Statistical Learning In Genetics
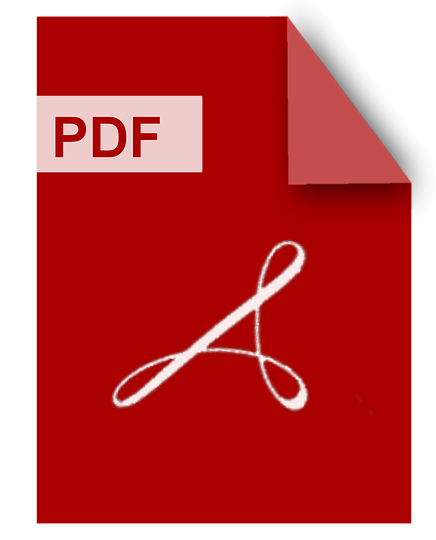
DOWNLOAD
Download Statistical Learning In Genetics PDF/ePub or read online books in Mobi eBooks. Click Download or Read Online button to get Statistical Learning In Genetics book now. This website allows unlimited access to, at the time of writing, more than 1.5 million titles, including hundreds of thousands of titles in various foreign languages. If the content not found or just blank you must refresh this page
Statistical Learning In Genetics
DOWNLOAD
Author : Daniel Sorensen
language : en
Publisher: Springer Nature
Release Date : 2023-09-19
Statistical Learning In Genetics written by Daniel Sorensen and has been published by Springer Nature this book supported file pdf, txt, epub, kindle and other format this book has been release on 2023-09-19 with Mathematics categories.
This book provides an introduction to computer-based methods for the analysis of genomic data. Breakthroughs in molecular and computational biology have contributed to the emergence of vast data sets, where millions of genetic markers for each individual are coupled with medical records, generating an unparalleled resource for linking human genetic variation to human biology and disease. Similar developments have taken place in animal and plant breeding, where genetic marker information is combined with production traits. An important task for the statistical geneticist is to adapt, construct and implement models that can extract information from these large-scale data. An initial step is to understand the methodology that underlies the probability models and to learn the modern computer-intensive methods required for fitting these models. The objective of this book, suitable for readers who wish to develop analytic skills to perform genomic research, is to provide guidance to take this first step. This book is addressed to numerate biologists who typically lack the formal mathematical background of the professional statistician. For this reason, considerably more detail in explanations and derivations is offered. It is written in a concise style and examples are used profusely. A large proportion of the examples involve programming with the open-source package R. The R code needed to solve the exercises is provided. The MarkDown interface allows the students to implement the code on their own computer, contributing to a better understanding of the underlying theory. Part I presents methods of inference based on likelihood and Bayesian methods, including computational techniques for fitting likelihood and Bayesian models. Part II discusses prediction for continuous and binary data using both frequentist and Bayesian approaches. Some of the models used for prediction are also used for gene discovery. The challenge is to find promising genes without incurring a large proportion of false positive results. Therefore, Part II includes a detour on False Discovery Rate assuming frequentist and Bayesian perspectives. The last chapter of Part II provides an overview of a selected number of non-parametric methods. Part III consists of exercises and their solutions. Daniel Sorensen holds PhD and DSc degrees from the University of Edinburgh and is an elected Fellow of the American Statistical Association. He was professor of Statistical Genetics at Aarhus University where, at present, he is professor emeritus.
Multivariate Statistical Machine Learning Methods For Genomic Prediction
DOWNLOAD
Author : Osval Antonio Montesinos López
language : en
Publisher: Springer Nature
Release Date : 2022-02-14
Multivariate Statistical Machine Learning Methods For Genomic Prediction written by Osval Antonio Montesinos López and has been published by Springer Nature this book supported file pdf, txt, epub, kindle and other format this book has been release on 2022-02-14 with Technology & Engineering categories.
This book is open access under a CC BY 4.0 license This open access book brings together the latest genome base prediction models currently being used by statisticians, breeders and data scientists. It provides an accessible way to understand the theory behind each statistical learning tool, the required pre-processing, the basics of model building, how to train statistical learning methods, the basic R scripts needed to implement each statistical learning tool, and the output of each tool. To do so, for each tool the book provides background theory, some elements of the R statistical software for its implementation, the conceptual underpinnings, and at least two illustrative examples with data from real-world genomic selection experiments. Lastly, worked-out examples help readers check their own comprehension.The book will greatly appeal to readers in plant (and animal) breeding, geneticists and statisticians, as it provides in a very accessible way the necessary theory, the appropriate R code, and illustrative examples for a complete understanding of each statistical learning tool. In addition, it weighs the advantages and disadvantages of each tool.
Machine Learning In Genome Wide Association Studies
DOWNLOAD
Author : Ting Hu
language : en
Publisher: Frontiers Media SA
Release Date : 2020-12-15
Machine Learning In Genome Wide Association Studies written by Ting Hu and has been published by Frontiers Media SA this book supported file pdf, txt, epub, kindle and other format this book has been release on 2020-12-15 with Science categories.
This eBook is a collection of articles from a Frontiers Research Topic. Frontiers Research Topics are very popular trademarks of the Frontiers Journals Series: they are collections of at least ten articles, all centered on a particular subject. With their unique mix of varied contributions from Original Research to Review Articles, Frontiers Research Topics unify the most influential researchers, the latest key findings and historical advances in a hot research area! Find out more on how to host your own Frontiers Research Topic or contribute to one as an author by contacting the Frontiers Editorial Office: frontiersin.org/about/contact.
Statistical Modeling And Machine Learning For Molecular Biology
DOWNLOAD
Author : Alan Moses
language : en
Publisher: CRC Press
Release Date : 2017-01-06
Statistical Modeling And Machine Learning For Molecular Biology written by Alan Moses and has been published by CRC Press this book supported file pdf, txt, epub, kindle and other format this book has been release on 2017-01-06 with Computers categories.
• Assumes no background in statistics or computers • Covers most major types of molecular biological data • Covers the statistical and machine learning concepts of most practical utility (P-values, clustering, regression, regularization and classification) • Intended for graduate students beginning careers in molecular biology, systems biology, bioengineering and genetics
A Statistical Approach To Genetic Epidemiology
DOWNLOAD
Author : Andreas Ziegler
language : en
Publisher: John Wiley & Sons
Release Date : 2011-08-24
A Statistical Approach To Genetic Epidemiology written by Andreas Ziegler and has been published by John Wiley & Sons this book supported file pdf, txt, epub, kindle and other format this book has been release on 2011-08-24 with Science categories.
A Statistical Approach to Genetic Epidemiology After studying statistics and mathematics at the University of Munich and obtaining his doctoral degree from the University of Dortmund, Andreas Ziegler received the Johann-Peter-Süssmilch-Medal of the German Association for Medical Informatics, Biometry and Epidemiology for his post-doctoral work on “Model Free Linkage Analysis of Quantitative Traits” in 1999. In 2004, he was one of the recipients of the Fritz-Linder-Forum-Award from the German Association for Surgery.
The Fundamentals Of Modern Statistical Genetics
DOWNLOAD
Author : Nan M. Laird
language : en
Publisher: Springer Science & Business Media
Release Date : 2010-12-13
The Fundamentals Of Modern Statistical Genetics written by Nan M. Laird and has been published by Springer Science & Business Media this book supported file pdf, txt, epub, kindle and other format this book has been release on 2010-12-13 with Medical categories.
This book covers the statistical models and methods that are used to understand human genetics, following the historical and recent developments of human genetics. Starting with Mendel’s first experiments to genome-wide association studies, the book describes how genetic information can be incorporated into statistical models to discover disease genes. All commonly used approaches in statistical genetics (e.g. aggregation analysis, segregation, linkage analysis, etc), are used, but the focus of the book is modern approaches to association analysis. Numerous examples illustrate key points throughout the text, both of Mendelian and complex genetic disorders. The intended audience is statisticians, biostatisticians, epidemiologists and quantitatively- oriented geneticists and health scientists wanting to learn about statistical methods for genetic analysis, whether to better analyze genetic data, or to pursue research in methodology. A background in intermediate level statistical methods is required. The authors include few mathematical derivations, and the exercises provide problems for students with a broad range of skill levels. No background in genetics is assumed.
Gene Expression Data Analysis
DOWNLOAD
Author : Pankaj Barah
language : en
Publisher: Chapman & Hall/CRC
Release Date : 2021-08
Gene Expression Data Analysis written by Pankaj Barah and has been published by Chapman & Hall/CRC this book supported file pdf, txt, epub, kindle and other format this book has been release on 2021-08 with Gene expression categories.
"The book introduces phenomenal growth of data generated by increasing numbers of genome sequencing projects and other throughput technology-led experimental efforts. It provides information about various sources of gene expression data, and pre-processing, analysis, and validation of such data"--
Computational Genomics With R
DOWNLOAD
Author : Altuna Akalin
language : en
Publisher: CRC Press
Release Date : 2020-12-16
Computational Genomics With R written by Altuna Akalin and has been published by CRC Press this book supported file pdf, txt, epub, kindle and other format this book has been release on 2020-12-16 with Mathematics categories.
Computational Genomics with R provides a starting point for beginners in genomic data analysis and also guides more advanced practitioners to sophisticated data analysis techniques in genomics. The book covers topics from R programming, to machine learning and statistics, to the latest genomic data analysis techniques. The text provides accessible information and explanations, always with the genomics context in the background. This also contains practical and well-documented examples in R so readers can analyze their data by simply reusing the code presented. As the field of computational genomics is interdisciplinary, it requires different starting points for people with different backgrounds. For example, a biologist might skip sections on basic genome biology and start with R programming, whereas a computer scientist might want to start with genome biology. After reading: You will have the basics of R and be able to dive right into specialized uses of R for computational genomics such as using Bioconductor packages. You will be familiar with statistics, supervised and unsupervised learning techniques that are important in data modeling, and exploratory analysis of high-dimensional data. You will understand genomic intervals and operations on them that are used for tasks such as aligned read counting and genomic feature annotation. You will know the basics of processing and quality checking high-throughput sequencing data. You will be able to do sequence analysis, such as calculating GC content for parts of a genome or finding transcription factor binding sites. You will know about visualization techniques used in genomics, such as heatmaps, meta-gene plots, and genomic track visualization. You will be familiar with analysis of different high-throughput sequencing data sets, such as RNA-seq, ChIP-seq, and BS-seq. You will know basic techniques for integrating and interpreting multi-omics datasets. Altuna Akalin is a group leader and head of the Bioinformatics and Omics Data Science Platform at the Berlin Institute of Medical Systems Biology, Max Delbrück Center, Berlin. He has been developing computational methods for analyzing and integrating large-scale genomics data sets since 2002. He has published an extensive body of work in this area. The framework for this book grew out of the yearly computational genomics courses he has been organizing and teaching since 2015.
Computational And Statistical Approaches To Genomics
DOWNLOAD
Author : Wei Zhang
language : en
Publisher: Springer Science & Business Media
Release Date : 2007-05-08
Computational And Statistical Approaches To Genomics written by Wei Zhang and has been published by Springer Science & Business Media this book supported file pdf, txt, epub, kindle and other format this book has been release on 2007-05-08 with Science categories.
Computational and Statistical Genomics aims to help researchers deal with current genomic challenges. Topics covered include: overviews of the role of supercomputers in genomics research, the existing challenges and directions in image processing for microarray technology, and web-based tools for microarray data analysis; approaches to the global modeling and analysis of gene regulatory networks and transcriptional control, using methods, theories, and tools from signal processing, machine learning, information theory, and control theory; state-of-the-art tools in Boolean function theory, time-frequency analysis, pattern recognition, and unsupervised learning, applied to cancer classification, identification of biologically active sites, and visualization of gene expression data; crucial issues associated with statistical analysis of microarray data, statistics and stochastic analysis of gene expression levels in a single cell, statistically sound design of microarray studies and experiments; and biological and medical implications of genomics research.
An Introduction To Genetic Algorithms
DOWNLOAD
Author : Melanie Mitchell
language : en
Publisher: MIT Press
Release Date : 1998-03-02
An Introduction To Genetic Algorithms written by Melanie Mitchell and has been published by MIT Press this book supported file pdf, txt, epub, kindle and other format this book has been release on 1998-03-02 with Computers categories.
Genetic algorithms have been used in science and engineering as adaptive algorithms for solving practical problems and as computational models of natural evolutionary systems. This brief, accessible introduction describes some of the most interesting research in the field and also enables readers to implement and experiment with genetic algorithms on their own. It focuses in depth on a small set of important and interesting topics—particularly in machine learning, scientific modeling, and artificial life—and reviews a broad span of research, including the work of Mitchell and her colleagues. The descriptions of applications and modeling projects stretch beyond the strict boundaries of computer science to include dynamical systems theory, game theory, molecular biology, ecology, evolutionary biology, and population genetics, underscoring the exciting "general purpose" nature of genetic algorithms as search methods that can be employed across disciplines. An Introduction to Genetic Algorithms is accessible to students and researchers in any scientific discipline. It includes many thought and computer exercises that build on and reinforce the reader's understanding of the text. The first chapter introduces genetic algorithms and their terminology and describes two provocative applications in detail. The second and third chapters look at the use of genetic algorithms in machine learning (computer programs, data analysis and prediction, neural networks) and in scientific models (interactions among learning, evolution, and culture; sexual selection; ecosystems; evolutionary activity). Several approaches to the theory of genetic algorithms are discussed in depth in the fourth chapter. The fifth chapter takes up implementation, and the last chapter poses some currently unanswered questions and surveys prospects for the future of evolutionary computation.