Kubeflow In Action
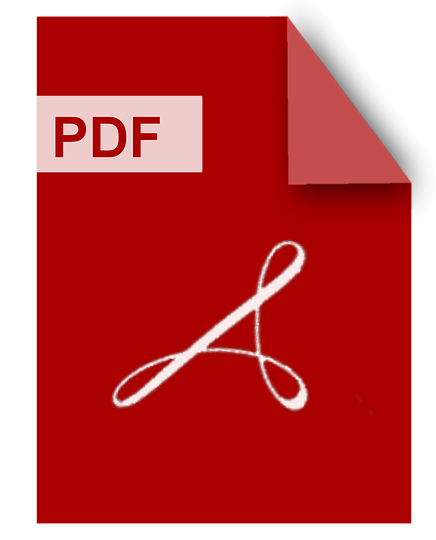
DOWNLOAD eBooks
Download Kubeflow In Action PDF/ePub or read online books in Mobi eBooks. Click Download or Read Online button to get Kubeflow In Action book now. This website allows unlimited access to, at the time of writing, more than 1.5 million titles, including hundreds of thousands of titles in various foreign languages. If the content not found or just blank you must refresh this page
Kubeflow In Action
DOWNLOAD eBooks
Author : Juana Nakfour
language : en
Publisher: Manning
Release Date : 2022-03-29
Kubeflow In Action written by Juana Nakfour and has been published by Manning this book supported file pdf, txt, epub, kindle and other format this book has been release on 2022-03-29 with Computers categories.
Kubeflow simplifies and automates machine learning tasks like interactive analysis, complex pipelines, and model training. Seamlessly push models to production in the containerized and distributed environment and scale your ML infrastructure from your laptop to a Kubernetes cluster. Kubeflow in Action shows you how to utilize Kubeflow to rapidly scale machine learning projects from a laptop to a distributed cluster. You’ll kick off with a rapid introduction to containers, benefit from careful guidance on Kubeflow’s installation and initial setup, and master core Kubeflow tasks like storing data, training models, and monitoring metrics. Detailed use cases help show how to construct complex pipelines, automate hyperparameter tuning, and implement network architecture search. You’ll quickly progress to a deep dive into Kubeflow’s more advanced uses, including training distributed models, deployment, A/B testing, and infrastructure monitoring to help trigger actions based on incoming data. Purchase of the print book includes a free eBook in PDF, Kindle, and ePub formats from Manning Publications.
Kubeflow For Machine Learning
DOWNLOAD eBooks
Author : Trevor Grant
language : en
Publisher: O'Reilly Media
Release Date : 2020-10-13
Kubeflow For Machine Learning written by Trevor Grant and has been published by O'Reilly Media this book supported file pdf, txt, epub, kindle and other format this book has been release on 2020-10-13 with Computers categories.
If you're training a machine learning model but aren't sure how to put it into production, this book will get you there. Kubeflow provides a collection of cloud native tools for different stages of a model's lifecycle, from data exploration, feature preparation, and model training to model serving. This guide helps data scientists build production-grade machine learning implementations with Kubeflow and shows data engineers how to make models scalable and reliable. Using examples throughout the book, authors Holden Karau, Trevor Grant, Ilan Filonenko, Richard Liu, and Boris Lublinsky explain how to use Kubeflow to train and serve your machine learning models on top of Kubernetes in the cloud or in a development environment on-premises. Understand Kubeflow's design, core components, and the problems it solves Understand the differences between Kubeflow on different cluster types Train models using Kubeflow with popular tools including Scikit-learn, TensorFlow, and Apache Spark Keep your model up to date with Kubeflow Pipelines Understand how to capture model training metadata Explore how to extend Kubeflow with additional open source tools Use hyperparameter tuning for training Learn how to serve your model in production
Kubeflow Operations Guide
DOWNLOAD eBooks
Author : Josh Patterson
language : en
Publisher: O'Reilly Media
Release Date : 2020-12-04
Kubeflow Operations Guide written by Josh Patterson and has been published by O'Reilly Media this book supported file pdf, txt, epub, kindle and other format this book has been release on 2020-12-04 with Computers categories.
Building models is a small part of the story when it comes to deploying machine learning applications. The entire process involves developing, orchestrating, deploying, and running scalable and portable machine learning workloads--a process Kubeflow makes much easier. This practical book shows data scientists, data engineers, and platform architects how to plan and execute a Kubeflow project to make their Kubernetes workflows portable and scalable. Authors Josh Patterson, Michael Katzenellenbogen, and Austin Harris demonstrate how this open source platform orchestrates workflows by managing machine learning pipelines. You'll learn how to plan and execute a Kubeflow platform that can support workflows from on-premises to cloud providers including Google, Amazon, and Microsoft. Dive into Kubeflow architecture and learn best practices for using the platform Understand the process of planning your Kubeflow deployment Install Kubeflow on an existing on-premises Kubernetes cluster Deploy Kubeflow on Google Cloud Platform step-by-step from the command line Use the managed Amazon Elastic Kubernetes Service (EKS) to deploy Kubeflow on AWS Deploy and manage Kubeflow across a network of Azure cloud data centers around the world Use KFServing to develop and deploy machine learning models
Kubeflow For Machine Learning
DOWNLOAD eBooks
Author : Trevor Grant
language : en
Publisher: "O'Reilly Media, Inc."
Release Date : 2020-10-13
Kubeflow For Machine Learning written by Trevor Grant and has been published by "O'Reilly Media, Inc." this book supported file pdf, txt, epub, kindle and other format this book has been release on 2020-10-13 with Computers categories.
If you're training a machine learning model but aren't sure how to put it into production, this book will get you there. Kubeflow provides a collection of cloud native tools for different stages of a model's lifecycle, from data exploration, feature preparation, and model training to model serving. This guide helps data scientists build production-grade machine learning implementations with Kubeflow and shows data engineers how to make models scalable and reliable. Using examples throughout the book, authors Holden Karau, Trevor Grant, Ilan Filonenko, Richard Liu, and Boris Lublinsky explain how to use Kubeflow to train and serve your machine learning models on top of Kubernetes in the cloud or in a development environment on-premises. Understand Kubeflow's design, core components, and the problems it solves Understand the differences between Kubeflow on different cluster types Train models using Kubeflow with popular tools including Scikit-learn, TensorFlow, and Apache Spark Keep your model up to date with Kubeflow Pipelines Understand how to capture model training metadata Explore how to extend Kubeflow with additional open source tools Use hyperparameter tuning for training Learn how to serve your model in production
Kubernetes In Action
DOWNLOAD eBooks
Author : Marko Luksa
language : en
Publisher: Manning Publications
Release Date : 2018-01-20
Kubernetes In Action written by Marko Luksa and has been published by Manning Publications this book supported file pdf, txt, epub, kindle and other format this book has been release on 2018-01-20 with Computers categories.
Summary Kubernetes in Action is a comprehensive guide to effectively developing and running applications in a Kubernetes environment. Before diving into Kubernetes, the book gives an overview of container technologies like Docker, including how to build containers, so that even readers who haven't used these technologies before can get up and running. Purchase of the print book includes a free eBook in PDF, Kindle, and ePub formats from Manning Publications. About the Technology Kubernetes is Greek for "helmsman," your guide through unknown waters. The Kubernetes container orchestration system safely manages the structure and flow of a distributed application, organizing containers and services for maximum efficiency. Kubernetes serves as an operating system for your clusters, eliminating the need to factor the underlying network and server infrastructure into your designs. About the Book Kubernetes in Action teaches you to use Kubernetes to deploy container-based distributed applications. You'll start with an overview of Docker and Kubernetes before building your first Kubernetes cluster. You'll gradually expand your initial application, adding features and deepening your knowledge of Kubernetes architecture and operation. As you navigate this comprehensive guide, you'll explore high-value topics like monitoring, tuning, and scaling. What's Inside Kubernetes' internals Deploying containers across a cluster Securing clusters Updating applications with zero downtime About the Reader Written for intermediate software developers with little or no familiarity with Docker or container orchestration systems. About the Author Marko Luksa is an engineer at Red Hat working on Kubernetes and OpenShift. Table of Contents PART 1 - OVERVIEW Introducing Kubernetes First steps with Docker and Kubernetes PART 2 - CORE CONCEPTS Pods: running containers in Kubernetes Replication and other controllers: deploying managed pods Services: enabling clients to discover and talk to pods Volumes: attaching disk storage to containers ConfigMaps and Secrets: configuring applications Accessing pod metadata and other resources from applications Deployments: updating applications declaratively StatefulSets: deploying replicated stateful applications PART 3 - BEYOND THE BASICS Understanding Kubernetes internals Securing the Kubernetes API server Securing cluster nodes and the network Managing pods' computational resources Automatic scaling of pods and cluster nodes Advanced scheduling Best practices for developing apps Extending Kubernetes
Kubeflow Operations Guide
DOWNLOAD eBooks
Author : Josh Patterson
language : en
Publisher:
Release Date : 2020
Kubeflow Operations Guide written by Josh Patterson and has been published by this book supported file pdf, txt, epub, kindle and other format this book has been release on 2020 with categories.
When deploying machine learning applications, building models is only a small part of the story. The entire process involves developing, orchestrating, deploying, and running scalable and portable machine learning workloads-a process Kubeflow makes much easier. With this practical guide, data scientists, data engineers, and platform architects will learn how to plan and execute a Kubeflow project that can support workflows from on-premises to the cloud. Kubeflow is an open source Kubernetes-native platform based on Google's internal machine learning pipelines, and yet major cloud vendors including AWS and Azure advocate the use of Kubernetes and Kubeflow to manage containers and machine learning infrastructure. In today's cloud-based world, this book is ideal for any team planning to build machine learning applications. With this book, you will: Get a concise overview of Kubernetes and Kubeflow Learn how to plan and build a Kubeflow installation Operate, monitor, and automate your installation Provide your Kubeflow installation with adequate security Serve machine learning models on Kubeflow.
Distributed Machine Learning Patterns
DOWNLOAD eBooks
Author : Yuan Tang
language : en
Publisher: Simon and Schuster
Release Date : 2024-01-30
Distributed Machine Learning Patterns written by Yuan Tang and has been published by Simon and Schuster this book supported file pdf, txt, epub, kindle and other format this book has been release on 2024-01-30 with Computers categories.
Practical patterns for scaling machine learning from your laptop to a distributed cluster. Distributing machine learning systems allow developers to handle extremely large datasets across multiple clusters, take advantage of automation tools, and benefit from hardware accelerations. This book reveals best practice techniques and insider tips for tackling the challenges of scaling machine learning systems. In Distributed Machine Learning Patterns you will learn how to: Apply distributed systems patterns to build scalable and reliable machine learning projects Build ML pipelines with data ingestion, distributed training, model serving, and more Automate ML tasks with Kubernetes, TensorFlow, Kubeflow, and Argo Workflows Make trade-offs between different patterns and approaches Manage and monitor machine learning workloads at scale Inside Distributed Machine Learning Patterns you’ll learn to apply established distributed systems patterns to machine learning projects—plus explore cutting-edge new patterns created specifically for machine learning. Firmly rooted in the real world, this book demonstrates how to apply patterns using examples based in TensorFlow, Kubernetes, Kubeflow, and Argo Workflows. Hands-on projects and clear, practical DevOps techniques let you easily launch, manage, and monitor cloud-native distributed machine learning pipelines. About the technology Deploying a machine learning application on a modern distributed system puts the spotlight on reliability, performance, security, and other operational concerns. In this in-depth guide, Yuan Tang, project lead of Argo and Kubeflow, shares patterns, examples, and hard-won insights on taking an ML model from a single device to a distributed cluster. About the book Distributed Machine Learning Patterns provides dozens of techniques for designing and deploying distributed machine learning systems. In it, you’ll learn patterns for distributed model training, managing unexpected failures, and dynamic model serving. You’ll appreciate the practical examples that accompany each pattern along with a full-scale project that implements distributed model training and inference with autoscaling on Kubernetes. What's inside Data ingestion, distributed training, model serving, and more Automating Kubernetes and TensorFlow with Kubeflow and Argo Workflows Manage and monitor workloads at scale About the reader For data analysts and engineers familiar with the basics of machine learning, Bash, Python, and Docker. About the author Yuan Tang is a project lead of Argo and Kubeflow, maintainer of TensorFlow and XGBoost, and author of numerous open source projects. Table of Contents PART 1 BASIC CONCEPTS AND BACKGROUND 1 Introduction to distributed machine learning systems PART 2 PATTERNS OF DISTRIBUTED MACHINE LEARNING SYSTEMS 2 Data ingestion patterns 3 Distributed training patterns 4 Model serving patterns 5 Workflow patterns 6 Operation patterns PART 3 BUILDING A DISTRIBUTED MACHINE LEARNING WORKFLOW 7 Project overview and system architecture 8 Overview of relevant technologies 9 A complete implementation
Building Machine Learning Pipelines
DOWNLOAD eBooks
Author : Hannes Hapke
language : en
Publisher: "O'Reilly Media, Inc."
Release Date : 2020-07-13
Building Machine Learning Pipelines written by Hannes Hapke and has been published by "O'Reilly Media, Inc." this book supported file pdf, txt, epub, kindle and other format this book has been release on 2020-07-13 with Computers categories.
Companies are spending billions on machine learning projects, but it’s money wasted if the models can’t be deployed effectively. In this practical guide, Hannes Hapke and Catherine Nelson walk you through the steps of automating a machine learning pipeline using the TensorFlow ecosystem. You’ll learn the techniques and tools that will cut deployment time from days to minutes, so that you can focus on developing new models rather than maintaining legacy systems. Data scientists, machine learning engineers, and DevOps engineers will discover how to go beyond model development to successfully productize their data science projects, while managers will better understand the role they play in helping to accelerate these projects. Understand the steps to build a machine learning pipeline Build your pipeline using components from TensorFlow Extended Orchestrate your machine learning pipeline with Apache Beam, Apache Airflow, and Kubeflow Pipelines Work with data using TensorFlow Data Validation and TensorFlow Transform Analyze a model in detail using TensorFlow Model Analysis Examine fairness and bias in your model performance Deploy models with TensorFlow Serving or TensorFlow Lite for mobile devices Learn privacy-preserving machine learning techniques
Google Anthos In Action
DOWNLOAD eBooks
Author : Antonio Gulli
language : en
Publisher: Simon and Schuster
Release Date : 2023-07-18
Google Anthos In Action written by Antonio Gulli and has been published by Simon and Schuster this book supported file pdf, txt, epub, kindle and other format this book has been release on 2023-07-18 with Computers categories.
Learn Anthos directly from the Google development team! Anthos delivers a consistent management platform for deploying and operating Linux and Windows applications anywhere—multicloud, edge, on-prem, bare metal, or VMware. Google Anthos in Action comes directly from the Anthos team at Google. This comprehensive book takes a true DevOps mindset, considering Google-tested patterns for how an application is designed, built, deployed, managed, monitored, and scaled. In a cloud-centric world, all deployment is becoming hybrid deployment. Anthos is a modern, Kubernetes-based cloud platform that enables you to run your software in multicloud, hybrid, or on-premises deployments using the same operations tools and approach. With powerful automation features, it boosts your efficiency along the whole development lifecycle. Google Anthos in Action demystifies Anthos with practical examples of Anthos at work and invaluable insights from the Google team that built it. Purchase of the print book includes a free eBook in PDF, Kindle, and ePub formats from Manning Publications.
Machine Learning Bookcamp
DOWNLOAD eBooks
Author : Alexey Grigorev
language : en
Publisher: Simon and Schuster
Release Date : 2021-11-23
Machine Learning Bookcamp written by Alexey Grigorev and has been published by Simon and Schuster this book supported file pdf, txt, epub, kindle and other format this book has been release on 2021-11-23 with Computers categories.
Time to flex your machine learning muscles! Take on the carefully designed challenges of the Machine Learning Bookcamp and master essential ML techniques through practical application. Summary In Machine Learning Bookcamp you will: Collect and clean data for training models Use popular Python tools, including NumPy, Scikit-Learn, and TensorFlow Apply ML to complex datasets with images Deploy ML models to a production-ready environment The only way to learn is to practice! In Machine Learning Bookcamp, you’ll create and deploy Python-based machine learning models for a variety of increasingly challenging projects. Taking you from the basics of machine learning to complex applications such as image analysis, each new project builds on what you’ve learned in previous chapters. You’ll build a portfolio of business-relevant machine learning projects that hiring managers will be excited to see. Purchase of the print book includes a free eBook in PDF, Kindle, and ePub formats from Manning Publications. About the technology Master key machine learning concepts as you build actual projects! Machine learning is what you need for analyzing customer behavior, predicting price trends, evaluating risk, and much more. To master ML, you need great examples, clear explanations, and lots of practice. This book delivers all three! About the book Machine Learning Bookcamp presents realistic, practical machine learning scenarios, along with crystal-clear coverage of key concepts. In it, you’ll complete engaging projects, such as creating a car price predictor using linear regression and deploying a churn prediction service. You’ll go beyond the algorithms and explore important techniques like deploying ML applications on serverless systems and serving models with Kubernetes and Kubeflow. Dig in, get your hands dirty, and have fun building your ML skills! What's inside Collect and clean data for training models Use popular Python tools, including NumPy, Scikit-Learn, and TensorFlow Deploy ML models to a production-ready environment About the reader Python programming skills assumed. No previous machine learning knowledge is required. About the author Alexey Grigorev is a principal data scientist at OLX Group. He runs DataTalks.Club, a community of people who love data. Table of Contents 1 Introduction to machine learning 2 Machine learning for regression 3 Machine learning for classification 4 Evaluation metrics for classification 5 Deploying machine learning models 6 Decision trees and ensemble learning 7 Neural networks and deep learning 8 Serverless deep learning 9 Serving models with Kubernetes and Kubeflow