Robust Model Selection In Regression
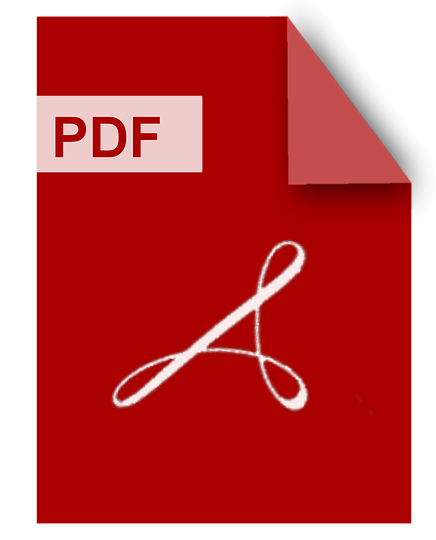
DOWNLOAD
Download Robust Model Selection In Regression PDF/ePub or read online books in Mobi eBooks. Click Download or Read Online button to get Robust Model Selection In Regression book now. This website allows unlimited access to, at the time of writing, more than 1.5 million titles, including hundreds of thousands of titles in various foreign languages. If the content not found or just blank you must refresh this page
The Robustness Of Model Selection Rules
DOWNLOAD
Author : Jochen A. Jungeilges
language : en
Publisher: LIT Verlag Münster
Release Date : 1992
The Robustness Of Model Selection Rules written by Jochen A. Jungeilges and has been published by LIT Verlag Münster this book supported file pdf, txt, epub, kindle and other format this book has been release on 1992 with Business & Economics categories.
Robust Model Selection In Regression
DOWNLOAD
Author : E. Ronchetti
language : en
Publisher:
Release Date : 1984
Robust Model Selection In Regression written by E. Ronchetti and has been published by this book supported file pdf, txt, epub, kindle and other format this book has been release on 1984 with categories.
A robust version of Akaike's model selection procedure for regression models is introduced and its relationship with robust testing procedures is discussed. (Author).
Regression And Time Series Model Selection
DOWNLOAD
Author : Allan D. R. McQuarrie
language : en
Publisher: World Scientific
Release Date : 1998
Regression And Time Series Model Selection written by Allan D. R. McQuarrie and has been published by World Scientific this book supported file pdf, txt, epub, kindle and other format this book has been release on 1998 with Mathematics categories.
This important book describes procedures for selecting a model from a large set of competing statistical models. It includes model selection techniques for univariate and multivariate regression models, univariate and multivariate autoregressive models, nonparametric (including wavelets) and semiparametric regression models, and quasi-likelihood and robust regression models. Information-based model selection criteria are discussed, and small sample and asymptotic properties are presented. The book also provides examples and large scale simulation studies comparing the performances of information-based model selection criteria, bootstrapping, and cross-validation selection methods over a wide range of models.
Essays On Robust Model Selection And Model Averaging For Linear Models
DOWNLOAD
Author : Le Chang
language : en
Publisher:
Release Date : 2017
Essays On Robust Model Selection And Model Averaging For Linear Models written by Le Chang and has been published by this book supported file pdf, txt, epub, kindle and other format this book has been release on 2017 with categories.
Model selection is central to all applied statistical work. Selecting the variables for use in a regression model is one important example of model selection. This thesis is a collection of essays on robust model selection procedures and model averaging for linear regression models. In the first essay, we propose robust Akaike information criteria (AIC) for MM-estimation and an adjusted robust scale based AIC for M and MM-estimation. Our proposed model selection criteria can maintain their robust properties in the presence of a high proportion of outliers and the outliers in the covariates. We compare our proposed criteria with other robust model selection criteria discussed in previous literature. Our simulation studies demonstrate a significant outperformance of robust AIC based on MM-estimation in the presence of outliers in the covariates. The real data example also shows a better performance of robust AIC based on MM-estimation. The second essay focuses on robust versions of the "Least Absolute Shrinkage and Selection Operator" (lasso). The adaptive lasso is a method for performing simultaneous parameter estimation and variable selection. The adaptive weights used in its penalty term mean that the adaptive lasso achieves the oracle property. In this essay, we propose an extension of the adaptive lasso named the Tukey-lasso. By using Tukey's biweight criterion, instead of squared loss, the Tukey-lasso is resistant to outliers in both the response and covariates. Importantly, we demonstrate that the Tukey-lasso also enjoys the oracle property. A fast accelerated proximal gradient (APG) algorithm is proposed and implemented for computing the Tukey-lasso. Our extensive simulations show that the Tukey-lasso, implemented with the APG algorithm, achieves very reliable results, including for high-dimensional data where p>n. In the presence of outliers, the Tukey-lasso is shown to offer substantial improvements in performance compared to the adaptive lasso and other robust implementations of the lasso. Real data examples further demonstrate the utility of the Tukey-lasso. In many statistical analyses, a single model is used for statistical inference, ignoring the process that leads to the model being selected. To account for this model uncertainty, many model averaging procedures have been proposed. In the last essay, we propose an extension of a bootstrap model averaging approach, called bootstrap lasso averaging (BLA). BLA utilizes the lasso for model selection. This is in contrast to other forms of bootstrap model averaging that use AIC or Bayesian information criteria (BIC). The use of the lasso improves the computation speed and allows BLA to be applied even when the number of variables p is larger than the sample size n. Extensive simulations confirm that BLA has outstanding finite sample performance, in terms of both variable and prediction accuracies, compared with traditional model selection and model averaging methods. Several real data examples further demonstrate an improved out-of-sample predictive performance of BLA.
The Quest For Robust Model Selection Methods In Linear Regression
DOWNLOAD
Author : Prakash Borpatra Gohain
language : en
Publisher:
Release Date : 2022
The Quest For Robust Model Selection Methods In Linear Regression written by Prakash Borpatra Gohain and has been published by this book supported file pdf, txt, epub, kindle and other format this book has been release on 2022 with categories.
Robust Regression
DOWNLOAD
Author : Kenneth D. Lawrence
language : en
Publisher: Routledge
Release Date : 2019-05-20
Robust Regression written by Kenneth D. Lawrence and has been published by Routledge this book supported file pdf, txt, epub, kindle and other format this book has been release on 2019-05-20 with Mathematics categories.
Robust Regression: Analysis and Applications characterizes robust estimators in terms of how much they weight each observation discusses generalized properties of Lp-estimators. Includes an algorithm for identifying outliers using least absolute value criterion in regression modeling reviews redescending M-estimators studies Li linear regression proposes the best linear unbiased estimators for fixed parameters and random errors in the mixed linear model summarizes known properties of Li estimators for time series analysis examines ordinary least squares, latent root regression, and a robust regression weighting scheme and evaluates results from five different robust ridge regression estimators.
Robust Model Selection In Regression Via Weighted Likelihood Methodology
DOWNLOAD
Author : Claudio Agostinelli
language : en
Publisher:
Release Date : 1999
Robust Model Selection In Regression Via Weighted Likelihood Methodology written by Claudio Agostinelli and has been published by this book supported file pdf, txt, epub, kindle and other format this book has been release on 1999 with categories.
Robust And Misspecification Resistant Model Selection In Regression Models With Information Complexity And Genetic Algorithms
DOWNLOAD
Author :
language : en
Publisher:
Release Date : 2007
Robust And Misspecification Resistant Model Selection In Regression Models With Information Complexity And Genetic Algorithms written by and has been published by this book supported file pdf, txt, epub, kindle and other format this book has been release on 2007 with categories.
In this dissertation, we develop novel computationally efficient model subset selection methods for multiple and multivariate linear regression models which are both robust and misspecification resistant. Our approach is to use a three-way hybrid method which employs the information theoretic measure of complexity (ICOMP) computed on robust M-estimators as model subset selection criteria, integrated with genetic algorithms (GA) as the subset model searching engine. Despite the rich literature on the robust estimation techniques, bridging the theoretical and applied aspects related to robust model subset selection has been somewhat neglected. A few information criteria in the multiple regression literature are robust. However, none of them is model misspecification resistant and none of them could be generalized to the misspecified multivariate regression. In this dissertation, we introduce for the first time both robust and misspecification resistant information complexity (ICOMP) criterion to fill in the gap in the literature. More specifically in multiple linear regression, we introduce robust M-estimators with misspecification resistant ICOMP and use the new information criterion as the fitness function in GA to carry out the model subset selection. For multivariate linear regression, we derive the two-stage robust Mahalanobis distance (RMD) estimator and introduce this RMD estimator in the computation of information criteria. The new information criteria are used as the fitness function in the GA to perform the model subset selection. Comparative studies on the simulated data for both multiple and multivariate regression show that the robust and misspecification resistant ICOMP outperforms the other robust information criteria and the non-robust ICOMP computed using OLS (or MLE) when the data contain outliers and error terms in the model deviate from a normal distribution. Compared with the all possible model subset selection, GA combined with the robust and misspecification resistant information criteria is proved to be an effective method which can quickly find the a near optimal subset, if not the best, without having to search the whole subset model space.
On Model Selection In Robust Linear Regression
DOWNLOAD
Author : Guoqi Qian
language : en
Publisher:
Release Date : 1996
On Model Selection In Robust Linear Regression written by Guoqi Qian and has been published by this book supported file pdf, txt, epub, kindle and other format this book has been release on 1996 with categories.
Robust Model Selection And Outlier Detection In Linear Regressions
DOWNLOAD
Author : Lauren McCann (Ph. D.)
language : en
Publisher:
Release Date : 2006
Robust Model Selection And Outlier Detection In Linear Regressions written by Lauren McCann (Ph. D.) and has been published by this book supported file pdf, txt, epub, kindle and other format this book has been release on 2006 with categories.
(cont.) Finally, we discuss the problem of outlier detection. In addition to model selection, outliers can adversely influence many other outcomes of regression-based data analysis. We describe a new outlier diagnostic tool, which we call diagnostic data traces. This tool can be used to detect outliers and study their influence on a variety of regression statistics. We demonstrate our tool on several data sets, which are considered benchmarks in the field of outlier detection.