Bayesian Modeling In Bioinformatics
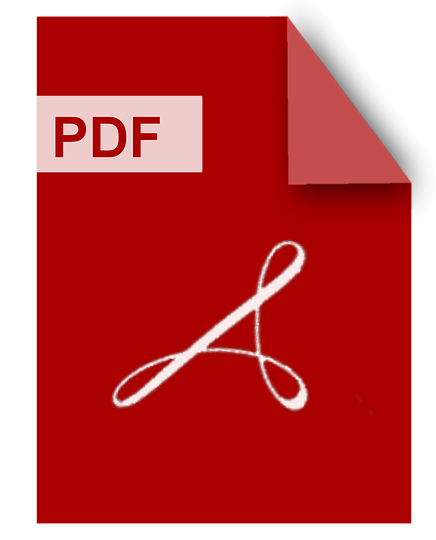
DOWNLOAD
Download Bayesian Modeling In Bioinformatics PDF/ePub or read online books in Mobi eBooks. Click Download or Read Online button to get Bayesian Modeling In Bioinformatics book now. This website allows unlimited access to, at the time of writing, more than 1.5 million titles, including hundreds of thousands of titles in various foreign languages. If the content not found or just blank you must refresh this page
Bayesian Modeling In Bioinformatics
DOWNLOAD
Author : Dipak K. Dey
language : en
Publisher: CRC Press
Release Date : 2010-09-03
Bayesian Modeling In Bioinformatics written by Dipak K. Dey and has been published by CRC Press this book supported file pdf, txt, epub, kindle and other format this book has been release on 2010-09-03 with Mathematics categories.
Bayesian Modeling in Bioinformatics discusses the development and application of Bayesian statistical methods for the analysis of high-throughput bioinformatics data arising from problems in molecular and structural biology and disease-related medical research, such as cancer. It presents a broad overview of statistical inference, clustering, and c
Bayesian Methods In Structural Bioinformatics
DOWNLOAD
Author : Thomas Hamelryck
language : en
Publisher: Springer
Release Date : 2012-03-23
Bayesian Methods In Structural Bioinformatics written by Thomas Hamelryck and has been published by Springer this book supported file pdf, txt, epub, kindle and other format this book has been release on 2012-03-23 with Medical categories.
This book is an edited volume, the goal of which is to provide an overview of the current state-of-the-art in statistical methods applied to problems in structural bioinformatics (and in particular protein structure prediction, simulation, experimental structure determination and analysis). It focuses on statistical methods that have a clear interpretation in the framework of statistical physics, rather than ad hoc, black box methods based on neural networks or support vector machines. In addition, the emphasis is on methods that deal with biomolecular structure in atomic detail. The book is highly accessible, and only assumes background knowledge on protein structure, with a minimum of mathematical knowledge. Therefore, the book includes introductory chapters that contain a solid introduction to key topics such as Bayesian statistics and concepts in machine learning and statistical physics.
Probabilistic Modeling In Bioinformatics And Medical Informatics
DOWNLOAD
Author : Dirk Husmeier
language : en
Publisher: Springer Science & Business Media
Release Date : 2006-05-06
Probabilistic Modeling In Bioinformatics And Medical Informatics written by Dirk Husmeier and has been published by Springer Science & Business Media this book supported file pdf, txt, epub, kindle and other format this book has been release on 2006-05-06 with Computers categories.
Probabilistic Modelling in Bioinformatics and Medical Informatics has been written for researchers and students in statistics, machine learning, and the biological sciences. The first part of this book provides a self-contained introduction to the methodology of Bayesian networks. The following parts demonstrate how these methods are applied in bioinformatics and medical informatics. All three fields - the methodology of probabilistic modeling, bioinformatics, and medical informatics - are evolving very quickly. The text should therefore be seen as an introduction, offering both elementary tutorials as well as more advanced applications and case studies.
Bayesian Inference For Gene Expression And Proteomics
DOWNLOAD
Author : Kim-Anh Do
language : en
Publisher: Cambridge University Press
Release Date : 2006-07-24
Bayesian Inference For Gene Expression And Proteomics written by Kim-Anh Do and has been published by Cambridge University Press this book supported file pdf, txt, epub, kindle and other format this book has been release on 2006-07-24 with Mathematics categories.
Expert overviews of Bayesian methodology, tools and software for multi-platform high-throughput experimentation.
Probabilistic Methods For Bioinformatics
DOWNLOAD
Author : Richard E. Neapolitan
language : en
Publisher: Morgan Kaufmann
Release Date : 2009-06-12
Probabilistic Methods For Bioinformatics written by Richard E. Neapolitan and has been published by Morgan Kaufmann this book supported file pdf, txt, epub, kindle and other format this book has been release on 2009-06-12 with Mathematics categories.
The Bayesian network is one of the most important architectures for representing and reasoning with multivariate probability distributions. When used in conjunction with specialized informatics, possibilities of real-world applications are achieved. Probabilistic Methods for BioInformatics explains the application of probability and statistics, in particular Bayesian networks, to genetics. This book provides background material on probability, statistics, and genetics, and then moves on to discuss Bayesian networks and applications to bioinformatics. Rather than getting bogged down in proofs and algorithms, probabilistic methods used for biological information and Bayesian networks are explained in an accessible way using applications and case studies. The many useful applications of Bayesian networks that have been developed in the past 10 years are discussed. Forming a review of all the significant work in the field that will arguably become the most prevalent method in biological data analysis. - Unique coverage of probabilistic reasoning methods applied to bioinformatics data--those methods that are likely to become the standard analysis tools for bioinformatics. - Shares insights about when and why probabilistic methods can and cannot be used effectively; - Complete review of Bayesian networks and probabilistic methods with a practical approach.
Handbook Of Statistical Genomics
DOWNLOAD
Author : David J. Balding
language : en
Publisher: John Wiley & Sons
Release Date : 2019-07-09
Handbook Of Statistical Genomics written by David J. Balding and has been published by John Wiley & Sons this book supported file pdf, txt, epub, kindle and other format this book has been release on 2019-07-09 with Science categories.
A timely update of a highly popular handbook on statistical genomics This new, two-volume edition of a classic text provides a thorough introduction to statistical genomics, a vital resource for advanced graduate students, early-career researchers and new entrants to the field. It introduces new and updated information on developments that have occurred since the 3rd edition. Widely regarded as the reference work in the field, it features new chapters focusing on statistical aspects of data generated by new sequencing technologies, including sequence-based functional assays. It expands on previous coverage of the many processes between genotype and phenotype, including gene expression and epigenetics, as well as metabolomics. It also examines population genetics and evolutionary models and inference, with new chapters on the multi-species coalescent, admixture and ancient DNA, as well as genetic association studies including causal analyses and variant interpretation. The Handbook of Statistical Genomics focuses on explaining the main ideas, analysis methods and algorithms, citing key recent and historic literature for further details and references. It also includes a glossary of terms, acronyms and abbreviations, and features extensive cross-referencing between chapters, tying the different areas together. With heavy use of up-to-date examples and references to web-based resources, this continues to be a must-have reference in a vital area of research. Provides much-needed, timely coverage of new developments in this expanding area of study Numerous, brand new chapters, for example covering bacterial genomics, microbiome and metagenomics Detailed coverage of application areas, with chapters on plant breeding, conservation and forensic genetics Extensive coverage of human genetic epidemiology, including ethical aspects Edited by one of the leading experts in the field along with rising stars as his co-editors Chapter authors are world-renowned experts in the field, and newly emerging leaders. The Handbook of Statistical Genomics is an excellent introductory text for advanced graduate students and early-career researchers involved in statistical genetics.
Bayesian Modeling And Computation In Python
DOWNLOAD
Author : Osvaldo A. Martin
language : en
Publisher: CRC Press
Release Date : 2021-12-28
Bayesian Modeling And Computation In Python written by Osvaldo A. Martin and has been published by CRC Press this book supported file pdf, txt, epub, kindle and other format this book has been release on 2021-12-28 with Computers categories.
Bayesian Modeling and Computation in Python aims to help beginner Bayesian practitioners to become intermediate modelers. It uses a hands on approach with PyMC3, Tensorflow Probability, ArviZ and other libraries focusing on the practice of applied statistics with references to the underlying mathematical theory. The book starts with a refresher of the Bayesian Inference concepts. The second chapter introduces modern methods for Exploratory Analysis of Bayesian Models. With an understanding of these two fundamentals the subsequent chapters talk through various models including linear regressions, splines, time series, Bayesian additive regression trees. The final chapters include Approximate Bayesian Computation, end to end case studies showing how to apply Bayesian modelling in different settings, and a chapter about the internals of probabilistic programming languages. Finally the last chapter serves as a reference for the rest of the book by getting closer into mathematical aspects or by extending the discussion of certain topics. This book is written by contributors of PyMC3, ArviZ, Bambi, and Tensorflow Probability among other libraries.
New Insights Into Bayesian Inference
DOWNLOAD
Author : Mohammad Saber Fallah Nezhad
language : en
Publisher: BoD – Books on Demand
Release Date : 2018-05-02
New Insights Into Bayesian Inference written by Mohammad Saber Fallah Nezhad and has been published by BoD – Books on Demand this book supported file pdf, txt, epub, kindle and other format this book has been release on 2018-05-02 with Mathematics categories.
This book is an introduction to the mathematical analysis of Bayesian decision-making when the state of the problem is unknown but further data about it can be obtained. The objective of such analysis is to determine the optimal decision or solution that is logically consistent with the preferences of the decision-maker, that can be analyzed using numerical utilities or criteria with the probabilities assigned to the possible state of the problem, such that these probabilities are updated by gathering new information.
Nonparametric Bayesian Inference In Biostatistics
DOWNLOAD
Author : Riten Mitra
language : en
Publisher: Springer
Release Date : 2015-07-25
Nonparametric Bayesian Inference In Biostatistics written by Riten Mitra and has been published by Springer this book supported file pdf, txt, epub, kindle and other format this book has been release on 2015-07-25 with Medical categories.
As chapters in this book demonstrate, BNP has important uses in clinical sciences and inference for issues like unknown partitions in genomics. Nonparametric Bayesian approaches (BNP) play an ever expanding role in biostatistical inference from use in proteomics to clinical trials. Many research problems involve an abundance of data and require flexible and complex probability models beyond the traditional parametric approaches. As this book's expert contributors show, BNP approaches can be the answer. Survival Analysis, in particular survival regression, has traditionally used BNP, but BNP's potential is now very broad. This applies to important tasks like arrangement of patients into clinically meaningful subpopulations and segmenting the genome into functionally distinct regions. This book is designed to both review and introduce application areas for BNP. While existing books provide theoretical foundations, this book connects theory to practice through engaging examples and research questions. Chapters cover: clinical trials, spatial inference, proteomics, genomics, clustering, survival analysis and ROC curve.
Probabilistic Boolean Networks
DOWNLOAD
Author : Ilya Shmulevich
language : en
Publisher: SIAM
Release Date : 2010-01-01
Probabilistic Boolean Networks written by Ilya Shmulevich and has been published by SIAM this book supported file pdf, txt, epub, kindle and other format this book has been release on 2010-01-01 with Mathematics categories.
This is the first comprehensive treatment of probabilistic Boolean networks (PBNs), an important model class for studying genetic regulatory networks. This book covers basic model properties, including the relationships between network structure and dynamics, steady-state analysis, and relationships to other model classes." "Researchers in mathematics, computer science, and engineering are exposed to important applications in systems biology and presented with ample opportunities for developing new approaches and methods. The book is also appropriate for advanced undergraduates, graduate students, and scientists working in the fields of computational biology, genomic signal processing, control and systems theory, and computer science.