Robust Model Selection By Cross Validation Via Weighted Likelihood Methodology
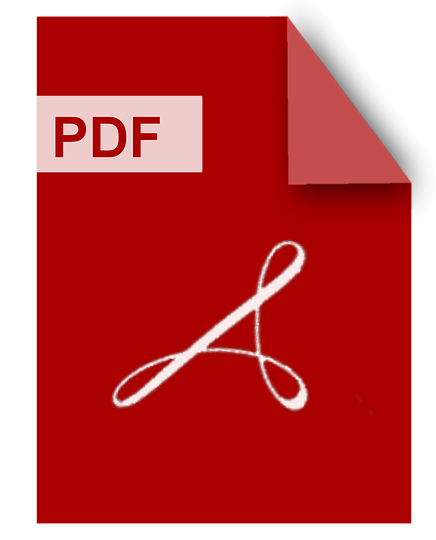
DOWNLOAD
Download Robust Model Selection By Cross Validation Via Weighted Likelihood Methodology PDF/ePub or read online books in Mobi eBooks. Click Download or Read Online button to get Robust Model Selection By Cross Validation Via Weighted Likelihood Methodology book now. This website allows unlimited access to, at the time of writing, more than 1.5 million titles, including hundreds of thousands of titles in various foreign languages. If the content not found or just blank you must refresh this page
Robust Model Selection By Cross Validation Via Weighted Likelihood Methodology
DOWNLOAD
Author : Claudio Agostinelli
language : en
Publisher:
Release Date : 1999
Robust Model Selection By Cross Validation Via Weighted Likelihood Methodology written by Claudio Agostinelli and has been published by this book supported file pdf, txt, epub, kindle and other format this book has been release on 1999 with categories.
Proceedings Of Compstat 2010
DOWNLOAD
Author : Yves Lechevallier
language : en
Publisher: Springer Science & Business Media
Release Date : 2010-11-08
Proceedings Of Compstat 2010 written by Yves Lechevallier and has been published by Springer Science & Business Media this book supported file pdf, txt, epub, kindle and other format this book has been release on 2010-11-08 with Computers categories.
Proceedings of the 19th international symposium on computational statistics, held in Paris august 22-27, 2010.Together with 3 keynote talks, there were 14 invited sessions and more than 100 peer-reviewed contributed communications.
Pattern Recognition
DOWNLOAD
Author : Katrin Franke
language : en
Publisher: Springer Science & Business Media
Release Date : 2006-09-11
Pattern Recognition written by Katrin Franke and has been published by Springer Science & Business Media this book supported file pdf, txt, epub, kindle and other format this book has been release on 2006-09-11 with Computers categories.
This book constitutes the refereed proceedings of the 28th Symposium of the German Association for Pattern Recognition, DAGM 2006. The book presents 32 revised full papers and 44 revised poster papers together with 5 invited papers. Topical sections include image filtering, restoration and segmentation, shape analysis and representation, recognition, categorization and detection, computer vision and image retrieval, machine learning and statistical data analysis, biomedical data analysis, and more.
Journal Of The American Statistical Association
DOWNLOAD
Author :
language : en
Publisher:
Release Date : 2007
Journal Of The American Statistical Association written by and has been published by this book supported file pdf, txt, epub, kindle and other format this book has been release on 2007 with Electronic journals categories.
Discrepancy Based Model Selection Criteria Using Cross Validation
DOWNLOAD
Author : Simon Lee Davies
language : en
Publisher:
Release Date : 2002
Discrepancy Based Model Selection Criteria Using Cross Validation written by Simon Lee Davies and has been published by this book supported file pdf, txt, epub, kindle and other format this book has been release on 2002 with Linear models (Statistics) categories.
An important component of any linear modeling problem consists of determining an appropriate size and form of the design matrix. Improper specification may substantially impact both estimators of the model parameters and predictors of the response variable: underspecification may lead to results which are severely biased, whereas overspecification may lead to results with unnecessarily high variability. Model selection criteria provide a powerful and useful tool for choosing a suitable design matrix. Once a setting has been proposed for an experiment, data can be collected, leading to a set of competing candidate models. One may then attempt to select an appropriate model from this set using a model selection criterion. In this thesis we establish four frameworks which initialize with previously proposed model selection criteria targeting well-known traditional discrepancies, namely the Kullback-Leibler discrepancy, the Gauss discrepancy, the transformed Gauss discrepancy, and the Kullback symmetric discrepancy. These criteria are developed using the bias adjustment approach. Prior work has focused on finding approximately or exactly unbiased estimators of these discrepancies. We expand on this work to additionally show that the criteria which are exactly unbiased serve as the minimum variance unbiased estimators. In many situations, the predictive ability of a candidate model is its most important attribute. In light of our interest in this property, we also concentrate on model selection techniques based on cross validation. New cross validation model selection criteria that serve as counterparts to the standard bias adjusted forms are introduced, together with descriptions of the target discrepancies upon which they are based. We then develop model selection criteria which are minimum variance unbiased estimators of the cross validation discrepancies. Furthermore, we argue that these criteria serve as approximate minimum variance unbiased estimators of the corresponding traditional discrepancies. We propose a general framework to unify and elucidate part of our cross validation criterion development. We show that for the cross validation analogue of a traditional discrepancy, we can always find a "natural" criterion which serves as an exactly unbiased estimator. We study how the cross validation criteria compare to the standard bias adjusted criteria as selection rules in the linear regression framework. This is done by concluding our development of each of the four frameworks with simulation results which illustrate how frequently each criterion identifies the correctly specified model among a sequence of nested fitted candidate models. Our results indicate that the cross validation criteria tend to outperform their bias adjusted counterparts. We close by evaluating the performance of all the model selection criteria considered throughout our work by investigating the results of a simulation study compiled using a sample of data from the Missouri Trauma Registry.
Mixture Models Robustness And The Weighted Likelihood Methodology
DOWNLOAD
Author : Marianthi Markatou
language : en
Publisher:
Release Date : 1998
Mixture Models Robustness And The Weighted Likelihood Methodology written by Marianthi Markatou and has been published by this book supported file pdf, txt, epub, kindle and other format this book has been release on 1998 with categories.
A Penalized Approach To Mixed Model Selection Via Cross Validation
DOWNLOAD
Author : Jingwei Xiong
language : en
Publisher:
Release Date : 2017
A Penalized Approach To Mixed Model Selection Via Cross Validation written by Jingwei Xiong and has been published by this book supported file pdf, txt, epub, kindle and other format this book has been release on 2017 with Linear models (Statistics) categories.
A linear mixed model is a useful technique to explain observations by regarding them as realizations of random variables, especially when repeated measurements are made to statistical units, such as longitudinal data. However, in practice, there are often too many potential factors considered affecting the observations, while actually, they are not. Therefore, statisticians have been trying to select significant factors out of all the potential factors, where we call the process model selection. Among those approaches for linear mixed model selection, penalized methods have been developed profoundly over the last several decades. In this dissertation, to solve the overfitting problem in most penalized methods and improve the selection accuracy, we mainly focus on a penalized approach via cross-validation. Unlike the existing methods using the whole data to fit and select models, we split the fitting process and selection into two stages. More specifically, an adaptive lasso penalized function is customized in the first stage and marginal BIC criterion is used in the second stage. We consider that the main advantage of our approach is to reduce the dependency between models construction and evaluation. Because of the complex structure of mixed models, we adopt a modified Cholesky decomposition to reparameterize the model, which in turn significantly reduces the dimension of the penalized function. Additionally, since random effects are missing, there is no closed form for the maximizer of the penalized function, thus we implement EM algorithm to obtain a full inference of parameters. Furthermore, due to the computation limit and moderately small samples in practice, some noisy factors may still remain in the model, which is particularly obvious for fixed effects. To eliminate the noisy factors, a likelihood ratio test is employed to screen the fixed effects. Regarding the overall process, we call it adaptive lasso via cross-validation. Additionally, we demonstrate that the proposed approach possesses selection and estimation consistency simultaneously. Moreover, simulation studies and real data examples are both provided to justify the method validity. At the very end, a brief conclusion is drawn and some possible further improvements are discussed.
Robust Methods In Biostatistics
DOWNLOAD
Author : Stephane Heritier
language : en
Publisher: John Wiley & Sons
Release Date : 2009-05-11
Robust Methods In Biostatistics written by Stephane Heritier and has been published by John Wiley & Sons this book supported file pdf, txt, epub, kindle and other format this book has been release on 2009-05-11 with Medical categories.
Robust statistics is an extension of classical statistics that specifically takes into account the concept that the underlying models used to describe data are only approximate. Its basic philosophy is to produce statistical procedures which are stable when the data do not exactly match the postulated models as it is the case for example with outliers. Robust Methods in Biostatistics proposes robust alternatives to common methods used in statistics in general and in biostatistics in particular and illustrates their use on many biomedical datasets. The methods introduced include robust estimation, testing, model selection, model check and diagnostics. They are developed for the following general classes of models: Linear regression Generalized linear models Linear mixed models Marginal longitudinal data models Cox survival analysis model The methods are introduced both at a theoretical and applied level within the framework of each general class of models, with a particular emphasis put on practical data analysis. This book is of particular use for research students,applied statisticians and practitioners in the health field interested in more stable statistical techniques. An accompanying website provides R code for computing all of the methods described, as well as for analyzing all the datasets used in the book.
Bayesian Models
DOWNLOAD
Author : N. Thompson Hobbs
language : en
Publisher: Princeton University Press
Release Date : 2015-08-04
Bayesian Models written by N. Thompson Hobbs and has been published by Princeton University Press this book supported file pdf, txt, epub, kindle and other format this book has been release on 2015-08-04 with Science categories.
Bayesian modeling has become an indispensable tool for ecological research because it is uniquely suited to deal with complexity in a statistically coherent way. This textbook provides a comprehensive and accessible introduction to the latest Bayesian methods—in language ecologists can understand. Unlike other books on the subject, this one emphasizes the principles behind the computations, giving ecologists a big-picture understanding of how to implement this powerful statistical approach. Bayesian Models is an essential primer for non-statisticians. It begins with a definition of probability and develops a step-by-step sequence of connected ideas, including basic distribution theory, network diagrams, hierarchical models, Markov chain Monte Carlo, and inference from single and multiple models. This unique book places less emphasis on computer coding, favoring instead a concise presentation of the mathematical statistics needed to understand how and why Bayesian analysis works. It also explains how to write out properly formulated hierarchical Bayesian models and use them in computing, research papers, and proposals. This primer enables ecologists to understand the statistical principles behind Bayesian modeling and apply them to research, teaching, policy, and management. Presents the mathematical and statistical foundations of Bayesian modeling in language accessible to non-statisticians Covers basic distribution theory, network diagrams, hierarchical models, Markov chain Monte Carlo, and more Deemphasizes computer coding in favor of basic principles Explains how to write out properly factored statistical expressions representing Bayesian models
Neural Network Model Selection Using Asymptotic Jackknife Estimator And Cross Validation Method
DOWNLOAD
Author :
language : en
Publisher:
Release Date : 1993
Neural Network Model Selection Using Asymptotic Jackknife Estimator And Cross Validation Method written by and has been published by this book supported file pdf, txt, epub, kindle and other format this book has been release on 1993 with categories.
Two theorems and a lemma are presented about the use of jackknife estimator and the cross-validation method for model selection. Theorem 1 gives the asymptotic form for the jackknife estimator. Combined with the model selection criterion, this asymptotic form can be used to obtain the fit of a model. The model selection criterion we used is the negative of the average predictive likelihood, the choice of which is based on the idea of the cross- validation method. Lemma 1 provides a formula for further exploration of the asymptotics of the model selection criterion. Theorem 2 given an asymptotic form of the model selection criterion for the regression case, when the parameters optimization criterion has a penalty term. Theorem 2 also proves the asymptotic equivalence of Moody's model selection criterion (Moody, 1992) and the cross- validation method, when the distance measure between response y and regression function takes the form of a squared difference ... Neural networks, Model selection, Jackknife, Cross-validation.