The Text In The Machine
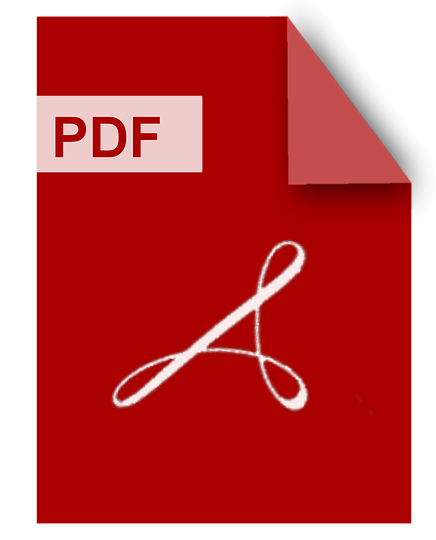
DOWNLOAD
Download The Text In The Machine PDF/ePub or read online books in Mobi eBooks. Click Download or Read Online button to get The Text In The Machine book now. This website allows unlimited access to, at the time of writing, more than 1.5 million titles, including hundreds of thousands of titles in various foreign languages. If the content not found or just blank you must refresh this page
The Text In The Machine
DOWNLOAD
Author : Toby Burrows
language : en
Publisher: CRC Press
Release Date : 1999-04-09
The Text In The Machine written by Toby Burrows and has been published by CRC Press this book supported file pdf, txt, epub, kindle and other format this book has been release on 1999-04-09 with Language Arts & Disciplines categories.
The first comprehensive guide to explore the growing field of electronic information, The Text in the Machine: Electronic Texts in the Humanities will help you create and use electronic texts. This book explains the processes involved in developing computerized books on library Web sites, CD-ROMs, or your own Web site. With the information provided by The Text in the Machine, you?ll be able to successfully transfer written words to a digitized form and increase access to any kind of information. Keeping the perspectives of scholars, students, librarians, users, and publishers in mind, this book outlines the necessary steps for electronic conversion in a comprehensive manner. The Text in the Machine addresses many variables that need to be taken into consideration to help you digitize texts, such as: defining types of markup, markup systems, and their uses identifying characteristics of the written text, such as its linguistic and physical nature, before choosing a markup scheme ensuring accuracy in electronic texts by keying in information up to three times and choosing software that is compatible with the markup systems you are using examining the best file formats for scanning written texts and converting them to digital form explaining the delivery systems available for electronic texts, such as CD-ROMs, the Internet, magnetic tape, and the variety of software that will interpret these interfaces designing the structure of electronic texts with linear presentation, segmented text, or image files to increase readability and accessibility Containing lists of suggested readings and examples of electronic text Web sites, this book provides you with the opportunity to see how other libraries and scholars are creating and publishing digital texts. From The Text in the Machine, you?ll receive the knowledge to make this medium of information accessible and beneficial to patrons and scholars around the world.
Machine Learning For Text
DOWNLOAD
Author : Charu C. Aggarwal
language : en
Publisher: Springer
Release Date : 2018-03-19
Machine Learning For Text written by Charu C. Aggarwal and has been published by Springer this book supported file pdf, txt, epub, kindle and other format this book has been release on 2018-03-19 with Computers categories.
Text analytics is a field that lies on the interface of information retrieval,machine learning, and natural language processing, and this textbook carefully covers a coherently organized framework drawn from these intersecting topics. The chapters of this textbook is organized into three categories: - Basic algorithms: Chapters 1 through 7 discuss the classical algorithms for machine learning from text such as preprocessing, similarity computation, topic modeling, matrix factorization, clustering, classification, regression, and ensemble analysis. - Domain-sensitive mining: Chapters 8 and 9 discuss the learning methods from text when combined with different domains such as multimedia and the Web. The problem of information retrieval and Web search is also discussed in the context of its relationship with ranking and machine learning methods. - Sequence-centric mining: Chapters 10 through 14 discuss various sequence-centric and natural language applications, such as feature engineering, neural language models, deep learning, text summarization, information extraction, opinion mining, text segmentation, and event detection. This textbook covers machine learning topics for text in detail. Since the coverage is extensive,multiple courses can be offered from the same book, depending on course level. Even though the presentation is text-centric, Chapters 3 to 7 cover machine learning algorithms that are often used indomains beyond text data. Therefore, the book can be used to offer courses not just in text analytics but also from the broader perspective of machine learning (with text as a backdrop). This textbook targets graduate students in computer science, as well as researchers, professors, and industrial practitioners working in these related fields. This textbook is accompanied with a solution manual for classroom teaching.
Supervised Machine Learning For Text Analysis In R
DOWNLOAD
Author : Emil Hvitfeldt
language : en
Publisher: CRC Press
Release Date : 2021-11-03
Supervised Machine Learning For Text Analysis In R written by Emil Hvitfeldt and has been published by CRC Press this book supported file pdf, txt, epub, kindle and other format this book has been release on 2021-11-03 with Computers categories.
Text data is important for many domains, from healthcare to marketing to the digital humanities, but specialized approaches are necessary to create features for machine learning from language. Supervised Machine Learning for Text Analysis in R explains how to preprocess text data for modeling, train models, and evaluate model performance using tools from the tidyverse and tidymodels ecosystem. Models like these can be used to make predictions for new observations, to understand what natural language features or characteristics contribute to differences in the output, and more. If you are already familiar with the basics of predictive modeling, use the comprehensive, detailed examples in this book to extend your skills to the domain of natural language processing. This book provides practical guidance and directly applicable knowledge for data scientists and analysts who want to integrate unstructured text data into their modeling pipelines. Learn how to use text data for both regression and classification tasks, and how to apply more straightforward algorithms like regularized regression or support vector machines as well as deep learning approaches. Natural language must be dramatically transformed to be ready for computation, so we explore typical text preprocessing and feature engineering steps like tokenization and word embeddings from the ground up. These steps influence model results in ways we can measure, both in terms of model metrics and other tangible consequences such as how fair or appropriate model results are.
Handbook Of Research On Emerging Trends And Applications Of Machine Learning
DOWNLOAD
Author : Solanki, Arun
language : en
Publisher: IGI Global
Release Date : 2019-12-13
Handbook Of Research On Emerging Trends And Applications Of Machine Learning written by Solanki, Arun and has been published by IGI Global this book supported file pdf, txt, epub, kindle and other format this book has been release on 2019-12-13 with Computers categories.
As today’s world continues to advance, Artificial Intelligence (AI) is a field that has become a staple of technological development and led to the advancement of numerous professional industries. An application within AI that has gained attention is machine learning. Machine learning uses statistical techniques and algorithms to give computer systems the ability to understand and its popularity has circulated through many trades. Understanding this technology and its countless implementations is pivotal for scientists and researchers across the world. The Handbook of Research on Emerging Trends and Applications of Machine Learning provides a high-level understanding of various machine learning algorithms along with modern tools and techniques using Artificial Intelligence. In addition, this book explores the critical role that machine learning plays in a variety of professional fields including healthcare, business, and computer science. While highlighting topics including image processing, predictive analytics, and smart grid management, this book is ideally designed for developers, data scientists, business analysts, information architects, finance agents, healthcare professionals, researchers, retail traders, professors, and graduate students seeking current research on the benefits, implementations, and trends of machine learning.
The World Of Failing Machines
DOWNLOAD
Author : Grant Hamilton
language : en
Publisher: John Hunt Publishing
Release Date : 2016-09-30
The World Of Failing Machines written by Grant Hamilton and has been published by John Hunt Publishing this book supported file pdf, txt, epub, kindle and other format this book has been release on 2016-09-30 with Literary Criticism categories.
The World of Failing Machines offers the first full-length discussion of the relationship between speculative realism and literary criticism. In identifying some of the most significant coordinates of speculative-realist thought, this book asks what the implications might be for the study of literature. It is argued that the first casualty might well be the form of the traditional essay.
Text As Data
DOWNLOAD
Author : Justin Grimmer
language : en
Publisher: Princeton University Press
Release Date : 2022-03-29
Text As Data written by Justin Grimmer and has been published by Princeton University Press this book supported file pdf, txt, epub, kindle and other format this book has been release on 2022-03-29 with Computers categories.
A guide for using computational text analysis to learn about the social world From social media posts and text messages to digital government documents and archives, researchers are bombarded with a deluge of text reflecting the social world. This textual data gives unprecedented insights into fundamental questions in the social sciences, humanities, and industry. Meanwhile new machine learning tools are rapidly transforming the way science and business are conducted. Text as Data shows how to combine new sources of data, machine learning tools, and social science research design to develop and evaluate new insights. Text as Data is organized around the core tasks in research projects using text—representation, discovery, measurement, prediction, and causal inference. The authors offer a sequential, iterative, and inductive approach to research design. Each research task is presented complete with real-world applications, example methods, and a distinct style of task-focused research. Bridging many divides—computer science and social science, the qualitative and the quantitative, and industry and academia—Text as Data is an ideal resource for anyone wanting to analyze large collections of text in an era when data is abundant and computation is cheap, but the enduring challenges of social science remain. Overview of how to use text as data Research design for a world of data deluge Examples from across the social sciences and industry
A First Course In Artificial Intelligence
DOWNLOAD
Author : Osondu Oguike
language : en
Publisher: Bentham Science Publishers
Release Date : 2021-07-14
A First Course In Artificial Intelligence written by Osondu Oguike and has been published by Bentham Science Publishers this book supported file pdf, txt, epub, kindle and other format this book has been release on 2021-07-14 with Computers categories.
The importance of Artificial Intelligence cannot be over-emphasised in current times, where automation is already an integral part of industrial and business processes. A First Course in Artificial Intelligence is a comprehensive textbook for beginners which covers all the fundamentals of Artificial Intelligence. Seven chapters (divided into thirty-three units) introduce the student to key concepts of the discipline in simple language, including expert system, natural language processing, machine learning, machine learning applications, sensory perceptions (computer vision, tactile perception) and robotics. Each chapter provides information in separate units about relevant history, applications, algorithm and programming with relevant case studies and examples. The simplified approach to the subject enables beginners in computer science who have a basic knowledge of Java programming to easily understand the contents. The text also introduces Python programming language basics, with demonstrations of natural language processing. It also introduces readers to the Waikato Environment for Knowledge Analysis (WEKA), as a tool for machine learning. The book is suitable for students and teachers involved in introductory courses in undergraduate and diploma level courses which have appropriate modules on artificial intelligence.
Knowledge Acquisition From Text And Pictures
DOWNLOAD
Author : H. Mandl
language : en
Publisher: Elsevier
Release Date : 1989-04-01
Knowledge Acquisition From Text And Pictures written by H. Mandl and has been published by Elsevier this book supported file pdf, txt, epub, kindle and other format this book has been release on 1989-04-01 with Language Arts & Disciplines categories.
Media-didactics have recently become more firmly grounded on cognitive theory, with an increasing concern for the internal processes of knowledge representation and acquisition. With this cognitive aspect in mind, an international group of researchers held a meeting in Tübingen, Federal Republic of Germany, to present and discuss the theoretical approaches to and empirical investigations of knowledge acquisition from text and pictures. This volume contains the revised contributions resulting from that meeting.
Machine Learning And Principles And Practice Of Knowledge Discovery In Databases
DOWNLOAD
Author : Irena Koprinska
language : en
Publisher: Springer Nature
Release Date : 2023-01-30
Machine Learning And Principles And Practice Of Knowledge Discovery In Databases written by Irena Koprinska and has been published by Springer Nature this book supported file pdf, txt, epub, kindle and other format this book has been release on 2023-01-30 with Computers categories.
This volume constitutes the papers of several workshops which were held in conjunction with the International Workshops of ECML PKDD 2022 on Machine Learning and Principles and Practice of Knowledge Discovery in Databases, ECML PKDD 2022, held in Grenoble, France, during September 19–23, 2022. The 73 revised full papers and 6 short papers presented in this book were carefully reviewed and selected from 143 submissions. ECML PKDD 2022 presents the following workshops: Workshop on Data Science for Social Good (SoGood 2022) Workshop on New Frontiers in Mining Complex Patterns (NFMCP 2022) Workshop on Explainable Knowledge Discovery in Data Mining (XKDD 2022) Workshop on Uplift Modeling (UMOD 2022) Workshop on IoT, Edge and Mobile for Embedded Machine Learning (ITEM 2022) Workshop on Mining Data for Financial Application (MIDAS 2022) Workshop on Machine Learning for Cybersecurity (MLCS 2022) Workshop on Machine Learning for Buildings Energy Management (MLBEM 2022) Workshop on Machine Learning for Pharma and Healthcare Applications (PharML 2022) Workshop on Data Analysis in Life Science (DALS 2022) Workshop on IoT Streams for Predictive Maintenance (IoT-PdM 2022)
Becoming A Translator For Dummies
DOWNLOAD
Author : Regina Galasso
language : en
Publisher: John Wiley & Sons
Release Date : 2024-08-20
Becoming A Translator For Dummies written by Regina Galasso and has been published by John Wiley & Sons this book supported file pdf, txt, epub, kindle and other format this book has been release on 2024-08-20 with Language Arts & Disciplines categories.
Unlock the intriguing world of translation and embark on a fulfilling career path Becoming A Translator For Dummies is your gateway to the exciting world of translation. This comprehensive guide will equip you with the tools and knowledge to succeed in this dynamic field, regardless of the specific field you choose to enter. Dr. Regina Galasso unveils the secrets of becoming a successful translator. You'll explore the different facets of translation, understand the nuances between translation and interpretation, and uncover the myriad exciting career opportunities available in this ever-expanding industry. Learn about the skills you’ll need to master to become a professional translator Explore freelance, part-time, and full-time job options, and market yourself effectively Discover specialized translation opportunities like legal, medical, technical, and literary translation Find top-notch translator programs and certifications Prepare yourself for a career that knows no boundaries! Language enthusiasts, novice translators, and those already studying to become translation pros will love the valuable insights and practical advice in Becoming A Translator For Dummies.