Bayesian Reasoning And Gaussian Processes For Machine Learning Applications
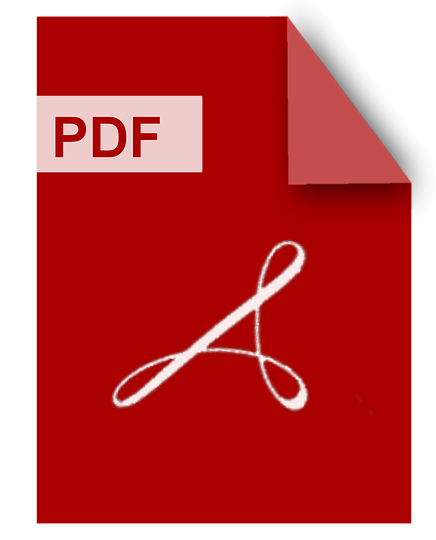
DOWNLOAD
Download Bayesian Reasoning And Gaussian Processes For Machine Learning Applications PDF/ePub or read online books in Mobi eBooks. Click Download or Read Online button to get Bayesian Reasoning And Gaussian Processes For Machine Learning Applications book now. This website allows unlimited access to, at the time of writing, more than 1.5 million titles, including hundreds of thousands of titles in various foreign languages. If the content not found or just blank you must refresh this page
Bayesian Reasoning And Gaussian Processes For Machine Learning Applications
DOWNLOAD
Author : Hemachandran K
language : en
Publisher: CRC Press
Release Date : 2022-04-14
Bayesian Reasoning And Gaussian Processes For Machine Learning Applications written by Hemachandran K and has been published by CRC Press this book supported file pdf, txt, epub, kindle and other format this book has been release on 2022-04-14 with Business & Economics categories.
This book introduces Bayesian reasoning and Gaussian processes into machine learning applications. Bayesian methods are applied in many areas, such as game development, decision making, and drug discovery. It is very effective for machine learning algorithms in handling missing data and extracting information from small datasets. Bayesian Reasoning and Gaussian Processes for Machine Learning Applications uses a statistical background to understand continuous distributions and how learning can be viewed from a probabilistic framework. The chapters progress into such machine learning topics as belief network and Bayesian reinforcement learning, which is followed by Gaussian process introduction, classification, regression, covariance, and performance analysis of Gaussian processes with other models. FEATURES Contains recent advancements in machine learning Highlights applications of machine learning algorithms Offers both quantitative and qualitative research Includes numerous case studies This book is aimed at graduates, researchers, and professionals in the field of data science and machine learning.
Bayesian Reasoning And Gaussian Processes For Machine Learning Applications
DOWNLOAD
Author : Hemachandran K
language : en
Publisher: CRC Press
Release Date : 2022-04-14
Bayesian Reasoning And Gaussian Processes For Machine Learning Applications written by Hemachandran K and has been published by CRC Press this book supported file pdf, txt, epub, kindle and other format this book has been release on 2022-04-14 with Business & Economics categories.
This book introduces Bayesian reasoning and Gaussian processes into machine learning applications. Bayesian methods are applied in many areas, such as game development, decision making, and drug discovery. It is very effective for machine learning algorithms in handling missing data and extracting information from small datasets. Bayesian Reasoning and Gaussian Processes for Machine Learning Applications uses a statistical background to understand continuous distributions and how learning can be viewed from a probabilistic framework. The chapters progress into such machine learning topics as belief network and Bayesian reinforcement learning, which is followed by Gaussian process introduction, classification, regression, covariance, and performance analysis of Gaussian processes with other models. FEATURES Contains recent advancements in machine learning Highlights applications of machine learning algorithms Offers both quantitative and qualitative research Includes numerous case studies This book is aimed at graduates, researchers, and professionals in the field of data science and machine learning.
Gaussian Processes For Machine Learning
DOWNLOAD
Author : Carl Edward Rasmussen
language : en
Publisher: MIT Press
Release Date : 2005-11-23
Gaussian Processes For Machine Learning written by Carl Edward Rasmussen and has been published by MIT Press this book supported file pdf, txt, epub, kindle and other format this book has been release on 2005-11-23 with Computers categories.
A comprehensive and self-contained introduction to Gaussian processes, which provide a principled, practical, probabilistic approach to learning in kernel machines. Gaussian processes (GPs) provide a principled, practical, probabilistic approach to learning in kernel machines. GPs have received increased attention in the machine-learning community over the past decade, and this book provides a long-needed systematic and unified treatment of theoretical and practical aspects of GPs in machine learning. The treatment is comprehensive and self-contained, targeted at researchers and students in machine learning and applied statistics. The book deals with the supervised-learning problem for both regression and classification, and includes detailed algorithms. A wide variety of covariance (kernel) functions are presented and their properties discussed. Model selection is discussed both from a Bayesian and a classical perspective. Many connections to other well-known techniques from machine learning and statistics are discussed, including support-vector machines, neural networks, splines, regularization networks, relevance vector machines and others. Theoretical issues including learning curves and the PAC-Bayesian framework are treated, and several approximation methods for learning with large datasets are discussed. The book contains illustrative examples and exercises, and code and datasets are available on the Web. Appendixes provide mathematical background and a discussion of Gaussian Markov processes.
Efficient Reinforcement Learning Using Gaussian Processes
DOWNLOAD
Author : Marc Peter Deisenroth
language : en
Publisher: KIT Scientific Publishing
Release Date : 2010
Efficient Reinforcement Learning Using Gaussian Processes written by Marc Peter Deisenroth and has been published by KIT Scientific Publishing this book supported file pdf, txt, epub, kindle and other format this book has been release on 2010 with Electronic computers. Computer science categories.
This book examines Gaussian processes in both model-based reinforcement learning (RL) and inference in nonlinear dynamic systems.First, we introduce PILCO, a fully Bayesian approach for efficient RL in continuous-valued state and action spaces when no expert knowledge is available. PILCO takes model uncertainties consistently into account during long-term planning to reduce model bias. Second, we propose principled algorithms for robust filtering and smoothing in GP dynamic systems.
Bayesian Reasoning And Machine Learning
DOWNLOAD
Author : David Barber
language : en
Publisher: Cambridge University Press
Release Date : 2012-02-02
Bayesian Reasoning And Machine Learning written by David Barber and has been published by Cambridge University Press this book supported file pdf, txt, epub, kindle and other format this book has been release on 2012-02-02 with Computers categories.
Machine learning methods extract value from vast data sets quickly and with modest resources. They are established tools in a wide range of industrial applications, including search engines, DNA sequencing, stock market analysis, and robot locomotion, and their use is spreading rapidly. People who know the methods have their choice of rewarding jobs. This hands-on text opens these opportunities to computer science students with modest mathematical backgrounds. It is designed for final-year undergraduates and master's students with limited background in linear algebra and calculus. Comprehensive and coherent, it develops everything from basic reasoning to advanced techniques within the framework of graphical models. Students learn more than a menu of techniques, they develop analytical and problem-solving skills that equip them for the real world. Numerous examples and exercises, both computer based and theoretical, are included in every chapter. Resources for students and instructors, including a MATLAB toolbox, are available online.
Bayesian Reasoning And Machine Learning
DOWNLOAD
Author : David Barber
language : en
Publisher: Cambridge University Press
Release Date : 2012-02-02
Bayesian Reasoning And Machine Learning written by David Barber and has been published by Cambridge University Press this book supported file pdf, txt, epub, kindle and other format this book has been release on 2012-02-02 with Computers categories.
A practical introduction perfect for final-year undergraduate and graduate students without a solid background in linear algebra and calculus.
Bayesian Learning For Neural Networks
DOWNLOAD
Author : Radford M. Neal
language : en
Publisher: Springer Science & Business Media
Release Date : 2012-12-06
Bayesian Learning For Neural Networks written by Radford M. Neal and has been published by Springer Science & Business Media this book supported file pdf, txt, epub, kindle and other format this book has been release on 2012-12-06 with Mathematics categories.
Artificial "neural networks" are widely used as flexible models for classification and regression applications, but questions remain about how the power of these models can be safely exploited when training data is limited. This book demonstrates how Bayesian methods allow complex neural network models to be used without fear of the "overfitting" that can occur with traditional training methods. Insight into the nature of these complex Bayesian models is provided by a theoretical investigation of the priors over functions that underlie them. A practical implementation of Bayesian neural network learning using Markov chain Monte Carlo methods is also described, and software for it is freely available over the Internet. Presupposing only basic knowledge of probability and statistics, this book should be of interest to researchers in statistics, engineering, and artificial intelligence.
Machine Learning And Its Applications
DOWNLOAD
Author : Georgios Paliouras
language : en
Publisher: Springer
Release Date : 2003-06-29
Machine Learning And Its Applications written by Georgios Paliouras and has been published by Springer this book supported file pdf, txt, epub, kindle and other format this book has been release on 2003-06-29 with Computers categories.
In recent years machine learning has made its way from artificial intelligence into areas of administration, commerce, and industry. Data mining is perhaps the most widely known demonstration of this migration, complemented by less publicized applications of machine learning like adaptive systems in industry, financial prediction, medical diagnosis and the construction of user profiles for Web browsers. This book presents the capabilities of machine learning methods and ideas on how these methods could be used to solve real-world problems. The first ten chapters assess the current state of the art of machine learning, from symbolic concept learning and conceptual clustering to case-based reasoning, neural networks, and genetic algorithms. The second part introduces the reader to innovative applications of ML techniques in fields such as data mining, knowledge discovery, human language technology, user modeling, data analysis, discovery science, agent technology, finance, etc.
Bayesian Analysis With Python
DOWNLOAD
Author : Osvaldo Martin
language : en
Publisher: Packt Publishing Ltd
Release Date : 2016-11-25
Bayesian Analysis With Python written by Osvaldo Martin and has been published by Packt Publishing Ltd this book supported file pdf, txt, epub, kindle and other format this book has been release on 2016-11-25 with Computers categories.
Unleash the power and flexibility of the Bayesian framework About This Book Simplify the Bayes process for solving complex statistical problems using Python; Tutorial guide that will take the you through the journey of Bayesian analysis with the help of sample problems and practice exercises; Learn how and when to use Bayesian analysis in your applications with this guide. Who This Book Is For Students, researchers and data scientists who wish to learn Bayesian data analysis with Python and implement probabilistic models in their day to day projects. Programming experience with Python is essential. No previous statistical knowledge is assumed. What You Will Learn Understand the essentials Bayesian concepts from a practical point of view Learn how to build probabilistic models using the Python library PyMC3 Acquire the skills to sanity-check your models and modify them if necessary Add structure to your models and get the advantages of hierarchical models Find out how different models can be used to answer different data analysis questions When in doubt, learn to choose between alternative models. Predict continuous target outcomes using regression analysis or assign classes using logistic and softmax regression. Learn how to think probabilistically and unleash the power and flexibility of the Bayesian framework In Detail The purpose of this book is to teach the main concepts of Bayesian data analysis. We will learn how to effectively use PyMC3, a Python library for probabilistic programming, to perform Bayesian parameter estimation, to check models and validate them. This book begins presenting the key concepts of the Bayesian framework and the main advantages of this approach from a practical point of view. Moving on, we will explore the power and flexibility of generalized linear models and how to adapt them to a wide array of problems, including regression and classification. We will also look into mixture models and clustering data, and we will finish with advanced topics like non-parametrics models and Gaussian processes. With the help of Python and PyMC3 you will learn to implement, check and expand Bayesian models to solve data analysis problems. Style and approach Bayes algorithms are widely used in statistics, machine learning, artificial intelligence, and data mining. This will be a practical guide allowing the readers to use Bayesian methods for statistical modelling and analysis using Python.
Bayesian Optimization With Application To Computer Experiments
DOWNLOAD
Author : Tony Pourmohamad
language : en
Publisher: Springer Nature
Release Date : 2021-10-04
Bayesian Optimization With Application To Computer Experiments written by Tony Pourmohamad and has been published by Springer Nature this book supported file pdf, txt, epub, kindle and other format this book has been release on 2021-10-04 with Mathematics categories.
This book introduces readers to Bayesian optimization, highlighting advances in the field and showcasing its successful applications to computer experiments. R code is available as online supplementary material for most included examples, so that readers can better comprehend and reproduce methods. Compact and accessible, the volume is broken down into four chapters. Chapter 1 introduces the reader to the topic of computer experiments; it includes a variety of examples across many industries. Chapter 2 focuses on the task of surrogate model building and contains a mix of several different surrogate models that are used in the computer modeling and machine learning communities. Chapter 3 introduces the core concepts of Bayesian optimization and discusses unconstrained optimization. Chapter 4 moves on to constrained optimization, and showcases some of the most novel methods found in the field. This will be a useful companion to researchers and practitioners working with computer experiments and computer modeling. Additionally, readers with a background in machine learning but minimal background in computer experiments will find this book an interesting case study of the applicability of Bayesian optimization outside the realm of machine learning.