Maximum Likelihood Estimation In Small Samples
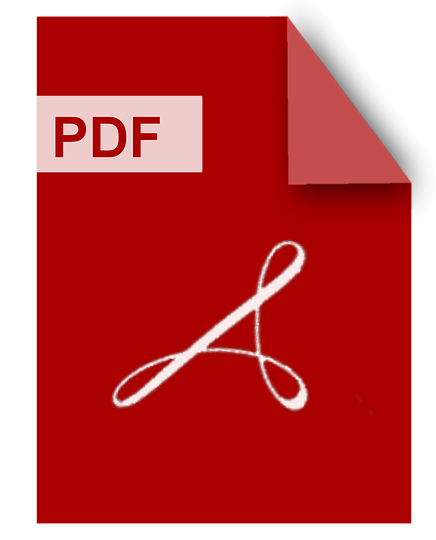
DOWNLOAD
AUDIOBOOK
READ ONLINE
Download Maximum Likelihood Estimation In Small Samples PDF/ePub or read online books in Mobi eBooks. Click Download or Read Online button to get Maximum Likelihood Estimation In Small Samples book now. This website allows unlimited access to, at the time of writing, more than 1.5 million titles, including hundreds of thousands of titles in various foreign languages. If the content not found or just blank you must refresh this page
Maximum Likelihood Estimation In Small Samples
DOWNLOAD
AUDIOBOOK
READ ONLINE
Author : L. R. Shenton
language : en
Publisher:
Release Date : 1977
Maximum Likelihood Estimation In Small Samples written by L. R. Shenton and has been published by this book supported file pdf, txt, epub, kindle and other format this book has been release on 1977 with Estimation theory categories.
Maximum Likelihood Estimation
DOWNLOAD
AUDIOBOOK
READ ONLINE
Author : Scott R. Eliason
language : en
Publisher: SAGE
Release Date : 1993
Maximum Likelihood Estimation written by Scott R. Eliason and has been published by SAGE this book supported file pdf, txt, epub, kindle and other format this book has been release on 1993 with Mathematics categories.
This is a short introduction to Maximum Likelihood (ML) Estimation. It provides a general modeling framework that utilizes the tools of ML methods to outline a flexible modeling strategy that accommodates cases from the simplest linear models (such as the normal error regression model) to the most complex nonlinear models linking endogenous and exogenous variables with non-normal distributions. Using examples to illustrate the techniques of finding ML estimators and estimates, the author discusses what properties are desirable in an estimator, basic techniques for finding maximum likelihood solutions, the general form of the covariance matrix for ML estimates, the sampling distribution of ML estimators; the use of ML in the normal as well as other distributions, and some useful illustrations of likelihoods.
On The Small Sample Properties Of Norm Restricted Maximum Likelihood Estimators For Logistic Regression Models
DOWNLOAD
AUDIOBOOK
READ ONLINE
Author : Diane E. Duffy
language : en
Publisher:
Release Date : 1989
On The Small Sample Properties Of Norm Restricted Maximum Likelihood Estimators For Logistic Regression Models written by Diane E. Duffy and has been published by this book supported file pdf, txt, epub, kindle and other format this book has been release on 1989 with categories.
Estimation Of Sample Size
DOWNLOAD
AUDIOBOOK
READ ONLINE
Author : N. L. Johnson
language : en
Publisher:
Release Date : 1960
Estimation Of Sample Size written by N. L. Johnson and has been published by this book supported file pdf, txt, epub, kindle and other format this book has been release on 1960 with Functions categories.
The problem considered is that of estimating the total number of individuals in a sample, given a number of the observations in the sample ranked either from the greatest or the least. Attention is directed especially to the case when the r̲ smallest values in the sample are known; and the population distribution of the observed character is also known. It is shown that in this case the largest of teh observations (i.e. the r-th smallest in the complete sample) is a sufficient statistic for the sample size. This is also true if the r-th smallest and any subset of the (r-1) smaller observations are available. Methods of estimation by (a) discriminant analysis (b) maximum likelihood, and (c) confidence intervals are discussed. To attain a specified accuracy in distinguishing between two sample sizes n0. n1, where n1/n0 = k, it is found that the required value of r̲ approaches a finite limit (depending on k and the required accuracy) as n0, n1 approach infinity.
Maximum Likelihood Estimation For Sample Surveys
DOWNLOAD
AUDIOBOOK
READ ONLINE
Author : Raymond L. Chambers
language : en
Publisher:
Release Date : 2012
Maximum Likelihood Estimation For Sample Surveys written by Raymond L. Chambers and has been published by this book supported file pdf, txt, epub, kindle and other format this book has been release on 2012 with Sampling (Statistics) categories.
Sample surveys provide data used by researcher in a large range of disciplines to analyze important relationships using well-established and widely-used likelihood methods. The methods used to select samples often result in the sample differing in important ways from the target population and standard application of likelihood methods can lead to biased and inefficient estimates. Maximum Likelihood Estimation for Sample Surveys presents an overview of likelihood methods for the analysis of sample survey data that account for the selection methods used, and includes all necessary background mat.
Econometric Modelling With Time Series
DOWNLOAD
AUDIOBOOK
READ ONLINE
Author : Vance Martin
language : en
Publisher: Cambridge University Press
Release Date : 2013
Econometric Modelling With Time Series written by Vance Martin and has been published by Cambridge University Press this book supported file pdf, txt, epub, kindle and other format this book has been release on 2013 with Business & Economics categories.
"Maximum likelihood estimation is a general method for estimating the parameters of econometric models from observed data. The principle of maximum likelihood plays a central role in the exposition of this book, since a number of estimators used in econometrics can be derived within this framework. Examples include ordinary least squares, generalized least squares and full-information maximum likelihood. In deriving the maximum likelihood estimator, a key concept is the joint probability density function (pdf) of the observed random variables, yt. Maximum likelihood estimation requires that the following conditions are satisfied. (1) The form of the joint pdf of yt is known. (2) The specification of the moments of the joint pdf are known. (3) The joint pdf can be evaluated for all values of the parameters, 9. Parts ONE and TWO of this book deal with models in which all these conditions are satisfied. Part THREE investigates models in which these conditions are not satisfied and considers four important cases. First, if the distribution of yt is misspecified, resulting in both conditions 1 and 2 being violated, estimation is by quasi-maximum likelihood (Chapter 9). Second, if condition 1 is not satisfied, a generalized method of moments estimator (Chapter 10) is required. Third, if condition 2 is not satisfied, estimation relies on nonparametric methods (Chapter 11). Fourth, if condition 3 is violated, simulation-based estimation methods are used (Chapter 12). 1.2 Motivating Examples To highlight the role of probability distributions in maximum likelihood estimation, this section emphasizes the link between observed sample data and 4 The Maximum Likelihood Principle the probability distribution from which they are drawn"-- publisher.
Maximum Likelihood Estimation And Inference
DOWNLOAD
AUDIOBOOK
READ ONLINE
Author : Russell B. Millar
language : en
Publisher: John Wiley & Sons
Release Date : 2011-07-26
Maximum Likelihood Estimation And Inference written by Russell B. Millar and has been published by John Wiley & Sons this book supported file pdf, txt, epub, kindle and other format this book has been release on 2011-07-26 with Mathematics categories.
This book takes a fresh look at the popular and well-established method of maximum likelihood for statistical estimation and inference. It begins with an intuitive introduction to the concepts and background of likelihood, and moves through to the latest developments in maximum likelihood methodology, including general latent variable models and new material for the practical implementation of integrated likelihood using the free ADMB software. Fundamental issues of statistical inference are also examined, with a presentation of some of the philosophical debates underlying the choice of statistical paradigm. Key features: Provides an accessible introduction to pragmatic maximum likelihood modelling. Covers more advanced topics, including general forms of latent variable models (including non-linear and non-normal mixed-effects and state-space models) and the use of maximum likelihood variants, such as estimating equations, conditional likelihood, restricted likelihood and integrated likelihood. Adopts a practical approach, with a focus on providing the relevant tools required by researchers and practitioners who collect and analyze real data. Presents numerous examples and case studies across a wide range of applications including medicine, biology and ecology. Features applications from a range of disciplines, with implementation in R, SAS and/or ADMB. Provides all program code and software extensions on a supporting website. Confines supporting theory to the final chapters to maintain a readable and pragmatic focus of the preceding chapters. This book is not just an accessible and practical text about maximum likelihood, it is a comprehensive guide to modern maximum likelihood estimation and inference. It will be of interest to readers of all levels, from novice to expert. It will be of great benefit to researchers, and to students of statistics from senior undergraduate to graduate level. For use as a course text, exercises are provided at the end of each chapter.
Robust Estimation And Hypothesis Testing
DOWNLOAD
AUDIOBOOK
READ ONLINE
Author : Moti Lal Tiku
language : en
Publisher: New Age International
Release Date : 2004
Robust Estimation And Hypothesis Testing written by Moti Lal Tiku and has been published by New Age International this book supported file pdf, txt, epub, kindle and other format this book has been release on 2004 with Estimation theory categories.
In statistical theory and practice, a certain distribution is usually assumed and then optimal solutions sought. Since deviations from an assumed distribution are very common, one cannot feel comfortable with assuming a particular distribution and believing it to be exactly correct. That brings the robustness issue in focus. In this book, we have given statistical procedures which are robust to plausible deviations from an assumed mode. The method of modified maximum likelihood estimation is used in formulating these procedures. The modified maximum likelihood estimators are explicit functions of sample observations and are easy to compute. They are asymptotically fully efficient and are as efficient as the maximum likelihood estimators for small sample sizes. The maximum likelihood estimators have computational problems and are, therefore, elusive. A broad range of topics are covered in this book. Solutions are given which are easy to implement and are efficient. The solutions are also robust to data anomalies: outliers, inliers, mixtures and data contaminations. Numerous real life applications of the methodology are given.
Statistical Strategies For Small Sample Research
DOWNLOAD
AUDIOBOOK
READ ONLINE
Author : Rick H. Hoyle
language : en
Publisher: SAGE Publications
Release Date : 1999-03-30
Statistical Strategies For Small Sample Research written by Rick H. Hoyle and has been published by SAGE Publications this book supported file pdf, txt, epub, kindle and other format this book has been release on 1999-03-30 with Social Science categories.
Newer statistical models, such as structural equation modeling and hierarchical linear modeling, require large sample sizes inappropriate for many research questions or unrealistic for many research arenas. How can researchers get the sophistication and flexibility of large sample studies without the requirement of prohibitively large samples? This book describes and illustrates statistical strategies that meet the sophistication/flexibility criteria for analyzing data from small samples of fewer than 150 cases. Contributions from some of the leading researchers in the field cover the use of multiple imputation software and how it can be used profitably with small data sets and missing data; ways to increase statistical power when sample size cannot be increased; and strategies for computing effect sizes and combining effect sizes across studies. Other contributions describe how to hypothesis test using the bootstrap; methods for pooling effect size indicators from single-case studies; frameworks for drawing inferences from cross-tabulated data; how to determine whether a correlation or covariance matrix warrants structure analysis; and what conditions indicate latent variable modeling is a viable approach to correct for unreliability in the mediator. Other topics include the use of dynamic factor analysis to model temporal processes by analyzing multivariate; time-series data from small numbers of individuals; techniques for coping with estimation problems in confirmatory factor analysis in small samples; how the state space model can be used with surprising accuracy with small data samples; and the use of partial least squares as a viable alternative to covariance-based SEM when the N is small and/or the number of variables in a model is large.
Small Sample Size Solutions
DOWNLOAD
AUDIOBOOK
READ ONLINE
Author : Rens van de Schoot
language : en
Publisher: Routledge
Release Date : 2020-02-13
Small Sample Size Solutions written by Rens van de Schoot and has been published by Routledge this book supported file pdf, txt, epub, kindle and other format this book has been release on 2020-02-13 with Psychology categories.
Researchers often have difficulties collecting enough data to test their hypotheses, either because target groups are small or hard to access, or because data collection entails prohibitive costs. Such obstacles may result in data sets that are too small for the complexity of the statistical model needed to answer the research question. This unique book provides guidelines and tools for implementing solutions to issues that arise in small sample research. Each chapter illustrates statistical methods that allow researchers to apply the optimal statistical model for their research question when the sample is too small. This essential book will enable social and behavioral science researchers to test their hypotheses even when the statistical model required for answering their research question is too complex for the sample sizes they can collect. The statistical models in the book range from the estimation of a population mean to models with latent variables and nested observations, and solutions include both classical and Bayesian methods. All proposed solutions are described in steps researchers can implement with their own data and are accompanied with annotated syntax in R. The methods described in this book will be useful for researchers across the social and behavioral sciences, ranging from medical sciences and epidemiology to psychology, marketing, and economics.