The Quest For Robust Model Selection Methods In Linear Regression
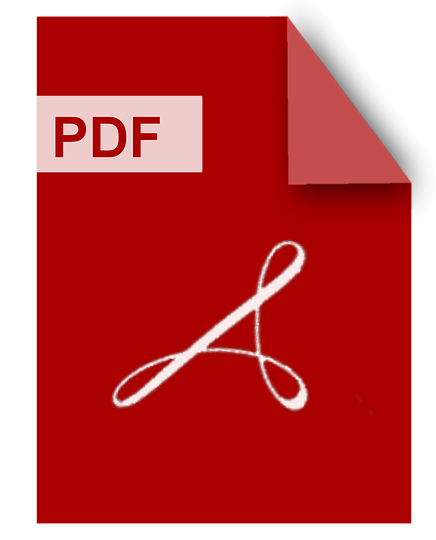
DOWNLOAD
Download The Quest For Robust Model Selection Methods In Linear Regression PDF/ePub or read online books in Mobi eBooks. Click Download or Read Online button to get The Quest For Robust Model Selection Methods In Linear Regression book now. This website allows unlimited access to, at the time of writing, more than 1.5 million titles, including hundreds of thousands of titles in various foreign languages. If the content not found or just blank you must refresh this page
The Quest For Robust Model Selection Methods In Linear Regression
DOWNLOAD
Author : Prakash Borpatra Gohain
language : en
Publisher:
Release Date : 2022
The Quest For Robust Model Selection Methods In Linear Regression written by Prakash Borpatra Gohain and has been published by this book supported file pdf, txt, epub, kindle and other format this book has been release on 2022 with categories.
Regression And Time Series Model Selection
DOWNLOAD
Author : Allan D. R. McQuarrie
language : en
Publisher: World Scientific
Release Date : 1998
Regression And Time Series Model Selection written by Allan D. R. McQuarrie and has been published by World Scientific this book supported file pdf, txt, epub, kindle and other format this book has been release on 1998 with Mathematics categories.
This important book describes procedures for selecting a model from a large set of competing statistical models. It includes model selection techniques for univariate and multivariate regression models, univariate and multivariate autoregressive models, nonparametric (including wavelets) and semiparametric regression models, and quasi-likelihood and robust regression models. Information-based model selection criteria are discussed, and small sample and asymptotic properties are presented. The book also provides examples and large scale simulation studies comparing the performances of information-based model selection criteria, bootstrapping, and cross-validation selection methods over a wide range of models.
Essays On Robust Model Selection And Model Averaging For Linear Models
DOWNLOAD
Author : Le Chang
language : en
Publisher:
Release Date : 2017
Essays On Robust Model Selection And Model Averaging For Linear Models written by Le Chang and has been published by this book supported file pdf, txt, epub, kindle and other format this book has been release on 2017 with categories.
Model selection is central to all applied statistical work. Selecting the variables for use in a regression model is one important example of model selection. This thesis is a collection of essays on robust model selection procedures and model averaging for linear regression models. In the first essay, we propose robust Akaike information criteria (AIC) for MM-estimation and an adjusted robust scale based AIC for M and MM-estimation. Our proposed model selection criteria can maintain their robust properties in the presence of a high proportion of outliers and the outliers in the covariates. We compare our proposed criteria with other robust model selection criteria discussed in previous literature. Our simulation studies demonstrate a significant outperformance of robust AIC based on MM-estimation in the presence of outliers in the covariates. The real data example also shows a better performance of robust AIC based on MM-estimation. The second essay focuses on robust versions of the "Least Absolute Shrinkage and Selection Operator" (lasso). The adaptive lasso is a method for performing simultaneous parameter estimation and variable selection. The adaptive weights used in its penalty term mean that the adaptive lasso achieves the oracle property. In this essay, we propose an extension of the adaptive lasso named the Tukey-lasso. By using Tukey's biweight criterion, instead of squared loss, the Tukey-lasso is resistant to outliers in both the response and covariates. Importantly, we demonstrate that the Tukey-lasso also enjoys the oracle property. A fast accelerated proximal gradient (APG) algorithm is proposed and implemented for computing the Tukey-lasso. Our extensive simulations show that the Tukey-lasso, implemented with the APG algorithm, achieves very reliable results, including for high-dimensional data where p>n. In the presence of outliers, the Tukey-lasso is shown to offer substantial improvements in performance compared to the adaptive lasso and other robust implementations of the lasso. Real data examples further demonstrate the utility of the Tukey-lasso. In many statistical analyses, a single model is used for statistical inference, ignoring the process that leads to the model being selected. To account for this model uncertainty, many model averaging procedures have been proposed. In the last essay, we propose an extension of a bootstrap model averaging approach, called bootstrap lasso averaging (BLA). BLA utilizes the lasso for model selection. This is in contrast to other forms of bootstrap model averaging that use AIC or Bayesian information criteria (BIC). The use of the lasso improves the computation speed and allows BLA to be applied even when the number of variables p is larger than the sample size n. Extensive simulations confirm that BLA has outstanding finite sample performance, in terms of both variable and prediction accuracies, compared with traditional model selection and model averaging methods. Several real data examples further demonstrate an improved out-of-sample predictive performance of BLA.
Robust And Misspecification Resistant Model Selection In Regression Models With Information Complexity And Genetic Algorithms
DOWNLOAD
Author :
language : en
Publisher:
Release Date : 2007
Robust And Misspecification Resistant Model Selection In Regression Models With Information Complexity And Genetic Algorithms written by and has been published by this book supported file pdf, txt, epub, kindle and other format this book has been release on 2007 with categories.
In this dissertation, we develop novel computationally efficient model subset selection methods for multiple and multivariate linear regression models which are both robust and misspecification resistant. Our approach is to use a three-way hybrid method which employs the information theoretic measure of complexity (ICOMP) computed on robust M-estimators as model subset selection criteria, integrated with genetic algorithms (GA) as the subset model searching engine. Despite the rich literature on the robust estimation techniques, bridging the theoretical and applied aspects related to robust model subset selection has been somewhat neglected. A few information criteria in the multiple regression literature are robust. However, none of them is model misspecification resistant and none of them could be generalized to the misspecified multivariate regression. In this dissertation, we introduce for the first time both robust and misspecification resistant information complexity (ICOMP) criterion to fill in the gap in the literature. More specifically in multiple linear regression, we introduce robust M-estimators with misspecification resistant ICOMP and use the new information criterion as the fitness function in GA to carry out the model subset selection. For multivariate linear regression, we derive the two-stage robust Mahalanobis distance (RMD) estimator and introduce this RMD estimator in the computation of information criteria. The new information criteria are used as the fitness function in the GA to perform the model subset selection. Comparative studies on the simulated data for both multiple and multivariate regression show that the robust and misspecification resistant ICOMP outperforms the other robust information criteria and the non-robust ICOMP computed using OLS (or MLE) when the data contain outliers and error terms in the model deviate from a normal distribution. Compared with the all possible model subset selection, GA combined with the robust and misspecification resistant information criteria is proved to be an effective method which can quickly find the a near optimal subset, if not the best, without having to search the whole subset model space.
On Model Selection In Robust Linear Regression
DOWNLOAD
Author : Guoqi Qian
language : en
Publisher:
Release Date : 1996
On Model Selection In Robust Linear Regression written by Guoqi Qian and has been published by this book supported file pdf, txt, epub, kindle and other format this book has been release on 1996 with categories.
Robust Variable Selection In Linear Regression Models
DOWNLOAD
Author : Shokrya Saleha A. Alshqaq
language : en
Publisher:
Release Date : 2015
Robust Variable Selection In Linear Regression Models written by Shokrya Saleha A. Alshqaq and has been published by this book supported file pdf, txt, epub, kindle and other format this book has been release on 2015 with Linear models (Statistics) categories.
Robust Model Selection In Regression
DOWNLOAD
Author : E. Ronchetti
language : en
Publisher:
Release Date : 1984
Robust Model Selection In Regression written by E. Ronchetti and has been published by this book supported file pdf, txt, epub, kindle and other format this book has been release on 1984 with categories.
A robust version of Akaike's model selection procedure for regression models is introduced and its relationship with robust testing procedures is discussed. (Author).
Modern Methods For Robust Regression
DOWNLOAD
Author : Robert Andersen
language : en
Publisher: SAGE
Release Date : 2008
Modern Methods For Robust Regression written by Robert Andersen and has been published by SAGE this book supported file pdf, txt, epub, kindle and other format this book has been release on 2008 with Mathematics categories.
Offering an in-depth treatment of robust and resistant regression, this volume takes an applied approach and offers readers empirical examples to illustrate key concepts.
Linear Regression Analysis
DOWNLOAD
Author : George A. F. Seber
language : en
Publisher: John Wiley & Sons
Release Date : 2012-01-20
Linear Regression Analysis written by George A. F. Seber and has been published by John Wiley & Sons this book supported file pdf, txt, epub, kindle and other format this book has been release on 2012-01-20 with Mathematics categories.
Concise, mathematically clear, and comprehensive treatment of the subject. * Expanded coverage of diagnostics and methods of model fitting. * Requires no specialized knowledge beyond a good grasp of matrix algebra and some acquaintance with straight-line regression and simple analysis of variance models. * More than 200 problems throughout the book plus outline solutions for the exercises. * This revision has been extensively class-tested.
Introduction To Linear Regression Analysis
DOWNLOAD
Author : Douglas C. Montgomery
language : en
Publisher: Wiley-Interscience
Release Date : 2001-04-16
Introduction To Linear Regression Analysis written by Douglas C. Montgomery and has been published by Wiley-Interscience this book supported file pdf, txt, epub, kindle and other format this book has been release on 2001-04-16 with Computers categories.
A comprehensive and thoroughly up-to-date look at regression analysis-still the most widely used technique in statistics today As basic to statistics as the Pythagorean theorem is to geometry, regression analysis is a statistical technique for investigating and modeling the relationship between variables. With far-reaching applications in almost every field, regression analysis is used in engineering, the physical and chemical sciences, economics, management, life and biological sciences, and the social sciences. Clearly balancing theory with applications, Introduction to Linear Regression Analysis describes conventional uses of the technique, as well as less common ones, placing linear regression in the practical context of today's mathematical and scientific research. Beginning with a general introduction to regression modeling, including typical applications, the book then outlines a host of technical tools that form the linear regression analytical arsenal, including: basic inference procedures and introductory aspects of model adequacy checking; how transformations and weighted least squares can be used to resolve problems of model inadequacy; how to deal with influential observations; and polynomial regression models and their variations. Succeeding chapters include detailed coverage of: ? Indicator variables, making the connection between regression and analysis-of-variance modelss ? Variable selection and model-building techniques ? The multicollinearity problem, including its sources, harmful effects, diagnostics, and remedial measures ? Robust regression techniques, including M-estimators, Least Median of Squares, and S-estimation ? Generalized linear models The book also includes material on regression models with autocorrelated errors, bootstrapping regression estimates, classification and regression trees, and regression model validation. Topics not usually found in a linear regression textbook, such as nonlinear regression and generalized linear models, yet critical to engineering students and professionals, have also been included. The new critical role of the computer in regression analysis is reflected in the book's expanded discussion of regression diagnostics, where major analytical procedures now available in contemporary software packages, such as SAS, Minitab, and S-Plus, are detailed. The Appendix now includes ample background material on the theory of linear models underlying regression analysis. Data sets from the book, extensive problem solutions, and software hints are available on the ftp site. For other Wiley books by Doug Montgomery, visit our website at www.wiley.com/college/montgomery.