Multi Armed Bandit Problem And Application
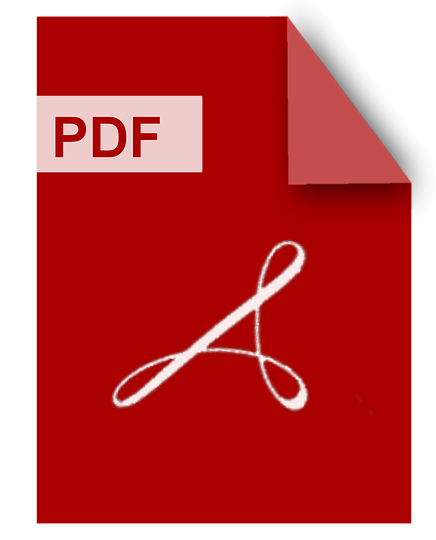
DOWNLOAD
Download Multi Armed Bandit Problem And Application PDF/ePub or read online books in Mobi eBooks. Click Download or Read Online button to get Multi Armed Bandit Problem And Application book now. This website allows unlimited access to, at the time of writing, more than 1.5 million titles, including hundreds of thousands of titles in various foreign languages. If the content not found or just blank you must refresh this page
Multi Armed Bandit Problem And Application
DOWNLOAD
Author : Djallel Bouneffouf
language : en
Publisher: Djallel Bouneffouf
Release Date : 2023-03-14
Multi Armed Bandit Problem And Application written by Djallel Bouneffouf and has been published by Djallel Bouneffouf this book supported file pdf, txt, epub, kindle and other format this book has been release on 2023-03-14 with Computers categories.
In recent years, the multi-armed bandit (MAB) framework has attracted a lot of attention in various applications, from recommender systems and information retrieval to healthcare and finance. This success is due to its stellar performance combined with attractive properties, such as learning from less feedback. The multiarmed bandit field is currently experiencing a renaissance, as novel problem settings and algorithms motivated by various practical applications are being introduced, building on top of the classical bandit problem. This book aims to provide a comprehensive review of top recent developments in multiple real-life applications of the multi-armed bandit. Specifically, we introduce a taxonomy of common MAB-based applications and summarize the state-of-the-art for each of those domains. Furthermore, we identify important current trends and provide new perspectives pertaining to the future of this burgeoning field.
Multi Armed Bandits
DOWNLOAD
Author : Qing Zhao
language : en
Publisher: Synthesis Lectures on Communic
Release Date : 2019-11-21
Multi Armed Bandits written by Qing Zhao and has been published by Synthesis Lectures on Communic this book supported file pdf, txt, epub, kindle and other format this book has been release on 2019-11-21 with Computers categories.
Multi-armed bandit problems pertain to optimal sequential decision making and learning in unknown environments. Since the first bandit problem posed by Thompson in 1933 for the application of clinical trials, bandit problems have enjoyed lasting attention from multiple research communities and have found a wide range of applications across diverse domains. This book covers classic results and recent development on both Bayesian and frequentist bandit problems. We start in Chapter 1 with a brief overview on the history of bandit problems, contrasting the two schools-Bayesian and frequentis -of approaches and highlighting foundational results and key applications. Chapters 2 and 4 cover, respectively, the canonical Bayesian and frequentist bandit models. In Chapters 3 and 5, we discuss major variants of the canonical bandit models that lead to new directions, bring in new techniques, and broaden the applications of this classical problem. In Chapter 6, we present several representative application examples in communication networks and social-economic systems, aiming to illuminate the connections between the Bayesian and the frequentist formulations of bandit problems and how structural results pertaining to one may be leveraged to obtain solutions under the other.
Multi Armed Bandit Allocation Indices
DOWNLOAD
Author : John Gittins
language : en
Publisher: John Wiley & Sons
Release Date : 2011-02-18
Multi Armed Bandit Allocation Indices written by John Gittins and has been published by John Wiley & Sons this book supported file pdf, txt, epub, kindle and other format this book has been release on 2011-02-18 with Mathematics categories.
In 1989 the first edition of this book set out Gittins' pioneering index solution to the multi-armed bandit problem and his subsequent investigation of a wide of sequential resource allocation and stochastic scheduling problems. Since then there has been a remarkable flowering of new insights, generalizations and applications, to which Glazebrook and Weber have made major contributions. This second edition brings the story up to date. There are new chapters on the achievable region approach to stochastic optimization problems, the construction of performance bounds for suboptimal policies, Whittle's restless bandits, and the use of Lagrangian relaxation in the construction and evaluation of index policies. Some of the many varied proofs of the index theorem are discussed along with the insights that they provide. Many contemporary applications are surveyed, and over 150 new references are included. Over the past 40 years the Gittins index has helped theoreticians and practitioners to address a huge variety of problems within chemometrics, economics, engineering, numerical analysis, operational research, probability, statistics and website design. This new edition will be an important resource for others wishing to use this approach.
Introduction To Multi Armed Bandits
DOWNLOAD
Author : Aleksandrs Slivkins
language : en
Publisher:
Release Date : 2019-10-31
Introduction To Multi Armed Bandits written by Aleksandrs Slivkins and has been published by this book supported file pdf, txt, epub, kindle and other format this book has been release on 2019-10-31 with Computers categories.
Multi-armed bandits is a rich, multi-disciplinary area that has been studied since 1933, with a surge of activity in the past 10-15 years. This is the first book to provide a textbook like treatment of the subject.
Bandit Algorithms
DOWNLOAD
Author : Tor Lattimore
language : en
Publisher: Cambridge University Press
Release Date : 2020-07-16
Bandit Algorithms written by Tor Lattimore and has been published by Cambridge University Press this book supported file pdf, txt, epub, kindle and other format this book has been release on 2020-07-16 with Business & Economics categories.
A comprehensive and rigorous introduction for graduate students and researchers, with applications in sequential decision-making problems.
Randomized Sequential Decision Rules
DOWNLOAD
Author : A. R. Abdel Hamid
language : en
Publisher:
Release Date : 1981
Randomized Sequential Decision Rules written by A. R. Abdel Hamid and has been published by this book supported file pdf, txt, epub, kindle and other format this book has been release on 1981 with categories.
Multi Armed Bandits In Large Scale Complex Systems
DOWNLOAD
Author : Xiao Xu
language : en
Publisher:
Release Date : 2020
Multi Armed Bandits In Large Scale Complex Systems written by Xiao Xu and has been published by this book supported file pdf, txt, epub, kindle and other format this book has been release on 2020 with categories.
This dissertation focuses on the multi-armed bandit problem (MAB) where the objective is a sequential arm selection policy that maximizes the total reward over time. In canonical formulations of MAB, the following assumptions are adopted: the size of the action space is much smaller than the length of the time horizon, computation resources such as memory are unlimited in the learning process, and the generative models of arm rewards are time-invariant. This dissertation aims to relax these assumptions, which are unrealistic in emerging applications involving large-scale complex systems, and develop corresponding techniques to address the resulting new issues. The first part of the dissertation aims to address the issue of a massive number of actions. A stochastic bandit problem with side information on arm similarity and dissimilarity is studied. The main results include a unit interval graph (UIG) representation of the action space that succinctly models the side information and a two-step learning structure that fully exploits the topological structure of the UIG to achieve an optimal scaling of the learning cost with the size of the action space. Specifically, in the UIG representation, each node represents an arm and the presence (absence) of an edge between two nodes indicates similarity (dissimilarity) between their mean rewards. Based on whether the UIG is fully revealed by the side information, two settings with complete and partial side information are considered. For each setting, a two-step learning policy consisting of an offline reduction of the action space and online aggregation of reward observations from similar arms is developed. The computation efficiency and the order optimality of the proposed strategies in terms of the size of the action space and the time length are established. Numerical experiments on both synthetic and real-world datasets are conducted to verify the performance of the proposed policies in practice. In the second part of the dissertation, the issue of limited memory during the learning process is studied in the adversarial bandit setting. Specifically, a learning policy can only store the statistics of a subset of arms summarizing their reward history. A general hierarchical learning structure that trades off the regret order with memory complexity is developed based on multi-level partitions of the arm set into groups and the time horizon into epochs. The proposed learning policy requires only a sublinear order of memory space in terms of the number of arms. Its sublinear regret orders with respect to the time horizon are established for both weak regret and shifting regret in expectation and/or with high probability, when appropriate learning strategies are adopted as subroutines at all levels. By properly choosing the number of levels in the adopted hierarchy, the policy adapts to different sizes of the available memory space. A memory-dependent regret bound is established to characterize the tradeoff between memory complexity and the regret performance of the policy. Numerical examples are provided to verify the performance of the policy. The third part of the dissertation focuses on the issue of time-varying rewards within the contextual bandit framework, which finds applications in various online recommendation systems. The main results include two reward models characterizing the fact that the preferences of users toward different items change asynchronously and distinctly, and a learning algorithm that adapts to the dynamic environment. In particular, the two models assume disjoint and hybrid rewards. In the disjoint setting, the mean reward of playing an arm is determined by an arm-specific preference vector, which is piecewise-stationary with asynchronous change times across arms. In the hybrid setting, the mean reward of an arm also depends on a joint coefficient vector shared by all arms representing the time-invariant component of user interests, in addition to the arm-specific one that is time-varying. Two algorithms based on change detection and restarts are developed in the two settings respectively, of which the performance is verified through simulations on both synthetic and real-world data. Theoretical regret analysis of the algorithm with certain modifications is provided under the disjoint reward model, which shows that a near-optimal regret order in the time length is achieved.
Algorithmic Learning Theory
DOWNLOAD
Author : Ricard Gavaldà
language : en
Publisher: Springer
Release Date : 2009-09-29
Algorithmic Learning Theory written by Ricard Gavaldà and has been published by Springer this book supported file pdf, txt, epub, kindle and other format this book has been release on 2009-09-29 with Computers categories.
This book constitutes the refereed proceedings of the 20th International Conference on Algorithmic Learning Theory, ALT 2009, held in Porto, Portugal, in October 2009, co-located with the 12th International Conference on Discovery Science, DS 2009. The 26 revised full papers presented together with the abstracts of 5 invited talks were carefully reviewed and selected from 60 submissions. The papers are divided into topical sections of papers on online learning, learning graphs, active learning and query learning, statistical learning, inductive inference, and semisupervised and unsupervised learning. The volume also contains abstracts of the invited talks: Sanjoy Dasgupta, The Two Faces of Active Learning; Hector Geffner, Inference and Learning in Planning; Jiawei Han, Mining Heterogeneous; Information Networks By Exploring the Power of Links, Yishay Mansour, Learning and Domain Adaptation; Fernando C.N. Pereira, Learning on the Web.
Regret Analysis Of Stochastic And Nonstochastic Multi Armed Bandit Problems
DOWNLOAD
Author : Sébastien Bubeck
language : en
Publisher: Now Pub
Release Date : 2012
Regret Analysis Of Stochastic And Nonstochastic Multi Armed Bandit Problems written by Sébastien Bubeck and has been published by Now Pub this book supported file pdf, txt, epub, kindle and other format this book has been release on 2012 with Computers categories.
In this monograph, the focus is on two extreme cases in which the analysis of regret is particularly simple and elegant: independent and identically distributed payoffs and adversarial payoffs. Besides the basic setting of finitely many actions, it analyzes some of the most important variants and extensions, such as the contextual bandit model.
Regulating Exploration In Multi Armed Bandit Problems With Time Patterns And Dying Arms
DOWNLOAD
Author : Stefano Tracà
language : en
Publisher:
Release Date : 2018
Regulating Exploration In Multi Armed Bandit Problems With Time Patterns And Dying Arms written by Stefano Tracà and has been published by this book supported file pdf, txt, epub, kindle and other format this book has been release on 2018 with categories.
In retail, there are predictable yet dramatic time-dependent patterns in customer behavior, such as periodic changes in the number of visitors, or increases in customers just before major holidays. The standard paradigm of multi-armed bandit analysis does not take these known patterns into account. This means that for applications in retail, where prices are fixed for periods of time, current bandit algorithms will not suffice. This work provides a framework and methods that take the time-dependent patterns into account. In the corrected methods, exploitation (greed) is regulated over time, so that more exploitation occurs during higher reward periods, and more exploration occurs in periods of low reward. In order to understand why regret is reduced with the corrected methods, a set of bounds on the expected regret are presented and insights into why we would want to exploit during periods of high reward are discussed. When the set of available options changes over time, mortal bandits algorithms have proven to be extremely useful in a number of settings, for example, for providing news article recommendations, or running automated online advertising campaigns. Previous work on this problem showed how to regulate exploration of new arms when they have recently appeared, but they do not adapt when the arms are about to disappear. Since in most applications we can determine either exactly or approximately when arms will disappear, we can leverage this information to improve performance: we should not be exploring arms that are about to disappear. Also for this framework, adaptations of algorithms and regret bounds are provided. The proposed methods perform well in experiments, and were inspired by a high-scoring entry in the Exploration and Exploitation 3 contest using data from Yahoo! Front Page. That entry heavily used time-series methods to regulate greed over time, which was substantially more effective than other contextual bandit methods.