Machine Learning Methods For Multi Omics Data Integration
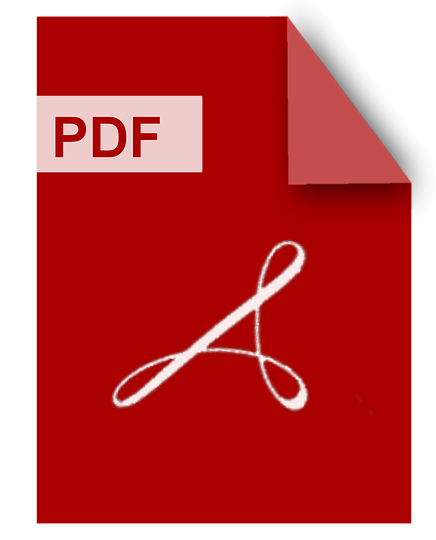
DOWNLOAD
Download Machine Learning Methods For Multi Omics Data Integration PDF/ePub or read online books in Mobi eBooks. Click Download or Read Online button to get Machine Learning Methods For Multi Omics Data Integration book now. This website allows unlimited access to, at the time of writing, more than 1.5 million titles, including hundreds of thousands of titles in various foreign languages. If the content not found or just blank you must refresh this page
Machine Learning Methods For Multi Omics Data Integration
DOWNLOAD
Author : Abedalrhman Alkhateeb
language : en
Publisher: Springer
Release Date : 2023-11-14
Machine Learning Methods For Multi Omics Data Integration written by Abedalrhman Alkhateeb and has been published by Springer this book supported file pdf, txt, epub, kindle and other format this book has been release on 2023-11-14 with Science categories.
The advancement of biomedical engineering has enabled the generation of multi-omics data by developing high-throughput technologies, such as next-generation sequencing, mass spectrometry, and microarrays. Large-scale data sets for multiple omics platforms, including genomics, transcriptomics, proteomics, and metabolomics, have become more accessible and cost-effective over time. Integrating multi-omics data has become increasingly important in many research fields, such as bioinformatics, genomics, and systems biology. This integration allows researchers to understand complex interactions between biological molecules and pathways. It enables us to comprehensively understand complex biological systems, leading to new insights into disease mechanisms, drug discovery, and personalized medicine. Still, integrating various heterogeneous data types into a single learning model also comes with challenges. In this regard, learning algorithms have been vital in analyzing and integrating these large-scale heterogeneous data sets into one learning model. This book overviews the latest multi-omics technologies, machine learning techniques for data integration, and multi-omics databases for validation. It covers different types of learning for supervised and unsupervised learning techniques, including standard classifiers, deep learning, tensor factorization, ensemble learning, and clustering, among others. The book categorizes different levels of integrations, ranging from early, middle, or late-stage among multi-view models. The underlying models target different objectives, such as knowledge discovery, pattern recognition, disease-related biomarkers, and validation tools for multi-omics data. Finally, the book emphasizes practical applications and case studies, making it an essential resource for researchers and practitioners looking to apply machine learning to their multi-omics data sets. The book covers data preprocessing, feature selection, and model evaluation, providing readers with a practical guide to implementing machine learning techniques on various multi-omics data sets.
Methodologies Of Multi Omics Data Integration And Data Mining
DOWNLOAD
Author : Kang Ning
language : en
Publisher: Springer Nature
Release Date : 2023-01-15
Methodologies Of Multi Omics Data Integration And Data Mining written by Kang Ning and has been published by Springer Nature this book supported file pdf, txt, epub, kindle and other format this book has been release on 2023-01-15 with Medical categories.
This book features multi-omics big-data integration and data-mining techniques. In the omics age, paramount of multi-omics data from various sources is the new challenge we are facing, but it also provides clues for several biomedical or clinical applications. This book focuses on data integration and data mining methods for multi-omics research, which explains in detail and with supportive examples the “What”, “Why” and “How” of the topic. The contents are organized into eight chapters, out of which one is for the introduction, followed by four chapters dedicated for omics integration techniques focusing on several omics data resources and data-mining methods, and three chapters dedicated for applications of multi-omics analyses with application being demonstrated by several data mining methods. This book is an attempt to bridge the gap between the biomedical multi-omics big data and the data-mining techniques for the best practice of contemporary bioinformatics and the in-depth insights for the biomedical questions. It would be of interests for the researchers and practitioners who want to conduct the multi-omics studies in cancer, inflammation disease, and microbiome researches.
Application Of Novel Statistical And Machine Learning Methods To High Dimensional Clinical Cancer And Multi Omics Data
DOWNLOAD
Author : Chao Xu
language : en
Publisher: Frontiers Media SA
Release Date : 2022-02-02
Application Of Novel Statistical And Machine Learning Methods To High Dimensional Clinical Cancer And Multi Omics Data written by Chao Xu and has been published by Frontiers Media SA this book supported file pdf, txt, epub, kindle and other format this book has been release on 2022-02-02 with Science categories.
Machine Learning Methods For Multi Omics Data Integration
DOWNLOAD
Author : Abedalrhman Alkhateeb
language : en
Publisher: Springer Nature
Release Date : 2023-12-15
Machine Learning Methods For Multi Omics Data Integration written by Abedalrhman Alkhateeb and has been published by Springer Nature this book supported file pdf, txt, epub, kindle and other format this book has been release on 2023-12-15 with Science categories.
The advancement of biomedical engineering has enabled the generation of multi-omics data by developing high-throughput technologies, such as next-generation sequencing, mass spectrometry, and microarrays. Large-scale data sets for multiple omics platforms, including genomics, transcriptomics, proteomics, and metabolomics, have become more accessible and cost-effective over time. Integrating multi-omics data has become increasingly important in many research fields, such as bioinformatics, genomics, and systems biology. This integration allows researchers to understand complex interactions between biological molecules and pathways. It enables us to comprehensively understand complex biological systems, leading to new insights into disease mechanisms, drug discovery, and personalized medicine. Still, integrating various heterogeneous data types into a single learning model also comes with challenges. In this regard, learning algorithms have been vital in analyzing and integrating these large-scale heterogeneous data sets into one learning model. This book overviews the latest multi-omics technologies, machine learning techniques for data integration, and multi-omics databases for validation. It covers different types of learning for supervised and unsupervised learning techniques, including standard classifiers, deep learning, tensor factorization, ensemble learning, and clustering, among others. The book categorizes different levels of integrations, ranging from early, middle, or late-stage among multi-view models. The underlying models target different objectives, such as knowledge discovery, pattern recognition, disease-related biomarkers, and validation tools for multi-omics data. Finally, the book emphasizes practical applications and case studies, making it an essential resource for researchers and practitioners looking to apply machine learning to their multi-omics data sets. The book covers data preprocessing, feature selection, and model evaluation, providing readers with a practical guide to implementing machine learning techniques on various multi-omics data sets.
Machine Learning Advanced Dynamic Omics Data Analysis For Precision Medicine
DOWNLOAD
Author : Tao Zeng
language : en
Publisher: Frontiers Media SA
Release Date : 2020-03-30
Machine Learning Advanced Dynamic Omics Data Analysis For Precision Medicine written by Tao Zeng and has been published by Frontiers Media SA this book supported file pdf, txt, epub, kindle and other format this book has been release on 2020-03-30 with categories.
Advances In Methods And Tools For Multi Omics Data Analysis
DOWNLOAD
Author : Ornella Cominetti
language : en
Publisher: Frontiers Media SA
Release Date : 2023-05-12
Advances In Methods And Tools For Multi Omics Data Analysis written by Ornella Cominetti and has been published by Frontiers Media SA this book supported file pdf, txt, epub, kindle and other format this book has been release on 2023-05-12 with Science categories.
Learning To Classify Text Using Support Vector Machines
DOWNLOAD
Author : Thorsten Joachims
language : en
Publisher: Springer Science & Business Media
Release Date : 2012-12-06
Learning To Classify Text Using Support Vector Machines written by Thorsten Joachims and has been published by Springer Science & Business Media this book supported file pdf, txt, epub, kindle and other format this book has been release on 2012-12-06 with Computers categories.
Based on ideas from Support Vector Machines (SVMs), Learning To Classify Text Using Support Vector Machines presents a new approach to generating text classifiers from examples. The approach combines high performance and efficiency with theoretical understanding and improved robustness. In particular, it is highly effective without greedy heuristic components. The SVM approach is computationally efficient in training and classification, and it comes with a learning theory that can guide real-world applications. Learning To Classify Text Using Support Vector Machines gives a complete and detailed description of the SVM approach to learning text classifiers, including training algorithms, transductive text classification, efficient performance estimation, and a statistical learning model of text classification. In addition, it includes an overview of the field of text classification, making it self-contained even for newcomers to the field. This book gives a concise introduction to SVMs for pattern recognition, and it includes a detailed description of how to formulate text-classification tasks for machine learning.
Systems Analytics And Integration Of Big Omics Data
DOWNLOAD
Author : Gary Hardiman
language : en
Publisher: MDPI
Release Date : 2020-04-15
Systems Analytics And Integration Of Big Omics Data written by Gary Hardiman and has been published by MDPI this book supported file pdf, txt, epub, kindle and other format this book has been release on 2020-04-15 with Science categories.
A “genotype" is essentially an organism's full hereditary information which is obtained from its parents. A "phenotype" is an organism's actual observed physical and behavioral properties. These may include traits such as morphology, size, height, eye color, metabolism, etc. One of the pressing challenges in computational and systems biology is genotype-to-phenotype prediction. This is challenging given the amount of data generated by modern Omics technologies. This “Big Data” is so large and complex that traditional data processing applications are not up to the task. Challenges arise in collection, analysis, mining, sharing, transfer, visualization, archiving, and integration of these data. In this Special Issue, there is a focus on the systems-level analysis of Omics data, recent developments in gene ontology annotation, and advances in biological pathways and network biology. The integration of Omics data with clinical and biomedical data using machine learning is explored. This Special Issue covers new methodologies in the context of gene–environment interactions, tissue-specific gene expression, and how external factors or host genetics impact the microbiome.
Computational Genomics With R
DOWNLOAD
Author : Altuna Akalin
language : en
Publisher: CRC Press
Release Date : 2020-12-16
Computational Genomics With R written by Altuna Akalin and has been published by CRC Press this book supported file pdf, txt, epub, kindle and other format this book has been release on 2020-12-16 with Mathematics categories.
Computational Genomics with R provides a starting point for beginners in genomic data analysis and also guides more advanced practitioners to sophisticated data analysis techniques in genomics. The book covers topics from R programming, to machine learning and statistics, to the latest genomic data analysis techniques. The text provides accessible information and explanations, always with the genomics context in the background. This also contains practical and well-documented examples in R so readers can analyze their data by simply reusing the code presented. As the field of computational genomics is interdisciplinary, it requires different starting points for people with different backgrounds. For example, a biologist might skip sections on basic genome biology and start with R programming, whereas a computer scientist might want to start with genome biology. After reading: You will have the basics of R and be able to dive right into specialized uses of R for computational genomics such as using Bioconductor packages. You will be familiar with statistics, supervised and unsupervised learning techniques that are important in data modeling, and exploratory analysis of high-dimensional data. You will understand genomic intervals and operations on them that are used for tasks such as aligned read counting and genomic feature annotation. You will know the basics of processing and quality checking high-throughput sequencing data. You will be able to do sequence analysis, such as calculating GC content for parts of a genome or finding transcription factor binding sites. You will know about visualization techniques used in genomics, such as heatmaps, meta-gene plots, and genomic track visualization. You will be familiar with analysis of different high-throughput sequencing data sets, such as RNA-seq, ChIP-seq, and BS-seq. You will know basic techniques for integrating and interpreting multi-omics datasets. Altuna Akalin is a group leader and head of the Bioinformatics and Omics Data Science Platform at the Berlin Institute of Medical Systems Biology, Max Delbrück Center, Berlin. He has been developing computational methods for analyzing and integrating large-scale genomics data sets since 2002. He has published an extensive body of work in this area. The framework for this book grew out of the yearly computational genomics courses he has been organizing and teaching since 2015.
Deep Learning For Biomedical Data Analysis
DOWNLOAD
Author : Mourad Elloumi
language : en
Publisher: Springer Nature
Release Date : 2021-07-13
Deep Learning For Biomedical Data Analysis written by Mourad Elloumi and has been published by Springer Nature this book supported file pdf, txt, epub, kindle and other format this book has been release on 2021-07-13 with Medical categories.
This book is the first overview on Deep Learning (DL) for biomedical data analysis. It surveys the most recent techniques and approaches in this field, with both a broad coverage and enough depth to be of practical use to working professionals. This book offers enough fundamental and technical information on these techniques, approaches and the related problems without overcrowding the reader's head. It presents the results of the latest investigations in the field of DL for biomedical data analysis. The techniques and approaches presented in this book deal with the most important and/or the newest topics encountered in this field. They combine fundamental theory of Artificial Intelligence (AI), Machine Learning (ML) and DL with practical applications in Biology and Medicine. Certainly, the list of topics covered in this book is not exhaustive but these topics will shed light on the implications of the presented techniques and approaches on other topics in biomedical data analysis. The book finds a balance between theoretical and practical coverage of a wide range of issues in the field of biomedical data analysis, thanks to DL. The few published books on DL for biomedical data analysis either focus on specific topics or lack technical depth. The chapters presented in this book were selected for quality and relevance. The book also presents experiments that provide qualitative and quantitative overviews in the field of biomedical data analysis. The reader will require some familiarity with AI, ML and DL and will learn about techniques and approaches that deal with the most important and/or the newest topics encountered in the field of DL for biomedical data analysis. He/she will discover both the fundamentals behind DL techniques and approaches, and their applications on biomedical data. This book can also serve as a reference book for graduate courses in Bioinformatics, AI, ML and DL. The book aims not only at professional researchers and practitioners but also graduate students, senior undergraduate students and young researchers. This book will certainly show the way to new techniques and approaches to make new discoveries.