Machine Learning For Text
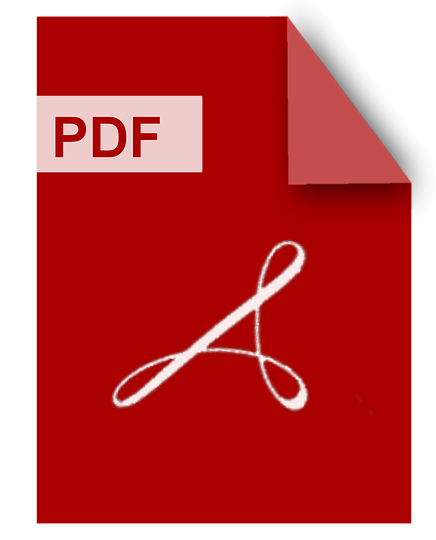
DOWNLOAD
Download Machine Learning For Text PDF/ePub or read online books in Mobi eBooks. Click Download or Read Online button to get Machine Learning For Text book now. This website allows unlimited access to, at the time of writing, more than 1.5 million titles, including hundreds of thousands of titles in various foreign languages. If the content not found or just blank you must refresh this page
Machine Learning For Text
DOWNLOAD
Author : Charu C. Aggarwal
language : en
Publisher: Springer
Release Date : 2018-03-19
Machine Learning For Text written by Charu C. Aggarwal and has been published by Springer this book supported file pdf, txt, epub, kindle and other format this book has been release on 2018-03-19 with Computers categories.
Text analytics is a field that lies on the interface of information retrieval,machine learning, and natural language processing, and this textbook carefully covers a coherently organized framework drawn from these intersecting topics. The chapters of this textbook is organized into three categories: - Basic algorithms: Chapters 1 through 7 discuss the classical algorithms for machine learning from text such as preprocessing, similarity computation, topic modeling, matrix factorization, clustering, classification, regression, and ensemble analysis. - Domain-sensitive mining: Chapters 8 and 9 discuss the learning methods from text when combined with different domains such as multimedia and the Web. The problem of information retrieval and Web search is also discussed in the context of its relationship with ranking and machine learning methods. - Sequence-centric mining: Chapters 10 through 14 discuss various sequence-centric and natural language applications, such as feature engineering, neural language models, deep learning, text summarization, information extraction, opinion mining, text segmentation, and event detection. This textbook covers machine learning topics for text in detail. Since the coverage is extensive,multiple courses can be offered from the same book, depending on course level. Even though the presentation is text-centric, Chapters 3 to 7 cover machine learning algorithms that are often used indomains beyond text data. Therefore, the book can be used to offer courses not just in text analytics but also from the broader perspective of machine learning (with text as a backdrop). This textbook targets graduate students in computer science, as well as researchers, professors, and industrial practitioners working in these related fields. This textbook is accompanied with a solution manual for classroom teaching.
Supervised Machine Learning For Text Analysis In R
DOWNLOAD
Author : Emil Hvitfeldt
language : en
Publisher: CRC Press
Release Date : 2021-11-03
Supervised Machine Learning For Text Analysis In R written by Emil Hvitfeldt and has been published by CRC Press this book supported file pdf, txt, epub, kindle and other format this book has been release on 2021-11-03 with Computers categories.
Text data is important for many domains, from healthcare to marketing to the digital humanities, but specialized approaches are necessary to create features for machine learning from language. Supervised Machine Learning for Text Analysis in R explains how to preprocess text data for modeling, train models, and evaluate model performance using tools from the tidyverse and tidymodels ecosystem. Models like these can be used to make predictions for new observations, to understand what natural language features or characteristics contribute to differences in the output, and more. If you are already familiar with the basics of predictive modeling, use the comprehensive, detailed examples in this book to extend your skills to the domain of natural language processing. This book provides practical guidance and directly applicable knowledge for data scientists and analysts who want to integrate unstructured text data into their modeling pipelines. Learn how to use text data for both regression and classification tasks, and how to apply more straightforward algorithms like regularized regression or support vector machines as well as deep learning approaches. Natural language must be dramatically transformed to be ready for computation, so we explore typical text preprocessing and feature engineering steps like tokenization and word embeddings from the ground up. These steps influence model results in ways we can measure, both in terms of model metrics and other tangible consequences such as how fair or appropriate model results are.
Text Mining With Machine Learning
DOWNLOAD
Author : Jan Žižka
language : en
Publisher: CRC Press
Release Date : 2019-10-31
Text Mining With Machine Learning written by Jan Žižka and has been published by CRC Press this book supported file pdf, txt, epub, kindle and other format this book has been release on 2019-10-31 with Computers categories.
This book provides a perspective on the application of machine learning-based methods in knowledge discovery from natural languages texts. By analysing various data sets, conclusions which are not normally evident, emerge and can be used for various purposes and applications. The book provides explanations of principles of time-proven machine learning algorithms applied in text mining together with step-by-step demonstrations of how to reveal the semantic contents in real-world datasets using the popular R-language with its implemented machine learning algorithms. The book is not only aimed at IT specialists, but is meant for a wider audience that needs to process big sets of text documents and has basic knowledge of the subject, e.g. e-mail service providers, online shoppers, librarians, etc. The book starts with an introduction to text-based natural language data processing and its goals and problems. It focuses on machine learning, presenting various algorithms with their use and possibilities, and reviews the positives and negatives. Beginning with the initial data pre-processing, a reader can follow the steps provided in the R-language including the subsuming of various available plug-ins into the resulting software tool. A big advantage is that R also contains many libraries implementing machine learning algorithms, so a reader can concentrate on the principal target without the need to implement the details of the algorithms her- or himself. To make sense of the results, the book also provides explanations of the algorithms, which supports the final evaluation and interpretation of the results. The examples are demonstrated using realworld data from commonly accessible Internet sources.
Deep Learning
DOWNLOAD
Author : Ian Goodfellow
language : en
Publisher: MIT Press
Release Date : 2016-11-10
Deep Learning written by Ian Goodfellow and has been published by MIT Press this book supported file pdf, txt, epub, kindle and other format this book has been release on 2016-11-10 with Computers categories.
An introduction to a broad range of topics in deep learning, covering mathematical and conceptual background, deep learning techniques used in industry, and research perspectives. “Written by three experts in the field, Deep Learning is the only comprehensive book on the subject.” —Elon Musk, cochair of OpenAI; cofounder and CEO of Tesla and SpaceX Deep learning is a form of machine learning that enables computers to learn from experience and understand the world in terms of a hierarchy of concepts. Because the computer gathers knowledge from experience, there is no need for a human computer operator to formally specify all the knowledge that the computer needs. The hierarchy of concepts allows the computer to learn complicated concepts by building them out of simpler ones; a graph of these hierarchies would be many layers deep. This book introduces a broad range of topics in deep learning. The text offers mathematical and conceptual background, covering relevant concepts in linear algebra, probability theory and information theory, numerical computation, and machine learning. It describes deep learning techniques used by practitioners in industry, including deep feedforward networks, regularization, optimization algorithms, convolutional networks, sequence modeling, and practical methodology; and it surveys such applications as natural language processing, speech recognition, computer vision, online recommendation systems, bioinformatics, and videogames. Finally, the book offers research perspectives, covering such theoretical topics as linear factor models, autoencoders, representation learning, structured probabilistic models, Monte Carlo methods, the partition function, approximate inference, and deep generative models. Deep Learning can be used by undergraduate or graduate students planning careers in either industry or research, and by software engineers who want to begin using deep learning in their products or platforms. A website offers supplementary material for both readers and instructors.
Handbook Of Research On Emerging Trends And Applications Of Machine Learning
DOWNLOAD
Author : Solanki, Arun
language : en
Publisher: IGI Global
Release Date : 2019-12-13
Handbook Of Research On Emerging Trends And Applications Of Machine Learning written by Solanki, Arun and has been published by IGI Global this book supported file pdf, txt, epub, kindle and other format this book has been release on 2019-12-13 with Computers categories.
As today’s world continues to advance, Artificial Intelligence (AI) is a field that has become a staple of technological development and led to the advancement of numerous professional industries. An application within AI that has gained attention is machine learning. Machine learning uses statistical techniques and algorithms to give computer systems the ability to understand and its popularity has circulated through many trades. Understanding this technology and its countless implementations is pivotal for scientists and researchers across the world. The Handbook of Research on Emerging Trends and Applications of Machine Learning provides a high-level understanding of various machine learning algorithms along with modern tools and techniques using Artificial Intelligence. In addition, this book explores the critical role that machine learning plays in a variety of professional fields including healthcare, business, and computer science. While highlighting topics including image processing, predictive analytics, and smart grid management, this book is ideally designed for developers, data scientists, business analysts, information architects, finance agents, healthcare professionals, researchers, retail traders, professors, and graduate students seeking current research on the benefits, implementations, and trends of machine learning.
Applied Text Analysis With Python
DOWNLOAD
Author : Benjamin Bengfort
language : en
Publisher: "O'Reilly Media, Inc."
Release Date : 2018-06-11
Applied Text Analysis With Python written by Benjamin Bengfort and has been published by "O'Reilly Media, Inc." this book supported file pdf, txt, epub, kindle and other format this book has been release on 2018-06-11 with Computers categories.
From news and speeches to informal chatter on social media, natural language is one of the richest and most underutilized sources of data. Not only does it come in a constant stream, always changing and adapting in context; it also contains information that is not conveyed by traditional data sources. The key to unlocking natural language is through the creative application of text analytics. This practical book presents a data scientist’s approach to building language-aware products with applied machine learning. You’ll learn robust, repeatable, and scalable techniques for text analysis with Python, including contextual and linguistic feature engineering, vectorization, classification, topic modeling, entity resolution, graph analysis, and visual steering. By the end of the book, you’ll be equipped with practical methods to solve any number of complex real-world problems. Preprocess and vectorize text into high-dimensional feature representations Perform document classification and topic modeling Steer the model selection process with visual diagnostics Extract key phrases, named entities, and graph structures to reason about data in text Build a dialog framework to enable chatbots and language-driven interaction Use Spark to scale processing power and neural networks to scale model complexity
Text As Data
DOWNLOAD
Author : Justin Grimmer
language : en
Publisher: Princeton University Press
Release Date : 2022-03-29
Text As Data written by Justin Grimmer and has been published by Princeton University Press this book supported file pdf, txt, epub, kindle and other format this book has been release on 2022-03-29 with Computers categories.
A guide for using computational text analysis to learn about the social world From social media posts and text messages to digital government documents and archives, researchers are bombarded with a deluge of text reflecting the social world. This textual data gives unprecedented insights into fundamental questions in the social sciences, humanities, and industry. Meanwhile new machine learning tools are rapidly transforming the way science and business are conducted. Text as Data shows how to combine new sources of data, machine learning tools, and social science research design to develop and evaluate new insights. Text as Data is organized around the core tasks in research projects using text—representation, discovery, measurement, prediction, and causal inference. The authors offer a sequential, iterative, and inductive approach to research design. Each research task is presented complete with real-world applications, example methods, and a distinct style of task-focused research. Bridging many divides—computer science and social science, the qualitative and the quantitative, and industry and academia—Text as Data is an ideal resource for anyone wanting to analyze large collections of text in an era when data is abundant and computation is cheap, but the enduring challenges of social science remain. Overview of how to use text as data Research design for a world of data deluge Examples from across the social sciences and industry
Learning To Classify Text Using Support Vector Machines
DOWNLOAD
Author : Thorsten Joachims
language : en
Publisher: Springer Science & Business Media
Release Date : 2012-12-06
Learning To Classify Text Using Support Vector Machines written by Thorsten Joachims and has been published by Springer Science & Business Media this book supported file pdf, txt, epub, kindle and other format this book has been release on 2012-12-06 with Computers categories.
Based on ideas from Support Vector Machines (SVMs), Learning To Classify Text Using Support Vector Machines presents a new approach to generating text classifiers from examples. The approach combines high performance and efficiency with theoretical understanding and improved robustness. In particular, it is highly effective without greedy heuristic components. The SVM approach is computationally efficient in training and classification, and it comes with a learning theory that can guide real-world applications. Learning To Classify Text Using Support Vector Machines gives a complete and detailed description of the SVM approach to learning text classifiers, including training algorithms, transductive text classification, efficient performance estimation, and a statistical learning model of text classification. In addition, it includes an overview of the field of text classification, making it self-contained even for newcomers to the field. This book gives a concise introduction to SVMs for pattern recognition, and it includes a detailed description of how to formulate text-classification tasks for machine learning.
Interpretable Machine Learning
DOWNLOAD
Author : Christoph Molnar
language : en
Publisher: Lulu.com
Release Date : 2020
Interpretable Machine Learning written by Christoph Molnar and has been published by Lulu.com this book supported file pdf, txt, epub, kindle and other format this book has been release on 2020 with Computers categories.
This book is about making machine learning models and their decisions interpretable. After exploring the concepts of interpretability, you will learn about simple, interpretable models such as decision trees, decision rules and linear regression. Later chapters focus on general model-agnostic methods for interpreting black box models like feature importance and accumulated local effects and explaining individual predictions with Shapley values and LIME. All interpretation methods are explained in depth and discussed critically. How do they work under the hood? What are their strengths and weaknesses? How can their outputs be interpreted? This book will enable you to select and correctly apply the interpretation method that is most suitable for your machine learning project.
Natural Language Processing Recipes
DOWNLOAD
Author : Akshay Kulkarni
language : en
Publisher: Apress
Release Date : 2021-08-26
Natural Language Processing Recipes written by Akshay Kulkarni and has been published by Apress this book supported file pdf, txt, epub, kindle and other format this book has been release on 2021-08-26 with Computers categories.
Focus on implementing end-to-end projects using Python and leverage state-of-the-art algorithms. This book teaches you to efficiently use a wide range of natural language processing (NLP) packages to: implement text classification, identify parts of speech, utilize topic modeling, text summarization, sentiment analysis, information retrieval, and many more applications of NLP. The book begins with text data collection, web scraping, and the different types of data sources. It explains how to clean and pre-process text data, and offers ways to analyze data with advanced algorithms. You then explore semantic and syntactic analysis of the text. Complex NLP solutions that involve text normalization are covered along with advanced pre-processing methods, POS tagging, parsing, text summarization, sentiment analysis, word2vec, seq2seq, and much more. The book presents the fundamentals necessary for applications of machine learning and deep learning in NLP. This second edition goes over advanced techniques to convert text to features such as Glove, Elmo, Bert, etc. It also includes an understanding of how transformers work, taking sentence BERT and GPT as examples. The final chapters explain advanced industrial applications of NLP with solution implementation and leveraging the power of deep learning techniques for NLP problems. It also employs state-of-the-art advanced RNNs, such as long short-term memory, to solve complex text generation tasks. After reading this book, you will have a clear understanding of the challenges faced by different industries and you will have worked on multiple examples of implementing NLP in the real world. What You Will Learn Know the core concepts of implementing NLP and various approaches to natural language processing (NLP), including NLP using Python libraries such as NLTK, textblob, SpaCy, Standford CoreNLP, and more Implement text pre-processing and feature engineering in NLP, including advanced methods of feature engineering Understand and implement the concepts of information retrieval, text summarization, sentiment analysis, text classification, and other advanced NLP techniques leveraging machine learning and deep learning Who This Book Is For Data scientists who want to refresh and learn various concepts of natural language processing (NLP) through coding exercises