Machine Learning Based Methods For Rna Data Analysis
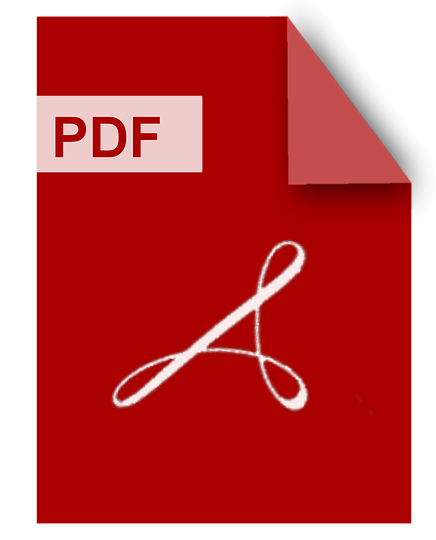
DOWNLOAD
Download Machine Learning Based Methods For Rna Data Analysis PDF/ePub or read online books in Mobi eBooks. Click Download or Read Online button to get Machine Learning Based Methods For Rna Data Analysis book now. This website allows unlimited access to, at the time of writing, more than 1.5 million titles, including hundreds of thousands of titles in various foreign languages. If the content not found or just blank you must refresh this page
Machine Learning Based Methods For Rna Data Analysis
DOWNLOAD
Author : Lihong Peng
language : en
Publisher: Frontiers Media SA
Release Date : 2022-06-16
Machine Learning Based Methods For Rna Data Analysis written by Lihong Peng and has been published by Frontiers Media SA this book supported file pdf, txt, epub, kindle and other format this book has been release on 2022-06-16 with Science categories.
Machine Learning Based Methods For Rna Data Analysis Volume Ii
DOWNLOAD
Author : Lihong Peng
language : en
Publisher: Frontiers Media SA
Release Date : 2023-01-02
Machine Learning Based Methods For Rna Data Analysis Volume Ii written by Lihong Peng and has been published by Frontiers Media SA this book supported file pdf, txt, epub, kindle and other format this book has been release on 2023-01-02 with Science categories.
Machine Learning Based Methods For Rna Data Analysis Volume Iii
DOWNLOAD
Author : Lihong Peng
language : en
Publisher: Frontiers Media SA
Release Date : 2023-02-17
Machine Learning Based Methods For Rna Data Analysis Volume Iii written by Lihong Peng and has been published by Frontiers Media SA this book supported file pdf, txt, epub, kindle and other format this book has been release on 2023-02-17 with Science categories.
Gene Expression Data Analysis
DOWNLOAD
Author : Pankaj Barah
language : en
Publisher: CRC Press
Release Date : 2021-11-21
Gene Expression Data Analysis written by Pankaj Barah and has been published by CRC Press this book supported file pdf, txt, epub, kindle and other format this book has been release on 2021-11-21 with Computers categories.
Development of high-throughput technologies in molecular biology during the last two decades has contributed to the production of tremendous amounts of data. Microarray and RNA sequencing are two such widely used high-throughput technologies for simultaneously monitoring the expression patterns of thousands of genes. Data produced from such experiments are voluminous (both in dimensionality and numbers of instances) and evolving in nature. Analysis of huge amounts of data toward the identification of interesting patterns that are relevant for a given biological question requires high-performance computational infrastructure as well as efficient machine learning algorithms. Cross-communication of ideas between biologists and computer scientists remains a big challenge. Gene Expression Data Analysis: A Statistical and Machine Learning Perspective has been written with a multidisciplinary audience in mind. The book discusses gene expression data analysis from molecular biology, machine learning, and statistical perspectives. Readers will be able to acquire both theoretical and practical knowledge of methods for identifying novel patterns of high biological significance. To measure the effectiveness of such algorithms, we discuss statistical and biological performance metrics that can be used in real life or in a simulated environment. This book discusses a large number of benchmark algorithms, tools, systems, and repositories that are commonly used in analyzing gene expression data and validating results. This book will benefit students, researchers, and practitioners in biology, medicine, and computer science by enabling them to acquire in-depth knowledge in statistical and machine-learning-based methods for analyzing gene expression data. Key Features: An introduction to the Central Dogma of molecular biology and information flow in biological systems A systematic overview of the methods for generating gene expression data Background knowledge on statistical modeling and machine learning techniques Detailed methodology of analyzing gene expression data with an example case study Clustering methods for finding co-expression patterns from microarray, bulkRNA, and scRNA data A large number of practical tools, systems, and repositories that are useful for computational biologists to create, analyze, and validate biologically relevant gene expression patterns Suitable for multidisciplinary researchers and practitioners in computer science and biological sciences
Handbook Of Machine Learning Applications For Genomics
DOWNLOAD
Author : Sanjiban Sekhar Roy
language : en
Publisher: Springer Nature
Release Date : 2022-06-23
Handbook Of Machine Learning Applications For Genomics written by Sanjiban Sekhar Roy and has been published by Springer Nature this book supported file pdf, txt, epub, kindle and other format this book has been release on 2022-06-23 with Technology & Engineering categories.
Currently, machine learning is playing a pivotal role in the progress of genomics. The applications of machine learning are helping all to understand the emerging trends and the future scope of genomics. This book provides comprehensive coverage of machine learning applications such as DNN, CNN, and RNN, for predicting the sequence of DNA and RNA binding proteins, expression of the gene, and splicing control. In addition, the book addresses the effect of multiomics data analysis of cancers using tensor decomposition, machine learning techniques for protein engineering, CNN applications on genomics, challenges of long noncoding RNAs in human disease diagnosis, and how machine learning can be used as a tool to shape the future of medicine. More importantly, it gives a comparative analysis and validates the outcomes of machine learning methods on genomic data to the functional laboratory tests or by formal clinical assessment. The topics of this book will cater interest to academicians, practitioners working in the field of functional genomics, and machine learning. Also, this book shall guide comprehensively the graduate, postgraduates, and Ph.D. scholars working in these fields.
Benchmarking Statistical And Machine Learning Methods For Single Cell Rna Sequencing Data
DOWNLOAD
Author : Nan Xi
language : en
Publisher:
Release Date : 2021
Benchmarking Statistical And Machine Learning Methods For Single Cell Rna Sequencing Data written by Nan Xi and has been published by this book supported file pdf, txt, epub, kindle and other format this book has been release on 2021 with categories.
The large-scale, high-dimensional, and sparse single-cell RNA sequencing (scRNA-seq) data have raised great challenges in the pipeline of data analysis. A large number of statistical and machine learning methods have been developed to analyze scRNA-seq data and answer related scientific questions. Although different methods claim advantages in certain circumstances, it is difficult for users to select appropriate methods for their analysis tasks. Benchmark studies aim to provide recommendations for method selection based on an objective, accurate, and comprehensive comparison among cutting-edge methods. They can also offer suggestions for further methodological development through massive evaluations conducted on real data. In Chapter 2, we conduct the first, systematic benchmark study of nine cutting-edge computational doublet-detection methods. In scRNA-seq, doublets form when two cells are encapsulated into one reaction volume by chance. The existence of doublets, which appear as but are not real cells, is a key confounder in scRNA-seq data analysis. Computational methods have been developed to detect doublets in scRNA-seq data; however, the scRNA-seq field lacks a comprehensive benchmarking of these methods, making it difficult for researchers to choose an appropriate method for their specific analysis needs. Our benchmark study compares doublet-detection methods in terms of their detection accuracy under various experimental settings, impacts on downstream analyses, and computational efficiency. Our results show that existing methods exhibited diverse performance and distinct advantages in different aspects. In Chapter 3, we develop an R package DoubletCollection to integrate the installation and execution of different doublet-detection methods. Traditional benchmark studies can be quickly out-of-date due to their static design and the rapid growth of available methods. DoubletCollection addresses this issue in benchmarking doublet-detection methods for scRNA-seq data. DoubletCollection provides a unified interface to perform and visualize downstream analysis after doublet-detection. Additionally, we created a protocol using DoubletCollection to execute and benchmark doublet-detection methods. This protocol can automatically accommodate new doublet-detection methods in the fast-growing scRNA-seq field. In Chapter 4, we conduct the first comprehensive empirical study to explore the best modeling strategy for autoencoder-based imputation methods specific to scRNA-seq data. The autoencoder-based imputation method is a family of promising methods to denoise sparse scRNA-seq data; however, the design of autoencoders has not been formally discussed in the literature. Current autoencoder-based imputation methods either borrow the practice from other fields or design the model on an ad hoc basis. We find that the method performance is sensitive to the key hyperparameter of autoencoders, including architecture, activation function, and regularization. Their optimal settings on scRNA-seq are largely different from those on other data types. Our results emphasize the importance of exploring hyperparameter space in such complex and flexible methods. Our work also points out the future direction of improving current methods.
Machine Learning Based Sequence Analysis Bioinformatics And Nanopore Transduction Detection
DOWNLOAD
Author : Stephen Winters-Hilt
language : en
Publisher: Lulu.com
Release Date : 2011-05-01
Machine Learning Based Sequence Analysis Bioinformatics And Nanopore Transduction Detection written by Stephen Winters-Hilt and has been published by Lulu.com this book supported file pdf, txt, epub, kindle and other format this book has been release on 2011-05-01 with Computers categories.
This is intended to be a simple and accessible book on machine learning methods and their application in computational genomics and nanopore transduction detection. This book has arisen from eight years of teaching one-semester courses on various machine-learning, cheminformatics, and bioinformatics topics. The book begins with a description of ad hoc signal acquisition methods and how to orient on signal processing problems with the standard tools from information theory and signal analysis. A general stochastic sequential analysis (SSA) signal processing architecture is then described that implements Hidden Markov Model (HMM) methods. Methods are then shown for classification and clustering using generalized Support Vector Machines, for use with the SSA Protocol, or independent of that approach. Optimization metaheuristics are used for tuning over algorithmic parameters throughout. Hardware implementations and short code examples of the various methods are also described.
Machine Learning Methods For Single Cell Rna Sequencing Data Analysis
DOWNLOAD
Author : Chuanqi Wang
language : en
Publisher:
Release Date : 2021
Machine Learning Methods For Single Cell Rna Sequencing Data Analysis written by Chuanqi Wang and has been published by this book supported file pdf, txt, epub, kindle and other format this book has been release on 2021 with categories.
Machine Learning Strategies For Alternative Splicing
DOWNLOAD
Author : Zhicheng Pan
language : en
Publisher:
Release Date : 2021
Machine Learning Strategies For Alternative Splicing written by Zhicheng Pan and has been published by this book supported file pdf, txt, epub, kindle and other format this book has been release on 2021 with categories.
Alternative splicing (AS) is a fundamental biological process that diversifies the transcriptomes and proteomes. Aberrant splicing is the main cause of rare diseases and cancers. Our understanding of AS is far from complete, resulting in a limited comprehension of phenotypic effects of splicing dysregulation. Recent advances in next-generation sequencing (NGS) technologies have revolutionized the discoveries of AS. There are considerable efforts put into generating a large compendium of RNA-seq datasets. These datasets offer an opportunity to study the regulation of AS in tissues, cell stages, and perturbation of biological conditions at unprecedented resolutions and scales. However, utilizing the large number of datasets to make biological discoveries remains a challenge. In this dissertation, we developed machine-learning-based strategies to integrate various types of RNA-seq datasets and transform them into biological knowledge, thereby enabling discoveries towards regulatory mechanisms and functional consequences of AS. In the first part of the dissertation, we report a deep-learning-based computational framework, Deep-learning Augmented RNA-seq analysis of Transcript Splicing (DARTS), that utilizes the Bayesian integration of deep-learning-based predictions with empirical RNA datasets to make inference of differential alternative splicing between biological samples. RNA sequencing (RNA-seq) analysis of alternative splicing is largely limited by depending on high sequencing coverage. DARTS transforms large amounts of publicly available RNA-seq datasets into biological knowledge of how splicing is regulated through deep learning, thus enabling researchers to better characterize alternative splicing inaccessible from RNA-seq datasets with modest coverage. In the second part of the dissertation, we present a computational tool, Systematic Investigation of Retained Introns (SIRI), to quantify unspliced introns and describe a deep-learning-based computational framework to investigate the sequence preferences of different intron groups across subcellular locations. Steps of mRNA maturation occur in distinct cellular locations, while subcellular distribution of processed and unprocessed transcripts often miss in transcriptomic analyses. We employed SIRI to measure intron levels in subcellular locations across cell development and identified four intron groups that have disparate patterns of RNA enrichment across subcellular locations. Through the deep-learning based framework, we identified a set of triplet motifs and sequence conservation patterns that are predictive of intron behavior among biological conditions. In the third part of the dissertation, we exhibit a deep-learning-based tissue-specific framework, individualized Deep-learning Analysis of RNA Transcript Splicing (iDARTS), for predicting splicing levels. The rapid accumulation of RNA-seq datasets matched with whole exome or genome sequencing yields enormous variants underlying diseases, traits, and cancer. Interpreting the functional consequences of these variants remains a challenge in disease diagnostics and precision medicine. iDARTS leverages the publicly available RNA-seq datasets to model the cis RNA sequence features and trans RNA binding protein levels determinants of AS, allowing precise predictions of genetic splice-altering variants. We demonstrated that predicted splice-altering variants are functionally relevant and related to cancer development when analysing ~10 million intronic and exonic variants with iDARTS. Applying iDARTS to interpret functional consequences of variants of uncertain significance in clinical studies, we found that predicted splice-altering variants are ten times enriched in pathogenic categories over benign categories. Our results indicate that iDARTS will benefit large-scale screening disease-implicated variants, thus improving disease diagnosis and enabling discoveries for precision medicine. In the fourth part of the dissertation, we study the underlying mechanisms of N6-methyladenosine (m6A) RNA modification by investigating the biological consequences of arginine methylation of METTL14 through transcriptome-wide profiling of m6A. Arginine methylation of METTL14 controls m6A deposition in mammalian cells. Mouse embryonic stem cells (mESCs) expressing arginine methylation-deficient METTL14 exhibit significantly reduced global m6A levels. These arginine methylation-dependent m6A sites identified from transcriptome-wide analysis are associated with enhanced translation of genes essential for the repair of DNA interstrand crosslinks. Collectively, these findings reveal important aspects of m6A regulation and new functions of arginine methylation in RNA metabolism.
Machine Learning Advanced Dynamic Omics Data Analysis For Precision Medicine
DOWNLOAD
Author : Tao Zeng
language : en
Publisher: Frontiers Media SA
Release Date : 2020-03-30
Machine Learning Advanced Dynamic Omics Data Analysis For Precision Medicine written by Tao Zeng and has been published by Frontiers Media SA this book supported file pdf, txt, epub, kindle and other format this book has been release on 2020-03-30 with categories.