Uncertainty Modelling In Data Science
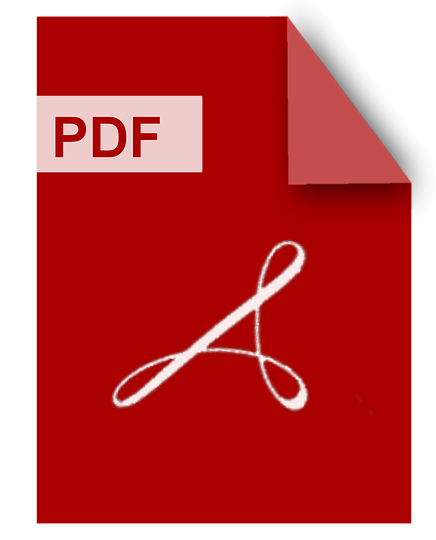
DOWNLOAD eBooks
Download Uncertainty Modelling In Data Science PDF/ePub or read online books in Mobi eBooks. Click Download or Read Online button to get Uncertainty Modelling In Data Science book now. This website allows unlimited access to, at the time of writing, more than 1.5 million titles, including hundreds of thousands of titles in various foreign languages. If the content not found or just blank you must refresh this page
Uncertainty Modelling In Data Science
DOWNLOAD eBooks
Author : Sébastien Destercke
language : en
Publisher:
Release Date : 2019
Uncertainty Modelling In Data Science written by Sébastien Destercke and has been published by this book supported file pdf, txt, epub, kindle and other format this book has been release on 2019 with Big data categories.
This book features 29 peer-reviewed papers presented at the 9th International Conference on Soft Methods in Probability and Statistics (SMPS 2018), which was held in conjunction with the 5th International Conference on Belief Functions (BELIEF 2018) in Compiègne, France on September 17-21, 2018. It includes foundational, methodological and applied contributions on topics as varied as imprecise data handling, linguistic summaries, model coherence, imprecise Markov chains, and robust optimisation. These proceedings were produced using EasyChair. Over recent decades, interest in extensions and alternatives to probability and statistics has increased significantly in diverse areas, including decision-making, data mining and machine learning, and optimisation. This interest stems from the need to enrich existing models, in order to include different facets of uncertainty, like ignorance, vagueness, randomness, conflict or imprecision. Frameworks such as rough sets, fuzzy sets, fuzzy random variables, random sets, belief functions, possibility theory, imprecise probabilities, lower previsions, and desirable gambles all share this goal, but have emerged from different needs. The advances, results and tools presented in this book are important in the ubiquitous and fast-growing fields of data science, machine learning and artificial intelligence. Indeed, an important aspect of some of the learned predictive models is the trust placed in them. Modelling the uncertainty associated with the data and the models carefully and with principled methods is one of the means of increasing this trust, as the model will then be able to distinguish between reliable and less reliable predictions. In addition, extensions such as fuzzy sets can be explicitly designed to provide interpretable predictive models, facilitating user interaction and increasing trust.--Provided by publisher.
Uncertainty Modelling In Data Science
DOWNLOAD eBooks
Author : Sébastien Destercke
language : en
Publisher: Springer
Release Date : 2018-07-24
Uncertainty Modelling In Data Science written by Sébastien Destercke and has been published by Springer this book supported file pdf, txt, epub, kindle and other format this book has been release on 2018-07-24 with Technology & Engineering categories.
This book features 29 peer-reviewed papers presented at the 9th International Conference on Soft Methods in Probability and Statistics (SMPS 2018), which was held in conjunction with the 5th International Conference on Belief Functions (BELIEF 2018) in Compiègne, France on September 17–21, 2018. It includes foundational, methodological and applied contributions on topics as varied as imprecise data handling, linguistic summaries, model coherence, imprecise Markov chains, and robust optimisation. These proceedings were produced using EasyChair. Over recent decades, interest in extensions and alternatives to probability and statistics has increased significantly in diverse areas, including decision-making, data mining and machine learning, and optimisation. This interest stems from the need to enrich existing models, in order to include different facets of uncertainty, like ignorance, vagueness, randomness, conflict or imprecision. Frameworks such as rough sets, fuzzy sets, fuzzy random variables, random sets, belief functions, possibility theory, imprecise probabilities, lower previsions, and desirable gambles all share this goal, but have emerged from different needs. The advances, results and tools presented in this book are important in the ubiquitous and fast-growing fields of data science, machine learning and artificial intelligence. Indeed, an important aspect of some of the learned predictive models is the trust placed in them. Modelling the uncertainty associated with the data and the models carefully and with principled methods is one of the means of increasing this trust, as the model will then be able to distinguish between reliable and less reliable predictions. In addition, extensions such as fuzzy sets can be explicitly designed to provide interpretable predictive models, facilitating user interaction and increasing trust.
Data Science
DOWNLOAD eBooks
Author : Ivo D. Dinov
language : en
Publisher: Walter de Gruyter GmbH & Co KG
Release Date : 2021-12-06
Data Science written by Ivo D. Dinov and has been published by Walter de Gruyter GmbH & Co KG this book supported file pdf, txt, epub, kindle and other format this book has been release on 2021-12-06 with Computers categories.
The amount of new information is constantly increasing, faster than our ability to fully interpret and utilize it to improve human experiences. Addressing this asymmetry requires novel and revolutionary scientific methods and effective human and artificial intelligence interfaces. By lifting the concept of time from a positive real number to a 2D complex time (kime), this book uncovers a connection between artificial intelligence (AI), data science, and quantum mechanics. It proposes a new mathematical foundation for data science based on raising the 4D spacetime to a higher dimension where longitudinal data (e.g., time-series) are represented as manifolds (e.g., kime-surfaces). This new framework enables the development of innovative data science analytical methods for model-based and model-free scientific inference, derived computed phenotyping, and statistical forecasting. The book provides a transdisciplinary bridge and a pragmatic mechanism to translate quantum mechanical principles, such as particles and wavefunctions, into data science concepts, such as datum and inference-functions. It includes many open mathematical problems that still need to be solved, technological challenges that need to be tackled, and computational statistics algorithms that have to be fully developed and validated. Spacekime analytics provide mechanisms to effectively handle, process, and interpret large, heterogeneous, and continuously-tracked digital information from multiple sources. The authors propose computational methods, probability model-based techniques, and analytical strategies to estimate, approximate, or simulate the complex time phases (kime directions). This allows transforming time-varying data, such as time-series observations, into higher-dimensional manifolds representing complex-valued and kime-indexed surfaces (kime-surfaces). The book includes many illustrations of model-based and model-free spacekime analytic techniques applied to economic forecasting, identification of functional brain activation, and high-dimensional cohort phenotyping. Specific case-study examples include unsupervised clustering using the Michigan Consumer Sentiment Index (MCSI), model-based inference using functional magnetic resonance imaging (fMRI) data, and model-free inference using the UK Biobank data archive. The material includes mathematical, inferential, computational, and philosophical topics such as Heisenberg uncertainty principle and alternative approaches to large sample theory, where a few spacetime observations can be amplified by a series of derived, estimated, or simulated kime-phases. The authors extend Newton-Leibniz calculus of integration and differentiation to the spacekime manifold and discuss possible solutions to some of the "problems of time". The coverage also includes 5D spacekime formulations of classical 4D spacetime mathematical equations describing natural laws of physics, as well as, statistical articulation of spacekime analytics in a Bayesian inference framework. The steady increase of the volume and complexity of observed and recorded digital information drives the urgent need to develop novel data analytical strategies. Spacekime analytics represents one new data-analytic approach, which provides a mechanism to understand compound phenomena that are observed as multiplex longitudinal processes and computationally tracked by proxy measures. This book may be of interest to academic scholars, graduate students, postdoctoral fellows, artificial intelligence and machine learning engineers, biostatisticians, econometricians, and data analysts. Some of the material may also resonate with philosophers, futurists, astrophysicists, space industry technicians, biomedical researchers, health practitioners, and the general public.
Uncertainty Modeling For Data Mining
DOWNLOAD eBooks
Author : Zengchang Qin
language : en
Publisher: Springer
Release Date : 2014-10-30
Uncertainty Modeling For Data Mining written by Zengchang Qin and has been published by Springer this book supported file pdf, txt, epub, kindle and other format this book has been release on 2014-10-30 with Computers categories.
Machine learning and data mining are inseparably connected with uncertainty. The observable data for learning is usually imprecise, incomplete or noisy. Uncertainty Modeling for Data Mining: A Label Semantics Approach introduces 'label semantics', a fuzzy-logic-based theory for modeling uncertainty. Several new data mining algorithms based on label semantics are proposed and tested on real-world datasets. A prototype interpretation of label semantics and new prototype-based data mining algorithms are also discussed. This book offers a valuable resource for postgraduates, researchers and other professionals in the fields of data mining, fuzzy computing and uncertainty reasoning. Zengchang Qin is an associate professor at the School of Automation Science and Electrical Engineering, Beihang University, China; Yongchuan Tang is an associate professor at the College of Computer Science, Zhejiang University, China.
Uncertainty Modelling And Quality Control For Spatial Data
DOWNLOAD eBooks
Author : Shi Wenzhong
language : en
Publisher: CRC Press
Release Date : 2015-11-04
Uncertainty Modelling And Quality Control For Spatial Data written by Shi Wenzhong and has been published by CRC Press this book supported file pdf, txt, epub, kindle and other format this book has been release on 2015-11-04 with Mathematics categories.
Offers New Insight on Uncertainty Modelling Focused on major research relative to spatial information, Uncertainty Modelling and Quality Control for Spatial Data introduces methods for managing uncertainties—such as data of questionable quality—in geographic information science (GIS) applications. By using original research, current advancement, and emerging developments in the field, the authors compile various aspects of spatial data quality control. From multidimensional and multi-scale data integration to uncertainties in spatial data mining, this book launches into areas that are rarely addressed. Topics covered include: New developments of uncertainty modelling, quality control of spatial data, and related research issues in spatial analysis Spatial statistical solutions in spatial data quality Eliminating systematic error in the analytical results of GIS applications A data quality perspective for GIS function workflow design Data quality in multi-dimensional integration Research challenges on data quality in the integration and analysis of data from multiple sources A new approach for imprecision management in the qualitative data warehouse A multi-dimensional quality assessment of photogrammetric and LiDAR datasets based on a vector approach An analysis on the uncertainty of multi-scale representation for street-block settlement Uncertainty Modelling and Quality Control for Spatial Data serves university students, researchers and professionals in GIS, and investigates the uncertainty modelling and quality control in multi-dimensional data integration, multi-scale data representation, national or regional spatial data products, and new spatial data mining methods.
Uncertainty Modeling In Knowledge Engineering And Decision Making
DOWNLOAD eBooks
Author :
language : en
Publisher: World Scientific
Release Date : 2012
Uncertainty Modeling In Knowledge Engineering And Decision Making written by and has been published by World Scientific this book supported file pdf, txt, epub, kindle and other format this book has been release on 2012 with Computers categories.
FLINS, originally an acronym for Fuzzy Logic and Intelligent Technologies in Nuclear Science, is now extended to Computational Intelligence for applied research. The contributions to the 10th of FLINS conference cover state-of-the-art research, development, and technology for computational intelligence systems, both from the foundations and the applications points-of-view. Sample Chapter(s). Foreword (55 KB). Evaluation of Manufacturing Technology of Photovoltaic Cells (124 KB). Contents: Decision Making and Decision Support Systems; Uncertainty Modeling; Foundations of Computational Intelligence; Statistics, Data Analysis and Data Mining; Intelligent Information Processing; Productivity and Reliability; Applied Research. Readership: Graduate students, researchers, and academics in artificial intelligence/machine learning, information management, decision sciences, databases/information sciences and fuzzy logic.
Data Science
DOWNLOAD eBooks
Author : Carlos Alberto De Bragança Pereira
language : en
Publisher: MDPI
Release Date : 2021-09-02
Data Science written by Carlos Alberto De Bragança Pereira and has been published by MDPI this book supported file pdf, txt, epub, kindle and other format this book has been release on 2021-09-02 with Technology & Engineering categories.
With the increase in data processing and storage capacity, a large amount of data is available. Data without analysis does not have much value. Thus, the demand for data analysis is increasing daily, and the consequence is the appearance of a large number of jobs and published articles. Data science has emerged as a multidisciplinary field to support data-driven activities, integrating and developing ideas, methods, and processes to extract information from data. This includes methods built from different knowledge areas: Statistics, Computer Science, Mathematics, Physics, Information Science, and Engineering. This mixture of areas has given rise to what we call Data Science. New solutions to the new problems are reproducing rapidly to generate large volumes of data. Current and future challenges require greater care in creating new solutions that satisfy the rationality for each type of problem. Labels such as Big Data, Data Science, Machine Learning, Statistical Learning, and Artificial Intelligence are demanding more sophistication in the foundations and how they are being applied. This point highlights the importance of building the foundations of Data Science. This book is dedicated to solutions and discussions of measuring uncertainties in data analysis problems.
Mathematics Of Uncertainty Modeling In The Analysis Of Engineering And Science Problems
DOWNLOAD eBooks
Author : Chakraverty, S.
language : en
Publisher: IGI Global
Release Date : 2014-01-31
Mathematics Of Uncertainty Modeling In The Analysis Of Engineering And Science Problems written by Chakraverty, S. and has been published by IGI Global this book supported file pdf, txt, epub, kindle and other format this book has been release on 2014-01-31 with Mathematics categories.
"This book provides the reader with basic concepts for soft computing and other methods for various means of uncertainty in handling solutions, analysis, and applications"--Provided by publisher.
Computer Information Systems And Industrial Management
DOWNLOAD eBooks
Author : Khalid Saeed
language : en
Publisher: Springer
Release Date : 2013-09-20
Computer Information Systems And Industrial Management written by Khalid Saeed and has been published by Springer this book supported file pdf, txt, epub, kindle and other format this book has been release on 2013-09-20 with Computers categories.
This book constitutes the proceedings of the 12th IFIP TC 8 International Conference, CISIM 2013, held in Cracow, Poland, in September 2013. The 44 papers presented in this volume were carefully reviewed and selected from over 60 submissions. They are organized in topical sections on biometric and biomedical applications; pattern recognition and image processing; various aspects of computer security, networking, algorithms, and industrial applications. The book also contains full papers of a keynote speech and the invited talk.
Uncertainty
DOWNLOAD eBooks
Author : William Briggs
language : en
Publisher: Springer
Release Date : 2016-07-15
Uncertainty written by William Briggs and has been published by Springer this book supported file pdf, txt, epub, kindle and other format this book has been release on 2016-07-15 with Mathematics categories.
This book presents a philosophical approach to probability and probabilistic thinking, considering the underpinnings of probabilistic reasoning and modeling, which effectively underlie everything in data science. The ultimate goal is to call into question many standard tenets and lay the philosophical and probabilistic groundwork and infrastructure for statistical modeling. It is the first book devoted to the philosophy of data aimed at working scientists and calls for a new consideration in the practice of probability and statistics to eliminate what has been referred to as the "Cult of Statistical Significance." The book explains the philosophy of these ideas and not the mathematics, though there are a handful of mathematical examples. The topics are logically laid out, starting with basic philosophy as related to probability, statistics, and science, and stepping through the key probabilistic ideas and concepts, and ending with statistical models. Its jargon-free approach asserts that standard methods, such as out-of-the-box regression, cannot help in discovering cause. This new way of looking at uncertainty ties together disparate fields — probability, physics, biology, the “soft” sciences, computer science — because each aims at discovering cause (of effects). It broadens the understanding beyond frequentist and Bayesian methods to propose a Third Way of modeling.