Sparse Model Building From Genome Wide Variation With Graphical Models
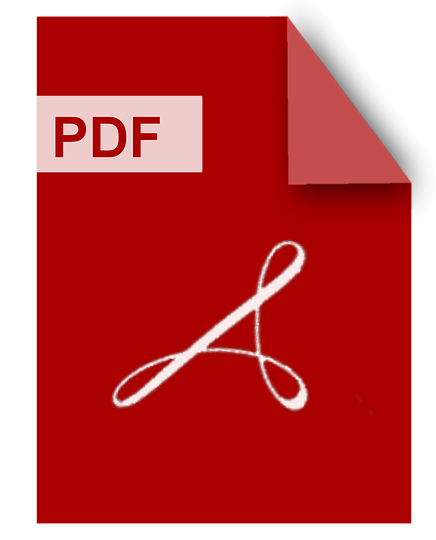
DOWNLOAD
Download Sparse Model Building From Genome Wide Variation With Graphical Models PDF/ePub or read online books in Mobi eBooks. Click Download or Read Online button to get Sparse Model Building From Genome Wide Variation With Graphical Models book now. This website allows unlimited access to, at the time of writing, more than 1.5 million titles, including hundreds of thousands of titles in various foreign languages. If the content not found or just blank you must refresh this page
Sparse Model Building From Genome Wide Variation With Graphical Models
DOWNLOAD
Author : Benjamin Alexander Logsdon
language : en
Publisher:
Release Date : 2011
Sparse Model Building From Genome Wide Variation With Graphical Models written by Benjamin Alexander Logsdon and has been published by this book supported file pdf, txt, epub, kindle and other format this book has been release on 2011 with categories.
High throughput sequencing and expression characterization have lead to an explosion of phenotypic and genotypic molecular data underlying both experimental studies and outbred populations. We develop a novel class of algorithms to reconstruct sparse models among these molecular phenotypes (e.g. expression products) and genotypes (e.g. single nucleotide polymorphisms), via both a Bayesian hierarchical model, when the sample size is much smaller than the model dimension (i.e. p n) and the well characterized adaptive lasso algo- rithm. Specifically, we propose novel approaches to the problems of increasing power to detect additional loci in genome-wide association studies using our variational algorithm, efficiently learning directed cyclic graphs from expression and genotype data using the adaptive lasso, and constructing genomewide undirected graphs among genotype, expression and downstream phenotype data using an extension of the variational feature selection algorithm. The Bayesian hierarchical model is derived for a parametric multiple regression model with a mixture prior of a point mass and normal distribution for each regression coefficient, and appropriate priors for the set of hyperparameters. When combined with a probabilistic consistency bound on the model dimension, this approach leads to very sparse solutions without the need for cross validation. We use a variational Bayes approximate inference approach in our algorithm, where we impose a complete factorization across all parameters for the approximate posterior distribution, and then minimize the KullbackLeibler divergence between the approximate and true posterior distributions. Since the prior distribution is non-convex, we restart the algorithm many times to find multiple posterior modes, and combine information across all discovered modes in an approximate Bayesian model averaging framework, to reduce the variance of the posterior probability estimates. We perform analysis of three major publicly available data-sets: the HapMap 2 genotype and expression data collected on immortalized lymphoblastoid cell lines, the genome-wide gene expression and genetic marker data collected for a yeast intercross, and genomewide gene expression, genetic marker, and downstream phenotypes related to weight in a mouse F2 intercross. Based on both simulations and data analysis we show that our algorithms can outperform other state of the art model selection procedures when including thousands to hundreds of thousands of genotypes and expression traits, in terms of aggressively controlling false discovery rate, and generating rich simultaneous statistical models.
Probabilistic Graphical Models For Genetics Genomics And Postgenomics
DOWNLOAD
Author : Christine Sinoquet
language : en
Publisher: OUP Oxford
Release Date : 2014-09-18
Probabilistic Graphical Models For Genetics Genomics And Postgenomics written by Christine Sinoquet and has been published by OUP Oxford this book supported file pdf, txt, epub, kindle and other format this book has been release on 2014-09-18 with Science categories.
Nowadays bioinformaticians and geneticists are faced with myriad high-throughput data usually presenting the characteristics of uncertainty, high dimensionality and large complexity. These data will only allow insights into this wealth of so-called 'omics' data if represented by flexible and scalable models, prior to any further analysis. At the interface between statistics and machine learning, probabilistic graphical models (PGMs) represent a powerful formalism to discover complex networks of relations. These models are also amenable to incorporating a priori biological information. Network reconstruction from gene expression data represents perhaps the most emblematic area of research where PGMs have been successfully applied. However these models have also created renewed interest in genetics in the broad sense, in particular regarding association genetics, causality discovery, prediction of outcomes, detection of copy number variations, and epigenetics. This book provides an overview of the applications of PGMs to genetics, genomics and postgenomics to meet this increased interest. A salient feature of bioinformatics, interdisciplinarity, reaches its limit when an intricate cooperation between domain specialists is requested. Currently, few people are specialists in the design of advanced methods using probabilistic graphical models for postgenomics or genetics. This book deciphers such models so that their perceived difficulty no longer hinders their use and focuses on fifteen illustrations showing the mechanisms behind the models. Probabilistic Graphical Models for Genetics, Genomics and Postgenomics covers six main themes: (1) Gene network inference (2) Causality discovery (3) Association genetics (4) Epigenetics (5) Detection of copy number variations (6) Prediction of outcomes from high-dimensional genomic data. Written by leading international experts, this is a collection of the most advanced work at the crossroads of probabilistic graphical models and genetics, genomics, and postgenomics. The self-contained chapters provide an enlightened account of the pros and cons of applying these powerful techniques.
Introduction To Graphical Modelling
DOWNLOAD
Author : David Edwards
language : en
Publisher: Springer Science & Business Media
Release Date : 2012-12-06
Introduction To Graphical Modelling written by David Edwards and has been published by Springer Science & Business Media this book supported file pdf, txt, epub, kindle and other format this book has been release on 2012-12-06 with Mathematics categories.
A useful introduction to this topic for both students and researchers, with an emphasis on applications and practicalities rather than on a formal development. It is based on the popular software package for graphical modelling, MIM, freely available for downloading from the Internet. Following a description of some of the basic ideas of graphical modelling, subsequent chapters describe particular families of models, including log-linear models, Gaussian models, and models for mixed discrete and continuous variables. Further chapters cover hypothesis testing and model selection. Chapters 7 and 8 are new to this second edition and describe the use of directed, chain, and other graphs, complete with a summary of recent work on causal inference.
Learning From Data
DOWNLOAD
Author : Doug Fisher
language : en
Publisher: Springer Science & Business Media
Release Date : 1996-05-02
Learning From Data written by Doug Fisher and has been published by Springer Science & Business Media this book supported file pdf, txt, epub, kindle and other format this book has been release on 1996-05-02 with Computers categories.
This volume contains a revised collection of papers originally presented at the Fifth International Workshop on Artificial Intelligence and Statistics in 1995. The topics represented in this volume are diverse, and include natural language application causality and graphical models, classification, learning, knowledge discovery, and exploratory data analysis. The chapters illustrate the rich possibilities for interdisciplinary study at the interface of artificial intelligence and statistics. The chapters vary in the background that they assume, but moderate familiarity with techniques of artificial intelligence and statistics is desirable in most cases.
Statistical Analysis For High Dimensional Data
DOWNLOAD
Author : Arnoldo Frigessi
language : en
Publisher: Springer
Release Date : 2016-02-16
Statistical Analysis For High Dimensional Data written by Arnoldo Frigessi and has been published by Springer this book supported file pdf, txt, epub, kindle and other format this book has been release on 2016-02-16 with Mathematics categories.
This book features research contributions from The Abel Symposium on Statistical Analysis for High Dimensional Data, held in Nyvågar, Lofoten, Norway, in May 2014. The focus of the symposium was on statistical and machine learning methodologies specifically developed for inference in “big data” situations, with particular reference to genomic applications. The contributors, who are among the most prominent researchers on the theory of statistics for high dimensional inference, present new theories and methods, as well as challenging applications and computational solutions. Specific themes include, among others, variable selection and screening, penalised regression, sparsity, thresholding, low dimensional structures, computational challenges, non-convex situations, learning graphical models, sparse covariance and precision matrices, semi- and non-parametric formulations, multiple testing, classification, factor models, clustering, and preselection. Highlighting cutting-edge research and casting light on future research directions, the contributions will benefit graduate students and researchers in computational biology, statistics and the machine learning community.
Sparse Modeling For Image And Vision Processing
DOWNLOAD
Author : Julien Mairal
language : en
Publisher: Now Publishers
Release Date : 2014-12-19
Sparse Modeling For Image And Vision Processing written by Julien Mairal and has been published by Now Publishers this book supported file pdf, txt, epub, kindle and other format this book has been release on 2014-12-19 with Computers categories.
Sparse Modeling for Image and Vision Processing offers a self-contained view of sparse modeling for visual recognition and image processing. More specifically, it focuses on applications where the dictionary is learned and adapted to data, yielding a compact representation that has been successful in various contexts.
Probabilistic Boolean Networks
DOWNLOAD
Author : Ilya Shmulevich
language : en
Publisher: SIAM
Release Date : 2010-01-21
Probabilistic Boolean Networks written by Ilya Shmulevich and has been published by SIAM this book supported file pdf, txt, epub, kindle and other format this book has been release on 2010-01-21 with Mathematics categories.
The first comprehensive treatment of probabilistic Boolean networks, unifying different strands of current research and addressing emerging issues.
Protein Protein Interactions And Networks
DOWNLOAD
Author : Anna Panchenko
language : en
Publisher: Springer Science & Business Media
Release Date : 2010-04-06
Protein Protein Interactions And Networks written by Anna Panchenko and has been published by Springer Science & Business Media this book supported file pdf, txt, epub, kindle and other format this book has been release on 2010-04-06 with Science categories.
The biological interactions of living organisms, and protein-protein interactions in particular, are astonishingly diverse. This comprehensive book provides a broad, thorough and multidisciplinary coverage of its field. It integrates different approaches from bioinformatics, biochemistry, computational analysis and systems biology to offer the reader a comprehensive global view of the diverse data on protein-protein interactions and protein interaction networks.
Graphical Models Exponential Families And Variational Inference
DOWNLOAD
Author : Martin J. Wainwright
language : en
Publisher: Now Publishers Inc
Release Date : 2008
Graphical Models Exponential Families And Variational Inference written by Martin J. Wainwright and has been published by Now Publishers Inc this book supported file pdf, txt, epub, kindle and other format this book has been release on 2008 with Computers categories.
The core of this paper is a general set of variational principles for the problems of computing marginal probabilities and modes, applicable to multivariate statistical models in the exponential family.
Cladag 2017 Book Of Short Papers
DOWNLOAD
Author : Francesca Greselin
language : en
Publisher: Universitas Studiorum
Release Date : 2017-09-29
Cladag 2017 Book Of Short Papers written by Francesca Greselin and has been published by Universitas Studiorum this book supported file pdf, txt, epub, kindle and other format this book has been release on 2017-09-29 with Mathematics categories.
This book is the collection of the Abstract / Short Papers submitted by the authors of the International Conference of The CLAssification and Data Analysis Group (CLADAG) of the Italian Statistical Society (SIS), held in Milan (Italy) on September 13-15, 2017.