Why Machines Learn
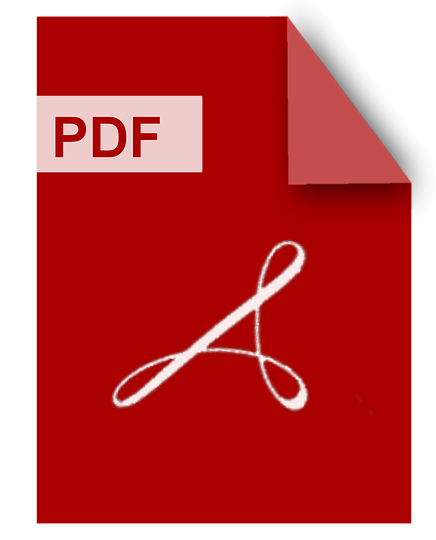
DOWNLOAD
Download Why Machines Learn PDF/ePub or read online books in Mobi eBooks. Click Download or Read Online button to get Why Machines Learn book now. This website allows unlimited access to, at the time of writing, more than 1.5 million titles, including hundreds of thousands of titles in various foreign languages. If the content not found or just blank you must refresh this page
Why Machines Learn
DOWNLOAD
Author : Anil Ananthaswamy
language : en
Publisher: Penguin
Release Date : 2024-07-16
Why Machines Learn written by Anil Ananthaswamy and has been published by Penguin this book supported file pdf, txt, epub, kindle and other format this book has been release on 2024-07-16 with Computers categories.
A rich, narrative explanation of the mathematics that has brought us machine learning and the ongoing explosion of artificial intelligence Machine learning systems are making life-altering decisions for us: approving mortgage loans, determining whether a tumour is cancerous, or deciding whether someone gets bail. They now influence developments and discoveries in chemistry, biology, and physics—the study of genomes, extra-solar planets, even the intricacies of quantum systems. And all this before large language models such as ChatGPT came on the scene. We are living through a revolution in machine learning-powered AI that shows no signs of slowing down. This technology is based on relatively simple mathematical ideas, some of which go back centuries, including linear algebra and calculus, the stuff of seventeenth- and eighteenth-century mathematics. It took the birth and advancement of computer science and the kindling of 1990s computer chips designed for video games to ignite the explosion of AI that we see today. In this enlightening book, Anil Ananthaswamy explains the fundamental math behind machine learning, while suggesting intriguing links between artifical and natural intelligence. Might the same math underpin them both? As Ananthaswamy resonantly concludes, to make safe and effective use of artificial intelligence, we need to understand its profound capabilities and limitations, the clues to which lie in the math that makes machine learning possible.
Why Machines Learn
DOWNLOAD
Author : Anil Ananthaswamy
language : en
Publisher: Random House
Release Date : 2024-07-16
Why Machines Learn written by Anil Ananthaswamy and has been published by Random House this book supported file pdf, txt, epub, kindle and other format this book has been release on 2024-07-16 with Computers categories.
'An invaluable companion for anyone who wants a deep understanding of what’s under the hood of often inscrutable machines' Melanie Mitchell A rich, narrative explanation of the mathematics that has brought us machine learning and the ongoing explosion of artificial intelligence Machine-learning systems are making life-altering decisions for us: approving mortgage loans, determining whether a tumour is cancerous, or deciding whether someone gets bail. They now influence discoveries in chemistry, biology and physics - the study of genomes, extra-solar planets, even the intricacies of quantum systems. We are living through a revolution in artificial intelligence that is not slowing down. This major shift is based on simple mathematics, some of which goes back centuries: linear algebra and calculus, the stuff of eighteenth-century mathematics. Indeed by the mid-1850s, a lot of the groundwork was all done. It took the development of computer science and the kindling of 1990s computer chips designed for video games to ignite the explosion of AI that we see all around us today. In this enlightening book, Anil Ananthaswamy explains the fundamental maths behind AI, which suggests that the basics of natural and artificial intelligence might follow the same mathematical rules. As Ananthaswamy resonantly concludes, to make the most of our most wondrous technologies we need to understand their profound limitations - the clues lie in the maths that makes AI possible.
Machine Learning For Beginners
DOWNLOAD
Author : Steven Cooper
language : en
Publisher: Roland Bind
Release Date : 2018-09-07
Machine Learning For Beginners written by Steven Cooper and has been published by Roland Bind this book supported file pdf, txt, epub, kindle and other format this book has been release on 2018-09-07 with Computers categories.
If you are looking for a complete beginners guide to learn machine learning with examples, in just a few hours, then you need to continue reading. Machine learning is an incredibly dense topic. It's hard to imagine condensing it into an easily readable and digestible format. However, this book aims to do exactly that. ★★ Grab your copy today and learn ★★ ♦ The different types of learning algorithm that you can expect to encounter ♦ The numerous applications of machine learning ♦ The different types of machine learning and how they differ ♦ The best practices for picking up machine learning ♦ What languages and libraries to work with ♦ The future of machine learning ♦ The various problems that you can solve with machine learning algorithms ♦ And much more... Starting from nothing, we slowly work our way through all the concepts that are central to machine learning. By the end of this book, you're going to feel as though you have an extremely firm understanding of what machine learning is, how it can be used, and most importantly, how it can change the world. You're also going to have an understanding of the logic behind the algorithms and what they aim to accomplish. Don't waste your time working with a book that's only going to make an already complicated topic even more complicated. Scroll up and click the buy now button to learn everything you need to know about Machine Learning!
Artificial Intelligence For Business
DOWNLOAD
Author : Doug Rose
language : en
Publisher: FT Press
Release Date : 2020-12-09
Artificial Intelligence For Business written by Doug Rose and has been published by FT Press this book supported file pdf, txt, epub, kindle and other format this book has been release on 2020-12-09 with Business & Economics categories.
The Easy Introduction to Machine Learning (Ml) for Nontechnical People--In Business and Beyond Artificial Intelligence for Business is your plain-English guide to Artificial Intelligence (AI) and Machine Learning (ML): how they work, what they can and cannot do, and how to start profiting from them. Writing for nontechnical executives and professionals, Doug Rose demystifies AI/ML technology with intuitive analogies and explanations honed through years of teaching and consulting. Rose explains everything from early “expert systems” to advanced deep learning networks. First, Rose explains how AI and ML emerged, exploring pivotal early ideas that continue to influence the field. Next, he deepens your understanding of key ML concepts, showing how machines can create strategies and learn from mistakes. Then, Rose introduces current powerful neural networks: systems inspired by the structure and function of the human brain. He concludes by introducing leading AI applications, from automated customer interactions to event prediction. Throughout, Rose stays focused on business: applying these technologies to leverage new opportunities and solve real problems. Compare the ways a machine can learn, and explore current leading ML algorithms Start with the right problems, and avoid common AI/ML project mistakes Use neural networks to automate decision-making and identify unexpected patterns Help neural networks learn more quickly and effectively Harness AI chatbots, virtual assistants, virtual agents, and conversational AI applications
Exploring Machine Learning A Beginners Perspective
DOWNLOAD
Author : Dr. Raghuram Bhukya
language : en
Publisher: Horizon Books ( A Division of Ignited Minds Edutech P Ltd)
Release Date : 2021-04-20
Exploring Machine Learning A Beginners Perspective written by Dr. Raghuram Bhukya and has been published by Horizon Books ( A Division of Ignited Minds Edutech P Ltd) this book supported file pdf, txt, epub, kindle and other format this book has been release on 2021-04-20 with Computers categories.
Machine learning is a field of Artificial intelligence that provides algorithms those can learn and improve from experiences. Machine learning algorithms are turned as integral parts of today’s digital life. Its applications include recommender systems, targeted campaigns, text categorization, computer vision and auto security systems etc. Machine learning also considered as essential part of data science due to its capability of providing predictive analytics, capability in handling variety of data and suitability for big data applications. Its capability for predictive analytics resulted of its general structure that is building statistical models out of training data. In other hand easy scalability advantage of machine learning algorithms is making them to be suitable for big data applications. The different types of learning algorithms includes supervised learning, unsupervised learning, reinforcement learning, feature learning, rule based learning, Robot or expert system learning, sparse dictionary and anomaly detection. These learning algorithms can be realized by computing models artificial neural networks, decision trees, support vector machines, regression analysis, Bayesian networks, Genetic algorithms and soft computing. The familiar tools to implement machine learning algorithms include Python, R, Matlab, Scala, Clojure and Ruby. Involving of such open source programming languages, tools and social network communities makes the machine learning most progressing filed of computer science. The machine learning life cycle includes defining project objectives, explore the types and format, modeling data to fit for machine learning algorithms, deciding suitable machine learning model and implement and decide best result from data for decision making. These days, machine learning is observing great interest by the society and it has turned as one of the significant responsibility of top level managers to transform their business in the profitable means by exploring its basic functionalities. The world is at the sheer of realizing a situation where machines will work in agreement with human being to work together, operation, and advertise their services in a novel way which is targeted, valuable, and well-versed. In order to achieve this, they can influence machine learning distinctiveness. Dr. Raghuram Bhukya
Grokking Deep Learning
DOWNLOAD
Author : Andrew W. Trask
language : en
Publisher: Simon and Schuster
Release Date : 2019-01-23
Grokking Deep Learning written by Andrew W. Trask and has been published by Simon and Schuster this book supported file pdf, txt, epub, kindle and other format this book has been release on 2019-01-23 with Computers categories.
Summary Grokking Deep Learning teaches you to build deep learning neural networks from scratch! In his engaging style, seasoned deep learning expert Andrew Trask shows you the science under the hood, so you grok for yourself every detail of training neural networks. Purchase of the print book includes a free eBook in PDF, Kindle, and ePub formats from Manning Publications. About the Technology Deep learning, a branch of artificial intelligence, teaches computers to learn by using neural networks, technology inspired by the human brain. Online text translation, self-driving cars, personalized product recommendations, and virtual voice assistants are just a few of the exciting modern advancements possible thanks to deep learning. About the Book Grokking Deep Learning teaches you to build deep learning neural networks from scratch! In his engaging style, seasoned deep learning expert Andrew Trask shows you the science under the hood, so you grok for yourself every detail of training neural networks. Using only Python and its math-supporting library, NumPy, you'll train your own neural networks to see and understand images, translate text into different languages, and even write like Shakespeare! When you're done, you'll be fully prepared to move on to mastering deep learning frameworks. What's inside The science behind deep learning Building and training your own neural networks Privacy concepts, including federated learning Tips for continuing your pursuit of deep learning About the Reader For readers with high school-level math and intermediate programming skills. About the Author Andrew Trask is a PhD student at Oxford University and a research scientist at DeepMind. Previously, Andrew was a researcher and analytics product manager at Digital Reasoning, where he trained the world's largest artificial neural network and helped guide the analytics roadmap for the Synthesys cognitive computing platform. Table of Contents Introducing deep learning: why you should learn it Fundamental concepts: how do machines learn? Introduction to neural prediction: forward propagation Introduction to neural learning: gradient descent Learning multiple weights at a time: generalizing gradient descent Building your first deep neural network: introduction to backpropagation How to picture neural networks: in your head and on paper Learning signal and ignoring noise:introduction to regularization and batching Modeling probabilities and nonlinearities: activation functions Neural learning about edges and corners: intro to convolutional neural networks Neural networks that understand language: king - man + woman == ? Neural networks that write like Shakespeare: recurrent layers for variable-length data Introducing automatic optimization: let's build a deep learning framework Learning to write like Shakespeare: long short-term memory Deep learning on unseen data: introducing federated learning Where to go from here: a brief guide
Introduction To Machine Learning
DOWNLOAD
Author : Ethem Alpaydin
language : en
Publisher: MIT Press
Release Date : 2014-08-22
Introduction To Machine Learning written by Ethem Alpaydin and has been published by MIT Press this book supported file pdf, txt, epub, kindle and other format this book has been release on 2014-08-22 with Computers categories.
Introduction -- Supervised learning -- Bayesian decision theory -- Parametric methods -- Multivariate methods -- Dimensionality reduction -- Clustering -- Nonparametric methods -- Decision trees -- Linear discrimination -- Multilayer perceptrons -- Local models -- Kernel machines -- Graphical models -- Brief contents -- Hidden markov models -- Bayesian estimation -- Combining multiple learners -- Reinforcement learning -- Design and analysis of machine learning experiments.
Machine Learning For Beginners
DOWNLOAD
Author : Alec Gilliam
language : en
Publisher: Createspace Independent Publishing Platform
Release Date : 2018-05-09
Machine Learning For Beginners written by Alec Gilliam and has been published by Createspace Independent Publishing Platform this book supported file pdf, txt, epub, kindle and other format this book has been release on 2018-05-09 with categories.
Machine Learning Machine learning is the ability of artificial intelligence to learn and adapt without being explicitly programmed to perform a pre-prescribed outcome. It is a machine's ability to act on its own, to learn. Many forms of artificial intelligence use a wide variety of tactics to employ this, including pattern recognition and unsupervised learning algorithms. Currently, there is a lot of excitement and focus on machine learning in the world of programming, as it is often interpreted to imply a limitless future in artificial intelligence: a world in which machines could adapt and respond to a wide variety of stimuli and factors without direct programming response from a human. A world in which machines could learn and adapt on their own. Often, machine learning is seen as the missing "human" element in machines; the ability for machines to start to fill the gap between what makes us human and what makes machines; a sense of responsiveness and flexibility in a given environment and a set of circumstances. By and large, the goal of learning is to be able to generalize: to take a set of lived circumstances, and to be able to extrapolate a sense of patterning about what the future holds, and how the person should respond to similar given situations. Machine learning is similar; it is the attempt to program machines so that they can generalize about future possibilities and probabilities based on data sets. In short- machine learning is the quest to get machines to think like humans. This takes a wide variety of forms and is used for a wide variety of purposes. In the following book, we will explore the history of machine learning, the academic and scientific elements that make up the study, as well as touching on this moral and philosophical space that they occupy. In this book you will find: What is Machine Learning? The History of Machine Learning Examples of Machine Learning How Does Machine Learning Work? Common Approaches and Terms in Machine Learning Theoretical Computer Science Computational Learning Theory/Association Rule Learning Pattern Recognition Deep Learning Induction Logic Reasoning Neural networks Expert Systems Naive Bayes Systems Unsupervised and supervised learning algorithms Decision Trees Random Decision Forests The Moral and Philosophical Implications of Machine Learning
Machine Learning
DOWNLOAD
Author : Ryszard S. Michalski
language : en
Publisher: Morgan Kaufmann
Release Date : 1985-10-01
Machine Learning written by Ryszard S. Michalski and has been published by Morgan Kaufmann this book supported file pdf, txt, epub, kindle and other format this book has been release on 1985-10-01 with Computers categories.
Machine Learning: An Artificial Intelligence Approach contains tutorial overviews and research papers representative of trends in the area of machine learning as viewed from an artificial intelligence perspective. The book is organized into six parts. Part I provides an overview of machine learning and explains why machines should learn. Part II covers important issues affecting the design of learning programs-particularly programs that learn from examples. It also describes inductive learning systems. Part III deals with learning by analogy, by experimentation, and from experience. Parts IV and V discuss learning from observation and discovery, and learning from instruction, respectively. Part VI presents two studies on applied learning systems-one on the recovery of valuable information via inductive inference; the other on inducing models of simple algebraic skills from observed student performance in the context of the Leeds Modeling System (LMS). This book is intended for researchers in artificial intelligence, computer science, and cognitive psychology; students in artificial intelligence and related disciplines; and a diverse range of readers, including computer scientists, robotics experts, knowledge engineers, educators, philosophers, data analysts, psychologists, and electronic engineers.
Readings In Machine Learning
DOWNLOAD
Author : Jude W. Shavlik
language : en
Publisher: Morgan Kaufmann
Release Date : 1990
Readings In Machine Learning written by Jude W. Shavlik and has been published by Morgan Kaufmann this book supported file pdf, txt, epub, kindle and other format this book has been release on 1990 with Computers categories.
The ability to learn is a fundamental characteristic of intelligent behavior. Consequently, machine learning has been a focus of artificial intelligence since the beginnings of AI in the 1950s. The 1980s saw tremendous growth in the field, and this growth promises to continue with valuable contributions to science, engineering, and business. Readings in Machine Learning collects the best of the published machine learning literature, including papers that address a wide range of learning tasks, and that introduce a variety of techniques for giving machines the ability to learn. The editors, in cooperation with a group of expert referees, have chosen important papers that empirically study, theoretically analyze, or psychologically justify machine learning algorithms. The papers are grouped into a dozen categories, each of which is introduced by the editors.