Time Series And Dynamic Models
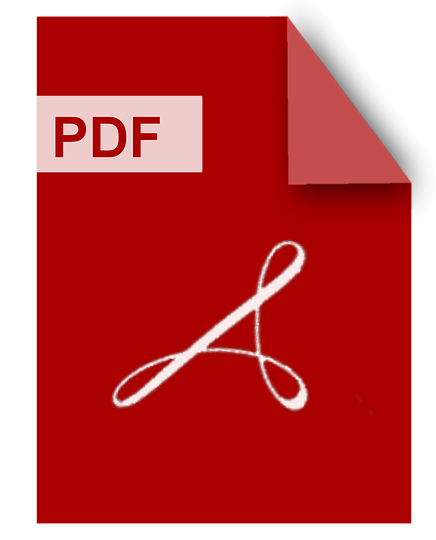
DOWNLOAD
Download Time Series And Dynamic Models PDF/ePub or read online books in Mobi eBooks. Click Download or Read Online button to get Time Series And Dynamic Models book now. This website allows unlimited access to, at the time of writing, more than 1.5 million titles, including hundreds of thousands of titles in various foreign languages. If the content not found or just blank you must refresh this page
Time Series And Dynamic Models
DOWNLOAD
Author : Christian Gourieroux
language : en
Publisher: Cambridge University Press
Release Date : 1997
Time Series And Dynamic Models written by Christian Gourieroux and has been published by Cambridge University Press this book supported file pdf, txt, epub, kindle and other format this book has been release on 1997 with Business & Economics categories.
In this book Christian Gourieroux and Alain Monfort provide an up-to-date and comprehensive analysis of modern time series econometrics. They have succeeded in synthesising in an organised and integrated way a broad and diverse literature. While the book does not assume a deep knowledge of economics, one of its most attractive features is the close attention it pays to economic models and phenomena throughout. The coverage represents a major reference tool for graduate students, researchers and applied economists. The book is divided into four sections. Section one gives a detailed treatment of classical seasonal adjustment or smoothing methods. Section two provides a thorough coverage of various mathematical tools. Section three is the heart of the book, and is devoted to a range of important topics including causality, exogeneity shocks, multipliers, cointegration and fractionally integrated models. The final section describes the main contribution of filtering and smoothing theory to time series econometric problems.
Time Series Models
DOWNLOAD
Author : Manfred Deistler
language : en
Publisher: Springer Nature
Release Date : 2022-10-21
Time Series Models written by Manfred Deistler and has been published by Springer Nature this book supported file pdf, txt, epub, kindle and other format this book has been release on 2022-10-21 with Mathematics categories.
This textbook provides a self-contained presentation of the theory and models of time series analysis. Putting an emphasis on weakly stationary processes and linear dynamic models, it describes the basic concepts, ideas, methods and results in a mathematically well-founded form and includes numerous examples and exercises. The first part presents the theory of weakly stationary processes in time and frequency domain, including prediction and filtering. The second part deals with multivariate AR, ARMA and state space models, which are the most important model classes for stationary processes, and addresses the structure of AR, ARMA and state space systems, Yule-Walker equations, factorization of rational spectral densities and Kalman filtering. Finally, there is a discussion of Granger causality, linear dynamic factor models and (G)ARCH models. The book provides a solid basis for advanced mathematics students and researchers in fields such as data-driven modeling, forecasting and filtering, which are important in statistics, control engineering, financial mathematics, econometrics and signal processing, among other subjects.
Bayesian Forecasting And Dynamic Models
DOWNLOAD
Author : Mike West
language : en
Publisher: Springer Science & Business Media
Release Date : 2013-06-29
Bayesian Forecasting And Dynamic Models written by Mike West and has been published by Springer Science & Business Media this book supported file pdf, txt, epub, kindle and other format this book has been release on 2013-06-29 with Mathematics categories.
In this book we are concerned with Bayesian learning and forecast ing in dynamic environments. We describe the structure and theory of classes of dynamic models, and their uses in Bayesian forecasting. The principles, models and methods of Bayesian forecasting have been developed extensively during the last twenty years. This devel opment has involved thorough investigation of mathematical and sta tistical aspects of forecasting models and related techniques. With this has come experience with application in a variety of areas in commercial and industrial, scientific and socio-economic fields. In deed much of the technical development has been driven by the needs of forecasting practitioners. As a result, there now exists a relatively complete statistical and mathematical framework, although much of this is either not properly documented or not easily accessible. Our primary goals in writing this book have been to present our view of this approach to modelling and forecasting, and to provide a rea sonably complete text for advanced university students and research workers. The text is primarily intended for advanced undergraduate and postgraduate students in statistics and mathematics. In line with this objective we present thorough discussion of mathematical and statistical features of Bayesian analyses of dynamic models, with illustrations, examples and exercises in each Chapter.
Time Series
DOWNLOAD
Author : Raquel Prado
language : en
Publisher: CRC Press
Release Date : 2021-07-27
Time Series written by Raquel Prado and has been published by CRC Press this book supported file pdf, txt, epub, kindle and other format this book has been release on 2021-07-27 with Mathematics categories.
• Expanded on aspects of core model theory and methodology. • Multiple new examples and exercises. • Detailed development of dynamic factor models. • Updated discussion and connections with recent and current research frontiers.
Dynamic Time Series Models Using R Inla
DOWNLOAD
Author : Nalini Ravishanker
language : en
Publisher: CRC Press
Release Date : 2022-08-10
Dynamic Time Series Models Using R Inla written by Nalini Ravishanker and has been published by CRC Press this book supported file pdf, txt, epub, kindle and other format this book has been release on 2022-08-10 with Mathematics categories.
Dynamic Time Series Models using R-INLA: An Applied Perspective is the outcome of a joint effort to systematically describe the use of R-INLA for analysing time series and showcasing the code and description by several examples. This book introduces the underpinnings of R-INLA and the tools needed for modelling different types of time series using an approximate Bayesian framework. The book is an ideal reference for statisticians and scientists who work with time series data. It provides an excellent resource for teaching a course on Bayesian analysis using state space models for time series. Key Features: Introduction and overview of R-INLA for time series analysis. Gaussian and non-Gaussian state space models for time series. State space models for time series with exogenous predictors. Hierarchical models for a potentially large set of time series. Dynamic modelling of stochastic volatility and spatio-temporal dependence.
Bayesian Analysis Of Time Series And Dynamic Models
DOWNLOAD
Author : James Spall
language : en
Publisher: CRC Press
Release Date : 1988-08-24
Bayesian Analysis Of Time Series And Dynamic Models written by James Spall and has been published by CRC Press this book supported file pdf, txt, epub, kindle and other format this book has been release on 1988-08-24 with Mathematics categories.
Contributors present a multidisciplinary overview of the subject covering non-Gaussian models, time and magnitude of changes in dynamic models, image processing based on satellite radiometer measurements, and stationary and nonstationary linear time series models. The topic bridges statistics, econo
State Space Modeling Of Time Series
DOWNLOAD
Author : Masanao Aoki
language : en
Publisher: Springer Science & Business Media
Release Date : 2013-03-09
State Space Modeling Of Time Series written by Masanao Aoki and has been published by Springer Science & Business Media this book supported file pdf, txt, epub, kindle and other format this book has been release on 2013-03-09 with Business & Economics categories.
In this book, the author adopts a state space approach to time series modeling to provide a new, computer-oriented method for building models for vector-valued time series. This second edition has been completely reorganized and rewritten. Background material leading up to the two types of estimators of the state space models is collected and presented coherently in four consecutive chapters. New, fuller descriptions are given of state space models for autoregressive models commonly used in the econometric and statistical literature. Backward innovation models are newly introduced in this edition in addition to the forward innovation models, and both are used to construct instrumental variable estimators for the model matrices. Further new items in this edition include statistical properties of the two types of estimators, more details on multiplier analysis and identification of structural models using estimated models, incorporation of exogenous signals and choice of model size. A whole new chapter is devoted to modeling of integrated, nearly integrated and co-integrated time series.
Time Series Analysis
DOWNLOAD
Author : George E. P. Box
language : en
Publisher: John Wiley & Sons
Release Date : 2015-05-29
Time Series Analysis written by George E. P. Box and has been published by John Wiley & Sons this book supported file pdf, txt, epub, kindle and other format this book has been release on 2015-05-29 with Mathematics categories.
Praise for the Fourth Edition "The book follows faithfully the style of the original edition. The approach is heavily motivated by real-world time series, and by developing a complete approach to model building, estimation, forecasting and control." —Mathematical Reviews Bridging classical models and modern topics, the Fifth Edition of Time Series Analysis: Forecasting and Control maintains a balanced presentation of the tools for modeling and analyzing time series. Also describing the latest developments that have occurred in the field over the past decade through applications from areas such as business, finance, and engineering, the Fifth Edition continues to serve as one of the most influential and prominent works on the subject. Time Series Analysis: Forecasting and Control, Fifth Edition provides a clearly written exploration of the key methods for building, classifying, testing, and analyzing stochastic models for time series and describes their use in five important areas of application: forecasting; determining the transfer function of a system; modeling the effects of intervention events; developing multivariate dynamic models; and designing simple control schemes. Along with these classical uses, the new edition covers modern topics with new features that include: A redesigned chapter on multivariate time series analysis with an expanded treatment of Vector Autoregressive, or VAR models, along with a discussion of the analytical tools needed for modeling vector time series An expanded chapter on special topics covering unit root testing, time-varying volatility models such as ARCH and GARCH, nonlinear time series models, and long memory models Numerous examples drawn from finance, economics, engineering, and other related fields The use of the publicly available R software for graphical illustrations and numerical calculations along with scripts that demonstrate the use of R for model building and forecasting Updates to literature references throughout and new end-of-chapter exercises Streamlined chapter introductions and revisions that update and enhance the exposition Time Series Analysis: Forecasting and Control, Fifth Edition is a valuable real-world reference for researchers and practitioners in time series analysis, econometrics, finance, and related fields. The book is also an excellent textbook for beginning graduate-level courses in advanced statistics, mathematics, economics, finance, engineering, and physics.
Time Series Models
DOWNLOAD
Author : Andrew C. Harvey
language : en
Publisher:
Release Date : 1993
Time Series Models written by Andrew C. Harvey and has been published by this book supported file pdf, txt, epub, kindle and other format this book has been release on 1993 with Econometrics categories.
A companion volume to "The Econometric Analysis of Time" series, this book focuses on the estimation, testing and specification of dynamic models which are not based on any behavioural theory. It covers univariate and multivariate time series and emphasizes autoregressive moving-average processes.
Forecasting Economic Time Series Using Score Driven Dynamic Models With Mixed Data Sampling
DOWNLOAD
Author : Paolo Gorgi
language : en
Publisher:
Release Date : 2018
Forecasting Economic Time Series Using Score Driven Dynamic Models With Mixed Data Sampling written by Paolo Gorgi and has been published by this book supported file pdf, txt, epub, kindle and other format this book has been release on 2018 with categories.
We introduce a mixed-frequency score-driven dynamic model for multiple time series where the score contributions from high-frequency variables are transformed by means of a mixed-data sampling weighting scheme. The resulting dynamic model delivers a flexible and easy-to-implement framework for the forecasting of a low-frequency time series variable through the use of timely information from high-frequency variables. We aim to verify in-sample and out-of-sample performances of the model in an empirical study on the forecasting of U.S.headline inflation. In particular, we forecast monthly inflation using daily oil prices and quarterly inflation using effective federal funds rates. The forecasting results and other findings are promising. Our proposed score-driven dynamic model with mixed-data sampling weighting outperforms competing models in terms of point and density forecasts.