Shrinkage Estimation
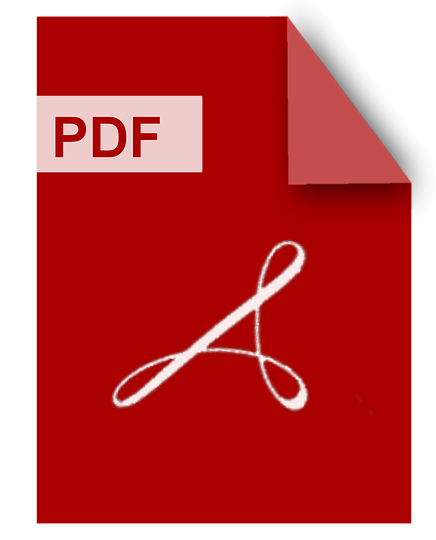
DOWNLOAD
Download Shrinkage Estimation PDF/ePub or read online books in Mobi eBooks. Click Download or Read Online button to get Shrinkage Estimation book now. This website allows unlimited access to, at the time of writing, more than 1.5 million titles, including hundreds of thousands of titles in various foreign languages. If the content not found or just blank you must refresh this page
Shrinkage Estimation
DOWNLOAD
Author : Dominique Fourdrinier
language : en
Publisher: Springer
Release Date : 2018-11-27
Shrinkage Estimation written by Dominique Fourdrinier and has been published by Springer this book supported file pdf, txt, epub, kindle and other format this book has been release on 2018-11-27 with Mathematics categories.
This book provides a coherent framework for understanding shrinkage estimation in statistics. The term refers to modifying a classical estimator by moving it closer to a target which could be known a priori or arise from a model. The goal is to construct estimators with improved statistical properties. The book focuses primarily on point and loss estimation of the mean vector of multivariate normal and spherically symmetric distributions. Chapter 1 reviews the statistical and decision theoretic terminology and results that will be used throughout the book. Chapter 2 is concerned with estimating the mean vector of a multivariate normal distribution under quadratic loss from a frequentist perspective. In Chapter 3 the authors take a Bayesian view of shrinkage estimation in the normal setting. Chapter 4 introduces the general classes of spherically and elliptically symmetric distributions. Point and loss estimation for these broad classes are studied in subsequent chapters. In particular, Chapter 5 extends many of the results from Chapters 2 and 3 to spherically and elliptically symmetric distributions. Chapter 6 considers the general linear model with spherically symmetric error distributions when a residual vector is available. Chapter 7 then considers the problem of estimating a location vector which is constrained to lie in a convex set. Much of the chapter is devoted to one of two types of constraint sets, balls and polyhedral cones. In Chapter 8 the authors focus on loss estimation and data-dependent evidence reports. Appendices cover a number of technical topics including weakly differentiable functions; examples where Stein’s identity doesn’t hold; Stein’s lemma and Stokes’ theorem for smooth boundaries; harmonic, superharmonic and subharmonic functions; and modified Bessel functions.
Shrinkage Estimation For Mean And Covariance Matrices
DOWNLOAD
Author : Hisayuki Tsukuma
language : en
Publisher: Springer Nature
Release Date : 2020-04-16
Shrinkage Estimation For Mean And Covariance Matrices written by Hisayuki Tsukuma and has been published by Springer Nature this book supported file pdf, txt, epub, kindle and other format this book has been release on 2020-04-16 with Medical categories.
This book provides a self-contained introduction to shrinkage estimation for matrix-variate normal distribution models. More specifically, it presents recent techniques and results in estimation of mean and covariance matrices with a high-dimensional setting that implies singularity of the sample covariance matrix. Such high-dimensional models can be analyzed by using the same arguments as for low-dimensional models, thus yielding a unified approach to both high- and low-dimensional shrinkage estimations. The unified shrinkage approach not only integrates modern and classical shrinkage estimation, but is also required for further development of the field. Beginning with the notion of decision-theoretic estimation, this book explains matrix theory, group invariance, and other mathematical tools for finding better estimators. It also includes examples of shrinkage estimators for improving standard estimators, such as least squares, maximum likelihood, and minimum risk invariant estimators, and discusses the historical background and related topics in decision-theoretic estimation of parameter matrices. This book is useful for researchers and graduate students in various fields requiring data analysis skills as well as in mathematical statistics.
Penalty Shrinkage And Pretest Strategies
DOWNLOAD
Author : S. Ejaz Ahmed
language : en
Publisher: Springer Science & Business Media
Release Date : 2013-12-11
Penalty Shrinkage And Pretest Strategies written by S. Ejaz Ahmed and has been published by Springer Science & Business Media this book supported file pdf, txt, epub, kindle and other format this book has been release on 2013-12-11 with Mathematics categories.
The objective of this book is to compare the statistical properties of penalty and non-penalty estimation strategies for some popular models. Specifically, it considers the full model, submodel, penalty, pretest and shrinkage estimation techniques for three regression models before presenting the asymptotic properties of the non-penalty estimators and their asymptotic distributional efficiency comparisons. Further, the risk properties of the non-penalty estimators and penalty estimators are explored through a Monte Carlo simulation study. Showcasing examples based on real datasets, the book will be useful for students and applied researchers in a host of applied fields. The book’s level of presentation and style make it accessible to a broad audience. It offers clear, succinct expositions of each estimation strategy. More importantly, it clearly describes how to use each estimation strategy for the problem at hand. The book is largely self-contained, as are the individual chapters, so that anyone interested in a particular topic or area of application may read only that specific chapter. The book is specially designed for graduate students who want to understand the foundations and concepts underlying penalty and non-penalty estimation and its applications. It is well-suited as a textbook for senior undergraduate and graduate courses surveying penalty and non-penalty estimation strategies, and can also be used as a reference book for a host of related subjects, including courses on meta-analysis. Professional statisticians will find this book to be a valuable reference work, since nearly all chapters are self-contained.
Shrinkage Estimation Of A Linear Regression
DOWNLOAD
Author : Kazuhiro Ohtani
language : en
Publisher:
Release Date : 2000
Shrinkage Estimation Of A Linear Regression written by Kazuhiro Ohtani and has been published by this book supported file pdf, txt, epub, kindle and other format this book has been release on 2000 with Business & Economics categories.
This book deals with shrinkage regression estimators obtained by shrinking the ordinary least squares (OLS) estimator towards the origin. The author's main concern is to compare the sampling properties of a family of Stein-rule estimators with those of a family of minimum mean squared error estimators. In this book, the author deals with shrinkage regression estimators obtained by shrinking the ordinary least squares (OLS) estimator towards the origin. In particular, he deals with a family of Stein-rule (SR) estimators and a family of minimum mean squared error (MMSE) estimators.
Statistical Portfolio Estimation
DOWNLOAD
Author : Masanobu Taniguchi
language : en
Publisher: CRC Press
Release Date : 2017-09-01
Statistical Portfolio Estimation written by Masanobu Taniguchi and has been published by CRC Press this book supported file pdf, txt, epub, kindle and other format this book has been release on 2017-09-01 with Mathematics categories.
The composition of portfolios is one of the most fundamental and important methods in financial engineering, used to control the risk of investments. This book provides a comprehensive overview of statistical inference for portfolios and their various applications. A variety of asset processes are introduced, including non-Gaussian stationary processes, nonlinear processes, non-stationary processes, and the book provides a framework for statistical inference using local asymptotic normality (LAN). The approach is generalized for portfolio estimation, so that many important problems can be covered. This book can primarily be used as a reference by researchers from statistics, mathematics, finance, econometrics, and genomics. It can also be used as a textbook by senior undergraduate and graduate students in these fields.
Shrinkage Estimation In Nonparametric Bayesian Survival Analysis
DOWNLOAD
Author : Kamta Rai
language : en
Publisher:
Release Date : 1979
Shrinkage Estimation In Nonparametric Bayesian Survival Analysis written by Kamta Rai and has been published by this book supported file pdf, txt, epub, kindle and other format this book has been release on 1979 with Bayesian statistical decision theory categories.
Handbook Of Financial Econometrics
DOWNLOAD
Author : Yacine Ait-Sahalia
language : en
Publisher: Elsevier
Release Date : 2009-10-19
Handbook Of Financial Econometrics written by Yacine Ait-Sahalia and has been published by Elsevier this book supported file pdf, txt, epub, kindle and other format this book has been release on 2009-10-19 with Business & Economics categories.
This collection of original articles—8 years in the making—shines a bright light on recent advances in financial econometrics. From a survey of mathematical and statistical tools for understanding nonlinear Markov processes to an exploration of the time-series evolution of the risk-return tradeoff for stock market investment, noted scholars Yacine Aït-Sahalia and Lars Peter Hansen benchmark the current state of knowledge while contributors build a framework for its growth. Whether in the presence of statistical uncertainty or the proven advantages and limitations of value at risk models, readers will discover that they can set few constraints on the value of this long-awaited volume. - Presents a broad survey of current research—from local characterizations of the Markov process dynamics to financial market trading activity - Contributors include Nobel Laureate Robert Engle and leading econometricians - Offers a clarity of method and explanation unavailable in other financial econometrics collections
Post Shrinkage Strategies In Statistical And Machine Learning For High Dimensional Data
DOWNLOAD
Author : Syed Ejaz Ahmed
language : en
Publisher: CRC Press
Release Date : 2023-05-25
Post Shrinkage Strategies In Statistical And Machine Learning For High Dimensional Data written by Syed Ejaz Ahmed and has been published by CRC Press this book supported file pdf, txt, epub, kindle and other format this book has been release on 2023-05-25 with Business & Economics categories.
This book presents some post-estimation and predictions strategies for the host of useful statistical models with applications in data science. It combines statistical learning and machine learning techniques in a unique and optimal way. It is well-known that machine learning methods are subject to many issues relating to bias, and consequently the mean squared error and prediction error may explode. For this reason, we suggest shrinkage strategies to control the bias by combining a submodel selected by a penalized method with a model with many features. Further, the suggested shrinkage methodology can be successfully implemented for high dimensional data analysis. Many researchers in statistics and medical sciences work with big data. They need to analyse this data through statistical modelling. Estimating the model parameters accurately is an important part of the data analysis. This book may be a repository for developing improve estimation strategies for statisticians. This book will help researchers and practitioners for their teaching and advanced research, and is an excellent textbook for advanced undergraduate and graduate courses involving shrinkage, statistical, and machine learning. The book succinctly reveals the bias inherited in machine learning method and successfully provides tools, tricks and tips to deal with the bias issue. Expertly sheds light on the fundamental reasoning for model selection and post estimation using shrinkage and related strategies. This presentation is fundamental, because shrinkage and other methods appropriate for model selection and estimation problems and there is a growing interest in this area to fill the gap between competitive strategies. Application of these strategies to real life data set from many walks of life. Analytical results are fully corroborated by numerical work and numerous worked examples are included in each chapter with numerous graphs for data visualization. The presentation and style of the book clearly makes it accessible to a broad audience. It offers rich, concise expositions of each strategy and clearly describes how to use each estimation strategy for the problem at hand. This book emphasizes that statistics/statisticians can play a dominant role in solving Big Data problems, and will put them on the precipice of scientific discovery. The book contributes novel methodologies for HDDA and will open a door for continued research in this hot area. The practical impact of the proposed work stems from wide applications. The developed computational packages will aid in analyzing a broad range of applications in many walks of life.
Shrinkage Estimation For Mean And Covariance Matrices
DOWNLOAD
Author : Hisayuki Tsukuma
language : en
Publisher: Springer
Release Date : 2020-04-17
Shrinkage Estimation For Mean And Covariance Matrices written by Hisayuki Tsukuma and has been published by Springer this book supported file pdf, txt, epub, kindle and other format this book has been release on 2020-04-17 with Medical categories.
This book provides a self-contained introduction to shrinkage estimation for matrix-variate normal distribution models. More specifically, it presents recent techniques and results in estimation of mean and covariance matrices with a high-dimensional setting that implies singularity of the sample covariance matrix. Such high-dimensional models can be analyzed by using the same arguments as for low-dimensional models, thus yielding a unified approach to both high- and low-dimensional shrinkage estimations. The unified shrinkage approach not only integrates modern and classical shrinkage estimation, but is also required for further development of the field. Beginning with the notion of decision-theoretic estimation, this book explains matrix theory, group invariance, and other mathematical tools for finding better estimators. It also includes examples of shrinkage estimators for improving standard estimators, such as least squares, maximum likelihood, and minimum risk invariant estimators, and discusses the historical background and related topics in decision-theoretic estimation of parameter matrices. This book is useful for researchers and graduate students in various fields requiring data analysis skills as well as in mathematical statistics.
Multilevel Analysis
DOWNLOAD
Author : J. J. Hox
language : en
Publisher: Psychology Press
Release Date : 2002
Multilevel Analysis written by J. J. Hox and has been published by Psychology Press this book supported file pdf, txt, epub, kindle and other format this book has been release on 2002 with Mathematics categories.
This volume provides an introduction to multilevel analysis for applied researchers. The book presents two types of multilevel models: the multilevel regression model and a model for multilevel covariance structures.