you to select and correctly apply the interpretation method that is most suitable for your machine learning project.
Interpretable Machine Learning
DOWNLOAD
Author : Christoph Molnar
language : en
Publisher:
Release Date : 2022
Interpretable Machine Learning written by Christoph Molnar and has been published by this book supported file pdf, txt, epub, kindle and other format this book has been release on 2022 with Machine learning categories.
"Machine learning has great potential for improving products, processes and research. But computers usually do not explain their predictions which is a barrier to the adoption of machine learning. This book is about making machine learning models and their decisions interpretable. After exploring the concepts of interpretability, you will learn about simple, interpretable models such as decision trees, decision rules and linear regression. The focus of the book is on model-agnostic methods for interpreting black box models such as feature importance and accumulated local effects, and explaining individual predictions with Shapley values and LIME. In addition, the book presents methods specific to deep neural networks. All interpretation methods are explained in depth and discussed critically. How do they work under the hood? What are their strengths and weaknesses? How can their outputs be interpreted? This book will enable you to select and correctly apply the interpretation method that is most suitable for your machine learning project. Reading the book is recommended for machine learning practitioners, data scientists, statisticians, and anyone else interested in making machine learning models interpretable."--Cover.
Interpretable Machine Learning
DOWNLOAD
Author : Christoph Molnar
language : en
Publisher:
Release Date : 2022
Interpretable Machine Learning written by Christoph Molnar and has been published by this book supported file pdf, txt, epub, kindle and other format this book has been release on 2022 with categories.
Explainable Artificial Intelligence An Introduction To Interpretable Machine Learning
DOWNLOAD
Author : Uday Kamath
language : en
Publisher: Springer Nature
Release Date : 2021-12-15
Explainable Artificial Intelligence An Introduction To Interpretable Machine Learning written by Uday Kamath and has been published by Springer Nature this book supported file pdf, txt, epub, kindle and other format this book has been release on 2021-12-15 with Computers categories.
This book is written both for readers entering the field, and for practitioners with a background in AI and an interest in developing real-world applications. The book is a great resource for practitioners and researchers in both industry and academia, and the discussed case studies and associated material can serve as inspiration for a variety of projects and hands-on assignments in a classroom setting. I will certainly keep this book as a personal resource for the courses I teach, and strongly recommend it to my students. --Dr. Carlotta Domeniconi, Associate Professor, Computer Science Department, GMU This book offers a curriculum for introducing interpretability to machine learning at every stage. The authors provide compelling examples that a core teaching practice like leading interpretive discussions can be taught and learned by teachers and sustained effort. And what better way to strengthen the quality of AI and Machine learning outcomes. I hope that this book will become a primer for teachers, data Science educators, and ML developers, and together we practice the art of interpretive machine learning. --Anusha Dandapani, Chief Data and Analytics Officer, UNICC and Adjunct Faculty, NYU This is a wonderful book! I’m pleased that the next generation of scientists will finally be able to learn this important topic. This is the first book I’ve seen that has up-to-date and well-rounded coverage. Thank you to the authors! --Dr. Cynthia Rudin, Professor of Computer Science, Electrical and Computer Engineering, Statistical Science, and Biostatistics & Bioinformatics Literature on Explainable AI has up until now been relatively scarce and featured mainly mainstream algorithms like SHAP and LIME. This book has closed this gap by providing an extremely broad review of various algorithms proposed in the scientific circles over the previous 5-10 years. This book is a great guide to anyone who is new to the field of XAI or is already familiar with the field and is willing to expand their knowledge. A comprehensive review of the state-of-the-art Explainable AI methods starting from visualization, interpretable methods, local and global explanations, time series methods, and finishing with deep learning provides an unparalleled source of information currently unavailable anywhere else. Additionally, notebooks with vivid examples are a great supplement that makes the book even more attractive for practitioners of any level. Overall, the authors provide readers with an enormous breadth of coverage without losing sight of practical aspects, which makes this book truly unique and a great addition to the library of any data scientist. Dr. Andrey Sharapov, Product Data Scientist, Explainable AI Expert and Speaker, Founder of Explainable AI-XAI Group
Explainable And Interpretable Models In Computer Vision And Machine Learning
DOWNLOAD
Author : Hugo Jair Escalante
language : en
Publisher: Springer
Release Date : 2018-11-29
Explainable And Interpretable Models In Computer Vision And Machine Learning written by Hugo Jair Escalante and has been published by Springer this book supported file pdf, txt, epub, kindle and other format this book has been release on 2018-11-29 with Computers categories.
This book compiles leading research on the development of explainable and interpretable machine learning methods in the context of computer vision and machine learning. Research progress in computer vision and pattern recognition has led to a variety of modeling techniques with almost human-like performance. Although these models have obtained astounding results, they are limited in their explainability and interpretability: what is the rationale behind the decision made? what in the model structure explains its functioning? Hence, while good performance is a critical required characteristic for learning machines, explainability and interpretability capabilities are needed to take learning machines to the next step to include them in decision support systems involving human supervision. This book, written by leading international researchers, addresses key topics of explainability and interpretability, including the following: · Evaluation and Generalization in Interpretable Machine Learning · Explanation Methods in Deep Learning · Learning Functional Causal Models with Generative Neural Networks · Learning Interpreatable Rules for Multi-Label Classification · Structuring Neural Networks for More Explainable Predictions · Generating Post Hoc Rationales of Deep Visual Classification Decisions · Ensembling Visual Explanations · Explainable Deep Driving by Visualizing Causal Attention · Interdisciplinary Perspective on Algorithmic Job Candidate Search · Multimodal Personality Trait Analysis for Explainable Modeling of Job Interview Decisions · Inherent Explainability Pattern Theory-based Video Event Interpretations
Explainable Ai Interpreting Explaining And Visualizing Deep Learning
DOWNLOAD
Author : Wojciech Samek
language : en
Publisher: Springer Nature
Release Date : 2019-09-10
Explainable Ai Interpreting Explaining And Visualizing Deep Learning written by Wojciech Samek and has been published by Springer Nature this book supported file pdf, txt, epub, kindle and other format this book has been release on 2019-09-10 with Computers categories.
The development of “intelligent” systems that can take decisions and perform autonomously might lead to faster and more consistent decisions. A limiting factor for a broader adoption of AI technology is the inherent risks that come with giving up human control and oversight to “intelligent” machines. For sensitive tasks involving critical infrastructures and affecting human well-being or health, it is crucial to limit the possibility of improper, non-robust and unsafe decisions and actions. Before deploying an AI system, we see a strong need to validate its behavior, and thus establish guarantees that it will continue to perform as expected when deployed in a real-world environment. In pursuit of that objective, ways for humans to verify the agreement between the AI decision structure and their own ground-truth knowledge have been explored. Explainable AI (XAI) has developed as a subfield of AI, focused on exposing complex AI models to humans in a systematic and interpretable manner. The 22 chapters included in this book provide a timely snapshot of algorithms, theory, and applications of interpretable and explainable AI and AI techniques that have been proposed recently reflecting the current discourse in this field and providing directions of future development. The book is organized in six parts: towards AI transparency; methods for interpreting AI systems; explaining the decisions of AI systems; evaluating interpretability and explanations; applications of explainable AI; and software for explainable AI.
Deep Learning Interpretability With Visual Analytics
DOWNLOAD
Author : Théo Jaunet
language : en
Publisher:
Release Date : 2022
Deep Learning Interpretability With Visual Analytics written by Théo Jaunet and has been published by this book supported file pdf, txt, epub, kindle and other format this book has been release on 2022 with categories.
Over the past few years, AI and machine learning have evolved from research areas secluded in laboratories far from the public, to technologies deployed on an industrial scale with a considerable impact on our everyday life. As these technologies are also used to solve critical problems such as finance and driving autonomous cars, in which their decisions can put people at risk, this trend has begun to raise legitimate concerns. Since much of the underlying complexity of the decision-making is learned from massive amounts of data, how these models make decisions remains unknown to both their creators and to the people impacted by such decisions. This has led to the new field of eXplainable AI (XAI) and the problem of analyzing the behavior of trained models, to shed light on their reasoning capabilities and the biases to which they are subject. In this thesis, we have contributed to this field with the design of visual analytics systems for the study and improvement of the interpretability of deep neural networks. Our goal was to provide experts with tools to help them better interpret the decisions of their models and eventually improve them. We also proposed applications designed to introduce deep learning methods to a non-expert audience. During this thesis, we focused on the underexplored challenge of model interpretation and improvement for robotics applications, where important decisions have to be made from high-level and high-dimensional inputs such as high level and high dimensional inputs such as images. All the tools designed during this thesis have been published as open-source projects, and when possible, our visualizations have been made available online as prototypes. These tools are highly interactive and can help encourage further interpretation of decision model decisions by the research community.
Interpretable Ai
DOWNLOAD
Author : Ajay Thampi
language : en
Publisher: Simon and Schuster
Release Date : 2022-07-26
Interpretable Ai written by Ajay Thampi and has been published by Simon and Schuster this book supported file pdf, txt, epub, kindle and other format this book has been release on 2022-07-26 with Computers categories.
AI doesn’t have to be a black box. These practical techniques help shine a light on your model’s mysterious inner workings. Make your AI more transparent, and you’ll improve trust in your results, combat data leakage and bias, and ensure compliance with legal requirements. In Interpretable AI, you will learn: Why AI models are hard to interpret Interpreting white box models such as linear regression, decision trees, and generalized additive models Partial dependence plots, LIME, SHAP and Anchors, and other techniques such as saliency mapping, network dissection, and representational learning What fairness is and how to mitigate bias in AI systems Implement robust AI systems that are GDPR-compliant Interpretable AI opens up the black box of your AI models. It teaches cutting-edge techniques and best practices that can make even complex AI systems interpretable. Each method is easy to implement with just Python and open source libraries. You’ll learn to identify when you can utilize models that are inherently transparent, and how to mitigate opacity when your problem demands the power of a hard-to-interpret deep learning model. About the technology It’s often difficult to explain how deep learning models work, even for the data scientists who create them. Improving transparency and interpretability in machine learning models minimizes errors, reduces unintended bias, and increases trust in the outcomes. This unique book contains techniques for looking inside “black box” models, designing accountable algorithms, and understanding the factors that cause skewed results. About the book Interpretable AI teaches you to identify the patterns your model has learned and why it produces its results. As you read, you’ll pick up algorithm-specific approaches, like interpreting regression and generalized additive models, along with tips to improve performance during training. You’ll also explore methods for interpreting complex deep learning models where some processes are not easily observable. AI transparency is a fast-moving field, and this book simplifies cutting-edge research into practical methods you can implement with Python. What's inside Techniques for interpreting AI models Counteract errors from bias, data leakage, and concept drift Measuring fairness and mitigating bias Building GDPR-compliant AI systems About the reader For data scientists and engineers familiar with Python and machine learning. About the author Ajay Thampi is a machine learning engineer focused on responsible AI and fairness. Table of Contents PART 1 INTERPRETABILITY BASICS 1 Introduction 2 White-box models PART 2 INTERPRETING MODEL PROCESSING 3 Model-agnostic methods: Global interpretability 4 Model-agnostic methods: Local interpretability 5 Saliency mapping PART 3 INTERPRETING MODEL REPRESENTATIONS 6 Understanding layers and units 7 Understanding semantic similarity PART 4 FAIRNESS AND BIAS 8 Fairness and mitigating bias 9 Path to explainable AI
Graph Neural Networks Foundations Frontiers And Applications
DOWNLOAD
Author : Lingfei Wu
language : en
Publisher: Springer Nature
Release Date : 2022-01-03
Graph Neural Networks Foundations Frontiers And Applications written by Lingfei Wu and has been published by Springer Nature this book supported file pdf, txt, epub, kindle and other format this book has been release on 2022-01-03 with Computers categories.
Deep Learning models are at the core of artificial intelligence research today. It is well known that deep learning techniques are disruptive for Euclidean data, such as images or sequence data, and not immediately applicable to graph-structured data such as text. This gap has driven a wave of research for deep learning on graphs, including graph representation learning, graph generation, and graph classification. The new neural network architectures on graph-structured data (graph neural networks, GNNs in short) have performed remarkably on these tasks, demonstrated by applications in social networks, bioinformatics, and medical informatics. Despite these successes, GNNs still face many challenges ranging from the foundational methodologies to the theoretical understandings of the power of the graph representation learning. This book provides a comprehensive introduction of GNNs. It first discusses the goals of graph representation learning and then reviews the history, current developments, and future directions of GNNs. The second part presents and reviews fundamental methods and theories concerning GNNs while the third part describes various frontiers that are built on the GNNs. The book concludes with an overview of recent developments in a number of applications using GNNs. This book is suitable for a wide audience including undergraduate and graduate students, postdoctoral researchers, professors and lecturers, as well as industrial and government practitioners who are new to this area or who already have some basic background but want to learn more about advanced and promising techniques and applications.