On Complexity Certification Of Active Set Qp Methods With Applications To Linear Mpc
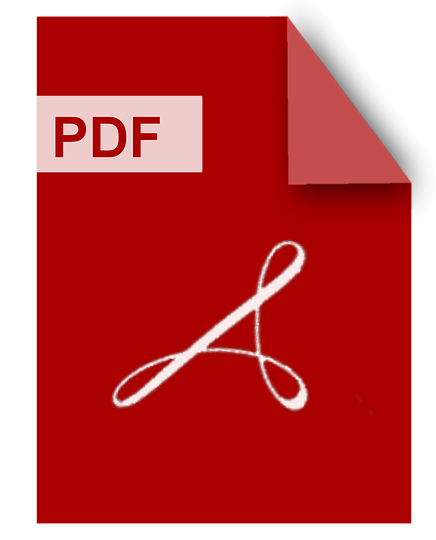
DOWNLOAD
Download On Complexity Certification Of Active Set Qp Methods With Applications To Linear Mpc PDF/ePub or read online books in Mobi eBooks. Click Download or Read Online button to get On Complexity Certification Of Active Set Qp Methods With Applications To Linear Mpc book now. This website allows unlimited access to, at the time of writing, more than 1.5 million titles, including hundreds of thousands of titles in various foreign languages. If the content not found or just blank you must refresh this page
On Complexity Certification Of Active Set Qp Methods With Applications To Linear Mpc
DOWNLOAD
Author : Daniel Arnström
language : en
Publisher: Linköping University Electronic Press
Release Date : 2021-03-03
On Complexity Certification Of Active Set Qp Methods With Applications To Linear Mpc written by Daniel Arnström and has been published by Linköping University Electronic Press this book supported file pdf, txt, epub, kindle and other format this book has been release on 2021-03-03 with categories.
In model predictive control (MPC) an optimization problem has to be solved at each time step, which in real-time applications makes it important to solve these efficiently and to have good upper bounds on worst-case solution time. Often for linear MPC problems, the optimization problem in question is a quadratic program (QP) that depends on parameters such as system states and reference signals. A popular class of methods for solving such QPs is active-set methods, where a sequence of linear systems of equations is solved. The primary contribution of this thesis is a method which determines which sequence of subproblems a popular class of such active-set algorithms need to solve, for every possible QP instance that might arise from a given linear MPC problem (i.e, for every possible state and reference signal). By knowing these sequences, worst-case bounds on how many iterations, floating-point operations and, ultimately, the maximum solution time, these active-set algorithms require to compute a solution can be determined, which is of importance when, e.g, linear MPC is used in safety-critical applications. After establishing this complexity certification method, its applicability is extended by showing how it can be used indirectly to certify the complexity of another, efficient, type of active-set QP algorithm which reformulates the QP as a nonnegative least-squares method. Finally, the proposed complexity certification method is extended further to situations when enhancements to the active-set algorithms are used, namely, when they are terminated early (to save computations) and when outer proximal-point iterations are performed (to improve numerical stability).
Nonlinear Model Predictive Control
DOWNLOAD
Author : Frank Allgöwer
language : en
Publisher: Birkhäuser
Release Date : 2012-12-06
Nonlinear Model Predictive Control written by Frank Allgöwer and has been published by Birkhäuser this book supported file pdf, txt, epub, kindle and other format this book has been release on 2012-12-06 with Mathematics categories.
During the past decade model predictive control (MPC), also referred to as receding horizon control or moving horizon control, has become the preferred control strategy for quite a number of industrial processes. There have been many significant advances in this area over the past years, one of the most important ones being its extension to nonlinear systems. This book gives an up-to-date assessment of the current state of the art in the new field of nonlinear model predictive control (NMPC). The main topic areas that appear to be of central importance for NMPC are covered, namely receding horizon control theory, modeling for NMPC, computational aspects of on-line optimization and application issues. The book consists of selected papers presented at the International Symposium on Nonlinear Model Predictive Control – Assessment and Future Directions, which took place from June 3 to 5, 1998, in Ascona, Switzerland. The book is geared towards researchers and practitioners in the area of control engineering and control theory. It is also suited for postgraduate students as the book contains several overview articles that give a tutorial introduction into the various aspects of nonlinear model predictive control, including systems theory, computations, modeling and applications.
Dgor Nsor
DOWNLOAD
Author : Helmut Schellhaas
language : de
Publisher: Springer-Verlag
Release Date : 2013-03-13
Dgor Nsor written by Helmut Schellhaas and has been published by Springer-Verlag this book supported file pdf, txt, epub, kindle and other format this book has been release on 2013-03-13 with Business & Economics categories.
Dieser Band gibt eine vollständige Übersicht über die Vorträge, die auf der 16. Jahrestagung der Deutschen Gesellschaft für Operations Research (DGOR) zusammen mit der Nederlandse Stichting voor Operations Research (NSOR) in der Zeit vom 23.-25. September 1987 im Kongreßzentrum Königshof in Veldhoven bei Eindhoven gehalten wurden. Die Proceedings informieren über 166 Fachvorträge und 5 Plenarvorträge. Während sämtliche Plenarvorträge in ausführlicher Fassung enthalten sind, wurden 50 Fachvorträge in Langfassung, die restlichen in Kurzfassung aufgenommen.
Encyclopedia Of Systems And Control
DOWNLOAD
Author : John Baillieul
language : en
Publisher: Springer
Release Date : 2015-07-29
Encyclopedia Of Systems And Control written by John Baillieul and has been published by Springer this book supported file pdf, txt, epub, kindle and other format this book has been release on 2015-07-29 with Technology & Engineering categories.
The Encyclopedia of Systems and Control collects a broad range of short expository articles that describe the current state of the art in the central topics of control and systems engineering as well as in many of the related fields in which control is an enabling technology. The editors have assembled the most comprehensive reference possible, and this has been greatly facilitated by the publisher’s commitment continuously to publish updates to the articles as they become available in the future. Although control engineering is now a mature discipline, it remains an area in which there is a great deal of research activity, and as new developments in both theory and applications become available, they will be included in the online version of the encyclopedia. A carefully chosen team of leading authorities in the field has written the well over 250 articles that comprise the work. The topics range from basic principles of feedback in servomechanisms to advanced topics such as the control of Boolean networks and evolutionary game theory. Because the content has been selected to reflect both foundational importance as well as subjects that are of current interest to the research and practitioner communities, a broad readership that includes students, application engineers, and research scientists will find material that is of interest.
Predictive Control For Linear And Hybrid Systems
DOWNLOAD
Author : Francesco Borrelli
language : en
Publisher: Cambridge University Press
Release Date : 2017-06-22
Predictive Control For Linear And Hybrid Systems written by Francesco Borrelli and has been published by Cambridge University Press this book supported file pdf, txt, epub, kindle and other format this book has been release on 2017-06-22 with Mathematics categories.
With a simple approach that includes real-time applications and algorithms, this book covers the theory of model predictive control (MPC).
Minimax Approaches To Robust Model Predictive Control
DOWNLOAD
Author : Johan Löfberg
language : en
Publisher: Linköping University Electronic Press
Release Date : 2003-04-11
Minimax Approaches To Robust Model Predictive Control written by Johan Löfberg and has been published by Linköping University Electronic Press this book supported file pdf, txt, epub, kindle and other format this book has been release on 2003-04-11 with Predictive control categories.
Controlling a system with control and state constraints is one of the most important problems in control theory, but also one of the most challenging. Another important but just as demanding topic is robustness against uncertainties in a controlled system. One of the most successful approaches, both in theory and practice, to control constrained systems is model predictive control (MPC). The basic idea in MPC is to repeatedly solve optimization problems on-line to find an optimal input to the controlled system. In recent years, much effort has been spent to incorporate the robustness problem into this framework. The main part of the thesis revolves around minimax formulations of MPC for uncertain constrained linear discrete-time systems. A minimax strategy in MPC means that worst-case performance with respect to uncertainties is optimized. Unfortunately, many minimax MPC formulations yield intractable optimization problems with exponential complexity. Minimax algorithms for a number of uncertainty models are derived in the thesis. These include systems with bounded external additive disturbances, systems with uncertain gain, and systems described with linear fractional transformations. The central theme in the different algorithms is semidefinite relaxations. This means that the minimax problems are written as uncertain semidefinite programs, and then conservatively approximated using robust optimization theory. The result is an optimization problem with polynomial complexity. The use of semidefinite relaxations enables a framework that allows extensions of the basic algorithms, such as joint minimax control and estimation, and approx- imation of closed-loop minimax MPC using a convex programming framework. Additional topics include development of an efficient optimization algorithm to solve the resulting semidefinite programs and connections between deterministic minimax MPC and stochastic risk-sensitive control. The remaining part of the thesis is devoted to stability issues in MPC for continuous-time nonlinear unconstrained systems. While stability of MPC for un-constrained linear systems essentially is solved with the linear quadratic controller, no such simple solution exists in the nonlinear case. It is shown how tools from modern nonlinear control theory can be used to synthesize finite horizon MPC controllers with guaranteed stability, and more importantly, how some of the tech- nical assumptions in the literature can be dispensed with by using a slightly more complex controller.
Uncertainties In Neural Networks
DOWNLOAD
Author : Magnus Malmström
language : en
Publisher: Linköping University Electronic Press
Release Date : 2021-04-06
Uncertainties In Neural Networks written by Magnus Malmström and has been published by Linköping University Electronic Press this book supported file pdf, txt, epub, kindle and other format this book has been release on 2021-04-06 with categories.
In science, technology, and engineering, creating models of the environment to predict future events has always been a key component. The models could be everything from how the friction of a tire depends on the wheels slip to how a pathogen is spread throughout society. As more data becomes available, the use of data-driven black-box models becomes more attractive. In many areas they have shown promising results, but for them to be used widespread in safety-critical applications such as autonomous driving some notion of uncertainty in the prediction is required. An example of such a black-box model is neural networks (NNs). This thesis aims to increase the usefulness of NNs by presenting an method where uncertainty in the prediction is obtained by linearization of the model. In system identification and sensor fusion, under the condition that the model structure is identifiable, this is a commonly used approach to get uncertainty in the prediction from a nonlinear model. If the model structure is not identifiable, such as for NNs, the ambiguities that cause this have to be taken care of in order to make the approach applicable. This is handled in the first part of the thesis where NNs are analyzed from a system identification perspective, and sources of uncertainty are discussed. Another problem with data-driven black-box models is that it is difficult to know how flexible the model needs to be in order to correctly model the true system. One solution to this problem is to use a model that is more flexible than necessary to make sure that the model is flexible enough. But how would that extra flexibility affect the uncertainty in the prediction? This is handled in the later part of the thesis where it is shown that the uncertainty in the prediction is bounded from below by the uncertainty in the prediction of the model with lowest flexibility required for representing true system accurately. In the literature, many other approaches to handle the uncertainty in predictions by NNs have been suggested, of which some are summarized in this work. Furthermore, a simulation and an experimental studies inspired by autonomous driving are conducted. In the simulation study, different sources of uncertainty are investigated, as well as how large the uncertainty in the predictions by NNs are in areas without training data. In the experimental study, the uncertainty in predictions done by different models are investigated. The results show that, compared to existing methods, the linearization method produces similar results for the uncertainty in predictions by NNs. An introduction video is available at https://youtu.be/O4ZcUTGXFN0 Inom forskning och utveckling har det har alltid varit centralt att skapa modeller av verkligheten. Dessa modeller har bland annat använts till att förutspå framtida händelser eller för att styra ett system till att bete sig som man önskar. Modellerna kan beskriva allt från hur friktionen hos ett bildäck påverkas av hur mycket hjulen glider till hur ett virus kan sprida sig i ett samhälle. I takt med att mer och mer data blir tillgänglig ökar potentialen för datadrivna black-box modeller. Dessa modeller är universella approximationer vilka ska kunna representera vilken godtycklig funktion som helst. Användningen av dessa modeller har haft stor framgång inom många områden men för att verkligen kunna etablera sig inom säkerhetskritiska områden såsom självkörande farkoster behövs en förståelse för osäkerhet i prediktionen från modellen. Neuronnät är ett exempel på en sådan black-box modell. I denna avhandling kommer olika sätt att tillförskaffa sig kunskap om osäkerhet i prediktionen av neuronnät undersökas. En metod som bygger på linjärisering av modellen för att tillförskaffa sig osäkerhet i prediktionen av neuronnätet kommer att presenteras. Denna metod är välbeprövad inom systemidentifiering och sensorfusion under antagandet att modellen är identifierbar. För modeller såsom neuronnät, vilka inte är identifierbara behövs det att det tas hänsyn till tvetydigheterna i modellen. En annan utmaning med datadrivna black-box modeller, är att veta om den valda modellmängden är tillräckligt generell för att kunna modellera det sanna systemet. En lösning på detta problem är att använda modeller som har mer flexibilitet än vad som behövs, det vill säga en överparameteriserad modell. Men hur påverkas osäkerheten i prediktionen av detta? Detta är något som undersöks i denna avhandling, vilken visar att osäkerheten i den överparameteriserad modellen kommer att vara begränsad underifrån av modellen med minst flexibilitet som ändå är tillräckligt generell för att modellera det sanna systemet. Som avslutning kommer dessa resultat att demonstreras i både en simuleringsstudie och en experimentstudie inspirerad av självkörande farkoster. Fokuset i simuleringsstudien är hur osäkerheten hos modellen är i områden med och utan tillgång till träningsdata medan experimentstudien fokuserar på jämförelsen mellan osäkerheten i olika typer av modeller.Resultaten från dessa studier visar att metoden som bygger på linjärisering ger liknande resultat för skattningen av osäkerheten i prediktionen av neuronnät, jämfört med existerande metoder.
Handbook Of Model Predictive Control
DOWNLOAD
Author : Saša V. Raković
language : en
Publisher: Springer
Release Date : 2018-09-01
Handbook Of Model Predictive Control written by Saša V. Raković and has been published by Springer this book supported file pdf, txt, epub, kindle and other format this book has been release on 2018-09-01 with Science categories.
Recent developments in model-predictive control promise remarkable opportunities for designing multi-input, multi-output control systems and improving the control of single-input, single-output systems. This volume provides a definitive survey of the latest model-predictive control methods available to engineers and scientists today. The initial set of chapters present various methods for managing uncertainty in systems, including stochastic model-predictive control. With the advent of affordable and fast computation, control engineers now need to think about using “computationally intensive controls,” so the second part of this book addresses the solution of optimization problems in “real” time for model-predictive control. The theory and applications of control theory often influence each other, so the last section of Handbook of Model Predictive Control rounds out the book with representative applications to automobiles, healthcare, robotics, and finance. The chapters in this volume will be useful to working engineers, scientists, and mathematicians, as well as students and faculty interested in the progression of control theory. Future developments in MPC will no doubt build from concepts demonstrated in this book and anyone with an interest in MPC will find fruitful information and suggestions for additional reading.
Practical Methods For Optimal Control And Estimation Using Nonlinear Programming
DOWNLOAD
Author : John T. Betts
language : en
Publisher: SIAM
Release Date : 2010-01-01
Practical Methods For Optimal Control And Estimation Using Nonlinear Programming written by John T. Betts and has been published by SIAM this book supported file pdf, txt, epub, kindle and other format this book has been release on 2010-01-01 with Mathematics categories.
A focused presentation of how sparse optimization methods can be used to solve optimal control and estimation problems.
Fighter Aircraft Maneuver Limiting Using Mpc Theory And Application
DOWNLOAD
Author : Daniel Simon
language : en
Publisher: Linköping University Electronic Press
Release Date : 2017-09-12
Fighter Aircraft Maneuver Limiting Using Mpc Theory And Application written by Daniel Simon and has been published by Linköping University Electronic Press this book supported file pdf, txt, epub, kindle and other format this book has been release on 2017-09-12 with categories.
Flight control design for modern fighter aircraft is a challenging task. Aircraft are dynamical systems, which naturally contain a variety of constraints and nonlinearities such as, e.g., maximum permissible load factor, angle of attack and control surface deflections. Taking these limitations into account in the design of control systems is becoming increasingly important as the performance and complexity of the aircraft is constantly increasing. The aeronautical industry has traditionally applied feedforward, anti-windup or similar techniques and different ad hoc engineering solutions to handle constraints on the aircraft. However these approaches often rely on engineering experience and insight rather than a theoretical foundation, and can often require a tremendous amount of time to tune. In this thesis we investigate model predictive control as an alternative design tool to handle the constraints that arises in the flight control design. We derive a simple reference tracking MPC algorithm for linear systems that build on the dual mode formulation with guaranteed stability and low complexity suitable for implementation in real time safety critical systems. To reduce the computational burden of nonlinear model predictive control we propose a method to handle the nonlinear constraints, using a set of dynamically generated local inner polytopic approximations. The main benefit of the proposed method is that while computationally cheap it still can guarantee recursive feasibility and convergence. An alternative to deriving MPC algorithms with guaranteed stability properties is to analyze the closed loop stability, post design. Here we focus on deriving a tool based on Mixed Integer Linear Programming for analysis of the closed loop stability and robust stability of linear systems controlled with MPC controllers. To test the performance of model predictive control for a real world example we design and implement a standard MPC controller in the development simulator for the JAS 39 Gripen aircraft at Saab Aeronautics. This part of the thesis focuses on practical and tuning aspects of designing MPC controllers for fighter aircraft. Finally we have compared the MPC design with an alternative approach to maneuver limiting using a command governor.