Low Rank Approximation
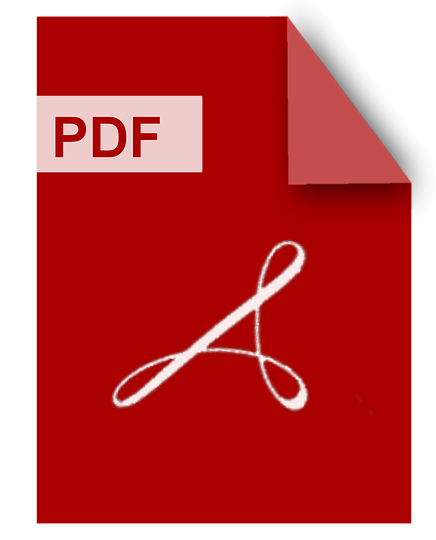
DOWNLOAD
Download Low Rank Approximation PDF/ePub or read online books in Mobi eBooks. Click Download or Read Online button to get Low Rank Approximation book now. This website allows unlimited access to, at the time of writing, more than 1.5 million titles, including hundreds of thousands of titles in various foreign languages. If the content not found or just blank you must refresh this page
Low Rank Approximation
DOWNLOAD
Author : Ivan Markovsky
language : en
Publisher: Springer
Release Date : 2019-01-10
Low Rank Approximation written by Ivan Markovsky and has been published by Springer this book supported file pdf, txt, epub, kindle and other format this book has been release on 2019-01-10 with Technology & Engineering categories.
This book is a comprehensive exposition of the theory, algorithms, and applications of structured low-rank approximation. Local optimization methods and effective suboptimal convex relaxations for Toeplitz, Hankel, and Sylvester structured problems are presented. A major part of the text is devoted to application of the theory with a range of applications from systems and control theory to psychometrics being described. Special knowledge of the application fields is not required. The second edition of /Low-Rank Approximation/ is a thoroughly edited and extensively rewritten revision. It contains new chapters and sections that introduce the topics of: • variable projection for structured low-rank approximation;• missing data estimation;• data-driven filtering and control;• stochastic model representation and identification;• identification of polynomial time-invariant systems; and• blind identification with deterministic input model. The book is complemented by a software implementation of the methods presented, which makes the theory directly applicable in practice. In particular, all numerical examples in the book are included in demonstration files and can be reproduced by the reader. This gives hands-on experience with the theory and methods detailed. In addition, exercises and MATLAB^® /Octave examples will assist the reader quickly to assimilate the theory on a chapter-by-chapter basis. “Each chapter is completed with a new section of exercises to which complete solutions are provided.” Low-Rank Approximation (second edition) is a broad survey of the Low-Rank Approximation theory and applications of its field which will be of direct interest to researchers in system identification, control and systems theory, numerical linear algebra and optimization. The supplementary problems and solutions render it suitable for use in teaching graduate courses in those subjects as well.
Low Rank Approximation
DOWNLOAD
Author : Ivan Markovsky
language : en
Publisher: Springer Science & Business Media
Release Date : 2011-11-19
Low Rank Approximation written by Ivan Markovsky and has been published by Springer Science & Business Media this book supported file pdf, txt, epub, kindle and other format this book has been release on 2011-11-19 with Technology & Engineering categories.
Data Approximation by Low-complexity Models details the theory, algorithms, and applications of structured low-rank approximation. Efficient local optimization methods and effective suboptimal convex relaxations for Toeplitz, Hankel, and Sylvester structured problems are presented. Much of the text is devoted to describing the applications of the theory including: system and control theory; signal processing; computer algebra for approximate factorization and common divisor computation; computer vision for image deblurring and segmentation; machine learning for information retrieval and clustering; bioinformatics for microarray data analysis; chemometrics for multivariate calibration; and psychometrics for factor analysis. Software implementation of the methods is given, making the theory directly applicable in practice. All numerical examples are included in demonstration files giving hands-on experience and exercises and MATLAB® examples assist in the assimilation of the theory.
Optimization And Dynamical Systems
DOWNLOAD
Author : Uwe Helmke
language : en
Publisher: Springer Science & Business Media
Release Date : 2012-12-06
Optimization And Dynamical Systems written by Uwe Helmke and has been published by Springer Science & Business Media this book supported file pdf, txt, epub, kindle and other format this book has been release on 2012-12-06 with Technology & Engineering categories.
This work is aimed at mathematics and engineering graduate students and researchers in the areas of optimization, dynamical systems, control sys tems, signal processing, and linear algebra. The motivation for the results developed here arises from advanced engineering applications and the emer gence of highly parallel computing machines for tackling such applications. The problems solved are those of linear algebra and linear systems the ory, and include such topics as diagonalizing a symmetric matrix, singular value decomposition, balanced realizations, linear programming, sensitivity minimization, and eigenvalue assignment by feedback control. The tools are those, not only of linear algebra and systems theory, but also of differential geometry. The problems are solved via dynamical sys tems implementation, either in continuous time or discrete time , which is ideally suited to distributed parallel processing. The problems tackled are indirectly or directly concerned with dynamical systems themselves, so there is feedback in that dynamical systems are used to understand and optimize dynamical systems. One key to the new research results has been the recent discovery of rather deep existence and uniqueness results for the solution of certain matrix least squares optimization problems in geomet ric invariant theory. These problems, as well as many other optimization problems arising in linear algebra and systems theory, do not always admit solutions which can be found by algebraic methods.
Generalized Low Rank Models
DOWNLOAD
Author : Madeleine Udell
language : en
Publisher:
Release Date : 2015
Generalized Low Rank Models written by Madeleine Udell and has been published by this book supported file pdf, txt, epub, kindle and other format this book has been release on 2015 with categories.
Principal components analysis (PCA) is a well-known technique for approximating a tabular data set by a low rank matrix. This dissertation extends the idea of PCA to handle arbitrary data sets consisting of numerical, Boolean, categorical, ordinal, and other data types. This framework encompasses many well known techniques in data analysis, such as nonnegative matrix factorization, matrix completion, sparse and robust PCA, k-means, k-SVD, and maximum margin matrix factorization. The method handles heterogeneous data sets, and leads to coherent schemes for compressing, denoising, and imputing missing entries across all data types simultaneously. It also admits a number of interesting interpretations of the low rank factors, which allow clustering of examples or of features. We propose several parallel algorithms for fitting generalized low rank models, and describe implementations and numerical results.
Symbolic Numeric Computation
DOWNLOAD
Author : Dongming Wang
language : en
Publisher: Springer Science & Business Media
Release Date : 2007-01-22
Symbolic Numeric Computation written by Dongming Wang and has been published by Springer Science & Business Media this book supported file pdf, txt, epub, kindle and other format this book has been release on 2007-01-22 with Mathematics categories.
The growing demand of speed, accuracy, and reliability in scientific and engineering computing has been accelerating the merging of symbolic and numeric computations. These two types of computation coexist in mathematics yet are separated in traditional research of mathematical computation. This book presents 27 research articles on the integration and interaction of symbolic and numeric computation.
Geometric Numerical Integration
DOWNLOAD
Author : Ernst Hairer
language : en
Publisher: Springer Science & Business Media
Release Date : 2013-03-09
Geometric Numerical Integration written by Ernst Hairer and has been published by Springer Science & Business Media this book supported file pdf, txt, epub, kindle and other format this book has been release on 2013-03-09 with Mathematics categories.
Numerical methods that preserve properties of Hamiltonian systems, reversible systems, differential equations on manifolds and problems with highly oscillatory solutions are the subject of this book. A complete self-contained theory of symplectic and symmetric methods, which include Runge-Kutta, composition, splitting, multistep and various specially designed integrators, is presented and their construction and practical merits are discussed. The long-time behaviour of the numerical solutions is studied using a backward error analysis (modified equations) combined with KAM theory. The book is illustrated by many figures, it treats applications from physics and astronomy and contains many numerical experiments and comparisons of different approaches.
Tensor Spaces And Numerical Tensor Calculus
DOWNLOAD
Author : Wolfgang Hackbusch
language : en
Publisher: Springer Nature
Release Date : 2019-12-16
Tensor Spaces And Numerical Tensor Calculus written by Wolfgang Hackbusch and has been published by Springer Nature this book supported file pdf, txt, epub, kindle and other format this book has been release on 2019-12-16 with Mathematics categories.
Special numerical techniques are already needed to deal with n × n matrices for large n. Tensor data are of size n × n ×...× n=nd, where nd exceeds the computer memory by far. They appear for problems of high spatial dimensions. Since standard methods fail, a particular tensor calculus is needed to treat such problems. This monograph describes the methods by which tensors can be practically treated and shows how numerical operations can be performed. Applications include problems from quantum chemistry, approximation of multivariate functions, solution of partial differential equations, for example with stochastic coefficients, and more. In addition to containing corrections of the unavoidable misprints, this revised second edition includes new parts ranging from single additional statements to new subchapters. The book is mainly addressed to numerical mathematicians and researchers working with high-dimensional data. It also touches problems related to Geometric Algebra.
Sketching As A Tool For Numerical Linear Algebra
DOWNLOAD
Author : David P. Woodruff
language : en
Publisher: Now Publishers
Release Date : 2014-11-14
Sketching As A Tool For Numerical Linear Algebra written by David P. Woodruff and has been published by Now Publishers this book supported file pdf, txt, epub, kindle and other format this book has been release on 2014-11-14 with Computers categories.
Sketching as a Tool for Numerical Linear Algebra highlights the recent advances in algorithms for numerical linear algebra that have come from the technique of linear sketching, whereby given a matrix, one first compressed it to a much smaller matrix by multiplying it by a (usually) random matrix with certain properties. Much of the expensive computation can then be performed on the smaller matrix, thereby accelerating the solution for the original problem. It is an ideal primer for researchers and students of theoretical computer science interested in how sketching techniques can be used to speed up numerical linear algebra applications.
An Introduction To Group Representation Theory
DOWNLOAD
Author : R. Keown
language : en
Publisher: Elsevier Science & Technology
Release Date : 1975
An Introduction To Group Representation Theory written by R. Keown and has been published by Elsevier Science & Technology this book supported file pdf, txt, epub, kindle and other format this book has been release on 1975 with Mathematics categories.
In this book, we study theoretical and practical aspects of computing methods for mathematical modelling of nonlinear systems. A number of computing techniques are considered, such as methods of operator approximation with any given accuracy; operator interpolation techniques including a non-Lagrange interpolation; methods of system representation subject to constraints associated with concepts of causality, memory and stationarity; methods of system representation with an accuracy that is the best within a given class of models; methods of covariance matrix estimation; methods for low-rank matrix approximations; hybrid methods based on a combination of iterative procedures and best operator approximation; and methods for information compression and filtering under condition that a filter model should satisfy restrictions associated with causality and different types of memory. As a result, the book represents a blend of new methods in general computational analysis, and specific, but also generic, techniques for study of systems theory ant its particular branches, such as optimal filtering and information compression. - Best operator approximation, - Non-Lagrange interpolation, - Generic Karhunen-Loeve transform - Generalised low-rank matrix approximation - Optimal data compression - Optimal nonlinear filtering
Low Rank Semidefinite Programming
DOWNLOAD
Author : Alex Lemon
language : en
Publisher: Now Publishers
Release Date : 2016-05-04
Low Rank Semidefinite Programming written by Alex Lemon and has been published by Now Publishers this book supported file pdf, txt, epub, kindle and other format this book has been release on 2016-05-04 with Mathematics categories.
Finding low-rank solutions of semidefinite programs is important in many applications. For example, semidefinite programs that arise as relaxations of polynomial optimization problems are exact relaxations when the semidefinite program has a rank-1 solution. Unfortunately, computing a minimum-rank solution of a semidefinite program is an NP-hard problem. This monograph reviews the theory of low-rank semidefinite programming, presenting theorems that guarantee the existence of a low-rank solution, heuristics for computing low-rank solutions, and algorithms for finding low-rank approximate solutions. It then presents applications of the theory to trust-region problems and signal processing.