Nonparametric Estimation Of Large Spot Volatility Matrices For High Frequency Financial Data
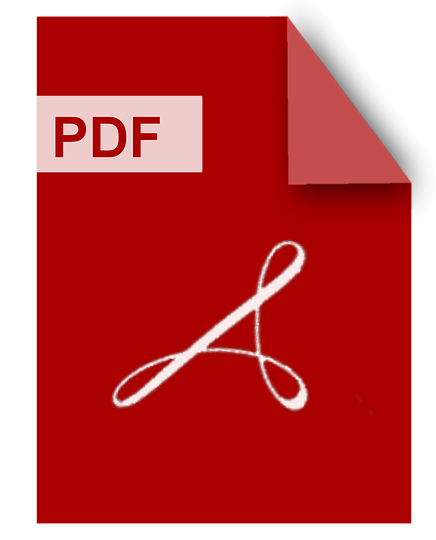
DOWNLOAD
Download Nonparametric Estimation Of Large Spot Volatility Matrices For High Frequency Financial Data PDF/ePub or read online books in Mobi eBooks. Click Download or Read Online button to get Nonparametric Estimation Of Large Spot Volatility Matrices For High Frequency Financial Data book now. This website allows unlimited access to, at the time of writing, more than 1.5 million titles, including hundreds of thousands of titles in various foreign languages. If the content not found or just blank you must refresh this page
Nonparametric Estimation Of Large Spot Volatility Matrices For High Frequency Financial Data
DOWNLOAD
Author : Ruijun Bu
language : en
Publisher:
Release Date : 2022
Nonparametric Estimation Of Large Spot Volatility Matrices For High Frequency Financial Data written by Ruijun Bu and has been published by this book supported file pdf, txt, epub, kindle and other format this book has been release on 2022 with categories.
Large Volatility Matrix Inference Based On High Frequency Financial Data
DOWNLOAD
Author :
language : en
Publisher:
Release Date : 2013
Large Volatility Matrix Inference Based On High Frequency Financial Data written by and has been published by this book supported file pdf, txt, epub, kindle and other format this book has been release on 2013 with categories.
Financial practices often need to estimate an integrated volatility matrix of a large number of assets using noisy high-frequency financial data. This estimation problem is a challenging one for four reasons: (1) high-frequency financial data are discrete observations of the underlying assets' price processes; (2) due to market micro-structure noise, high-frequency data are observed with measurement errors; (3) different assets are traded at different time points, which is the so-called non-synchronization phenomenon in high-frequency financial data; (4) the number of assets may be comparable to or even exceed the observations, and hence many existing estimators of small size volatility matrices become inconsistent when the size of the matrix is close to or larger than the sample size. In this dissertation, we focus on large volatility matrix inference for high-frequency financial data, which can be summarized in three aspects. On the methodological aspect, we propose a new threshold MSRVM estimator of large volatility matrix. This estimator can deal with all the four challenges, and is consistent when both sample size and matrix size go to infinity. On the theoretical aspect, we study the optimal convergence rate for the volatility matrix estimation, by building the asymptotic theory for the proposed estimator and deriving a minimax lower bound for this estimation problem. The proposed threshold MSRVM estimator has a risk matching with the lower bound up to a constant factor, and hence it achieves an optimal convergence rate. As for the applications, we develop a novel approach to predict the volatility matrix. The approach extends the applicability of classical low-frequency models such as matrix factor models and vector autoregressive models to the high-frequency data. With this approach, we pool together the strengths of both classical low-frequency models and new high-frequency estimation methodologies. Furthermore, numerical studies are conducted to test the finite sample performance of the proposed estimators, to support the established asymptotic theories.
Structured Volatility Matrix Estimation For Non Synchronized High Frequency Financial Data
DOWNLOAD
Author : Jianqing Fan
language : en
Publisher:
Release Date : 2017
Structured Volatility Matrix Estimation For Non Synchronized High Frequency Financial Data written by Jianqing Fan and has been published by this book supported file pdf, txt, epub, kindle and other format this book has been release on 2017 with categories.
Recently several large volatility matrix estimation procedures have been developed for factor-based Ito processes whose integrated volatility matrix consists of low-rank and sparse matrices. Their performance depends on the accuracy of input volatility matrix estimators. When estimating co-volatilities based on high-frequency data, one of the crucial challenges is non-synchronization for illiquid assets, which makes their co-volatility estimators inaccurate. In this paper, we study how to estimate the large integrated volatility matrix without using co-volatilities of illiquid assets. Specifically, we pretend that the co-volatilities for illiquid assets are missing, and estimate the low-rank matrix using a matrix completion scheme with a structured missing pattern. To further regularize the sparse volatility matrix, we employ the principal orthogonal complement thresholding method (POET). We also investigate the asymptotic properties of the proposed estimation procedure and demonstrate its advantages over using co-volatilities of illiquid assets. The advantages of our methods are also verified by an extensive simulation study and illustrated by high-frequency financial data for constituents of the S&P 500 index.
Robust High Dimensional Volatility Matrix Estimation For High Frequency Factor Model
DOWNLOAD
Author : Jianqing Fan
language : en
Publisher:
Release Date : 2017
Robust High Dimensional Volatility Matrix Estimation For High Frequency Factor Model written by Jianqing Fan and has been published by this book supported file pdf, txt, epub, kindle and other format this book has been release on 2017 with categories.
High-frequency financial data allow us to estimate large volatility matrices with relatively short time horizon. Many novel statistical methods have been introduced to address large volatility matrix estimation problems from a high-dimensional Ito process with microstructural noise contamination. Their asymptotic theories require sub-Gaussian or some finite high-order moments assumptions for observed log-returns. These assumptions are at odd with the heavy tail phenomenon that is pandemic in financial stock returns and new procedures are needed to mitigate the influence of heavy tails. In this paper, we introduce the Huber loss function with a diverging threshold to develop a robust realized volatility estimation. We show that it has the sub-Gaussian concentration around the volatility with only finite fourth moments of observed log-returns. With the proposed robust estimator as input, we further regularize it by using the principal orthogonal component thresholding (POET) procedure to estimate the large volatility matrix that admits an approximate factor structure. We establish the asymptotic theories for such low-rank plus sparse matrices. The simulation study is conducted to check the finite sample performance of the proposed estimation methods.
Factor Garch Ito Models For High Frequency Data With Application To Large Volatility Matrix Prediction
DOWNLOAD
Author : Donggyu Kim
language : en
Publisher:
Release Date : 2017
Factor Garch Ito Models For High Frequency Data With Application To Large Volatility Matrix Prediction written by Donggyu Kim and has been published by this book supported file pdf, txt, epub, kindle and other format this book has been release on 2017 with categories.
Several novel large volatility matrix estimation methods have been developed based on the high-frequency financial data. They often employ the approximate factor model that leads to a low-rank plus sparse structure for the integrated volatility matrix and facilitates estimation of large volatility matrices. However, for predicting future volatility matrices, these nonparametric estimators do not have a dynamic structure to implement. In this paper, we introduce a novel Ito diffusion process based on the approximate factor models and call it a factor GARCH-Ito model. We then investigate its properties and propose a quasi-maximum likelihood estimation method for the parameter of the factor GARCH-Ito model. We also apply it to estimating conditional expected large volatility matrices and establish their asymptotic properties. Simulation studies are conducted to validate the finite sample performance of the proposed estimation methods. The proposed method is also illustrated by using data from the constituents of the S&P 500 index and an application to constructing the minimum variance portfolio with gross exposure constraints.
A Comparative Study On Large Multivariate Volatility Matrix Modeling For High Frequency Financial Data
DOWNLOAD
Author : Dongchen Jiang
language : en
Publisher:
Release Date : 2015
A Comparative Study On Large Multivariate Volatility Matrix Modeling For High Frequency Financial Data written by Dongchen Jiang and has been published by this book supported file pdf, txt, epub, kindle and other format this book has been release on 2015 with categories.
Abstract: Modeling and forecasting the volatilities of high-frequency data observed on the prices of financial assets are vibrant research areas in econometrics and statistics. However, most of the available methods are not directly applicable when the number of assets involved is large, due to the lack of accuracy in estimating high-dimensional matrices. This paper compared two methodologies of vast volatility matrix estimation for high-frequency data. One is to estimate the Average Realized Volatility Matrix and to regularize it by banding and thresholding. In this method, first we select grids as pre-sampling frequencies, construct a realized volatility matrix using previous tick method according to each pre-sampling frequency and then take the average of the constructed realized volatility matrices as the stage one estimator, which we call the ARVM estimator. Then we regularize the ARVM estimator to yield good consistent estimators of the large integrated volatility matrix. We consider two regularizations: thresholding and banding. The other is Dynamic Conditional Correlation (DCC) which can be estimated for two stage, where in the rst stage univariate GARCH models are estimated for each residual series, and in the second stage, the residuals are used to estimate the parameters of the dynamic correlation. Asymptotic theory for the two proposed methodologies shows that the estimator are consistent. In numerical studies, the proposed two methodologies are applied to simulated data set and real high-frequency prices from top 100 S & P 500 stocks according to the trading volume over a period of 3 months, 64 trading days in 2013. From the perfomances of estimators, the conclusion is that TARVM estimator performs better than DCC volatility matrix. And its largest eigenvalues are more stable than those of DCC model so that it is more approriable in eigen-based anaylsis.
Volatility Estimation With Financial Data
DOWNLOAD
Author :
language : en
Publisher:
Release Date : 2015
Volatility Estimation With Financial Data written by and has been published by this book supported file pdf, txt, epub, kindle and other format this book has been release on 2015 with categories.
Modeling and estimating volatility plays a crucial role in financial practice. Devoted efforts are made to investigate this topic using both low-frequency and high-frequency financial data. Traditionally, volatility modeling and analysis are based on either historical price data or option data. Finance theory shows that option prices heavily depend on the underlying stocks' prices, and thus the two kinds of data are related. This thesis explores the approach that combines both stock price data and option data to perform the statistical analysis of volatility. We investigate the Black-Scholes model and an exponential GARCH model and derive the relationship among the Fisher information for volatility estimation based on stock price data alone or option data alone as well as joint volatility estimation for combining stock price data and option data. Under the Block-Scholes model, asymptotic theory for the joint estimation is established, and a simulation study was conducted to check finite sample performances of the proposed joint estimator. Being more accessible than ever, high-frequency data have provided researchers and practitioners with incredible tools to investigate assets pricing and market dynamics. Non-synchronous observations, microstructure noise, and complex pricing models are challenges coming along with high-frequency data. Moreover, large volatility matrix estimation is involved in many finance practices and encounters "curse of dimensionality". Although it is widely used in large covariance estimation, imposing sparsity assumption on the entire volatility matrix is not reasonable in financial practice. In fact, due to the existence of common factors, assets are widely correlated with each other and their volatility matrix is not sparse. In this thesis, we focus on incorporating the factor influence in asset price modeling and volatility matrix estimation. We propose to model asset price using a factor-based diffusion process. The idea is that assets' prices are governed by a common factor, and that assets with similar characteristics share the same association with the factor. Under the proposed factor-based model, we developed an estimation scheme called "Blocking and Regularizing", which deals with all of the four changeless. The asymptotic properties of the proposed estimator are studied, while its finite sample performance is tested via extensive numerical studies to support theoretical results.
High Frequency Financial Econometrics
DOWNLOAD
Author : Yacine Aït-Sahalia
language : en
Publisher: Princeton University Press
Release Date : 2014-07-21
High Frequency Financial Econometrics written by Yacine Aït-Sahalia and has been published by Princeton University Press this book supported file pdf, txt, epub, kindle and other format this book has been release on 2014-07-21 with Business & Economics categories.
A comprehensive introduction to the statistical and econometric methods for analyzing high-frequency financial data High-frequency trading is an algorithm-based computerized trading practice that allows firms to trade stocks in milliseconds. Over the last fifteen years, the use of statistical and econometric methods for analyzing high-frequency financial data has grown exponentially. This growth has been driven by the increasing availability of such data, the technological advancements that make high-frequency trading strategies possible, and the need of practitioners to analyze these data. This comprehensive book introduces readers to these emerging methods and tools of analysis. Yacine Aït-Sahalia and Jean Jacod cover the mathematical foundations of stochastic processes, describe the primary characteristics of high-frequency financial data, and present the asymptotic concepts that their analysis relies on. Aït-Sahalia and Jacod also deal with estimation of the volatility portion of the model, including methods that are robust to market microstructure noise, and address estimation and testing questions involving the jump part of the model. As they demonstrate, the practical importance and relevance of jumps in financial data are universally recognized, but only recently have econometric methods become available to rigorously analyze jump processes. Aït-Sahalia and Jacod approach high-frequency econometrics with a distinct focus on the financial side of matters while maintaining technical rigor, which makes this book invaluable to researchers and practitioners alike.
Statistical Inferences And Computing For Diffusion Models In Finance
DOWNLOAD
Author : Hai Xu
language : en
Publisher:
Release Date : 2006
Statistical Inferences And Computing For Diffusion Models In Finance written by Hai Xu and has been published by this book supported file pdf, txt, epub, kindle and other format this book has been release on 2006 with categories.
Quantitative Trading
DOWNLOAD
Author : Xin Guo
language : en
Publisher: CRC Press
Release Date : 2017-01-06
Quantitative Trading written by Xin Guo and has been published by CRC Press this book supported file pdf, txt, epub, kindle and other format this book has been release on 2017-01-06 with Business & Economics categories.
The first part of this book discusses institutions and mechanisms of algorithmic trading, market microstructure, high-frequency data and stylized facts, time and event aggregation, order book dynamics, trading strategies and algorithms, transaction costs, market impact and execution strategies, risk analysis, and management. The second part covers market impact models, network models, multi-asset trading, machine learning techniques, and nonlinear filtering. The third part discusses electronic market making, liquidity, systemic risk, recent developments and debates on the subject.