Robust Mixed Model Analysis
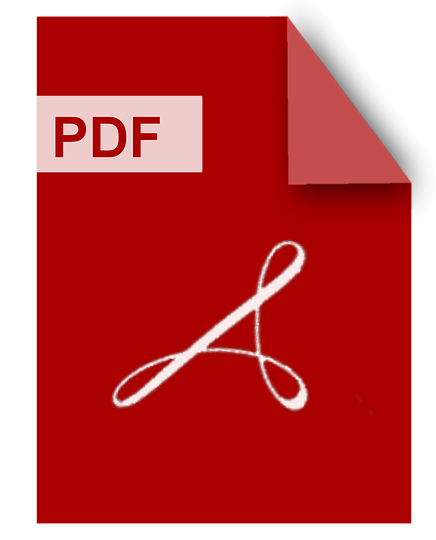
DOWNLOAD
Download Robust Mixed Model Analysis PDF/ePub or read online books in Mobi eBooks. Click Download or Read Online button to get Robust Mixed Model Analysis book now. This website allows unlimited access to, at the time of writing, more than 1.5 million titles, including hundreds of thousands of titles in various foreign languages. If the content not found or just blank you must refresh this page
Robust Mixed Model Analysis
DOWNLOAD
Author : Jiang Jiming
language : en
Publisher: World Scientific
Release Date : 2019-04-10
Robust Mixed Model Analysis written by Jiang Jiming and has been published by World Scientific this book supported file pdf, txt, epub, kindle and other format this book has been release on 2019-04-10 with Mathematics categories.
Mixed-effects models have found broad applications in various fields. As a result, the interest in learning and using these models is rapidly growing. On the other hand, some of these models, such as the linear mixed models and generalized linear mixed models, are highly parametric, involving distributional assumptions that may not be satisfied in real-life problems. Therefore, it is important, from a practical standpoint, that the methods of inference about these models are robust to violation of model assumptions. Fortunately, there is a full scale of methods currently available that are robust in certain aspects. Learning about these methods is essential for the practice of mixed-effects models.This research monograph provides a comprehensive account of methods of mixed model analysis that are robust in various aspects, such as to violation of model assumptions, or to outliers. It is suitable as a reference book for a practitioner who uses the mixed-effects models, and a researcher who studies these models. It can also be treated as a graduate text for a course on mixed-effects models and their applications.
Robust Statistics
DOWNLOAD
Author : Ricardo A. Maronna
language : en
Publisher: John Wiley & Sons
Release Date : 2018-10-19
Robust Statistics written by Ricardo A. Maronna and has been published by John Wiley & Sons this book supported file pdf, txt, epub, kindle and other format this book has been release on 2018-10-19 with Mathematics categories.
A new edition of this popular text on robust statistics, thoroughly updated to include new and improved methods and focus on implementation of methodology using the increasingly popular open-source software R. Classical statistics fail to cope well with outliers associated with deviations from standard distributions. Robust statistical methods take into account these deviations when estimating the parameters of parametric models, thus increasing the reliability of fitted models and associated inference. This new, second edition of Robust Statistics: Theory and Methods (with R) presents a broad coverage of the theory of robust statistics that is integrated with computing methods and applications. Updated to include important new research results of the last decade and focus on the use of the popular software package R, it features in-depth coverage of the key methodology, including regression, multivariate analysis, and time series modeling. The book is illustrated throughout by a range of examples and applications that are supported by a companion website featuring data sets and R code that allow the reader to reproduce the examples given in the book. Unlike other books on the market, Robust Statistics: Theory and Methods (with R) offers the most comprehensive, definitive, and up-to-date treatment of the subject. It features chapters on estimating location and scale; measuring robustness; linear regression with fixed and with random predictors; multivariate analysis; generalized linear models; time series; numerical algorithms; and asymptotic theory of M-estimates. Explains both the use and theoretical justification of robust methods Guides readers in selecting and using the most appropriate robust methods for their problems Features computational algorithms for the core methods Robust statistics research results of the last decade included in this 2nd edition include: fast deterministic robust regression, finite-sample robustness, robust regularized regression, robust location and scatter estimation with missing data, robust estimation with independent outliers in variables, and robust mixed linear models. Robust Statistics aims to stimulate the use of robust methods as a powerful tool to increase the reliability and accuracy of statistical modelling and data analysis. It is an ideal resource for researchers, practitioners, and graduate students in statistics, engineering, computer science, and physical and social sciences.
Robust Methods In Biostatistics
DOWNLOAD
Author : Stephane Heritier
language : en
Publisher: John Wiley & Sons
Release Date : 2009-05-11
Robust Methods In Biostatistics written by Stephane Heritier and has been published by John Wiley & Sons this book supported file pdf, txt, epub, kindle and other format this book has been release on 2009-05-11 with Medical categories.
Robust statistics is an extension of classical statistics that specifically takes into account the concept that the underlying models used to describe data are only approximate. Its basic philosophy is to produce statistical procedures which are stable when the data do not exactly match the postulated models as it is the case for example with outliers. Robust Methods in Biostatistics proposes robust alternatives to common methods used in statistics in general and in biostatistics in particular and illustrates their use on many biomedical datasets. The methods introduced include robust estimation, testing, model selection, model check and diagnostics. They are developed for the following general classes of models: Linear regression Generalized linear models Linear mixed models Marginal longitudinal data models Cox survival analysis model The methods are introduced both at a theoretical and applied level within the framework of each general class of models, with a particular emphasis put on practical data analysis. This book is of particular use for research students,applied statisticians and practitioners in the health field interested in more stable statistical techniques. An accompanying website provides R code for computing all of the methods described, as well as for analyzing all the datasets used in the book.
Robust Regression
DOWNLOAD
Author : Kenneth D. Lawrence
language : en
Publisher: Routledge
Release Date : 2019-05-20
Robust Regression written by Kenneth D. Lawrence and has been published by Routledge this book supported file pdf, txt, epub, kindle and other format this book has been release on 2019-05-20 with Mathematics categories.
Robust Regression: Analysis and Applications characterizes robust estimators in terms of how much they weight each observation discusses generalized properties of Lp-estimators. Includes an algorithm for identifying outliers using least absolute value criterion in regression modeling reviews redescending M-estimators studies Li linear regression proposes the best linear unbiased estimators for fixed parameters and random errors in the mixed linear model summarizes known properties of Li estimators for time series analysis examines ordinary least squares, latent root regression, and a robust regression weighting scheme and evaluates results from five different robust ridge regression estimators.
Robust Adaptive Scheme For Linear Mixed Model
DOWNLOAD
Author : Gabriel Asare Okyere
language : en
Publisher:
Release Date : 2011
Robust Adaptive Scheme For Linear Mixed Model written by Gabriel Asare Okyere and has been published by this book supported file pdf, txt, epub, kindle and other format this book has been release on 2011 with Mathematical statistics categories.
If the underlying distribution of a statistical model is known, then a procedure which maximizes power and efficiency can be selected. For example, if the distribution of errors is known to be normal in a linear model, then inference based on least squares maximizes power and efficiency. More generally, if this distribution is known, then a rank-based inference based on the appropriate rank score function has maximum efficiency. In practice, though, this distribution is not known. Adaptive schemes are procedures which hopefully select appropriate methods to optimize the analysis. Hogg (1974) presented an adaptive rank-based scheme for testing in the simple two-sample location model. It consists of a family of distribution free tests, each associated with a rank score function, and a selection procedure which chooses a test from this family. For continuous error distributions, Hogg's scheme is valid for testing; that is, it retains the level. This, however, is not true for estimation and fitting. Little has been done to extend Hogg's scheme beyond simple location problems. In this research we extend Hogg's adaptive scheme for testing to mixed models consisting of m clusters of observations. This is an important practical family of models, including, for example, repeated measure designs, multi-center clinical designs, and randomized block designs. Under the assumption of exchangeable errors, we establish that our testing scheme is valid. In practice, though, for these models fitting is crucial. Based on the fitting of a model, a residual analysis can be performed to check, say, quality of fit and to determine outliers. Further, standard errors of the estimates can then be obtained so that confidence intervals for contrasts of interests can readily be formed. With this in mind, we have also developed several adaptive fitting schemes for these models. These are based on several types of rank-based estimation procedures, including joint ranking and multiple ranking types of fitting. Our schemes are robust and higly efficient. A large Monte Carlo study over error distributions ranging from heavy-tailed to light-tailed distributions and from symmetric distributions to skewed distributions gives empirical credence to our adaptive procedures. We illustrate our procedures with several real clinical examples.
Robust Nonparametric Statistical Methods
DOWNLOAD
Author : Thomas P. Hettmansperger
language : en
Publisher: CRC Press
Release Date : 2010-12-20
Robust Nonparametric Statistical Methods written by Thomas P. Hettmansperger and has been published by CRC Press this book supported file pdf, txt, epub, kindle and other format this book has been release on 2010-12-20 with Mathematics categories.
Presenting an extensive set of tools and methods for data analysis, Robust Nonparametric Statistical Methods, Second Edition covers univariate tests and estimates with extensions to linear models, multivariate models, times series models, experimental designs, and mixed models. It follows the approach of the first edition by developing rank-based m
Mixed Effects Models For Complex Data
DOWNLOAD
Author : Lang Wu
language : en
Publisher: CRC Press
Release Date : 2009-11-11
Mixed Effects Models For Complex Data written by Lang Wu and has been published by CRC Press this book supported file pdf, txt, epub, kindle and other format this book has been release on 2009-11-11 with Mathematics categories.
Although standard mixed effects models are useful in a range of studies, other approaches must often be used in correlation with them when studying complex or incomplete data. Mixed Effects Models for Complex Data discusses commonly used mixed effects models and presents appropriate approaches to address dropouts, missing data, measurement errors, censoring, and outliers. For each class of mixed effects model, the author reviews the corresponding class of regression model for cross-sectional data. An overview of general models and methods, along with motivating examples After presenting real data examples and outlining general approaches to the analysis of longitudinal/clustered data and incomplete data, the book introduces linear mixed effects (LME) models, generalized linear mixed models (GLMMs), nonlinear mixed effects (NLME) models, and semiparametric and nonparametric mixed effects models. It also includes general approaches for the analysis of complex data with missing values, measurement errors, censoring, and outliers. Self-contained coverage of specific topics Subsequent chapters delve more deeply into missing data problems, covariate measurement errors, and censored responses in mixed effects models. Focusing on incomplete data, the book also covers survival and frailty models, joint models of survival and longitudinal data, robust methods for mixed effects models, marginal generalized estimating equation (GEE) models for longitudinal or clustered data, and Bayesian methods for mixed effects models. Background material In the appendix, the author provides background information, such as likelihood theory, the Gibbs sampler, rejection and importance sampling methods, numerical integration methods, optimization methods, bootstrap, and matrix algebra. Failure to properly address missing data, measurement errors, and other issues in statistical analyses can lead to severely biased or misleading results. This book explores the biases that arise when naïve methods are used and shows which approaches should be used to achieve accurate results in longitudinal data analysis.
Linear And Generalized Linear Mixed Models And Their Applications
DOWNLOAD
Author : Jiming Jiang
language : en
Publisher: Springer Science & Business Media
Release Date : 2007-05-30
Linear And Generalized Linear Mixed Models And Their Applications written by Jiming Jiang and has been published by Springer Science & Business Media this book supported file pdf, txt, epub, kindle and other format this book has been release on 2007-05-30 with Mathematics categories.
This book covers two major classes of mixed effects models, linear mixed models and generalized linear mixed models. It presents an up-to-date account of theory and methods in analysis of these models as well as their applications in various fields. The book offers a systematic approach to inference about non-Gaussian linear mixed models. Furthermore, it includes recently developed methods, such as mixed model diagnostics, mixed model selection, and jackknife method in the context of mixed models. The book is aimed at students, researchers and other practitioners who are interested in using mixed models for statistical data analysis.
Generalized Linear And Mixed Models
DOWNLOAD
Author : Charles E. McCulloch
language : en
Publisher: John Wiley & Sons
Release Date : 2011-09-20
Generalized Linear And Mixed Models written by Charles E. McCulloch and has been published by John Wiley & Sons this book supported file pdf, txt, epub, kindle and other format this book has been release on 2011-09-20 with Social Science categories.
An accessible and self-contained introduction to statistical models-now in a modernized new edition Generalized, Linear, and Mixed Models, Second Edition provides an up-to-date treatment of the essential techniques for developing and applying a wide variety of statistical models. The book presents thorough and unified coverage of the theory behind generalized, linear, and mixed models and highlights their similarities and differences in various construction, application, and computational aspects. A clear introduction to the basic ideas of fixed effects models, random effects models, and mixed models is maintained throughout, and each chapter illustrates how these models are applicable in a wide array of contexts. In addition, a discussion of general methods for the analysis of such models is presented with an emphasis on the method of maximum likelihood for the estimation of parameters. The authors also provide comprehensive coverage of the latest statistical models for correlated, non-normally distributed data. Thoroughly updated to reflect the latest developments in the field, the Second Edition features: A new chapter that covers omitted covariates, incorrect random effects distribution, correlation of covariates and random effects, and robust variance estimation A new chapter that treats shared random effects models, latent class models, and properties of models A revised chapter on longitudinal data, which now includes a discussion of generalized linear models, modern advances in longitudinal data analysis, and the use between and within covariate decompositions Expanded coverage of marginal versus conditional models Numerous new and updated examples With its accessible style and wealth of illustrative exercises, Generalized, Linear, and Mixed Models, Second Edition is an ideal book for courses on generalized linear and mixed models at the upper-undergraduate and beginning-graduate levels. It also serves as a valuable reference for applied statisticians, industrial practitioners, and researchers.
Multivariate Generalized Linear Mixed Models Using R
DOWNLOAD
Author : Damon Mark Berridge
language : en
Publisher: CRC Press
Release Date : 2011-04-25
Multivariate Generalized Linear Mixed Models Using R written by Damon Mark Berridge and has been published by CRC Press this book supported file pdf, txt, epub, kindle and other format this book has been release on 2011-04-25 with Mathematics categories.
Multivariate Generalized Linear Mixed Models Using R presents robust and methodologically sound models for analyzing large and complex data sets, enabling readers to answer increasingly complex research questions. The book applies the principles of modeling to longitudinal data from panel and related studies via the Sabre software package in R.A Un