Smoothing Filtering And Prediction
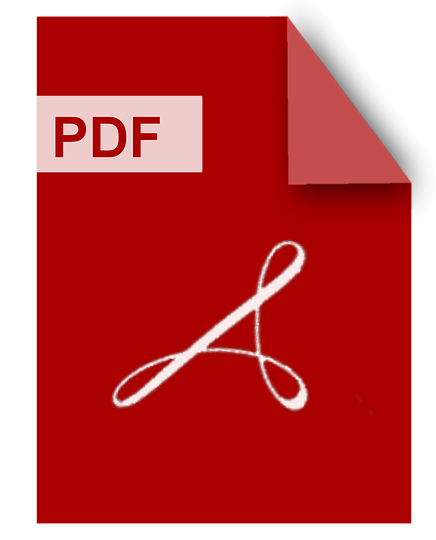
DOWNLOAD
Download Smoothing Filtering And Prediction PDF/ePub or read online books in Mobi eBooks. Click Download or Read Online button to get Smoothing Filtering And Prediction book now. This website allows unlimited access to, at the time of writing, more than 1.5 million titles, including hundreds of thousands of titles in various foreign languages. If the content not found or just blank you must refresh this page
Smoothing Filtering And Prediction
DOWNLOAD
Author : Jeremy Weissberg
language : en
Publisher:
Release Date : 2016-09-15
Smoothing Filtering And Prediction written by Jeremy Weissberg and has been published by this book supported file pdf, txt, epub, kindle and other format this book has been release on 2016-09-15 with categories.
Smoothing is often used to reduce noise within an image or to produce a less pixelated image. Most smoothing methods are based on low pass filters. Smoothing is also usually based on a single value representing the image, such as the average value of the image or the middle (median) value. In image processing, to smooth a data set is to create an approximating function that attempts to capture important patterns in the data, while leaving out noise or other fine-scale structures/rapid phenomena. In smoothing, the data points of a signal are modified so individual points (presumably because of noise) are reduced, and points that are lower than the adjacent points are increased leading to a smoother signal. Smoothing may be used in two important ways that can aid in data analysis; by being able to extract more information from the data as long as the assumption of smoothing is reasonable and; by being able to provide analyses that are both flexible and robust. Filtering and prediction is about observing moving objects when the observations are corrupted by random errors. Smoothing, Filtering and Prediction - Estimating The Past, Present and Future describes the classical smoothing, filtering and prediction techniques together with some more recently developed embellishments for improving performance within applications. It aims to present the subject in an accessible way, so that it can serve as a practical guide for undergraduates and newcomers to the field.
Smoothing Filtering And Prediction
DOWNLOAD
Author : Garry Einicke
language : en
Publisher: BoD – Books on Demand
Release Date : 2012-02-24
Smoothing Filtering And Prediction written by Garry Einicke and has been published by BoD – Books on Demand this book supported file pdf, txt, epub, kindle and other format this book has been release on 2012-02-24 with Computers categories.
This book describes the classical smoothing, filtering and prediction techniques together with some more recently developed embellishments for improving performance within applications. It aims to present the subject in an accessible way, so that it can serve as a practical guide for undergraduates and newcomers to the field. The material is organised as a ten-lecture course. The foundations are laid in Chapters 1 and 2, which explain minimum-mean-square-error solution construction and asymptotic behaviour. Chapters 3 and 4 introduce continuous-time and discrete-time minimum-variance filtering. Generalisations for missing data, deterministic inputs, correlated noises, direct feedthrough terms, output estimation and equalisation are described. Chapter 5 simplifies the minimum-variance filtering results for steady-state problems. Observability, Riccati equation solution convergence, asymptotic stability and Wiener filter equivalence are discussed. Chapters 6 and 7 cover the subject of continuous-time and discrete-time smoothing. The main fixed-lag, fixed-point and fixed-interval smoother results are derived. It is shown that the minimum-variance fixed-interval smoother attains the best performance. Chapter 8 attends to parameter estimation. As the above-mentioned approaches all rely on knowledge of the underlying model parameters, maximum-likelihood techniques within expectation-maximisation algorithms for joint state and parameter estimation are described. Chapter 9 is concerned with robust techniques that accommodate uncertainties within problem specifications. An extra term within Riccati equations enables designers to trade-off average error and peak error performance. Chapter 10 rounds off the course by applying the afore-mentioned linear techniques to nonlinear estimation problems. It is demonstrated that step-wise linearisations can be used within predictors, filters and smoothers, albeit by forsaking optimal performance guarantees.
Bayesian Filtering And Smoothing
DOWNLOAD
Author : Simo Särkkä
language : en
Publisher: Cambridge University Press
Release Date : 2013-09-05
Bayesian Filtering And Smoothing written by Simo Särkkä and has been published by Cambridge University Press this book supported file pdf, txt, epub, kindle and other format this book has been release on 2013-09-05 with Computers categories.
A unified Bayesian treatment of the state-of-the-art filtering, smoothing, and parameter estimation algorithms for non-linear state space models.
Theory And Principles Of Smoothing Filtering And Prediction
DOWNLOAD
Author : Graham Eanes
language : en
Publisher:
Release Date : 2015-02-23
Theory And Principles Of Smoothing Filtering And Prediction written by Graham Eanes and has been published by this book supported file pdf, txt, epub, kindle and other format this book has been release on 2015-02-23 with categories.
A descriptive account based on the theory as well as principles of smoothing, filtering and prediction techniques has been presented in this book. It aims to provide understanding of classical filtering, prediction techniques and smoothing techniques along with newly developed embellishments for enhancing performance in applications. It describes the domain in a vivid manner for the purpose of serving as a valuable guide for students as well as experts. It extensively discusses minimum-mean-square-error solution construction and asymptotic behavior, continuous-time and discrete-time minimum-variance filtering, minimum-variance filtering results for steady-state problems and continuous-time and discrete-time smoothing. It further elaborates on robust techniques that accommodate uncertainties within problem specifications, parameter estimation, applications of Riccati equations, etc. These afore-mentioned linear techniques have been applied to various nonlinear estimation problems towards the end of the book. Although they have a risk of assurance of optical performance, these mentioned linearizations can be employed in predictors, filters and smoothers. The book serves the objective of imparting practical knowledge amongst students interested in this field.
Smoothing Filtering And Prediction Estimating The Past Present And Future
DOWNLOAD
Author :
language : en
Publisher:
Release Date : 2012
Smoothing Filtering And Prediction Estimating The Past Present And Future written by and has been published by this book supported file pdf, txt, epub, kindle and other format this book has been release on 2012 with categories.
Optimal Filtering
DOWNLOAD
Author : Brian D. O. Anderson
language : en
Publisher: Courier Corporation
Release Date : 2012-05-23
Optimal Filtering written by Brian D. O. Anderson and has been published by Courier Corporation this book supported file pdf, txt, epub, kindle and other format this book has been release on 2012-05-23 with Science categories.
Graduate-level text extends studies of signal processing, particularly regarding communication systems and digital filtering theory. Topics include filtering, linear systems, and estimation; discrete-time Kalman filter; time-invariant filters; more. 1979 edition.
Forecasting Structural Time Series Models And The Kalman Filter
DOWNLOAD
Author : Andrew C. Harvey
language : en
Publisher: Cambridge University Press
Release Date : 1990
Forecasting Structural Time Series Models And The Kalman Filter written by Andrew C. Harvey and has been published by Cambridge University Press this book supported file pdf, txt, epub, kindle and other format this book has been release on 1990 with Business & Economics categories.
A synthesis of concepts and materials, that ordinarily appear separately in time series and econometrics literature, presents a comprehensive review of theoretical and applied concepts in modeling economic and social time series.
Introduction To Mathematical Systems Theory
DOWNLOAD
Author : Christiaan Heij
language : en
Publisher: Springer Science & Business Media
Release Date : 2006-12-18
Introduction To Mathematical Systems Theory written by Christiaan Heij and has been published by Springer Science & Business Media this book supported file pdf, txt, epub, kindle and other format this book has been release on 2006-12-18 with Science categories.
This book provides an introduction to the theory of linear systems and control for students in business mathematics, econometrics, computer science, and engineering; the focus is on discrete time systems. The subjects treated are among the central topics of deterministic linear system theory: controllability, observability, realization theory, stability and stabilization by feedback, LQ-optimal control theory. Kalman filtering and LQC-control of stochastic systems are also discussed, as are modeling, time series analysis and model specification, along with model validation.
Optimal And Robust State Estimation
DOWNLOAD
Author : Yuriy S. Shmaliy
language : en
Publisher: John Wiley & Sons
Release Date : 2022-07-20
Optimal And Robust State Estimation written by Yuriy S. Shmaliy and has been published by John Wiley & Sons this book supported file pdf, txt, epub, kindle and other format this book has been release on 2022-07-20 with Technology & Engineering categories.
A unified and systematic theoretical framework for solving problems related to finite impulse response (FIR) estimate Optimal and Robust State Estimation: Finite Impulse Response (FIR) and Kalman Approaches is a comprehensive investigation into batch state estimators and recursive forms. The work begins by introducing the reader to the state estimation approach and provides a brief historical overview. Next, the work discusses the specific properties of finite impulse response (FIR) state estimators. Further chapters give the basics of probability and stochastic processes, discuss the available linear and nonlinear state estimators, deal with optimal FIR filtering, and consider a limited memory batch and recursive algorithms. Other topics covered include solving the q-lag FIR smoothing problem, introducing the receding horizon (RH) FIR state estimation approach, and developing the theory of FIR state estimation under disturbances. The book closes by discussing the theory of FIR state estimation for uncertain systems and providing several applications where the FIR state estimators are used effectively. Key concepts covered in the work include: A holistic overview of the state estimation approach, which arose from the need to know the internal state of a real system, given that the input and output are both known Optimal, optimal unbiased, maximum likelihood, and unbiased and robust finite impulse response (FIR) structures FIR state estimation approach along with the infinite impulse response (IIR) and Kalman approaches Cost functions and the most critical properties of FIR and IIR state estimates Optimal and Robust State Estimation: Finite Impulse Response (FIR) and Kalman Approaches was written for professionals in the fields of microwave engineering, system engineering, and robotics who wish to move towards solving finite impulse response (FIR) estimate issues in both theoretical and practical applications. Graduate and senior undergraduate students with coursework dealing with state estimation will also be able to use the book to gain a valuable foundation of knowledge and become more adept in their chosen fields of study.
Introduction To Data Science
DOWNLOAD
Author : Rafael A. Irizarry
language : en
Publisher: CRC Press
Release Date : 2019-11-12
Introduction To Data Science written by Rafael A. Irizarry and has been published by CRC Press this book supported file pdf, txt, epub, kindle and other format this book has been release on 2019-11-12 with Mathematics categories.
Introduction to Data Science: Data Analysis and Prediction Algorithms with R introduces concepts and skills that can help you tackle real-world data analysis challenges. It covers concepts from probability, statistical inference, linear regression, and machine learning. It also helps you develop skills such as R programming, data wrangling, data visualization, predictive algorithm building, file organization with UNIX/Linux shell, version control with Git and GitHub, and reproducible document preparation. This book is a textbook for a first course in data science. No previous knowledge of R is necessary, although some experience with programming may be helpful. The book is divided into six parts: R, data visualization, statistics with R, data wrangling, machine learning, and productivity tools. Each part has several chapters meant to be presented as one lecture. The author uses motivating case studies that realistically mimic a data scientist’s experience. He starts by asking specific questions and answers these through data analysis so concepts are learned as a means to answering the questions. Examples of the case studies included are: US murder rates by state, self-reported student heights, trends in world health and economics, the impact of vaccines on infectious disease rates, the financial crisis of 2007-2008, election forecasting, building a baseball team, image processing of hand-written digits, and movie recommendation systems. The statistical concepts used to answer the case study questions are only briefly introduced, so complementing with a probability and statistics textbook is highly recommended for in-depth understanding of these concepts. If you read and understand the chapters and complete the exercises, you will be prepared to learn the more advanced concepts and skills needed to become an expert. A complete solutions manual is available to registered instructors who require the text for a course.