Semiparametric Theory And Missing Data
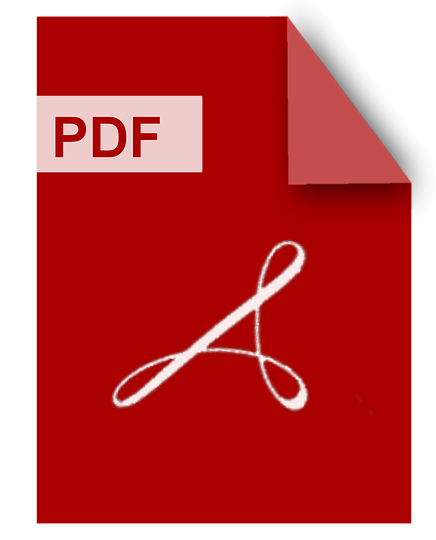
DOWNLOAD
Download Semiparametric Theory And Missing Data PDF/ePub or read online books in Mobi eBooks. Click Download or Read Online button to get Semiparametric Theory And Missing Data book now. This website allows unlimited access to, at the time of writing, more than 1.5 million titles, including hundreds of thousands of titles in various foreign languages. If the content not found or just blank you must refresh this page
Semiparametric Theory And Missing Data
DOWNLOAD
Author : Anastasios Tsiatis
language : en
Publisher: Springer Science & Business Media
Release Date : 2007-01-15
Semiparametric Theory And Missing Data written by Anastasios Tsiatis and has been published by Springer Science & Business Media this book supported file pdf, txt, epub, kindle and other format this book has been release on 2007-01-15 with Mathematics categories.
Missing data arise in almost all scientific disciplines. In many cases, the treatment of missing data in an analysis is carried out in a casual and ad-hoc manner, leading, in many cases, to invalid inference and erroneous conclusions. In the past 20 years or so, there has been a serious attempt to understand the underlying issues and difficulties that come about from missing data and their impact on subsequent analysis. There has been a great deal written on the theory developed for analyzing missing data for finite-dimensional parametric models. This includes an extensive literature on likelihood-based methods and multiple imputation. More recently, there has been increasing interest in semiparametric models which, roughly speaking, are models that include both a parametric and nonparametric component. Such models are popular because estimators in such models are more robust than in traditional parametric models. The theory of missing data applied to semiparametric models is scattered throughout the literature with no thorough comprehensive treatment of the subject. This book combines much of what is known in regard to the theory of estimation for semiparametric models with missing data in an organized and comprehensive manner. It starts with the study of semiparametric methods when there are no missing data. The description of the theory of estimation for semiparametric models is at a level that is both rigorous and intuitive, relying on geometric ideas to reinforce the intuition and understanding of the theory. These methods are then applied to problems with missing, censored, and coarsened data with the goal of deriving estimators that are as robust and efficient as possible.
Semiparametric Theory And Missing Data Springer Series In Statistics
DOWNLOAD
Author :
language : en
Publisher:
Release Date : 2006
Semiparametric Theory And Missing Data Springer Series In Statistics written by and has been published by this book supported file pdf, txt, epub, kindle and other format this book has been release on 2006 with categories.
Missing Data In Longitudinal Studies
DOWNLOAD
Author : Michael J. Daniels
language : en
Publisher: CRC Press
Release Date : 2008-03-11
Missing Data In Longitudinal Studies written by Michael J. Daniels and has been published by CRC Press this book supported file pdf, txt, epub, kindle and other format this book has been release on 2008-03-11 with Mathematics categories.
Drawing from the authors' own work and from the most recent developments in the field, Missing Data in Longitudinal Studies: Strategies for Bayesian Modeling and Sensitivity Analysis describes a comprehensive Bayesian approach for drawing inference from incomplete data in longitudinal studies. To illustrate these methods, the authors employ
Nonparametric And Semiparametric Models
DOWNLOAD
Author : Wolfgang Karl Härdle
language : en
Publisher: Springer Science & Business Media
Release Date : 2012-08-27
Nonparametric And Semiparametric Models written by Wolfgang Karl Härdle and has been published by Springer Science & Business Media this book supported file pdf, txt, epub, kindle and other format this book has been release on 2012-08-27 with Mathematics categories.
The statistical and mathematical principles of smoothing with a focus on applicable techniques are presented in this book. It naturally splits into two parts: The first part is intended for undergraduate students majoring in mathematics, statistics, econometrics or biometrics whereas the second part is intended to be used by master and PhD students or researchers. The material is easy to accomplish since the e-book character of the text gives a maximum of flexibility in learning (and teaching) intensity.
Bayesian Nonparametrics
DOWNLOAD
Author : J.K. Ghosh
language : en
Publisher: Springer Science & Business Media
Release Date : 2006-05-11
Bayesian Nonparametrics written by J.K. Ghosh and has been published by Springer Science & Business Media this book supported file pdf, txt, epub, kindle and other format this book has been release on 2006-05-11 with Mathematics categories.
This book is the first systematic treatment of Bayesian nonparametric methods and the theory behind them. It will also appeal to statisticians in general. The book is primarily aimed at graduate students and can be used as the text for a graduate course in Bayesian non-parametrics.
Applied Missing Data Analysis In The Health Sciences
DOWNLOAD
Author : Xiao-Hua Zhou
language : en
Publisher: John Wiley & Sons
Release Date : 2014-05-19
Applied Missing Data Analysis In The Health Sciences written by Xiao-Hua Zhou and has been published by John Wiley & Sons this book supported file pdf, txt, epub, kindle and other format this book has been release on 2014-05-19 with Medical categories.
Applied Missing Data Analysis in the Health Sciences A modern and practical guide to the essential concepts and ideas for analyzing data with missing observations in the field of biostatistics With an emphasis on hands-on applications, Applied Missing Data Analysis in the Health Sciences outlines the various statistical methods for the analysis of missing data. The authors acknowledge the limitations of established techniques and provide newly-developed methods with concrete applications in areas such as causal inference. Organized by types of data, chapter coverage begins with an overall introduction to the existence and limitations of missing data and continues into techniques for missing data inference, including likelihood-based, weighted GEE, multiple imputation, and Bayesian methods. The book subsequently covers cross-sectional, longitudinal, hierarchical, survival data. In addition, Applied Missing Data Analysis in the Health Sciences features: Multiple data sets that can be replicated using SAS®, Stata®, R, and WinBUGS software packages Numerous examples of case studies to illustrate real-world scenarios and demonstrate applications of discussed methodologies Detailed appendices to guide readers through the use of the presented data in various software environments Applied Missing Data Analysis in the Health Sciences is an excellent textbook for upper-undergraduate and graduate-level biostatistics courses as well as an ideal resource for health science researchers and applied statisticians.
Handbook Of Quantile Regression
DOWNLOAD
Author : Roger Koenker
language : en
Publisher: CRC Press
Release Date : 2017-10-12
Handbook Of Quantile Regression written by Roger Koenker and has been published by CRC Press this book supported file pdf, txt, epub, kindle and other format this book has been release on 2017-10-12 with Mathematics categories.
Quantile regression constitutes an ensemble of statistical techniques intended to estimate and draw inferences about conditional quantile functions. Median regression, as introduced in the 18th century by Boscovich and Laplace, is a special case. In contrast to conventional mean regression that minimizes sums of squared residuals, median regression minimizes sums of absolute residuals; quantile regression simply replaces symmetric absolute loss by asymmetric linear loss. Since its introduction in the 1970's by Koenker and Bassett, quantile regression has been gradually extended to a wide variety of data analytic settings including time series, survival analysis, and longitudinal data. By focusing attention on local slices of the conditional distribution of response variables it is capable of providing a more complete, more nuanced view of heterogeneous covariate effects. Applications of quantile regression can now be found throughout the sciences, including astrophysics, chemistry, ecology, economics, finance, genomics, medicine, and meteorology. Software for quantile regression is now widely available in all the major statistical computing environments. The objective of this volume is to provide a comprehensive review of recent developments of quantile regression methodology illustrating its applicability in a wide range of scientific settings. The intended audience of the volume is researchers and graduate students across a diverse set of disciplines.
Asymptotics In Statistics
DOWNLOAD
Author : Lucien Le Cam
language : en
Publisher: Springer Science & Business Media
Release Date : 2012-12-06
Asymptotics In Statistics written by Lucien Le Cam and has been published by Springer Science & Business Media this book supported file pdf, txt, epub, kindle and other format this book has been release on 2012-12-06 with Mathematics categories.
by Sara van de Geer. Also, we did not include material due to David Donoho, lain Johnstone, and their school. We found our selves unprepared to write a distillate of the material. We did touch briefly on "nonparametrics," but not on "semiparamet rics." This is because we feel that the semiparametric situation has not yet been properly structured. We hope that the reader will find this book interesting and challenging, in spite of its shortcomings. The material was typed in LaTeX form by the authors them selves, borrowing liberally from the 1990 script by Chris Bush. It was reviewed anonymously by distinguished colleagues. We thank them for their kind encouragement. Very special thanks are due to Professor David Pollard who took time out of a busy schedule to give us a long list of suggestions. We did not follow them all, but we at least made attempts. We wish also to thank the staff of Springer-Verlag for their help, in particular editor John Kimmel, who tried to make us work with all deliberate speed. Thanks are due to Paul Smith, Te-Ching Chen and Ju-Yi-Yen, who helped with the last-minute editorial corrections.
Information Criteria And Statistical Modeling
DOWNLOAD
Author : Sadanori Konishi
language : en
Publisher: Springer Science & Business Media
Release Date : 2008
Information Criteria And Statistical Modeling written by Sadanori Konishi and has been published by Springer Science & Business Media this book supported file pdf, txt, epub, kindle and other format this book has been release on 2008 with Business & Economics categories.
Statistical modeling is a critical tool in scientific research. This book provides comprehensive explanations of the concepts and philosophy of statistical modeling, together with a wide range of practical and numerical examples. The authors expect this work to be of great value not just to statisticians but also to researchers and practitioners in various fields of research such as information science, computer science, engineering, bioinformatics, economics, marketing and environmental science. It’s a crucial area of study, as statistical models are used to understand phenomena with uncertainty and to determine the structure of complex systems. They’re also used to control such systems, as well as to make reliable predictions in various natural and social science fields.
Partial Identification Of Probability Distributions
DOWNLOAD
Author : Charles F. Manski
language : en
Publisher: Springer Science & Business Media
Release Date : 2006-04-29
Partial Identification Of Probability Distributions written by Charles F. Manski and has been published by Springer Science & Business Media this book supported file pdf, txt, epub, kindle and other format this book has been release on 2006-04-29 with Mathematics categories.
Sample data alone never suffice to draw conclusions about populations. Inference always requires assumptions about the population and sampling process. Statistical theory has revealed much about how strength of assumptions affects the precision of point estimates, but has had much less to say about how it affects the identification of population parameters. Indeed, it has been commonplace to think of identification as a binary event – a parameter is either identified or not – and to view point identification as a precondition for inference. Yet there is enormous scope for fruitful inference using data and assumptions that partially identify population parameters. This book explains why and shows how. The book presents in a rigorous and thorough manner the main elements of Charles Manski's research on partial identification of probability distributions. One focus is prediction with missing outcome or covariate data. Another is decomposition of finite mixtures, with application to the analysis of contaminated sampling and ecological inference. A third major focus is the analysis of treatment response. Whatever the particular subject under study, the presentation follows a common path. The author first specifies the sampling process generating the available data and asks what may be learned about population parameters using the empirical evidence alone. He then ask how the (typically) setvalued identification regions for these parameters shrink if various assumptions are imposed. The approach to inference that runs throughout the book is deliberately conservative and thoroughly nonparametric.