Oracle Inequalities In Empirical Risk Minimization And Sparse Recovery Problems
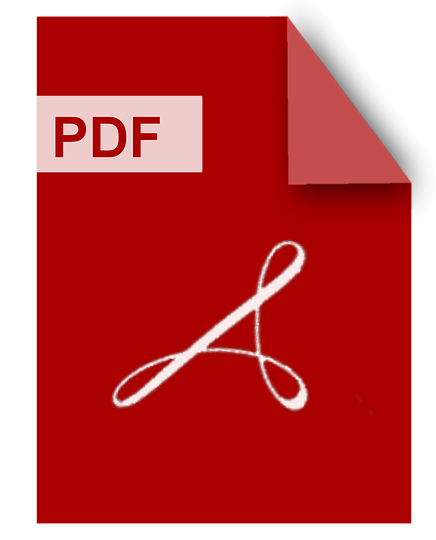
DOWNLOAD
Download Oracle Inequalities In Empirical Risk Minimization And Sparse Recovery Problems PDF/ePub or read online books in Mobi eBooks. Click Download or Read Online button to get Oracle Inequalities In Empirical Risk Minimization And Sparse Recovery Problems book now. This website allows unlimited access to, at the time of writing, more than 1.5 million titles, including hundreds of thousands of titles in various foreign languages. If the content not found or just blank you must refresh this page
Oracle Inequalities In Empirical Risk Minimization And Sparse Recovery Problems
DOWNLOAD
Author : Vladimir Koltchinskii
language : en
Publisher:
Release Date : 2011-08-02
Oracle Inequalities In Empirical Risk Minimization And Sparse Recovery Problems written by Vladimir Koltchinskii and has been published by this book supported file pdf, txt, epub, kindle and other format this book has been release on 2011-08-02 with categories.
Oracle Inequalities In Empirical Risk Minimization And Sparse Recovery Problems
DOWNLOAD
Author : Vladimir Koltchinskii
language : en
Publisher: Springer
Release Date : 2011-07-29
Oracle Inequalities In Empirical Risk Minimization And Sparse Recovery Problems written by Vladimir Koltchinskii and has been published by Springer this book supported file pdf, txt, epub, kindle and other format this book has been release on 2011-07-29 with Mathematics categories.
The purpose of these lecture notes is to provide an introduction to the general theory of empirical risk minimization with an emphasis on excess risk bounds and oracle inequalities in penalized problems. In recent years, there have been new developments in this area motivated by the study of new classes of methods in machine learning such as large margin classification methods (boosting, kernel machines). The main probabilistic tools involved in the analysis of these problems are concentration and deviation inequalities by Talagrand along with other methods of empirical processes theory (symmetrization inequalities, contraction inequality for Rademacher sums, entropy and generic chaining bounds). Sparse recovery based on l_1-type penalization and low rank matrix recovery based on the nuclear norm penalization are other active areas of research, where the main problems can be stated in the framework of penalized empirical risk minimization, and concentration inequalities and empirical processes tools have proved to be very useful.
Concentration Inequalities
DOWNLOAD
Author : Stéphane Boucheron
language : en
Publisher: OUP Oxford
Release Date : 2013-02-07
Concentration Inequalities written by Stéphane Boucheron and has been published by OUP Oxford this book supported file pdf, txt, epub, kindle and other format this book has been release on 2013-02-07 with Mathematics categories.
Concentration inequalities for functions of independent random variables is an area of probability theory that has witnessed a great revolution in the last few decades, and has applications in a wide variety of areas such as machine learning, statistics, discrete mathematics, and high-dimensional geometry. Roughly speaking, if a function of many independent random variables does not depend too much on any of the variables then it is concentrated in the sense that with high probability, it is close to its expected value. This book offers a host of inequalities to illustrate this rich theory in an accessible way by covering the key developments and applications in the field. The authors describe the interplay between the probabilistic structure (independence) and a variety of tools ranging from functional inequalities to transportation arguments to information theory. Applications to the study of empirical processes, random projections, random matrix theory, and threshold phenomena are also presented. A self-contained introduction to concentration inequalities, it includes a survey of concentration of sums of independent random variables, variance bounds, the entropy method, and the transportation method. Deep connections with isoperimetric problems are revealed whilst special attention is paid to applications to the supremum of empirical processes. Written by leading experts in the field and containing extensive exercise sections this book will be an invaluable resource for researchers and graduate students in mathematics, theoretical computer science, and engineering.
Estimation And Testing Under Sparsity
DOWNLOAD
Author : Sara van de Geer
language : en
Publisher: Springer
Release Date : 2016-06-28
Estimation And Testing Under Sparsity written by Sara van de Geer and has been published by Springer this book supported file pdf, txt, epub, kindle and other format this book has been release on 2016-06-28 with Mathematics categories.
Taking the Lasso method as its starting point, this book describes the main ingredients needed to study general loss functions and sparsity-inducing regularizers. It also provides a semi-parametric approach to establishing confidence intervals and tests. Sparsity-inducing methods have proven to be very useful in the analysis of high-dimensional data. Examples include the Lasso and group Lasso methods, and the least squares method with other norm-penalties, such as the nuclear norm. The illustrations provided include generalized linear models, density estimation, matrix completion and sparse principal components. Each chapter ends with a problem section. The book can be used as a textbook for a graduate or PhD course.
Empirical Inference
DOWNLOAD
Author : Bernhard Schölkopf
language : en
Publisher: Springer Science & Business Media
Release Date : 2013-12-11
Empirical Inference written by Bernhard Schölkopf and has been published by Springer Science & Business Media this book supported file pdf, txt, epub, kindle and other format this book has been release on 2013-12-11 with Computers categories.
This book honours the outstanding contributions of Vladimir Vapnik, a rare example of a scientist for whom the following statements hold true simultaneously: his work led to the inception of a new field of research, the theory of statistical learning and empirical inference; he has lived to see the field blossom; and he is still as active as ever. He started analyzing learning algorithms in the 1960s and he invented the first version of the generalized portrait algorithm. He later developed one of the most successful methods in machine learning, the support vector machine (SVM) – more than just an algorithm, this was a new approach to learning problems, pioneering the use of functional analysis and convex optimization in machine learning. Part I of this book contains three chapters describing and witnessing some of Vladimir Vapnik's contributions to science. In the first chapter, Léon Bottou discusses the seminal paper published in 1968 by Vapnik and Chervonenkis that lay the foundations of statistical learning theory, and the second chapter is an English-language translation of that original paper. In the third chapter, Alexey Chervonenkis presents a first-hand account of the early history of SVMs and valuable insights into the first steps in the development of the SVM in the framework of the generalised portrait method. The remaining chapters, by leading scientists in domains such as statistics, theoretical computer science, and mathematics, address substantial topics in the theory and practice of statistical learning theory, including SVMs and other kernel-based methods, boosting, PAC-Bayesian theory, online and transductive learning, loss functions, learnable function classes, notions of complexity for function classes, multitask learning, and hypothesis selection. These contributions include historical and context notes, short surveys, and comments on future research directions. This book will be of interest to researchers, engineers, and graduate students engaged with all aspects of statistical learning.
Weak Convergence And Empirical Processes
DOWNLOAD
Author : A. W. van der Vaart
language : en
Publisher: Springer Nature
Release Date : 2023-07-11
Weak Convergence And Empirical Processes written by A. W. van der Vaart and has been published by Springer Nature this book supported file pdf, txt, epub, kindle and other format this book has been release on 2023-07-11 with Mathematics categories.
This book provides an account of weak convergence theory, empirical processes, and their application to a wide variety of problems in statistics. The first part of the book presents a thorough treatment of stochastic convergence in its various forms. Part 2 brings together the theory of empirical processes in a form accessible to statisticians and probabilists. In Part 3, the authors cover a range of applications in statistics including rates of convergence of estimators; limit theorems for M− and Z−estimators; the bootstrap; the functional delta-method and semiparametric estimation. Most of the chapters conclude with “problems and complements.” Some of these are exercises to help the reader’s understanding of the material, whereas others are intended to supplement the text. This second edition includes many of the new developments in the field since publication of the first edition in 1996: Glivenko-Cantelli preservation theorems; new bounds on expectations of suprema of empirical processes; new bounds on covering numbers for various function classes; generic chaining; definitive versions of concentration bounds; and new applications in statistics including penalized M-estimation, the lasso, classification, and support vector machines. The approximately 200 additional pages also round out classical subjects, including chapters on weak convergence in Skorokhod space, on stable convergence, and on processes based on pseudo-observations.
Geometric Aspects Of Functional Analysis
DOWNLOAD
Author : Bo'az Klartag
language : en
Publisher: Springer
Release Date : 2017-04-17
Geometric Aspects Of Functional Analysis written by Bo'az Klartag and has been published by Springer this book supported file pdf, txt, epub, kindle and other format this book has been release on 2017-04-17 with Mathematics categories.
As in the previous Seminar Notes, the current volume reflects general trends in the study of Geometric Aspects of Functional Analysis, understood in a broad sense. A classical theme in the Local Theory of Banach Spaces which is well represented in this volume is the identification of lower-dimensional structures in high-dimensional objects. More recent applications of high-dimensionality are manifested by contributions in Random Matrix Theory, Concentration of Measure and Empirical Processes. Naturally, the Gaussian measure plays a central role in many of these topics, and is also studied in this volume; in particular, the recent breakthrough proof of the Gaussian Correlation Conjecture is revisited. The interplay of the theory with Harmonic and Spectral Analysis is also well apparent in several contributions. The classical relation to both the primal and dual Brunn-Minkowski theories is also well represented, and related algebraic structures pertaining to valuations and valent functions are discussed. All contributions are original research papers and were subject to the usual refereeing standards.
High Dimensional Probability Vi
DOWNLOAD
Author : Christian Houdré
language : en
Publisher: Springer Science & Business Media
Release Date : 2013-04-19
High Dimensional Probability Vi written by Christian Houdré and has been published by Springer Science & Business Media this book supported file pdf, txt, epub, kindle and other format this book has been release on 2013-04-19 with Mathematics categories.
This is a collection of papers by participants at High Dimensional Probability VI Meeting held from October 9-14, 2011 at the Banff International Research Station in Banff, Alberta, Canada. High Dimensional Probability (HDP) is an area of mathematics that includes the study of probability distributions and limit theorems in infinite-dimensional spaces such as Hilbert spaces and Banach spaces. The most remarkable feature of this area is that it has resulted in the creation of powerful new tools and perspectives, whose range of application has led to interactions with other areas of mathematics, statistics, and computer science. These include random matrix theory, nonparametric statistics, empirical process theory, statistical learning theory, concentration of measure phenomena, strong and weak approximations, distribution function estimation in high dimensions, combinatorial optimization, and random graph theory. The papers in this volume show that HDP theory continues to develop new tools, methods, techniques and perspectives to analyze the random phenomena. Both researchers and advanced students will find this book of great use for learning about new avenues of research.
Algorithmic Learning Theory
DOWNLOAD
Author : Ronald Ortner
language : en
Publisher: Springer
Release Date : 2016-10-12
Algorithmic Learning Theory written by Ronald Ortner and has been published by Springer this book supported file pdf, txt, epub, kindle and other format this book has been release on 2016-10-12 with Computers categories.
This book constitutes the refereed proceedings of the 27th International Conference on Algorithmic Learning Theory, ALT 2016, held in Bari, Italy, in October 2016, co-located with the 19th International Conference on Discovery Science, DS 2016. The 24 regular papers presented in this volume were carefully reviewed and selected from 45 submissions. In addition the book contains 5 abstracts of invited talks. The papers are organized in topical sections named: error bounds, sample compression schemes; statistical learning, theory, evolvability; exact and interactive learning; complexity of teaching models; inductive inference; online learning; bandits and reinforcement learning; and clustering.
Model Selection And Error Estimation In A Nutshell
DOWNLOAD
Author : Luca Oneto
language : en
Publisher: Springer
Release Date : 2019-07-17
Model Selection And Error Estimation In A Nutshell written by Luca Oneto and has been published by Springer this book supported file pdf, txt, epub, kindle and other format this book has been release on 2019-07-17 with Computers categories.
How can we select the best performing data-driven model? How can we rigorously estimate its generalization error? Statistical learning theory answers these questions by deriving non-asymptotic bounds on the generalization error of a model or, in other words, by upper bounding the true error of the learned model based just on quantities computed on the available data. However, for a long time, Statistical learning theory has been considered only an abstract theoretical framework, useful for inspiring new learning approaches, but with limited applicability to practical problems. The purpose of this book is to give an intelligible overview of the problems of model selection and error estimation, by focusing on the ideas behind the different statistical learning theory approaches and simplifying most of the technical aspects with the purpose of making them more accessible and usable in practice. The book starts by presenting the seminal works of the 80’s and includes the most recent results. It discusses open problems and outlines future directions for research.