Statistical Model Selection And Prediction For Non Standard Data
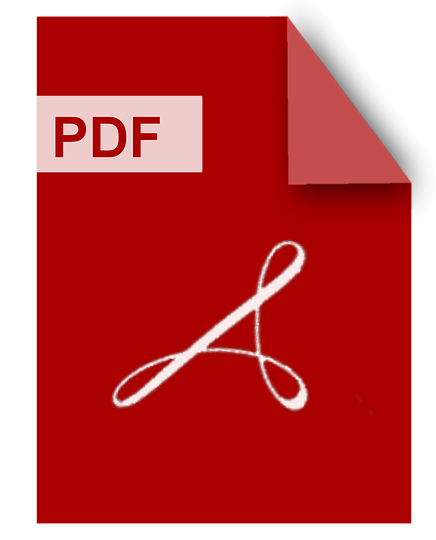
DOWNLOAD
Download Statistical Model Selection And Prediction For Non Standard Data PDF/ePub or read online books in Mobi eBooks. Click Download or Read Online button to get Statistical Model Selection And Prediction For Non Standard Data book now. This website allows unlimited access to, at the time of writing, more than 1.5 million titles, including hundreds of thousands of titles in various foreign languages. If the content not found or just blank you must refresh this page
Statistical Model Selection And Prediction For Non Standard Data
DOWNLOAD
Author : Konstantin Görgen
language : en
Publisher:
Release Date : 2022*
Statistical Model Selection And Prediction For Non Standard Data written by Konstantin Görgen and has been published by this book supported file pdf, txt, epub, kindle and other format this book has been release on 2022* with categories.
Practical Statistics For Data Scientists
DOWNLOAD
Author : Peter Bruce
language : en
Publisher: "O'Reilly Media, Inc."
Release Date : 2017-05-10
Practical Statistics For Data Scientists written by Peter Bruce and has been published by "O'Reilly Media, Inc." this book supported file pdf, txt, epub, kindle and other format this book has been release on 2017-05-10 with Computers categories.
Statistical methods are a key part of of data science, yet very few data scientists have any formal statistics training. Courses and books on basic statistics rarely cover the topic from a data science perspective. This practical guide explains how to apply various statistical methods to data science, tells you how to avoid their misuse, and gives you advice on what's important and what's not. Many data science resources incorporate statistical methods but lack a deeper statistical perspective. If you’re familiar with the R programming language, and have some exposure to statistics, this quick reference bridges the gap in an accessible, readable format. With this book, you’ll learn: Why exploratory data analysis is a key preliminary step in data science How random sampling can reduce bias and yield a higher quality dataset, even with big data How the principles of experimental design yield definitive answers to questions How to use regression to estimate outcomes and detect anomalies Key classification techniques for predicting which categories a record belongs to Statistical machine learning methods that “learn” from data Unsupervised learning methods for extracting meaning from unlabeled data
Computer Intensive Statistical Methods
DOWNLOAD
Author : J. S. Urban. Hjorth
language : en
Publisher: CRC Press
Release Date : 2017-10-19
Computer Intensive Statistical Methods written by J. S. Urban. Hjorth and has been published by CRC Press this book supported file pdf, txt, epub, kindle and other format this book has been release on 2017-10-19 with Mathematics categories.
This book focuses on computer intensive statistical methods, such as validation, model selection, and bootstrap, that help overcome obstacles that could not be previously solved by methods such as regression and time series modelling in the areas of economics, meteorology, and transportation.
Fundamentals Of Clinical Data Science
DOWNLOAD
Author : Pieter Kubben
language : en
Publisher: Springer
Release Date : 2018-12-21
Fundamentals Of Clinical Data Science written by Pieter Kubben and has been published by Springer this book supported file pdf, txt, epub, kindle and other format this book has been release on 2018-12-21 with Medical categories.
This open access book comprehensively covers the fundamentals of clinical data science, focusing on data collection, modelling and clinical applications. Topics covered in the first section on data collection include: data sources, data at scale (big data), data stewardship (FAIR data) and related privacy concerns. Aspects of predictive modelling using techniques such as classification, regression or clustering, and prediction model validation will be covered in the second section. The third section covers aspects of (mobile) clinical decision support systems, operational excellence and value-based healthcare. Fundamentals of Clinical Data Science is an essential resource for healthcare professionals and IT consultants intending to develop and refine their skills in personalized medicine, using solutions based on large datasets from electronic health records or telemonitoring programmes. The book’s promise is “no math, no code”and will explain the topics in a style that is optimized for a healthcare audience.
Traditional And Data Driven Predictive Statistical Models
DOWNLOAD
Author : Dr. Neeta Kishor Dhane
language : en
Publisher: Laxmi Book Publication
Release Date : 2021-07-23
Traditional And Data Driven Predictive Statistical Models written by Dr. Neeta Kishor Dhane and has been published by Laxmi Book Publication this book supported file pdf, txt, epub, kindle and other format this book has been release on 2021-07-23 with Art categories.
The desire to know the unknown has always been one of the human characteristics that distinguish humans from other living things on the earth. The past is known but cannot be changed, and hence is if no interest. The present is happening and everyone is witnessing it and therefore it is not exciting. But the future is both unknown and perhaps therefore uncertain, and is therefore both interesting and exciting. Using past experience for predicting the unknown future was initially treated as an art because it require careful choice of parts of the past that will make prediction both easy and accurate, and there were times when it was felt that it is impossible to formulate a method for this. Prediction was then not considered to be scientific empirical sciences that learn from scientist and professionals realized the scientific nature of the ability to predict. What then began as the preparation for developing a prediction formula involved finding common patterns in past data and their consequences so that the consequence can be predicted as soon as the relevant pattern is observed. At the same time the discipline of statistics developed the concept and methodology for building statistical models. With experience in the development and applications of different models, scientists and researchers identify models as belonging to four different classes namely, the class of descriptive models, the class of diagnostic models, the class of predictive models, and the class of prescriptive or prognostic models. The scientific or theoretical activity of building models and analyzing data accordingly is known as analytics. It has therefore been recognized that there are four classes of analytics, namely descriptive analytics, diagnostic analytics, prescriptive analytics and predictive analytics. These four classes are defined briefly for convenience of the reader.
Statistical Learning For Biomedical Data
DOWNLOAD
Author : James D. Malley
language : en
Publisher: Cambridge University Press
Release Date : 2011-02-24
Statistical Learning For Biomedical Data written by James D. Malley and has been published by Cambridge University Press this book supported file pdf, txt, epub, kindle and other format this book has been release on 2011-02-24 with Medical categories.
This book is for anyone who has biomedical data and needs to identify variables that predict an outcome, for two-group outcomes such as tumor/not-tumor, survival/death, or response from treatment. Statistical learning machines are ideally suited to these types of prediction problems, especially if the variables being studied may not meet the assumptions of traditional techniques. Learning machines come from the world of probability and computer science but are not yet widely used in biomedical research. This introduction brings learning machine techniques to the biomedical world in an accessible way, explaining the underlying principles in nontechnical language and using extensive examples and figures. The authors connect these new methods to familiar techniques by showing how to use the learning machine models to generate smaller, more easily interpretable traditional models. Coverage includes single decision trees, multiple-tree techniques such as Random ForestsTM, neural nets, support vector machines, nearest neighbors and boosting.
Machine Learning Toolbox For Social Scientists
DOWNLOAD
Author : Yigit Aydede
language : en
Publisher: CRC Press
Release Date : 2023-09-22
Machine Learning Toolbox For Social Scientists written by Yigit Aydede and has been published by CRC Press this book supported file pdf, txt, epub, kindle and other format this book has been release on 2023-09-22 with Computers categories.
Machine Learning Toolbox for Social Scientists covers predictive methods with complementary statistical "tools" that make it mostly self-contained. The inferential statistics is the traditional framework for most data analytics courses in social science and business fields, especially in Economics and Finance. The new organization that this book offers goes beyond standard machine learning code applications, providing intuitive backgrounds for new predictive methods that social science and business students can follow. The book also adds many other modern statistical tools complementary to predictive methods that cannot be easily found in "econometrics" textbooks: nonparametric methods, data exploration with predictive models, penalized regressions, model selection with sparsity, dimension reduction methods, nonparametric time-series predictions, graphical network analysis, algorithmic optimization methods, classification with imbalanced data, and many others. This book is targeted at students and researchers who have no advanced statistical background, but instead coming from the tradition of "inferential statistics". The modern statistical methods the book provides allows it to be effectively used in teaching in the social science and business fields. Key Features: The book is structured for those who have been trained in a traditional statistics curriculum. There is one long initial section that covers the differences in "estimation" and "prediction" for people trained for causal analysis. The book develops a background framework for Machine learning applications from Nonparametric methods. SVM and NN simple enough without too much detail. It’s self-sufficient. Nonparametric time-series predictions are new and covered in a separate section. Additional sections are added: Penalized Regressions, Dimension Reduction Methods, and Graphical Methods have been increasing in their popularity in social sciences.
Selecting Models From Data
DOWNLOAD
Author : P. Cheeseman
language : en
Publisher: Springer Science & Business Media
Release Date : 2012-12-06
Selecting Models From Data written by P. Cheeseman and has been published by Springer Science & Business Media this book supported file pdf, txt, epub, kindle and other format this book has been release on 2012-12-06 with Mathematics categories.
This volume is a selection of papers presented at the Fourth International Workshop on Artificial Intelligence and Statistics held in January 1993. These biennial workshops have succeeded in bringing together researchers from Artificial Intelligence and from Statistics to discuss problems of mutual interest. The exchange has broadened research in both fields and has strongly encour aged interdisciplinary work. The theme ofthe 1993 AI and Statistics workshop was: "Selecting Models from Data". The papers in this volume attest to the diversity of approaches to model selection and to the ubiquity of the problem. Both statistics and artificial intelligence have independently developed approaches to model selection and the corresponding algorithms to implement them. But as these papers make clear, there is a high degree of overlap between the different approaches. In particular, there is agreement that the fundamental problem is the avoidence of "overfitting"-Le., where a model fits the given data very closely, but is a poor predictor for new data; in other words, the model has partly fitted the "noise" in the original data.
Frontiers In Massive Data Analysis
DOWNLOAD
Author : National Research Council
language : en
Publisher: National Academies Press
Release Date : 2013-09-03
Frontiers In Massive Data Analysis written by National Research Council and has been published by National Academies Press this book supported file pdf, txt, epub, kindle and other format this book has been release on 2013-09-03 with Mathematics categories.
Data mining of massive data sets is transforming the way we think about crisis response, marketing, entertainment, cybersecurity and national intelligence. Collections of documents, images, videos, and networks are being thought of not merely as bit strings to be stored, indexed, and retrieved, but as potential sources of discovery and knowledge, requiring sophisticated analysis techniques that go far beyond classical indexing and keyword counting, aiming to find relational and semantic interpretations of the phenomena underlying the data. Frontiers in Massive Data Analysis examines the frontier of analyzing massive amounts of data, whether in a static database or streaming through a system. Data at that scale-terabytes and petabytes-is increasingly common in science (e.g., particle physics, remote sensing, genomics), Internet commerce, business analytics, national security, communications, and elsewhere. The tools that work to infer knowledge from data at smaller scales do not necessarily work, or work well, at such massive scale. New tools, skills, and approaches are necessary, and this report identifies many of them, plus promising research directions to explore. Frontiers in Massive Data Analysis discusses pitfalls in trying to infer knowledge from massive data, and it characterizes seven major classes of computation that are common in the analysis of massive data. Overall, this report illustrates the cross-disciplinary knowledge-from computer science, statistics, machine learning, and application disciplines-that must be brought to bear to make useful inferences from massive data.
Clinical Prediction Models
DOWNLOAD
Author : Ewout W. Steyerberg
language : en
Publisher: Springer Science & Business Media
Release Date : 2008-12-16
Clinical Prediction Models written by Ewout W. Steyerberg and has been published by Springer Science & Business Media this book supported file pdf, txt, epub, kindle and other format this book has been release on 2008-12-16 with Medical categories.
Prediction models are important in various fields, including medicine, physics, meteorology, and finance. Prediction models will become more relevant in the medical field with the increase in knowledge on potential predictors of outcome, e.g. from genetics. Also, the number of applications will increase, e.g. with targeted early detection of disease, and individualized approaches to diagnostic testing and treatment. The current era of evidence-based medicine asks for an individualized approach to medical decision-making. Evidence-based medicine has a central place for meta-analysis to summarize results from randomized controlled trials; similarly prediction models may summarize the effects of predictors to provide individu- ized predictions of a diagnostic or prognostic outcome. Why Read This Book? My motivation for working on this book stems primarily from the fact that the development and applications of prediction models are often suboptimal in medical publications. With this book I hope to contribute to better understanding of relevant issues and give practical advice on better modelling strategies than are nowadays widely used. Issues include: (a) Better predictive modelling is sometimes easily possible; e.g. a large data set with high quality data is available, but all continuous predictors are dich- omized, which is known to have several disadvantages.