Robust Model Selection
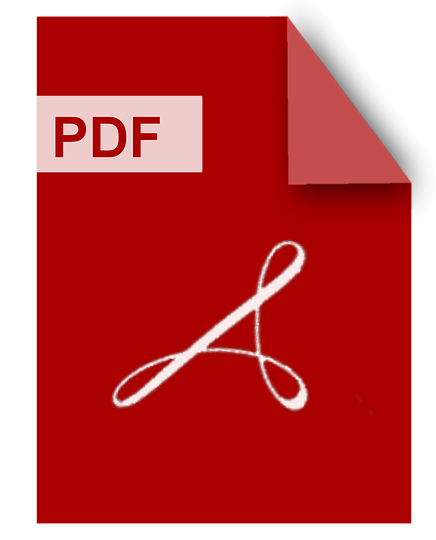
DOWNLOAD
Download Robust Model Selection PDF/ePub or read online books in Mobi eBooks. Click Download or Read Online button to get Robust Model Selection book now. This website allows unlimited access to, at the time of writing, more than 1.5 million titles, including hundreds of thousands of titles in various foreign languages. If the content not found or just blank you must refresh this page
Robust Model Selection In Regression
DOWNLOAD
Author : E. Ronchetti
language : en
Publisher:
Release Date : 1984
Robust Model Selection In Regression written by E. Ronchetti and has been published by this book supported file pdf, txt, epub, kindle and other format this book has been release on 1984 with categories.
A robust version of Akaike's model selection procedure for regression models is introduced and its relationship with robust testing procedures is discussed. (Author).
Essays On Robust Model Selection And Model Averaging For Linear Models
DOWNLOAD
Author : Le Chang
language : en
Publisher:
Release Date : 2017
Essays On Robust Model Selection And Model Averaging For Linear Models written by Le Chang and has been published by this book supported file pdf, txt, epub, kindle and other format this book has been release on 2017 with categories.
Model selection is central to all applied statistical work. Selecting the variables for use in a regression model is one important example of model selection. This thesis is a collection of essays on robust model selection procedures and model averaging for linear regression models. In the first essay, we propose robust Akaike information criteria (AIC) for MM-estimation and an adjusted robust scale based AIC for M and MM-estimation. Our proposed model selection criteria can maintain their robust properties in the presence of a high proportion of outliers and the outliers in the covariates. We compare our proposed criteria with other robust model selection criteria discussed in previous literature. Our simulation studies demonstrate a significant outperformance of robust AIC based on MM-estimation in the presence of outliers in the covariates. The real data example also shows a better performance of robust AIC based on MM-estimation. The second essay focuses on robust versions of the "Least Absolute Shrinkage and Selection Operator" (lasso). The adaptive lasso is a method for performing simultaneous parameter estimation and variable selection. The adaptive weights used in its penalty term mean that the adaptive lasso achieves the oracle property. In this essay, we propose an extension of the adaptive lasso named the Tukey-lasso. By using Tukey's biweight criterion, instead of squared loss, the Tukey-lasso is resistant to outliers in both the response and covariates. Importantly, we demonstrate that the Tukey-lasso also enjoys the oracle property. A fast accelerated proximal gradient (APG) algorithm is proposed and implemented for computing the Tukey-lasso. Our extensive simulations show that the Tukey-lasso, implemented with the APG algorithm, achieves very reliable results, including for high-dimensional data where p>n. In the presence of outliers, the Tukey-lasso is shown to offer substantial improvements in performance compared to the adaptive lasso and other robust implementations of the lasso. Real data examples further demonstrate the utility of the Tukey-lasso. In many statistical analyses, a single model is used for statistical inference, ignoring the process that leads to the model being selected. To account for this model uncertainty, many model averaging procedures have been proposed. In the last essay, we propose an extension of a bootstrap model averaging approach, called bootstrap lasso averaging (BLA). BLA utilizes the lasso for model selection. This is in contrast to other forms of bootstrap model averaging that use AIC or Bayesian information criteria (BIC). The use of the lasso improves the computation speed and allows BLA to be applied even when the number of variables p is larger than the sample size n. Extensive simulations confirm that BLA has outstanding finite sample performance, in terms of both variable and prediction accuracies, compared with traditional model selection and model averaging methods. Several real data examples further demonstrate an improved out-of-sample predictive performance of BLA.
The Robustness Of Model Selection Rules
DOWNLOAD
Author : Jochen A. Jungeilges
language : en
Publisher: LIT Verlag Münster
Release Date : 1992
The Robustness Of Model Selection Rules written by Jochen A. Jungeilges and has been published by LIT Verlag Münster this book supported file pdf, txt, epub, kindle and other format this book has been release on 1992 with Business & Economics categories.
Improving Robust Model Selection Tests For Dynamic Models
DOWNLOAD
Author : Hwan-sik Choi
language : en
Publisher:
Release Date : 2010
Improving Robust Model Selection Tests For Dynamic Models written by Hwan-sik Choi and has been published by this book supported file pdf, txt, epub, kindle and other format this book has been release on 2010 with categories.
We propose an improved model selection test for dynamic models based on the method of Rivers and Vuong (2002) using a new asymptotic approximation to the sampling distribution of a new test statistic. The model selection test is applicable to dynamic models with very general selection criteria and estimation methods. Since our test statistic does not assume the exact form of a true model, the test is essentially nonparametric once competing models are estimated. For the unkown serial correlation in data, we use a Heteroskedasticity/Autocorrelation Consistent (HAC) variance estimator, and the sampling distribution of the test statistic is approximated by the fixed-b asymptotic approximation (Kiefer and Vogelsang (2005)). The asymptotic approximation depends on kernel functions and bandwidth parameters used in HAC estimators. We compare the finite sample performance of the new test with the bootstrap methods as well as with the standard normal approximations, and show that the fixed-b asymptotics and the bootstrap methods are markedly superior to the standard normal approximation for a moderate sample size for time series data. An empirical application for foreign exchange rate forecasting models is presented, and the result shows the normal approximation to the distribution of the test statistic considered appears to overstate the data's ability to distinguish between two competing models.
Robust Model Selection In Dynamic Models With An Application To Comparing Predictive Accuracy
DOWNLOAD
Author : Nicholas M. Kiefer
language : en
Publisher:
Release Date : 2006
Robust Model Selection In Dynamic Models With An Application To Comparing Predictive Accuracy written by Nicholas M. Kiefer and has been published by this book supported file pdf, txt, epub, kindle and other format this book has been release on 2006 with categories.
A model selection procedure based on a general criterion function, with an example of the Kullback-Leibler Information Criterion (KLIC) using quasi-likelihood functions, is considered for dynamic non-nested models. We propose a robust test which generalizes Lien and Vuong's (1987) test with a Heteroscadasticity/Autocorrelation (HAC) variance estimator. We use the fixed-b asymptotics developed in Kiefer and Vogelsang (2005) to improve the asymptotic approximation to the sampling distribution of the test statistic. The fixed-b approach is compared with a bootstrap method and the standard normal approximation in Monte Carlo simulations. The fixed-b asymptotics and the bootstrap method are found to be markedly superior to the standard normal approximation. An empirical application for foreign exchange rate forecasting models is presented.
The Quest For Robust Model Selection Methods In Linear Regression
DOWNLOAD
Author : Prakash Borpatra Gohain
language : en
Publisher:
Release Date : 2022
The Quest For Robust Model Selection Methods In Linear Regression written by Prakash Borpatra Gohain and has been published by this book supported file pdf, txt, epub, kindle and other format this book has been release on 2022 with categories.
Robust Model Selection And Outlier Detection In Linear Regressions
DOWNLOAD
Author : Lauren McCann (Ph. D.)
language : en
Publisher:
Release Date : 2006
Robust Model Selection And Outlier Detection In Linear Regressions written by Lauren McCann (Ph. D.) and has been published by this book supported file pdf, txt, epub, kindle and other format this book has been release on 2006 with categories.
(cont.) Finally, we discuss the problem of outlier detection. In addition to model selection, outliers can adversely influence many other outcomes of regression-based data analysis. We describe a new outlier diagnostic tool, which we call diagnostic data traces. This tool can be used to detect outliers and study their influence on a variety of regression statistics. We demonstrate our tool on several data sets, which are considered benchmarks in the field of outlier detection.
Robust Model Selection In Regression Via Weighted Likelihood Methodology
DOWNLOAD
Author : Claudio Agostinelli
language : en
Publisher:
Release Date : 1999
Robust Model Selection In Regression Via Weighted Likelihood Methodology written by Claudio Agostinelli and has been published by this book supported file pdf, txt, epub, kindle and other format this book has been release on 1999 with categories.
On Robust Model Selection Within The Cox Model
DOWNLOAD
Author : Tadeusz Bednarski
language : en
Publisher:
Release Date : 2007
On Robust Model Selection Within The Cox Model written by Tadeusz Bednarski and has been published by this book supported file pdf, txt, epub, kindle and other format this book has been release on 2007 with categories.
Model selection methods have shown to be useful in the process of econometric modelling. The paper studies robust Akaike-Schwarz type information criteria of model choice within the Cox model. The criteria are based on a smooth modification of the partial likelihood function. Apart from asymptotic results, a Monte Carlo study is presented, which shows the finite sample behaviour of the procedure under discrepancies from the Cox model. Analysis of a real unemployment data case is also included.
Robust Model Selection By Cross Validation Via Weighted Likelihood Methodology
DOWNLOAD
Author : Claudio Agostinelli
language : en
Publisher:
Release Date : 1999
Robust Model Selection By Cross Validation Via Weighted Likelihood Methodology written by Claudio Agostinelli and has been published by this book supported file pdf, txt, epub, kindle and other format this book has been release on 1999 with categories.