Self Supervised Video Representation Learning
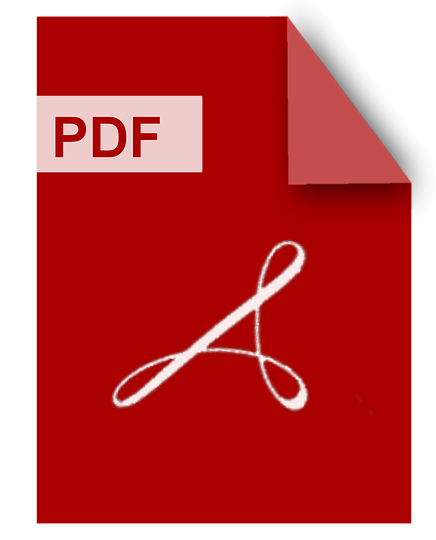
DOWNLOAD
Download Self Supervised Video Representation Learning PDF/ePub or read online books in Mobi eBooks. Click Download or Read Online button to get Self Supervised Video Representation Learning book now. This website allows unlimited access to, at the time of writing, more than 1.5 million titles, including hundreds of thousands of titles in various foreign languages. If the content not found or just blank you must refresh this page
Self Supervised Video Representation Learning
DOWNLOAD
Author : Tengda Han
language : en
Publisher:
Release Date : 2022
Self Supervised Video Representation Learning written by Tengda Han and has been published by this book supported file pdf, txt, epub, kindle and other format this book has been release on 2022 with categories.
Self Supervised Video Representation Learning By Recurrent Networks And Frame Order Predition
DOWNLOAD
Author : Sai Shashidhar Nagabandi
language : en
Publisher:
Release Date : 2020
Self Supervised Video Representation Learning By Recurrent Networks And Frame Order Predition written by Sai Shashidhar Nagabandi and has been published by this book supported file pdf, txt, epub, kindle and other format this book has been release on 2020 with Computer vision categories.
"The success of deep learning models in challenging tasks of computer vision and natural language processing depend on good vector representations of data. For example, learning efficient and salient video representations is one of the fundamental steps for many tasks like action recognition and next frame prediction. Most methods in deep learning rely on large datasets like ImageNet or MSCOCO for training, which is expensive and time consuming to collect. Some of the earlier works in video representation learning relied on encoder-decoder style networks in an unsupervised fashion, which would take in a few frames at a time. Research in the field of self-supervised learning is growing, and has shown promising results on image-related tasks to both learn data representations as well as pre-learn weights for networks using unlabeled data. However, many of these techniques use static architectures like AlexNet, which fail to take into account the temporal aspect of videos. Learning frame-to-frame temporal relationships is essential to learning latent representations of video. In our work, we propose to learn this temporality by pairing static encodings with a recurrent long short term memory network. This research will also investigate applying different methods of encoding architecture along with the recurrent network, to take in a range of different number of frames. We also introduce a novel self-supervised task in which the neural network has two tasks; predicting if a tuple of input frames is temporally consistent, and if not, predict the positioning of incorrect tuple. The efficacy is finally measured by using these trained networks on downstream tasks like action recognition on standard datasets UCF101 and HMDB51."--Abstract.
Learning Video Representation From Self Supervision
DOWNLOAD
Author : Brian Chen
language : en
Publisher:
Release Date : 2023
Learning Video Representation From Self Supervision written by Brian Chen and has been published by this book supported file pdf, txt, epub, kindle and other format this book has been release on 2023 with categories.
This thesis investigates the problem of learning video representations for video understanding. Previous works have explored the use of data-driven deep learning approaches, which have been shown to be effective in learning useful video representations. However, obtaining large amounts of labeled data can be costly and time-consuming. We investigate self-supervised approach as for multimodal video data to overcome this challenge. Video data typically contains multiple modalities, such as visual, audio, transcribed speech, and textual captions, which can serve as pseudo-labels for representation learning without needing manual labeling. By utilizing these modalities, we can train deep representations over large-scale video data consisting of millions of video clips collected from the internet. We demonstrate the scalability benefits of multimodal self-supervision by achieving new state-of-the-art performance in various domains, including video action recognition, text-to-video retrieval, and text-to-video grounding.
Deep Learning For Video Understanding
DOWNLOAD
Author : Zuxuan Wu
language : en
Publisher: Springer Nature
Release Date :
Deep Learning For Video Understanding written by Zuxuan Wu and has been published by Springer Nature this book supported file pdf, txt, epub, kindle and other format this book has been release on with categories.
Self Supervised Face Representation Learning
DOWNLOAD
Author : Vivek Sharma
language : en
Publisher:
Release Date : 2020*
Self Supervised Face Representation Learning written by Vivek Sharma and has been published by this book supported file pdf, txt, epub, kindle and other format this book has been release on 2020* with categories.
Self Supervised Scene Representation Learning
DOWNLOAD
Author : Vincent Simon Sitzmann
language : en
Publisher:
Release Date : 2020
Self Supervised Scene Representation Learning written by Vincent Simon Sitzmann and has been published by this book supported file pdf, txt, epub, kindle and other format this book has been release on 2020 with categories.
Unsupervised learning with generative models has the potential of discovering rich representations of 3D scenes. Such Neural Scene Representations may subsequently support a wide variety of downstream tasks, ranging from robotics to computer graphics to medical imaging. However, existing methods ignore one of the most fundamental properties of scenes: their three-dimensional structure. In this work, we make the case for equipping Neural Scene Representations with an inductive bias for 3D structure. We demonstrate how this inductive bias enables the unsupervised discovery of geometry and appearance, given only posed 2D images. By learning a distribution over a set of such 3D-structure aware neural representations, we can perform joint reconstruction of 3D shape and appearance given only a single 2D observation. We show that the features learned in this process enable 3D semantic segmentation of a whole class of objects, trained with as few as 30 labeled examples, demonstrating a strong link between 3D shape, appearance, and semantic segmentation. Finally, we reflect on the nature and potential role of scene representation learning in computer vision itself, and discuss promising avenues for future work.
Video Efficient Foundation Models
DOWNLOAD
Author : Fida Mohammad Thoker
language : en
Publisher:
Release Date : 2023
Video Efficient Foundation Models written by Fida Mohammad Thoker and has been published by this book supported file pdf, txt, epub, kindle and other format this book has been release on 2023 with categories.
"The thesis strives to endow video -efficiency in video understanding by addressing the research question '' What enables video- efficient video foundation models ?'' Video -efficiency encompasses developing video foundation models that are not only accurate but also exhibit label-efficiency i.e. require fewer labels, domain-efficiency i.e. applicable to a variety of video learning scenarios, and data-efficiency i.e. reduce the amount of video data needed for learning. The research question is addressed for RGB and non-RGB video modalities. In Chapter 2, we focus on improving the label- and domain-efficiency of non-RGB action recognition and detection. Chapter 3 introduces a new self-supervised approach for learning feature representations for 3D-skeleton video sequences. In Chapter 4, we conduct a large-scale study of existing RGB-based self-supervised video models to assess their performance across different facets of video -efficiency. Chapter 5 presents a new method for video self-supervision that explicitly aims to learn motion focused video -representations. To summarize, this thesis presents several novel approaches to improve the video -efficiency of video foundation models . Our research highlights the importance of transferring knowledge between RGB and non-RGB video modalities, exploring self- supervision for non- RGB video modeling, analyzing self-supervised models beyond canonical setups and carefully designing new self-supervised tasks to develop video foundation models that can exhibit different facets of video -efficiency. We hope that our work will inspire further research and development in this area, leading to even more video- efficient foundation models."--
Image Synthesis For Self Supervised Visual Representation Learning
DOWNLOAD
Author : Richard Zhang
language : en
Publisher:
Release Date : 2018
Image Synthesis For Self Supervised Visual Representation Learning written by Richard Zhang and has been published by this book supported file pdf, txt, epub, kindle and other format this book has been release on 2018 with categories.
Deep networks are extremely adept at mapping a noisy, high-dimensional signal to a clean, low-dimensional target output (e.g., image classification). By solving this heavy compression task, the network also learns about natural image priors. However, this process requires the curation of large, labeled datasets. Meanwhile, the world provides massive amounts of raw, unlabeled pixels for free. This thesis investigates learning representations of high-dimensional input signals by mapping them to \textit{high-dimensional} output targets. While more difficult, it is not only possible to learn a strong feature representation, but also to synthesize realistic images. Part I describes the use of deep networks for conditional image synthesis. The section begins by exploring the problem of image colorization, proposing both automatic and user-guided approaches. This section then proposes a system for general image-to-image translation problems, BicycleGAN, with the specific aim of capturing the multimodal nature of the output space. Part II explores the visual representations learned within deep networks. Colorization, as well as cross-channel prediction in general, is a simple but powerful pretext task for self-supervised learning. The representations from cross-channel prediction networks transfer strongly to high-level semantic tasks, such as image classification, and to low-level human perceptual similarity judgments. For the latter, a large-scale dataset of human perceptual similarity judgments is collected. The proposed cross-channel network method outperforms traditional metrics such as PSNR and SSIM. In fact, many unsupervised and self-supervised methods transfer strongly, even comparably to fully-supervised methods.
Self Supervised Video Understanding
DOWNLOAD
Author : Erika Lu
language : en
Publisher:
Release Date : 2021
Self Supervised Video Understanding written by Erika Lu and has been published by this book supported file pdf, txt, epub, kindle and other format this book has been release on 2021 with categories.
Computer Vision Eccv 2022
DOWNLOAD
Author : Shai Avidan
language : en
Publisher: Springer Nature
Release Date : 2022-10-21
Computer Vision Eccv 2022 written by Shai Avidan and has been published by Springer Nature this book supported file pdf, txt, epub, kindle and other format this book has been release on 2022-10-21 with Computers categories.
The 39-volume set, comprising the LNCS books 13661 until 13699, constitutes the refereed proceedings of the 17th European Conference on Computer Vision, ECCV 2022, held in Tel Aviv, Israel, during October 23–27, 2022. The 1645 papers presented in these proceedings were carefully reviewed and selected from a total of 5804 submissions. The papers deal with topics such as computer vision; machine learning; deep neural networks; reinforcement learning; object recognition; image classification; image processing; object detection; semantic segmentation; human pose estimation; 3d reconstruction; stereo vision; computational photography; neural networks; image coding; image reconstruction; object recognition; motion estimation.