Sparsity In Machine Learning
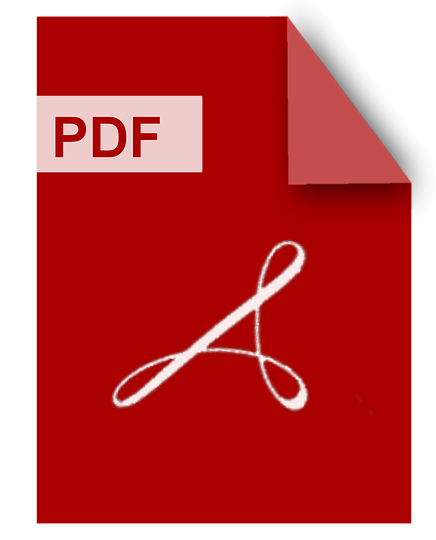
DOWNLOAD
Download Sparsity In Machine Learning PDF/ePub or read online books in Mobi eBooks. Click Download or Read Online button to get Sparsity In Machine Learning book now. This website allows unlimited access to, at the time of writing, more than 1.5 million titles, including hundreds of thousands of titles in various foreign languages. If the content not found or just blank you must refresh this page
Sparsity In Machine Learning
DOWNLOAD
Author : Siwei Feng
language : en
Publisher:
Release Date : 2019
Sparsity In Machine Learning written by Siwei Feng and has been published by this book supported file pdf, txt, epub, kindle and other format this book has been release on 2019 with categories.
Today we are living in a world awash with data. Large volumes of data are acquired, analyzed and applied to tasks through machine learning algorithms in nearly every area of science, business, and industry. For example, medical scientists analyze the gene expression data from a single specimen to learn the underlying causes of disease (e.g. cancer) and choose the best treatment; retailers can know more about customers' shopping habits from retail data to adjust their business strategies to better appeal to customers; suppliers can enhance supply chain success through supply chain systems built on knowledge sharing. However, it is also reasonable to doubt whether all the genes make contributions to a disease; whether all the data obtained from existing customers can be applied to a new customer; whether all shared knowledge in the supply network is useful to a specific supply scenario. Therefore, it is crucial to sort through the massive information provided by data and keep what we really need. This process is referred to as information selection, which keeps the information that helps improve the performance of corresponding machine learning tasks and discards information that is useless or even harmful to task performance. Sparse learning is a powerful tool to achieve information selection. In this thesis, we apply sparse learning to two major areas in machine learning -- feature selection and transfer learning. Feature selection is a dimensionality reduction technique that selects a subset of representative features. Recently, feature selection combined with sparse learning has attracted significant attention due to its outstanding performance compared with traditional feature selection methods that ignore correlation between features. However, they are restricted by design to linear data transformations, a potential drawback given that the underlying correlation structures of data are often non-linear. To leverage more sophisticated embedding than the linear model assumed by sparse learning, we propose an autoencoder-based unsupervised feature selection approach that leverages a single-layer autoencoder for a joint framework of feature selection and manifold learning. Additionally, we include spectral graph analysis on the projected data into the learning process to achieve local data geometry preservation from the original data space to the low-dimensional feature space. Transfer learning describes a set of methods that aim at transferring knowledge from related domains to alleviate the problems caused by limited/no labeled training data in machine learnig tasks. Many transfer learning techniques have been proposed to deal with different application scenarios. However, due to the differences in data distribution, feature space, label space, etc., between source domain and target domain, it is necessary to select and only transfer relevant information from source domain to improve the performance of target learner. Otherwise, the target learner can be negatively impacted by the weak-related knowledge from source domain, which is referred to as negative transfer. In this thesis, we focus on two transfer learning scenarios for which limited labeled training data are available in target domain. In the first scenario, no label information is avaible in source data. In the second scenario, large amounts of labeled source data are available, but there is no overlap between the source and target label spaces. The corresponding transfer learning technique to the former case is called \emph{self-taught learning}, while that for the latter case is called \emph{few-shot learning}. We apply self-taught learning to visual, textal, and audio data. We also apply few-shot learning to wearable sensor based human activity data. For both cases, we propose a metric for the relevance between a target sample/class and a source sample/class, and then extract information from the related samples/classes for knowledge transfer to perform information selection so that negative transfer caused by weakly related source information can be alleviated. Experimental results show that transfer learning can provide better performance with information selection.
Deep Learning Through Sparse And Low Rank Modeling
DOWNLOAD
Author : Zhangyang Wang
language : en
Publisher: Academic Press
Release Date : 2019-04-26
Deep Learning Through Sparse And Low Rank Modeling written by Zhangyang Wang and has been published by Academic Press this book supported file pdf, txt, epub, kindle and other format this book has been release on 2019-04-26 with Computers categories.
Deep Learning through Sparse Representation and Low-Rank Modeling bridges classical sparse and low rank models-those that emphasize problem-specific Interpretability-with recent deep network models that have enabled a larger learning capacity and better utilization of Big Data. It shows how the toolkit of deep learning is closely tied with the sparse/low rank methods and algorithms, providing a rich variety of theoretical and analytic tools to guide the design and interpretation of deep learning models. The development of the theory and models is supported by a wide variety of applications in computer vision, machine learning, signal processing, and data mining. This book will be highly useful for researchers, graduate students and practitioners working in the fields of computer vision, machine learning, signal processing, optimization and statistics. Combines classical sparse and low-rank models and algorithms with the latest advances in deep learning networks Shows how the structure and algorithms of sparse and low-rank methods improves the performance and interpretability of Deep Learning models Provides tactics on how to build and apply customized deep learning models for various applications
Sparse Modeling
DOWNLOAD
Author : Irina Rish
language : en
Publisher: CRC Press
Release Date : 2014-12-01
Sparse Modeling written by Irina Rish and has been published by CRC Press this book supported file pdf, txt, epub, kindle and other format this book has been release on 2014-12-01 with Business & Economics categories.
Sparse models are particularly useful in scientific applications, such as biomarker discovery in genetic or neuroimaging data, where the interpretability of a predictive model is essential. Sparsity can also dramatically improve the cost efficiency of signal processing. Sparse Modeling: Theory, Algorithms, and Applications provides an introduction to the growing field of sparse modeling, including application examples, problem formulations that yield sparse solutions, algorithms for finding such solutions, and recent theoretical results on sparse recovery. The book gets you up to speed on the latest sparsity-related developments and will motivate you to continue learning about the field. The authors first present motivating examples and a high-level survey of key recent developments in sparse modeling. The book then describes optimization problems involving commonly used sparsity-enforcing tools, presents essential theoretical results, and discusses several state-of-the-art algorithms for finding sparse solutions. The authors go on to address a variety of sparse recovery problems that extend the basic formulation to more sophisticated forms of structured sparsity and to different loss functions. They also examine a particular class of sparse graphical models and cover dictionary learning and sparse matrix factorizations.
Sparsity In Machine Learning
DOWNLOAD
Author : Driss Lahlou Kitane
language : en
Publisher:
Release Date : 2022
Sparsity In Machine Learning written by Driss Lahlou Kitane and has been published by this book supported file pdf, txt, epub, kindle and other format this book has been release on 2022 with categories.
Integer optimization is a highly effective tool in the conception of methods to tackle sparsity. It offers a rigorous framework to build sparse models and has proved to provide more accurate and sparse models than other approaches including the ones using sparsity-inducing regularization norms. This thesis focuses on the application of integer optimization to address sparsity problems.
Exploiting Sparsity For Machine Learning In Big Data
DOWNLOAD
Author : Rongda Zhu
language : en
Publisher:
Release Date : 2017
Exploiting Sparsity For Machine Learning In Big Data written by Rongda Zhu and has been published by this book supported file pdf, txt, epub, kindle and other format this book has been release on 2017 with categories.
Sparsity Methods For Systems And Control
DOWNLOAD
Author : Masaaki Nagahara
language : en
Publisher:
Release Date : 2020-09-30
Sparsity Methods For Systems And Control written by Masaaki Nagahara and has been published by this book supported file pdf, txt, epub, kindle and other format this book has been release on 2020-09-30 with categories.
The method of sparsity has been attracting a lot of attention in the fields related not only to signal processing, machine learning, and statistics, but also systems and control. The method is known as compressed sensing, compressive sampling, sparse representation, or sparse modeling. More recently, the sparsity method has been applied to systems and control to design resource-aware control systems. This book gives a comprehensive guide to sparsity methods for systems and control, from standard sparsity methods in finite-dimensional vector spaces (Part I) to optimal control methods in infinite-dimensional function spaces (Part II). The primary objective of this book is to show how to use sparsity methods for several engineering problems. For this, the author provides MATLAB programs by which the reader can try sparsity methods for themselves. Readers will obtain a deep understanding of sparsity methods by running these MATLAB programs. Sparsity Methods for Systems and Control is suitable for graduate level university courses, though it should also be comprehendible to undergraduate students who have a basic knowledge of linear algebra and elementary calculus. Also, especially part II of the book should appeal to professional researchers and engineers who are interested in applying sparsity methods to systems and control.
Statistical Learning With Sparsity
DOWNLOAD
Author : Trevor Hastie
language : en
Publisher: CRC Press
Release Date : 2015-05-07
Statistical Learning With Sparsity written by Trevor Hastie and has been published by CRC Press this book supported file pdf, txt, epub, kindle and other format this book has been release on 2015-05-07 with Business & Economics categories.
Discover New Methods for Dealing with High-Dimensional DataA sparse statistical model has only a small number of nonzero parameters or weights; therefore, it is much easier to estimate and interpret than a dense model. Statistical Learning with Sparsity: The Lasso and Generalizations presents methods that exploit sparsity to help recover the underl
Data Driven Science And Engineering
DOWNLOAD
Author : Steven L. Brunton
language : en
Publisher: Cambridge University Press
Release Date : 2022-05-05
Data Driven Science And Engineering written by Steven L. Brunton and has been published by Cambridge University Press this book supported file pdf, txt, epub, kindle and other format this book has been release on 2022-05-05 with Computers categories.
A textbook covering data-science and machine learning methods for modelling and control in engineering and science, with Python and MATLAB®.
Sparsity In Machine Learning
DOWNLOAD
Author : Zakria Hussain
language : en
Publisher:
Release Date : 2008
Sparsity In Machine Learning written by Zakria Hussain and has been published by this book supported file pdf, txt, epub, kindle and other format this book has been release on 2008 with categories.
Sparsity Prior In Efficient Deep Learning Based Solvers And Models
DOWNLOAD
Author : Xiaohan Chen
language : en
Publisher:
Release Date : 2022
Sparsity Prior In Efficient Deep Learning Based Solvers And Models written by Xiaohan Chen and has been published by this book supported file pdf, txt, epub, kindle and other format this book has been release on 2022 with categories.
Deep learning has been empirically successful in recent years thanks to the extremely over-parameterized deep models and the data-driven learning with enormous amounts of data. However, deep learning models are especially limited in terms of efficiency, which has two-fold meanings. Firstly, many deep models are designed in a black-box manner, which means these black-box models are unaware of the prior knowledge about the structure of the problems of interest and hence cannot efficiently utilize it. Such unawareness can cause redundancy in parameterization and inferior performance compared to more dedicated methods. Secondly, the extreme over-parameterization itself is inefficient in terms of model storage, memory requirements and computational complexity. This strictly constrains the realistic applications of deep learning on mobile devices with budget resources. Moreover, the financial and environmental costs of training such enormous deep models are unreasonably high, which is exactly the opposite of the call of green AI. In this work, we strive to address the inefficiency of deep models by introducing sparsity as an important prior knowledge to deep learning. Our efforts will be in three sub-directions. In the first direction, we aim at accelerating the solving process for a specific type of optimization problems with sparsity constraints. Instead of designing black-box deep learning models, we derive new parameterizations by absorbing insights from the sparse optimization field, which result in compact deep-learning-based solvers with significantly reduced training costs but superior empirical performance. In the second direction, we introduce sparsity to deep neural networks via weight pruning. Pruning reduces redundancy in over-parameterized deep networks by removing superfluous weights, thus naturally compressing the model storage and computational costs. We aim at pushing pruning to the limit by combining it with other compression techniques for extremely efficient deep models that can be deployed and fine-tuned on edge devices. In the third direction, we investigate what sparsity brings to deep networks. Creating sparsity in deep networks significantly changes the landscape of its loss function and thus behaviors during training. We aim at understanding what these changes are and how we can utilize them to train better sparse neural networks. The main content of this work can be summarized as below. Sparsity Prior in Efficient Deep Solvers. We adopt the algorithm unrolling method to transform classic optimization algorithms into feed-forward deep neural networks that can accelerate convergence by over 100x times. We also provide theoretical guarantees of linear convergence over the newly developed solvers, which is faster than the convergence rate achievable with classic optimization. Meanwhile, the number of parameters to be trained is reduced from millions to tens and even to 3 hyperparameters, decreasing the training time from hours to 6 minutes. Sparsity Prior in Efficient Deep Learning. We investigate compressing deep networks by unifying pruning, quantization and matrix factorization techniques to remove as much redundancy as possible, so that the resulting networks have low inference and/or training costs. The developed methods improve memory/storage efficiency and latency by at least 5x times, varying over data sets and models used. Sparsity Prior in Sparse Neural Networks. We discuss the properties and behaviors of sparse deep networks with the tool of lottery ticket hypothesis (LTH) and dynamic sparse training (DST) and explore their application for efficient training in computer vision, natural language processing and Internet-of-Things (IoT) systems. With our developed sparse neural networks, performance loss is significantly mitigated while by training much fewer parameters, bringing benefits of saving computation costs in general and communication costs specifically for IoT systems