Nonlinear Dimensionality Reduction
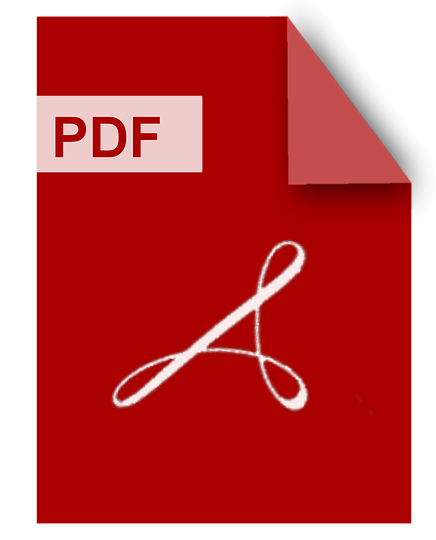
DOWNLOAD
Download Nonlinear Dimensionality Reduction PDF/ePub or read online books in Mobi eBooks. Click Download or Read Online button to get Nonlinear Dimensionality Reduction book now. This website allows unlimited access to, at the time of writing, more than 1.5 million titles, including hundreds of thousands of titles in various foreign languages. If the content not found or just blank you must refresh this page
Nonlinear Dimensionality Reduction
DOWNLOAD
Author : John A. Lee
language : en
Publisher: Springer Science & Business Media
Release Date : 2007-10-31
Nonlinear Dimensionality Reduction written by John A. Lee and has been published by Springer Science & Business Media this book supported file pdf, txt, epub, kindle and other format this book has been release on 2007-10-31 with Mathematics categories.
Methods of dimensionality reduction provide a way to understand and visualize the structure of complex data sets. Traditional methods like principal component analysis and classical metric multidimensional scaling suffer from being based on linear models. Until recently, very few methods were able to reduce the data dimensionality in a nonlinear way. However, since the late nineties, many new methods have been developed and nonlinear dimensionality reduction, also called manifold learning, has become a hot topic. New advances that account for this rapid growth are, e.g. the use of graphs to represent the manifold topology, and the use of new metrics like the geodesic distance. In addition, new optimization schemes, based on kernel techniques and spectral decomposition, have lead to spectral embedding, which encompasses many of the recently developed methods. This book describes existing and advanced methods to reduce the dimensionality of numerical databases. For each method, the description starts from intuitive ideas, develops the necessary mathematical details, and ends by outlining the algorithmic implementation. Methods are compared with each other with the help of different illustrative examples. The purpose of the book is to summarize clear facts and ideas about well-known methods as well as recent developments in the topic of nonlinear dimensionality reduction. With this goal in mind, methods are all described from a unifying point of view, in order to highlight their respective strengths and shortcomings. The book is primarily intended for statisticians, computer scientists and data analysts. It is also accessible to other practitioners having a basic background in statistics and/or computational learning, like psychologists (in psychometry) and economists.
Nonlinear Dimensionality Reduction
DOWNLOAD
Author : Fouad Sabry
language : en
Publisher: One Billion Knowledgeable
Release Date : 2024-12-17
Nonlinear Dimensionality Reduction written by Fouad Sabry and has been published by One Billion Knowledgeable this book supported file pdf, txt, epub, kindle and other format this book has been release on 2024-12-17 with Technology & Engineering categories.
1: Nonlinear dimensionality reduction: Explore foundational concepts and the importance of reducing highdimensional data for easier analysis. 2: Linear map: Introduces the basics of linear mapping and its role in reducing data dimensionality in machine learning. 3: Support vector machine: Learn how support vector machines apply dimensionality reduction in classification tasks and pattern recognition. 4: Principal component analysis: Delve into PCA's technique for transforming data into a set of linearly uncorrelated variables. 5: Isometry: Examine how isometric techniques preserve distances between points while reducing data dimensions. 6: Dimensionality reduction: Understand the broader scope of dimensionality reduction and its applications in various fields. 7: Semidefinite embedding: Study semidefinite programming and its connection to dimensionality reduction methods. 8: Kernel method: Discover the power of kernel methods in handling nonlinear relationships in data reduction. 9: Kernel principal component analysis: Explore KPCA’s capability to perform dimensionality reduction in a highdimensional feature space. 10: Numerical continuation: Learn how numerical continuation techniques assist in understanding highdimensional systems. 11: Spectral clustering: Understand how spectral clustering leverages dimensionality reduction to group similar data points. 12: Isomap: A look at Isomap, a technique that combines multidimensional scaling with geodesic distances for dimensionality reduction. 13: Johnson–Lindenstrauss lemma: Delve into the mathematics of the JohnsonLindenstrauss lemma, which ensures dimensionality reduction maintains geometric properties. 14: LinearnonlinearPoisson cascade model: Study how this model integrates linear and nonlinear methods in dimensionality reduction. 15: Manifold alignment: Learn about manifold alignment and its importance in aligning data from different domains in dimensionality reduction. 16: Diffusion map: Understand how diffusion maps use the diffusion process for dimensionality reduction in complex datasets. 17: Tdistributed stochastic neighbor embedding: Explore tSNE's ability to reduce dimensionality while preserving local structures in data. 18: Kernel embedding of distributions: Study how kernel embedding allows for dimensionality reduction on distributions, not just datasets. 19: Random projection: A practical approach to dimensionality reduction that relies on random projections for fast computation. 20: Manifold regularization: Learn about manifold regularization techniques and their impact on learning from highdimensional data. 21: Empirical dynamic modeling: Discover how empirical dynamic modeling aids in dimensionality reduction through time series data analysis.
Nonlinear Dimensionality Reduction Techniques
DOWNLOAD
Author : Sylvain Lespinats
language : en
Publisher: Springer Nature
Release Date : 2021-12-02
Nonlinear Dimensionality Reduction Techniques written by Sylvain Lespinats and has been published by Springer Nature this book supported file pdf, txt, epub, kindle and other format this book has been release on 2021-12-02 with Computers categories.
This book proposes tools for analysis of multidimensional and metric data, by establishing a state-of-the-art of the existing solutions and developing new ones. It mainly focuses on visual exploration of these data by a human analyst, relying on a 2D or 3D scatter plot display obtained through Dimensionality Reduction. Performing diagnosis of an energy system requires identifying relations between observed monitoring variables and the associated internal state of the system. Dimensionality reduction, which allows to represent visually a multidimensional dataset, constitutes a promising tool to help domain experts to analyse these relations. This book reviews existing techniques for visual data exploration and dimensionality reduction such as tSNE and Isomap, and proposes new solutions to challenges in that field. In particular, it presents the new unsupervised technique ASKI and the supervised methods ClassNeRV and ClassJSE. Moreover, MING, a new approach for local map quality evaluation is also introduced. These methods are then applied to the representation of expert-designed fault indicators for smart-buildings, I-V curves for photovoltaic systems and acoustic signals for Li-ion batteries.
Modern Multidimensional Scaling
DOWNLOAD
Author : I. Borg
language : en
Publisher: Springer Science & Business Media
Release Date : 2005-08-04
Modern Multidimensional Scaling written by I. Borg and has been published by Springer Science & Business Media this book supported file pdf, txt, epub, kindle and other format this book has been release on 2005-08-04 with Social Science categories.
The first edition was released in 1996 and has sold close to 2200 copies. Provides an up-to-date comprehensive treatment of MDS, a statistical technique used to analyze the structure of similarity or dissimilarity data in multidimensional space. The authors have added three chapters and exercise sets. The text is being moved from SSS to SSPP. The book is suitable for courses in statistics for the social or managerial sciences as well as for advanced courses on MDS. All the mathematics required for more advanced topics is developed systematically in the text.
Sufficient Dimension Reduction
DOWNLOAD
Author : Bing Li
language : en
Publisher: CRC Press
Release Date : 2018-04-27
Sufficient Dimension Reduction written by Bing Li and has been published by CRC Press this book supported file pdf, txt, epub, kindle and other format this book has been release on 2018-04-27 with Mathematics categories.
Sufficient dimension reduction is a rapidly developing research field that has wide applications in regression diagnostics, data visualization, machine learning, genomics, image processing, pattern recognition, and medicine, because they are fields that produce large datasets with a large number of variables. Sufficient Dimension Reduction: Methods and Applications with R introduces the basic theories and the main methodologies, provides practical and easy-to-use algorithms and computer codes to implement these methodologies, and surveys the recent advances at the frontiers of this field. Features Provides comprehensive coverage of this emerging research field. Synthesizes a wide variety of dimension reduction methods under a few unifying principles such as projection in Hilbert spaces, kernel mapping, and von Mises expansion. Reflects most recent advances such as nonlinear sufficient dimension reduction, dimension folding for tensorial data, as well as sufficient dimension reduction for functional data. Includes a set of computer codes written in R that are easily implemented by the readers. Uses real data sets available online to illustrate the usage and power of the described methods. Sufficient dimension reduction has undergone momentous development in recent years, partly due to the increased demands for techniques to process high-dimensional data, a hallmark of our age of Big Data. This book will serve as the perfect entry into the field for the beginning researchers or a handy reference for the advanced ones. The author Bing Li obtained his Ph.D. from the University of Chicago. He is currently a Professor of Statistics at the Pennsylvania State University. His research interests cover sufficient dimension reduction, statistical graphical models, functional data analysis, machine learning, estimating equations and quasilikelihood, and robust statistics. He is a fellow of the Institute of Mathematical Statistics and the American Statistical Association. He is an Associate Editor for The Annals of Statistics and the Journal of the American Statistical Association.
Nonlinear Dimensionality Reduction By Manifold Unfolding
DOWNLOAD
Author : Pooyan Khajehpour Tadavani
language : en
Publisher:
Release Date : 2013
Nonlinear Dimensionality Reduction By Manifold Unfolding written by Pooyan Khajehpour Tadavani and has been published by this book supported file pdf, txt, epub, kindle and other format this book has been release on 2013 with categories.
Every second, an enormous volume of data is being gathered from various sources and stored in huge data banks. Most of the time, monitoring a data source requires several parallel measurements, which form a high-dimensional sample vector. Due to the curse of dimensionality, applying machine learning methods, that is, studying and analyzing high-dimensional data, could be difficult. The essential task of dimensionality reduction is to faithfully represent a given set of high-dimensional data samples with a few variables. The goal of this thesis is to develop and propose new techniques for handling high-dimensional data, in order to address contemporary demand in machine learning applications. Most prominent nonlinear dimensionality reduction methods do not explicitly provide a way to handle out-of-samples. The starting point of this thesis is a nonlinear technique, called Embedding by Affine Transformations (EAT), which reduces the dimensionality of out-of-sample data as well. In this method, a convex optimization is solved for estimating a transformation between the high-dimensional input space and the low-dimensional embedding space. To the best of our knowledge, EAT is the only distance-preserving method for nonlinear dimensionality reduction capable of handling out-of-samples. The second method that we propose is TesseraMap. This method is a scalable extension of EAT. Conceptually, TesseraMap partitions the underlying manifold of data into a set of tesserae and then unfolds it by constructing a tessellation in a low-dimensional subspace of the embedding space. Crucially, the desired tessellation is obtained through solving a small semidefinite program; therefore, this method can efficiently handle tens of thousands of data points in a short time. The final outcome of this thesis is a novel method in dimensionality reduction called Isometric Patch Alignment (IPA). Intuitively speaking, IPA first considers a number of overlapping flat patches, which cover the underlying manifold of the high-dimensional input data. Then, IPA rearranges the patches and stitches the neighbors together on their overlapping parts. We prove that stitching two neighboring patches aligns them together; thereby, IPA unfolds the underlying manifold of data. Although this method and TesseraMap have similar approaches, IPA is more scalable; it embeds one million data points in only a few minutes. More importantly, unlike EAT and TesseraMap, which unfold the underlying manifold by stretching it, IPA constructs the unfolded manifold through patch alignment. We show this novel approach is advantageous in many cases. In addition, compared to the other well-known dimensionality reduction methods, IPA has several important characteristics; for example, it is noise tolerant, it handles non-uniform samples, and it can embed non-convex manifolds properly. In addition to these three dimensionality reduction methods, we propose a method for subspace clustering called Low-dimensional Localized Clustering (LDLC). In subspace clustering, data is partitioned into clusters, such that the points of each cluster lie close to a low-dimensional subspace. The unique property of LDLC is that it produces localized clusters on the underlying manifold of data. By conducting several experiments, we show this property is an asset in many machine learning tasks. This method can also be used for local dimensionality reduction. Moreover, LDLC is a suitable tool for forming the tesserae in TesseraMap, and also for creating the patches in IPA.
Data Analytics In Bioinformatics
DOWNLOAD
Author : Rabinarayan Satpathy
language : en
Publisher: John Wiley & Sons
Release Date : 2021-01-20
Data Analytics In Bioinformatics written by Rabinarayan Satpathy and has been published by John Wiley & Sons this book supported file pdf, txt, epub, kindle and other format this book has been release on 2021-01-20 with Computers categories.
Machine learning techniques are increasingly being used to address problems in computational biology and bioinformatics. Novel machine learning computational techniques to analyze high throughput data in the form of sequences, gene and protein expressions, pathways, and images are becoming vital for understanding diseases and future drug discovery. Machine learning techniques such as Markov models, support vector machines, neural networks, and graphical models have been successful in analyzing life science data because of their capabilities in handling randomness and uncertainty of data noise and in generalization. Machine Learning in Bioinformatics compiles recent approaches in machine learning methods and their applications in addressing contemporary problems in bioinformatics approximating classification and prediction of disease, feature selection, dimensionality reduction, gene selection and classification of microarray data and many more.
Elements Of Dimensionality Reduction And Manifold Learning
DOWNLOAD
Author : Benyamin Ghojogh
language : en
Publisher: Springer Nature
Release Date : 2023-02-02
Elements Of Dimensionality Reduction And Manifold Learning written by Benyamin Ghojogh and has been published by Springer Nature this book supported file pdf, txt, epub, kindle and other format this book has been release on 2023-02-02 with Computers categories.
Dimensionality reduction, also known as manifold learning, is an area of machine learning used for extracting informative features from data for better representation of data or separation between classes. This book presents a cohesive review of linear and nonlinear dimensionality reduction and manifold learning. Three main aspects of dimensionality reduction are covered: spectral dimensionality reduction, probabilistic dimensionality reduction, and neural network-based dimensionality reduction, which have geometric, probabilistic, and information-theoretic points of view to dimensionality reduction, respectively. The necessary background and preliminaries on linear algebra, optimization, and kernels are also explained to ensure a comprehensive understanding of the algorithms. The tools introduced in this book can be applied to various applications involving feature extraction, image processing, computer vision, and signal processing. This book is applicable to a wide audience who would like to acquire a deep understanding of the various ways to extract, transform, and understand the structure of data. The intended audiences are academics, students, and industry professionals. Academic researchers and students can use this book as a textbook for machine learning and dimensionality reduction. Data scientists, machine learning scientists, computer vision scientists, and computer scientists can use this book as a reference. It can also be helpful to statisticians in the field of statistical learning and applied mathematicians in the fields of manifolds and subspace analysis. Industry professionals, including applied engineers, data engineers, and engineers in various fields of science dealing with machine learning, can use this as a guidebook for feature extraction from their data, as the raw data in industry often require preprocessing. The book is grounded in theory but provides thorough explanations and diverse examples to improve the reader’s comprehension of the advanced topics. Advanced methods are explained in a step-by-step manner so that readers of all levels can follow the reasoning and come to a deep understanding of the concepts. This book does not assume advanced theoretical background in machine learning and provides necessary background, although an undergraduate-level background in linear algebra and calculus is recommended.
Python Data Science Handbook
DOWNLOAD
Author : Jake VanderPlas
language : en
Publisher: "O'Reilly Media, Inc."
Release Date : 2016-11-21
Python Data Science Handbook written by Jake VanderPlas and has been published by "O'Reilly Media, Inc." this book supported file pdf, txt, epub, kindle and other format this book has been release on 2016-11-21 with Computers categories.
For many researchers, Python is a first-class tool mainly because of its libraries for storing, manipulating, and gaining insight from data. Several resources exist for individual pieces of this data science stack, but only with the Python Data Science Handbook do you get them all—IPython, NumPy, Pandas, Matplotlib, Scikit-Learn, and other related tools. Working scientists and data crunchers familiar with reading and writing Python code will find this comprehensive desk reference ideal for tackling day-to-day issues: manipulating, transforming, and cleaning data; visualizing different types of data; and using data to build statistical or machine learning models. Quite simply, this is the must-have reference for scientific computing in Python. With this handbook, you’ll learn how to use: IPython and Jupyter: provide computational environments for data scientists using Python NumPy: includes the ndarray for efficient storage and manipulation of dense data arrays in Python Pandas: features the DataFrame for efficient storage and manipulation of labeled/columnar data in Python Matplotlib: includes capabilities for a flexible range of data visualizations in Python Scikit-Learn: for efficient and clean Python implementations of the most important and established machine learning algorithms