Learning Video Representation From Self Supervision
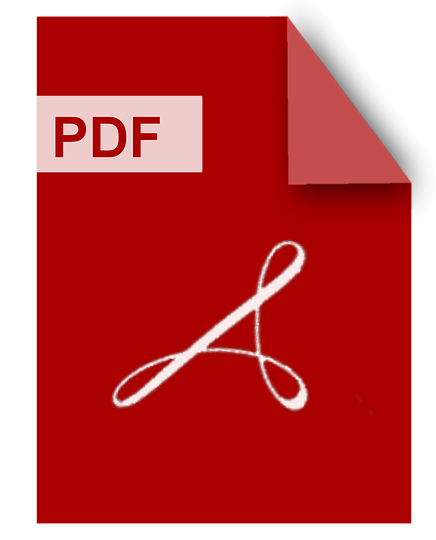
DOWNLOAD
Download Learning Video Representation From Self Supervision PDF/ePub or read online books in Mobi eBooks. Click Download or Read Online button to get Learning Video Representation From Self Supervision book now. This website allows unlimited access to, at the time of writing, more than 1.5 million titles, including hundreds of thousands of titles in various foreign languages. If the content not found or just blank you must refresh this page
Learning Video Representation From Self Supervision
DOWNLOAD
Author : Brian Chen
language : en
Publisher:
Release Date : 2023
Learning Video Representation From Self Supervision written by Brian Chen and has been published by this book supported file pdf, txt, epub, kindle and other format this book has been release on 2023 with categories.
This thesis investigates the problem of learning video representations for video understanding. Previous works have explored the use of data-driven deep learning approaches, which have been shown to be effective in learning useful video representations. However, obtaining large amounts of labeled data can be costly and time-consuming. We investigate self-supervised approach as for multimodal video data to overcome this challenge. Video data typically contains multiple modalities, such as visual, audio, transcribed speech, and textual captions, which can serve as pseudo-labels for representation learning without needing manual labeling. By utilizing these modalities, we can train deep representations over large-scale video data consisting of millions of video clips collected from the internet. We demonstrate the scalability benefits of multimodal self-supervision by achieving new state-of-the-art performance in various domains, including video action recognition, text-to-video retrieval, and text-to-video grounding.
Self Supervised Video Representation Learning
DOWNLOAD
Author : Tengda Han
language : en
Publisher:
Release Date : 2022
Self Supervised Video Representation Learning written by Tengda Han and has been published by this book supported file pdf, txt, epub, kindle and other format this book has been release on 2022 with categories.
Self Supervised Video Representation Learning By Recurrent Networks And Frame Order Predition
DOWNLOAD
Author : Sai Shashidhar Nagabandi
language : en
Publisher:
Release Date : 2020
Self Supervised Video Representation Learning By Recurrent Networks And Frame Order Predition written by Sai Shashidhar Nagabandi and has been published by this book supported file pdf, txt, epub, kindle and other format this book has been release on 2020 with Computer vision categories.
"The success of deep learning models in challenging tasks of computer vision and natural language processing depend on good vector representations of data. For example, learning efficient and salient video representations is one of the fundamental steps for many tasks like action recognition and next frame prediction. Most methods in deep learning rely on large datasets like ImageNet or MSCOCO for training, which is expensive and time consuming to collect. Some of the earlier works in video representation learning relied on encoder-decoder style networks in an unsupervised fashion, which would take in a few frames at a time. Research in the field of self-supervised learning is growing, and has shown promising results on image-related tasks to both learn data representations as well as pre-learn weights for networks using unlabeled data. However, many of these techniques use static architectures like AlexNet, which fail to take into account the temporal aspect of videos. Learning frame-to-frame temporal relationships is essential to learning latent representations of video. In our work, we propose to learn this temporality by pairing static encodings with a recurrent long short term memory network. This research will also investigate applying different methods of encoding architecture along with the recurrent network, to take in a range of different number of frames. We also introduce a novel self-supervised task in which the neural network has two tasks; predicting if a tuple of input frames is temporally consistent, and if not, predict the positioning of incorrect tuple. The efficacy is finally measured by using these trained networks on downstream tasks like action recognition on standard datasets UCF101 and HMDB51."--Abstract.
Deep Learning For Video Understanding
DOWNLOAD
Author : Zuxuan Wu
language : en
Publisher: Springer Nature
Release Date :
Deep Learning For Video Understanding written by Zuxuan Wu and has been published by Springer Nature this book supported file pdf, txt, epub, kindle and other format this book has been release on with categories.
Video Efficient Foundation Models
DOWNLOAD
Author : Fida Mohammad Thoker
language : en
Publisher:
Release Date : 2023
Video Efficient Foundation Models written by Fida Mohammad Thoker and has been published by this book supported file pdf, txt, epub, kindle and other format this book has been release on 2023 with categories.
"The thesis strives to endow video -efficiency in video understanding by addressing the research question '' What enables video- efficient video foundation models ?'' Video -efficiency encompasses developing video foundation models that are not only accurate but also exhibit label-efficiency i.e. require fewer labels, domain-efficiency i.e. applicable to a variety of video learning scenarios, and data-efficiency i.e. reduce the amount of video data needed for learning. The research question is addressed for RGB and non-RGB video modalities. In Chapter 2, we focus on improving the label- and domain-efficiency of non-RGB action recognition and detection. Chapter 3 introduces a new self-supervised approach for learning feature representations for 3D-skeleton video sequences. In Chapter 4, we conduct a large-scale study of existing RGB-based self-supervised video models to assess their performance across different facets of video -efficiency. Chapter 5 presents a new method for video self-supervision that explicitly aims to learn motion focused video -representations. To summarize, this thesis presents several novel approaches to improve the video -efficiency of video foundation models . Our research highlights the importance of transferring knowledge between RGB and non-RGB video modalities, exploring self- supervision for non- RGB video modeling, analyzing self-supervised models beyond canonical setups and carefully designing new self-supervised tasks to develop video foundation models that can exhibit different facets of video -efficiency. We hope that our work will inspire further research and development in this area, leading to even more video- efficient foundation models."--
Person Re Identification With Limited Supervision
DOWNLOAD
Author : Rameswar Panda
language : en
Publisher: Morgan & Claypool Publishers
Release Date : 2021-09-30
Person Re Identification With Limited Supervision written by Rameswar Panda and has been published by Morgan & Claypool Publishers this book supported file pdf, txt, epub, kindle and other format this book has been release on 2021-09-30 with Computers categories.
Person re-identification is the problem of associating observations of targets in different non-overlapping cameras. Most of the existing learning-based methods have resulted in improved performance on standard re-identification benchmarks, but at the cost of time-consuming and tediously labeled data. Motivated by this, learning person re-identification models with limited to no supervision has drawn a great deal of attention in recent years. In this book, we provide an overview of some of the literature in person re-identification, and then move on to focus on some specific problems in the context of person re-identification with limited supervision in multi-camera environments. We expect this to lead to interesting problems for researchers to consider in the future, beyond the conventional fully supervised setup that has been the framework for a lot of work in person re-identification. Chapter 1 starts with an overview of the problems in person re-identification and the major research directions. We provide an overview of the prior works that align most closely with the limited supervision theme of this book. Chapter 2 demonstrates how global camera network constraints in the form of consistency can be utilized for improving the accuracy of camera pair-wise person re-identification models and also selecting a minimal subset of image pairs for labeling without compromising accuracy. Chapter 3 presents two methods that hold the potential for developing highly scalable systems for video person re-identification with limited supervision. In the one-shot setting where only one tracklet per identity is labeled, the objective is to utilize this small labeled set along with a larger unlabeled set of tracklets to obtain a re-identification model. Another setting is completely unsupervised without requiring any identity labels. The temporal consistency in the videos allows us to infer about matching objects across the cameras with higher confidence, even with limited to no supervision. Chapter 4 investigates person re-identification in dynamic camera networks. Specifically, we consider a novel problem that has received very little attention in the community but is critically important for many applications where a new camera is added to an existing group observing a set of targets. We propose two possible solutions for on-boarding new camera(s) dynamically to an existing network using transfer learning with limited additional supervision. Finally, Chapter 5 concludes the book by highlighting the major directions for future research.
Computer Vision Eccv 2022
DOWNLOAD
Author : Shai Avidan
language : en
Publisher: Springer Nature
Release Date : 2022-11-02
Computer Vision Eccv 2022 written by Shai Avidan and has been published by Springer Nature this book supported file pdf, txt, epub, kindle and other format this book has been release on 2022-11-02 with Computers categories.
The 39-volume set, comprising the LNCS books 13661 until 13699, constitutes the refereed proceedings of the 17th European Conference on Computer Vision, ECCV 2022, held in Tel Aviv, Israel, during October 23–27, 2022. The 1645 papers presented in these proceedings were carefully reviewed and selected from a total of 5804 submissions. The papers deal with topics such as computer vision; machine learning; deep neural networks; reinforcement learning; object recognition; image classification; image processing; object detection; semantic segmentation; human pose estimation; 3d reconstruction; stereo vision; computational photography; neural networks; image coding; image reconstruction; object recognition; motion estimation.
Computer Vision Eccv 2022 Workshops
DOWNLOAD
Author : Leonid Karlinsky
language : en
Publisher: Springer Nature
Release Date : 2023-02-13
Computer Vision Eccv 2022 Workshops written by Leonid Karlinsky and has been published by Springer Nature this book supported file pdf, txt, epub, kindle and other format this book has been release on 2023-02-13 with Computers categories.
The 8-volume set, comprising the LNCS books 13801 until 13809, constitutes the refereed proceedings of 38 out of the 60 workshops held at the 17th European Conference on Computer Vision, ECCV 2022. The conference took place in Tel Aviv, Israel, during October 23-27, 2022; the workshops were held hybrid or online. The 367 full papers included in this volume set were carefully reviewed and selected for inclusion in the ECCV 2022 workshop proceedings. They were organized in individual parts as follows: Part I: W01 - AI for Space; W02 - Vision for Art; W03 - Adversarial Robustness in the Real World; W04 - Autonomous Vehicle Vision Part II: W05 - Learning With Limited and Imperfect Data; W06 - Advances in Image Manipulation; Part III: W07 - Medical Computer Vision; W08 - Computer Vision for Metaverse; W09 - Self-Supervised Learning: What Is Next?; Part IV: W10 - Self-Supervised Learning for Next-Generation Industry-Level Autonomous Driving; W11 - ISIC Skin Image Analysis; W12 - Cross-Modal Human-Robot Interaction; W13 - Text in Everything; W14 - BioImage Computing; W15 - Visual Object-Oriented Learning Meets Interaction: Discovery, Representations, and Applications; W16 - AI for Creative Video Editing and Understanding; W17 - Visual Inductive Priors for Data-Efficient Deep Learning; W18 - Mobile Intelligent Photography and Imaging; Part V: W19 - People Analysis: From Face, Body and Fashion to 3D Virtual Avatars; W20 - Safe Artificial Intelligence for Automated Driving; W21 - Real-World Surveillance: Applications and Challenges; W22 - Affective Behavior Analysis In-the-Wild; Part VI: W23 - Visual Perception for Navigation in Human Environments: The JackRabbot Human Body Pose Dataset and Benchmark; W24 - Distributed Smart Cameras; W25 - Causality in Vision; W26 - In-Vehicle Sensing and Monitorization; W27 - Assistive Computer Vision and Robotics; W28 - Computational Aspects of Deep Learning; Part VII: W29 - Computer Vision for Civil and Infrastructure Engineering; W30 - AI-Enabled Medical Image Analysis: Digital Pathology and Radiology/COVID19; W31 - Compositional and Multimodal Perception; Part VIII: W32 - Uncertainty Quantification for Computer Vision; W33 - Recovering 6D Object Pose; W34 - Drawings and Abstract Imagery: Representation and Analysis; W35 - Sign Language Understanding; W36 - A Challenge for Out-of-Distribution Generalization in Computer Vision; W37 - Vision With Biased or Scarce Data; W38 - Visual Object Tracking Challenge.
Pattern Recognition
DOWNLOAD
Author : Zeynep Akata
language : en
Publisher: Springer Nature
Release Date : 2021-03-16
Pattern Recognition written by Zeynep Akata and has been published by Springer Nature this book supported file pdf, txt, epub, kindle and other format this book has been release on 2021-03-16 with Computers categories.
This book constitutes the refereed proceedings of the 42nd German Conference on Pattern Recognition, DAGM GCPR 2020, which took place during September 28 until October 1, 2020. The conference was planned to take place in Tübingen, Germany, but had to change to an online format due to the COVID-19 pandemic. The 34 papers presented in this volume were carefully reviewed and selected from a total of 89 submissions. They were organized in topical sections named: Normalizing Flow, Semantics, Physics, Camera Calibration and Computer Vision, Pattern Recognition, Machine Learning.
Computer Vision Eccv 2020
DOWNLOAD
Author : Andrea Vedaldi
language : en
Publisher: Springer Nature
Release Date : 2020-11-18
Computer Vision Eccv 2020 written by Andrea Vedaldi and has been published by Springer Nature this book supported file pdf, txt, epub, kindle and other format this book has been release on 2020-11-18 with Computers categories.
The 30-volume set, comprising the LNCS books 12346 until 12375, constitutes the refereed proceedings of the 16th European Conference on Computer Vision, ECCV 2020, which was planned to be held in Glasgow, UK, during August 23-28, 2020. The conference was held virtually due to the COVID-19 pandemic. The 1360 revised papers presented in these proceedings were carefully reviewed and selected from a total of 5025 submissions. The papers deal with topics such as computer vision; machine learning; deep neural networks; reinforcement learning; object recognition; image classification; image processing; object detection; semantic segmentation; human pose estimation; 3d reconstruction; stereo vision; computational photography; neural networks; image coding; image reconstruction; object recognition; motion estimation.