Towards A Privacy Preserving Framework For Publishing Longitudinal Data
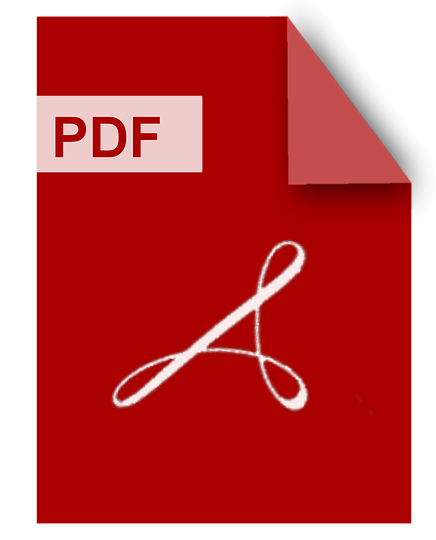
DOWNLOAD
Download Towards A Privacy Preserving Framework For Publishing Longitudinal Data PDF/ePub or read online books in Mobi eBooks. Click Download or Read Online button to get Towards A Privacy Preserving Framework For Publishing Longitudinal Data book now. This website allows unlimited access to, at the time of writing, more than 1.5 million titles, including hundreds of thousands of titles in various foreign languages. If the content not found or just blank you must refresh this page
Towards A Privacy Preserving Framework For Publishing Longitudinal Data
DOWNLOAD
Author : Morvarid Sehatkar
language : en
Publisher:
Release Date : 2014
Towards A Privacy Preserving Framework For Publishing Longitudinal Data written by Morvarid Sehatkar and has been published by this book supported file pdf, txt, epub, kindle and other format this book has been release on 2014 with Data protection categories.
Recent advances in information technology have enabled public organizations and corporations to collect and store huge amounts of individuals' data in data repositories. Such data are powerful sources of information about an individual's life such as interests, activities, and finances. Corporations can employ data mining and knowledge discovery techniques to extract useful knowledge and interesting patterns from large repositories of individuals' data. The extracted knowledge can be exploited to improve strategic decision making, enhance business performance, and improve services. However, person-specific data often contain sensitive information about individuals and publishing such data poses potential privacy risks. To deal with these privacy issues, data must be anonymized so that no sensitive information about individuals can be disclosed from published data while distortion is minimized to ensure usefulness of data in practice. In this thesis, we address privacy concerns in publishing longitudinal data. A data set is longitudinal if it contains information of the same observation or event about individuals collected at several points in time. For instance, the data set of multiple visits of patients of a hospital over a period of time is longitudinal. Due to temporal correlations among the events of each record, potential background knowledge of adversaries about an individual in the context of longitudinal data has specific characteristics. None of the previous anonymization techniques can effectively protect longitudinal data against an adversary with such knowledge. In this thesis we identify the potential privacy threats on longitudinal data and propose a novel framework of anonymization algorithms in a way that protects individuals' privacy against both identity disclosure and attribute disclosure, and preserves data utility. Particularly, we propose two privacy models: (K, C)P̂ -privacy and (K, C)-privacy, and for each of these models we propose efficient algorithms for anonymizing longitudinal data. An extensive experimental study demonstrates that our proposed framework can effectively and efficiently anonymize longitudinal data.
Introduction To Privacy Preserving Data Publishing
DOWNLOAD
Author : Benjamin C.M. Fung
language : en
Publisher: CRC Press
Release Date : 2010-08-02
Introduction To Privacy Preserving Data Publishing written by Benjamin C.M. Fung and has been published by CRC Press this book supported file pdf, txt, epub, kindle and other format this book has been release on 2010-08-02 with Computers categories.
Gaining access to high-quality data is a vital necessity in knowledge-based decision making. But data in its raw form often contains sensitive information about individuals. Providing solutions to this problem, the methods and tools of privacy-preserving data publishing enable the publication of useful information while protecting data privacy. Int
Elgar Encyclopedia Of Law And Data Science
DOWNLOAD
Author : Comandé, Giovanni
language : en
Publisher: Edward Elgar Publishing
Release Date : 2022-02-18
Elgar Encyclopedia Of Law And Data Science written by Comandé, Giovanni and has been published by Edward Elgar Publishing this book supported file pdf, txt, epub, kindle and other format this book has been release on 2022-02-18 with Law categories.
This Encyclopedia brings together jurists, computer scientists, and data analysts to map the emerging field of data science and law for the first time, uncovering the challenges, opportunities, and fault lines that arise as these groups are increasingly thrown together by expanding attempts to regulate and adapt to a data-driven world. It explains the concepts and tools at the crossroads of the many disciplines involved in data science and law, bridging scientific and applied domains. Entries span algorithmic fairness, consent, data protection, ethics, healthcare, machine learning, patents, surveillance, transparency and vulnerability.
Integrating Approaches To Privacy Across The Research Lifecycle
DOWNLOAD
Author :
language : en
Publisher:
Release Date : 2014
Integrating Approaches To Privacy Across The Research Lifecycle written by and has been published by this book supported file pdf, txt, epub, kindle and other format this book has been release on 2014 with Longitudinal method categories.
"This paper explores interdisciplinary approaches to privacy in long-term longitudinal studies of human subjects. Long-term longitudinal studies collect, at multiple points over a long period of time, highly-specific and often sensitive data describing the health, socioeconomic, or behavioral characteristics of human subjects. The value of such studies lies in part in their ability to link a set of behaviors and changes to each individual, but these factors tend to make the combination of observable characteristics associated with each subject unique and potentially identifiable. Using the research information lifecycle as a framework, the paper discusses the defining features of long-term longitudinal studies and the associated challenges for researchers tasked with collecting and analyzing such data while protecting the privacy of human subjects. It also describes the disclosure risks and common legal and technical approaches currently used to manage confidentiality in longitudinal data. Finally, it identifies urgent problems and areas for future research to advance the integration of various methods for preserving confidentiality in research data."--Publisher website.
Privacy Preserving Data Publishing
DOWNLOAD
Author : Raymond Chi-Wing Wong
language : en
Publisher: Springer
Release Date : 2010-02-22
Privacy Preserving Data Publishing written by Raymond Chi-Wing Wong and has been published by Springer this book supported file pdf, txt, epub, kindle and other format this book has been release on 2010-02-22 with Computers categories.
Privacy preservation has become a major issue in many data analysis applications. When a data set is released to other parties for data analysis, privacy-preserving techniques are often required to reduce the possibility of identifying sensitive information about individuals. For example, in medical data, sensitive information can be the fact that a particular patient suffers from HIV. In spatial data, sensitive information can be a specific location of an individual. In web surfing data, the information that a user browses certain websites may be considered sensitive. Consider a dataset containing some sensitive information is to be released to the public. In order to protect sensitive information, the simplest solution is not to disclose the information. However, this would be an overkill since it will hinder the process of data analysis over the data from which we can find interesting patterns. Moreover, in some applications, the data must be disclosed under the government regulations. Alternatively, the data owner can first modify the data such that the modified data can guarantee privacy and, at the same time, the modified data retains sufficient utility and can be released to other parties safely. This process is usually called as privacy-preserving data publishing. In this monograph, we study how the data owner can modify the data and how the modified data can preserve privacy and protect sensitive information. Table of Contents: Introduction / Fundamental Concepts / One-Time Data Publishing / Multiple-Time Data Publishing / Graph Data / Other Data Types / Future Research Directions
Privacy Preserving Data Publishing Using Deep Learning Techniques
DOWNLOAD
Author : Tanbir Ahmed
language : en
Publisher:
Release Date : 2021
Privacy Preserving Data Publishing Using Deep Learning Techniques written by Tanbir Ahmed and has been published by this book supported file pdf, txt, epub, kindle and other format this book has been release on 2021 with categories.
According to a recent study, around 99 percent of hospitals across the United States now use electronic health record systems. One of the most common types of EHR data is unstructured textual data and unlocking hidden details from this data is critical for improving current medical practices and research endeavors. However, these textual data contain sensitive information, which could compromise our privacy. Therefore, medical textual data cannot be released publicly without any privacy protection. De-identification is a process of detecting and removing all sensitive information present in EHRs, and it is a necessary step towards privacy-preserving EHR data sharing. Since 2016, we have seen several deep learning-based approaches for de-identification, which achieved over 98% accuracy. However, these models are trained with sensitive information and can unwittingly memorize some of their training data, and a careful analysis of these models can reveal patients' data. This thesis presents two contributions. First, We introduce new methods to de-identify textual based on self-attention mechanism and stacked Recurrent Neural Network. Experimental results on three different datasets show that our model performs better than all state-of-the-art mechanisms irrespective of the dataset. Additionally, our proposed method is significantly faster than existing techniques. We also introduced three utility metrics to judge the quality of the de-identified data. Second, we propose a differentially private ensemble framework for de-identification, allowing medical researchers to collaborate through publicly publishing the de-identification models. Experiments in three different datasets showed competitive results compared to the state-of-the-art methods with guaranteed differential privacy.
Challenges In Computational Statistics And Data Mining
DOWNLOAD
Author : Stan Matwin
language : en
Publisher: Springer
Release Date : 2015-07-07
Challenges In Computational Statistics And Data Mining written by Stan Matwin and has been published by Springer this book supported file pdf, txt, epub, kindle and other format this book has been release on 2015-07-07 with Technology & Engineering categories.
This volume contains nineteen research papers belonging to the areas of computational statistics, data mining, and their applications. Those papers, all written specifically for this volume, are their authors’ contributions to honour and celebrate Professor Jacek Koronacki on the occcasion of his 70th birthday. The book’s related and often interconnected topics, represent Jacek Koronacki’s research interests and their evolution. They also clearly indicate how close the areas of computational statistics and data mining are.
Toward A Universal Privacy And Information Preserving Framework For Individual Data Exchange
DOWNLOAD
Author : Nicolas Ruiz
language : en
Publisher:
Release Date : 2019
Toward A Universal Privacy And Information Preserving Framework For Individual Data Exchange written by Nicolas Ruiz and has been published by this book supported file pdf, txt, epub, kindle and other format this book has been release on 2019 with categories.
Data on individual subjects, which are increasingly gathered and exchanged, provide a rich amount of information that can inform statistical and policy analysis in a meaningful way. However, due to the legal obligations surrounding such data, this wealth of information is often not fully exploited in order to protect the confidentiality of respondents. The issue is thus the following: how to ensure a sufficient level of data protection to meet releasers' concerns in terms of legal and ethical requirements, while still offering users a reasonable level of information. This question has raised a range concerns about the privacy/information trade-off and has driven a quest for best practices that can be both useful to users but also respectful of individuals' privacy. Statistical disclosure control research has historically provided the analytical apparatus through which the privacy/information trade-off can be assessed and implemented. In recent years, the literature has burgeoned in many directions. In particular, techniques applicable to micro data offer a wide variety of tools to protect the confidentiality of respondents while maximizing the information content of the data released, for the benefit of society at large. Such diversity is undoubtedly useful but has several major drawbacks. In fact, there is currently a clear lack of agreement and clarity as to the appropriate choice of tools in a given context, and as a consequence, there is no comprehensive view (or at best an incomplete one) of the relative performances of the techniques available. The practical scope of current micro data protection methods is not fully exploited precisely because there is no overarching framework: all methods generally carry their own analytical environment, underlying approaches and definitions of privacy and information. Moreover, the evaluation of utility and privacy for each method is metric and data-dependent, meaning that comparisons across different methods and datasets is a daunting task. Against this backdrop, this thesis focuses on establishing some common grounds for individual data anonymization by developing a new, universal approach. Recent contributions to the literature point to the fact that permutations happen to be the essential principle upon which individual data anonymization can be based. In this thesis, we demonstrate that this principle allows for the proposal of a universal analytical environment for data anonymization. The first contribution of this thesis takes an ex-post approach by proposing some universal measures of disclosure risk and information loss that can be computed in a simple fashion and used for the evaluation of any anonymization method, independently of the context under which they operate. In particular, they exhibit distributional independence. These measures establish a common language for comparing different mechanisms, all with potentially varying parametrizations applied to the same data set or to different data sets. The second contribution of this thesis takes an ex-ante approach by developing a new approach to data anonymization. Bringing data anonymization closer to cryptography, it formulates a general cipher based on permutation keys which appears to be equivalent to a general form of rank swapping. Beyond all the existing methods that this cipher can universally reproduce, it also offers a new way to practice data anonymization based on the ex-ante exploration of different permutation structures. The subsequent study of the cipher's properties additionally reveals new insights as to the nature of the task of anonymization taken at a general level of functioning. The final two contributions of this thesis aim at exploring two specific areas using the above results. The first area is longitudinal data anonymization. Despite the fact that the SDC literature offers a wide variety of tools suited to different contexts and data types, there have been very few attempts to deal with the challenges posed by longitudinal data. This thesis thus develops a general framework and some associated metrics of disclosure risk and information loss, tailored to the specific challenges posed by longitudinal data anonymization. Notably, it builds on a permutation approach where the effect of time on time-variant attributes can be seen as an anonymization method that can be captured by temporal permutations. The second area considered is synthetic data. By challenging the information and privacy guarantees of synthetic data, it is shown that any synthetic data set can always be expressed as a permutation of the original data, in a way similar to non-synthetic SDC techniques. In fact, releasing synthetic data sets with the same privacy properties but with an improved level of information appears to be invariably possible as the marginal distributions can always be preserved without increasing risk. On the privacy front, this leads to the consequence that the distinction drawn in the literature between non-synthetic and synthetic data is not so clear-cut. Indeed, it is shown that the practice of releasing several synthetic data sets for a single original data set entails privacy issues that do not arise in non-synthetic anonymization.
Privacy Preserving Data Publishing
DOWNLOAD
Author : Bee-Chung Chen
language : en
Publisher: Now Publishers Inc
Release Date : 2009-10-14
Privacy Preserving Data Publishing written by Bee-Chung Chen and has been published by Now Publishers Inc this book supported file pdf, txt, epub, kindle and other format this book has been release on 2009-10-14 with Data mining categories.
This book is dedicated to those who have something to hide. It is a book about "privacy preserving data publishing" -- the art of publishing sensitive personal data, collected from a group of individuals, in a form that does not violate their privacy. This problem has numerous and diverse areas of application, including releasing Census data, search logs, medical records, and interactions on a social network. The purpose of this book is to provide a detailed overview of the current state of the art as well as open challenges, focusing particular attention on four key themes: RIGOROUS PRIVACY POLICIES Repeated and highly-publicized attacks on published data have demonstrated that simplistic approaches to data publishing do not work. Significant recent advances have exposed the shortcomings of naive (and not-so-naive) techniques. They have also led to the development of mathematically rigorous definitions of privacy that publishing techniques must satisfy; METRICS FOR DATA UTILITY While it is necessary to enforce stringent privacy policies, it is equally important to ensure that the published version of the data is useful for its intended purpose. The authors provide an overview of diverse approaches to measuring data utility; ENFORCEMENT MECHANISMS This book describes in detail various key data publishing mechanisms that guarantee privacy and utility; EMERGING APPLICATIONS The problem of privacy-preserving data publishing arises in diverse application domains with unique privacy and utility requirements. The authors elaborate on the merits and limitations of existing solutions, based on which we expect to see many advances in years to come.
E Democracy Privacy Preserving Secure Intelligent E Government Services
DOWNLOAD
Author : Sokratis K. Katsikas
language : en
Publisher: Springer
Release Date : 2017-11-16
E Democracy Privacy Preserving Secure Intelligent E Government Services written by Sokratis K. Katsikas and has been published by Springer this book supported file pdf, txt, epub, kindle and other format this book has been release on 2017-11-16 with Computers categories.
This book constitutes the refereed proceedings of the 7th International Conference on E-Democracy, E-Democracy 2017, held in Athens, Greece, in December 2017. The 18 revised full papers presented were carefully selected from 44 submissions. The papers are organized in topical sections on e-democracy; privacy; information dissemination and freedom of expression; social networks; electronic identity authentication; ICT in government and in the economy.