Robust Model Selection In Regression Via Weighted Likelihood Methodology
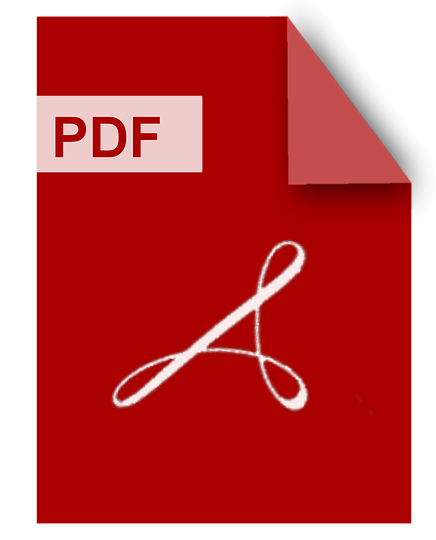
DOWNLOAD
Download Robust Model Selection In Regression Via Weighted Likelihood Methodology PDF/ePub or read online books in Mobi eBooks. Click Download or Read Online button to get Robust Model Selection In Regression Via Weighted Likelihood Methodology book now. This website allows unlimited access to, at the time of writing, more than 1.5 million titles, including hundreds of thousands of titles in various foreign languages. If the content not found or just blank you must refresh this page
Robust Model Selection In Regression Via Weighted Likelihood Methodology
DOWNLOAD
Author : Claudio Agostinelli
language : en
Publisher:
Release Date : 1999
Robust Model Selection In Regression Via Weighted Likelihood Methodology written by Claudio Agostinelli and has been published by this book supported file pdf, txt, epub, kindle and other format this book has been release on 1999 with categories.
Robust Model Selection By Cross Validation Via Weighted Likelihood Methodology
DOWNLOAD
Author : Claudio Agostinelli
language : en
Publisher:
Release Date : 1999
Robust Model Selection By Cross Validation Via Weighted Likelihood Methodology written by Claudio Agostinelli and has been published by this book supported file pdf, txt, epub, kindle and other format this book has been release on 1999 with categories.
Regression And Time Series Model Selection
DOWNLOAD
Author : Allan D. R. McQuarrie
language : en
Publisher: World Scientific
Release Date : 1998
Regression And Time Series Model Selection written by Allan D. R. McQuarrie and has been published by World Scientific this book supported file pdf, txt, epub, kindle and other format this book has been release on 1998 with Mathematics categories.
This important book describes procedures for selecting a model from a large set of competing statistical models. It includes model selection techniques for univariate and multivariate regression models, univariate and multivariate autoregressive models, nonparametric (including wavelets) and semiparametric regression models, and quasi-likelihood and robust regression models. Information-based model selection criteria are discussed, and small sample and asymptotic properties are presented. The book also provides examples and large scale simulation studies comparing the performances of information-based model selection criteria, bootstrapping, and cross-validation selection methods over a wide range of models.
Proceedings Of Compstat 2010
DOWNLOAD
Author : Yves Lechevallier
language : en
Publisher: Springer Science & Business Media
Release Date : 2010-11-08
Proceedings Of Compstat 2010 written by Yves Lechevallier and has been published by Springer Science & Business Media this book supported file pdf, txt, epub, kindle and other format this book has been release on 2010-11-08 with Computers categories.
Proceedings of the 19th international symposium on computational statistics, held in Paris august 22-27, 2010.Together with 3 keynote talks, there were 14 invited sessions and more than 100 peer-reviewed contributed communications.
Statistical Inference
DOWNLOAD
Author : Ayanendranath Basu
language : en
Publisher: CRC Press
Release Date : 2011-06-22
Statistical Inference written by Ayanendranath Basu and has been published by CRC Press this book supported file pdf, txt, epub, kindle and other format this book has been release on 2011-06-22 with Computers categories.
In many ways, estimation by an appropriate minimum distance method is one of the most natural ideas in statistics. However, there are many different ways of constructing an appropriate distance between the data and the model: the scope of study referred to by "Minimum Distance Estimation" is literally huge. Filling a statistical resource gap, Stati
Robust Semiparametric Regression Estimation Using Targeted Maximum Likelihood With Application To Biomarker Discovery And Epidemiology
DOWNLOAD
Author : Catherine Ann Tuglus
language : en
Publisher:
Release Date : 2010
Robust Semiparametric Regression Estimation Using Targeted Maximum Likelihood With Application To Biomarker Discovery And Epidemiology written by Catherine Ann Tuglus and has been published by this book supported file pdf, txt, epub, kindle and other format this book has been release on 2010 with categories.
In many scientific studies the goal is to determine the effect of a particular feature or variable on a given outcome in order to help understand, identify, and quantify the driving factors behind a particular phenomena. This type of analysis is commonly referred to as variable importance analysis. Parametric methods used to estimate these effects are prone to bias. This bias is often the result of incorrect model specification and improper inference for the parameter of interest. Alternative machine learning techniques, such as Random Forest, often result in abstract measures of importance whose inference depends on a computationally intensive bootstrap analysis. In this thesis, robust estimators for variable importance based on targeted maximum likelihood methodology are presented and developed for three types of outcomes (1) univariate continuous, (2) multivariate continuous, and (3) binary outcome. These estimators are specifically designed to target the effect of a variable of interest on an outcome while adjusting for confounders when the variable of interest is of general form (i.e. continuous or discrete). When the outcome is continuous (1,2), the effect is on an additive scale. When the outcome is binary (3), the effect is on a multiplicative scale, and the importance measure is a relative risk. The estimators are developed under a flexible semiparametric model, in which only components related to the variable of interest must be fully specified, and effect modification can be easily incorporated. Based on targeted maximum likelihood theory, the presented estimators are double robust and locally efficient, and correct inference for the parameter of interest is available using the corresponding influence curve. In this thesis, the three estimators relating to the three outcomes are derived from targeted maximum likelihood methodology and implemented by adapting standard statistical regression software. These estimators are applied in both simulation and application. In a simulated biomarker discovery analysis, the robustness of the estimator for a univariate continuous outcome is compared to other common methods of variable importance under increasing correlation among the covariates. In a repeated measures setting, the double robust property of the estimator for a multivariate continuous outcome is demonstrated in simulation, and the estimator is applied in a transcription factor analysis to determine the activity level of transcription factors during the cell cycle in yeast. For a binary outcome, the estimator for the relative risk is applied to estimate the effect of HIV genetic susceptibility scores on viral response. Effect modification is also explored and model selection methodology is introduced.
Modern Methods For Robust Regression
DOWNLOAD
Author : Robert Andersen
language : en
Publisher: SAGE
Release Date : 2008
Modern Methods For Robust Regression written by Robert Andersen and has been published by SAGE this book supported file pdf, txt, epub, kindle and other format this book has been release on 2008 with Mathematics categories.
Offering an in-depth treatment of robust and resistant regression, this volume takes an applied approach and offers readers empirical examples to illustrate key concepts.
Journal Of The American Statistical Association
DOWNLOAD
Author :
language : en
Publisher:
Release Date : 2007
Journal Of The American Statistical Association written by and has been published by this book supported file pdf, txt, epub, kindle and other format this book has been release on 2007 with Electronic journals categories.
Robust Regression Using Maximum Likelihood Weighting And Assuming Cauchy Distributed Random Error
DOWNLOAD
Author : Harry Richard Moore (II.)
language : en
Publisher:
Release Date : 1977
Robust Regression Using Maximum Likelihood Weighting And Assuming Cauchy Distributed Random Error written by Harry Richard Moore (II.) and has been published by this book supported file pdf, txt, epub, kindle and other format this book has been release on 1977 with categories.
Least-squares estimates of regression coefficients are extremely sensitive to large errors in even a single data point. Frequently, an ad-hoc procedure is used to weight the data in a manner of alleviate the effects of extreme observations. This thesis is a study of the effectiveness of an iterative regression method using weights derived through maximum-likelihood arguments. Actual weights are calculated on the assumption of Cauchy-distributed error as a worst-case situation in which the errors have long, fat tails and no finite moments. (Author).
Introduction To Linear Regression Analysis
DOWNLOAD
Author : Douglas C. Montgomery
language : en
Publisher: Wiley-Interscience
Release Date : 2001-04-16
Introduction To Linear Regression Analysis written by Douglas C. Montgomery and has been published by Wiley-Interscience this book supported file pdf, txt, epub, kindle and other format this book has been release on 2001-04-16 with Computers categories.
A comprehensive and thoroughly up-to-date look at regression analysis-still the most widely used technique in statistics today As basic to statistics as the Pythagorean theorem is to geometry, regression analysis is a statistical technique for investigating and modeling the relationship between variables. With far-reaching applications in almost every field, regression analysis is used in engineering, the physical and chemical sciences, economics, management, life and biological sciences, and the social sciences. Clearly balancing theory with applications, Introduction to Linear Regression Analysis describes conventional uses of the technique, as well as less common ones, placing linear regression in the practical context of today's mathematical and scientific research. Beginning with a general introduction to regression modeling, including typical applications, the book then outlines a host of technical tools that form the linear regression analytical arsenal, including: basic inference procedures and introductory aspects of model adequacy checking; how transformations and weighted least squares can be used to resolve problems of model inadequacy; how to deal with influential observations; and polynomial regression models and their variations. Succeeding chapters include detailed coverage of: ? Indicator variables, making the connection between regression and analysis-of-variance modelss ? Variable selection and model-building techniques ? The multicollinearity problem, including its sources, harmful effects, diagnostics, and remedial measures ? Robust regression techniques, including M-estimators, Least Median of Squares, and S-estimation ? Generalized linear models The book also includes material on regression models with autocorrelated errors, bootstrapping regression estimates, classification and regression trees, and regression model validation. Topics not usually found in a linear regression textbook, such as nonlinear regression and generalized linear models, yet critical to engineering students and professionals, have also been included. The new critical role of the computer in regression analysis is reflected in the book's expanded discussion of regression diagnostics, where major analytical procedures now available in contemporary software packages, such as SAS, Minitab, and S-Plus, are detailed. The Appendix now includes ample background material on the theory of linear models underlying regression analysis. Data sets from the book, extensive problem solutions, and software hints are available on the ftp site. For other Wiley books by Doug Montgomery, visit our website at www.wiley.com/college/montgomery.