Learning Probabilistic Graphical Models In R
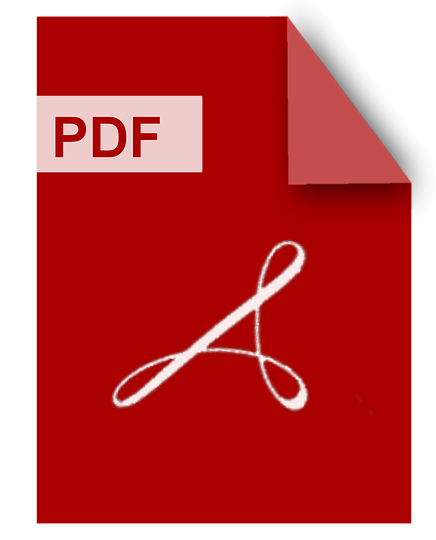
DOWNLOAD
Download Learning Probabilistic Graphical Models In R PDF/ePub or read online books in Mobi eBooks. Click Download or Read Online button to get Learning Probabilistic Graphical Models In R book now. This website allows unlimited access to, at the time of writing, more than 1.5 million titles, including hundreds of thousands of titles in various foreign languages. If the content not found or just blank you must refresh this page
Learning Probabilistic Graphical Models In R
DOWNLOAD
Author : David Bellot
language : en
Publisher: Packt Publishing Ltd
Release Date : 2016-04-29
Learning Probabilistic Graphical Models In R written by David Bellot and has been published by Packt Publishing Ltd this book supported file pdf, txt, epub, kindle and other format this book has been release on 2016-04-29 with Computers categories.
Familiarize yourself with probabilistic graphical models through real-world problems and illustrative code examples in R About This Book Predict and use a probabilistic graphical models (PGM) as an expert system Comprehend how your computer can learn Bayesian modeling to solve real-world problems Know how to prepare data and feed the models by using the appropriate algorithms from the appropriate R package Who This Book Is For This book is for anyone who has to deal with lots of data and draw conclusions from it, especially when the data is noisy or uncertain. Data scientists, machine learning enthusiasts, engineers, and those who curious about the latest advances in machine learning will find PGM interesting. What You Will Learn Understand the concepts of PGM and which type of PGM to use for which problem Tune the model's parameters and explore new models automatically Understand the basic principles of Bayesian models, from simple to advanced Transform the old linear regression model into a powerful probabilistic model Use standard industry models but with the power of PGM Understand the advanced models used throughout today's industry See how to compute posterior distribution with exact and approximate inference algorithms In Detail Probabilistic graphical models (PGM, also known as graphical models) are a marriage between probability theory and graph theory. Generally, PGMs use a graph-based representation. Two branches of graphical representations of distributions are commonly used, namely Bayesian networks and Markov networks. R has many packages to implement graphical models. We'll start by showing you how to transform a classical statistical model into a modern PGM and then look at how to do exact inference in graphical models. Proceeding, we'll introduce you to many modern R packages that will help you to perform inference on the models. We will then run a Bayesian linear regression and you'll see the advantage of going probabilistic when you want to do prediction. Next, you'll master using R packages and implementing its techniques. Finally, you'll be presented with machine learning applications that have a direct impact in many fields. Here, we'll cover clustering and the discovery of hidden information in big data, as well as two important methods, PCA and ICA, to reduce the size of big problems. Style and approach This book gives you a detailed and step-by-step explanation of each mathematical concept, which will help you build and analyze your own machine learning models and apply them to real-world problems. The mathematics is kept simple and each formula is explained thoroughly.
Graphical Models
DOWNLOAD
Author : Christian Borgelt
language : en
Publisher: John Wiley & Sons
Release Date : 2009-07-30
Graphical Models written by Christian Borgelt and has been published by John Wiley & Sons this book supported file pdf, txt, epub, kindle and other format this book has been release on 2009-07-30 with Mathematics categories.
Graphical models are of increasing importance in applied statistics, and in particular in data mining. Providing a self-contained introduction and overview to learning relational, probabilistic, and possibilistic networks from data, this second edition of Graphical Models is thoroughly updated to include the latest research in this burgeoning field, including a new chapter on visualization. The text provides graduate students, and researchers with all the necessary background material, including modelling under uncertainty, decomposition of distributions, graphical representation of distributions, and applications relating to graphical models and problems for further research.
Graphical Models With R
DOWNLOAD
Author : Søren Højsgaard
language : en
Publisher: Springer Science & Business Media
Release Date : 2012-02-22
Graphical Models With R written by Søren Højsgaard and has been published by Springer Science & Business Media this book supported file pdf, txt, epub, kindle and other format this book has been release on 2012-02-22 with Mathematics categories.
Graphical models in their modern form have been around since the late 1970s and appear today in many areas of the sciences. Along with the ongoing developments of graphical models, a number of different graphical modeling software programs have been written over the years. In recent years many of these software developments have taken place within the R community, either in the form of new packages or by providing an R interface to existing software. This book attempts to give the reader a gentle introduction to graphical modeling using R and the main features of some of these packages. In addition, the book provides examples of how more advanced aspects of graphical modeling can be represented and handled within R. Topics covered in the seven chapters include graphical models for contingency tables, Gaussian and mixed graphical models, Bayesian networks and modeling high dimensional data.
Building Probabilistic Graphical Models With Python
DOWNLOAD
Author : Kiran R. Karkera
language : en
Publisher:
Release Date : 2014
Building Probabilistic Graphical Models With Python written by Kiran R. Karkera and has been published by this book supported file pdf, txt, epub, kindle and other format this book has been release on 2014 with Graphical modeling (Statistics) categories.
"This is a short, practical guide that allows data scientists to understand the concepts of Graphical models and enables them to try them out using small Python code snippets, without being too mathematically complicated. If you are a data scientist who knows about machine learning and want to enhance your knowledge of graphical models, such as Bayes network, in order to use them to solve real-world problems using Python libraries, this book is for you.This book is intended for those who have some Python and machine learning experience, or are exploring the machine learning field."
Learning In Graphical Models
DOWNLOAD
Author : Michael Irwin Jordan
language : en
Publisher: MIT Press
Release Date : 1999
Learning In Graphical Models written by Michael Irwin Jordan and has been published by MIT Press this book supported file pdf, txt, epub, kindle and other format this book has been release on 1999 with Computers categories.
Presents an exploration of issues related to learning within the graphical model formalism. This text covers topics such as: inference for Bayesian networks; Monte Carlo methods; variational methods; and learning with Bayesian networks.
Bayesian Networks
DOWNLOAD
Author : Marco Scutari
language : en
Publisher: CRC Press
Release Date : 2021-07-28
Bayesian Networks written by Marco Scutari and has been published by CRC Press this book supported file pdf, txt, epub, kindle and other format this book has been release on 2021-07-28 with Computers categories.
Explains the material step-by-step starting from meaningful examples Steps detailed with R code in the spirit of reproducible research Real world data analyses from a Science paper reproduced and explained in detail Examples span a variety of fields across social and life sciences Overview of available software in and outside R
Graphical Models With R
DOWNLOAD
Author :
language : en
Publisher:
Release Date : 2012-02-19
Graphical Models With R written by and has been published by this book supported file pdf, txt, epub, kindle and other format this book has been release on 2012-02-19 with categories.
Mastering Probabilistic Graphical Models Using Python
DOWNLOAD
Author : Ankur Ankan
language : en
Publisher: Packt Publishing Ltd
Release Date : 2015-08-03
Mastering Probabilistic Graphical Models Using Python written by Ankur Ankan and has been published by Packt Publishing Ltd this book supported file pdf, txt, epub, kindle and other format this book has been release on 2015-08-03 with Computers categories.
Master probabilistic graphical models by learning through real-world problems and illustrative code examples in Python About This Book Gain in-depth knowledge of Probabilistic Graphical Models Model time-series problems using Dynamic Bayesian Networks A practical guide to help you apply PGMs to real-world problems Who This Book Is For If you are a researcher or a machine learning enthusiast, or are working in the data science field and have a basic idea of Bayesian Learning or Probabilistic Graphical Models, this book will help you to understand the details of Graphical Models and use it in your data science problems. This book will also help you select the appropriate model as well as the appropriate algorithm for your problem. What You Will Learn Get to know the basics of Probability theory and Graph Theory Work with Markov Networks Implement Bayesian Networks Exact Inference Techniques in Graphical Models such as the Variable Elimination Algorithm Understand approximate Inference Techniques in Graphical Models such as Message Passing Algorithms Sample algorithms in Graphical Models Grasp details of Naive Bayes with real-world examples Deploy PGMs using various libraries in Python Gain working details of Hidden Markov Models with real-world examples In Detail Probabilistic Graphical Models is a technique in machine learning that uses the concepts of graph theory to compactly represent and optimally predict values in our data problems. In real world problems, it's often difficult to select the appropriate graphical model as well as the appropriate inference algorithm, which can make a huge difference in computation time and accuracy. Thus, it is crucial to know the working details of these algorithms. This book starts with the basics of probability theory and graph theory, then goes on to discuss various models and inference algorithms. All the different types of models are discussed along with code examples to create and modify them, and also to run different inference algorithms on them. There is a complete chapter devoted to the most widely used networks Naive Bayes Model and Hidden Markov Models (HMMs). These models have been thoroughly discussed using real-world examples. Style and approach An easy-to-follow guide to help you understand Probabilistic Graphical Models using simple examples and numerous code examples, with an emphasis on more widely used models.
Sparse Graphical Modeling For High Dimensional Data
DOWNLOAD
Author : Faming Liang
language : en
Publisher: CRC Press
Release Date : 2023-08-02
Sparse Graphical Modeling For High Dimensional Data written by Faming Liang and has been published by CRC Press this book supported file pdf, txt, epub, kindle and other format this book has been release on 2023-08-02 with Mathematics categories.
A general framework for learning sparse graphical models with conditional independence tests Complete treatments for different types of data, Gaussian, Poisson, multinomial, and mixed data Unified treatments for data integration, network comparison, and covariate adjustment Unified treatments for missing data and heterogeneous data Efficient methods for joint estimation of multiple graphical models Effective methods of high-dimensional variable selection Effective methods of high-dimensional inference
Building Probabilistic Graphical Models With Python
DOWNLOAD
Author : Kiran K. Karkera
language : en
Publisher: CreateSpace
Release Date : 2015-05-18
Building Probabilistic Graphical Models With Python written by Kiran K. Karkera and has been published by CreateSpace this book supported file pdf, txt, epub, kindle and other format this book has been release on 2015-05-18 with categories.
With the increasing prominence in machine learning and data science applications, probabilistic graphical models are a new tool that machine learning users can use to discover and analyze structures in complex problems. The variety of tools and algorithms under the PGM framework extend to many domains such as natural language processing, speech processing, image processing, and disease diagnosis. You've probably heard of graphical models before, and you're keen to try out new landscapes in the machine learning area. This book gives you enough background information to get started on graphical models, while keeping the math to a minimum. Approach This is a short, practical guide that allows data scientists to understand the concepts of Graphical models and enables them to try them out using small Python code snippets, without being too mathematically complicated. Who this book is for If you are a data scientist who knows about machine learning and want to enhance your knowledge of graphical models, such as Bayes network, in order to use them to solve real-world problems using Python libraries, this book is for you.This book is intended for those who have some Python and machine learning experience, or are exploring the machine learning field.